Why bigger neural networks are more intelligent | Dario Amodei and Lex Fridman
Summary
TLDRIn this discussion, the speaker explores the relationship between neural network size, data scale, and intelligence. Drawing on concepts from physics, particularly the 'one over F' noise distribution, they explain how larger networks capture a wider range of complex patterns in data. The speaker reflects on the evolution of language and its inherent structure, suggesting that bigger models can identify more nuanced, complex patterns. While recognizing the potential for AI to surpass human understanding in some domains, they also acknowledge practical limits in fields like biology due to bureaucratic processes. Ultimately, the conversation highlights both the promise and the challenges in scaling AI systems.
Takeaways
- 😀 Larger neural networks can capture more complex patterns in data, leading to improved model performance.
- 😀 The 'one over F noise' concept from physics helps explain how networks capture both common and rare patterns.
- 😀 Small networks capture basic correlations in language (e.g., sentence structure), but struggle with more complex patterns.
- 😀 As networks grow, they become capable of understanding higher-level structures, such as paragraphs and thematic content.
- 😀 There's speculation that there's no ceiling to AI's potential beyond human-level intelligence, especially in fields like biology.
- 😀 In areas like speech recognition, the ceiling of AI’s capabilities may be closer to human performance.
- 😀 Complex biological systems, such as the immune system, remain a challenge for human understanding, and AI has the potential to make significant advancements here.
- 😀 Technological progress is influenced by bureaucratic systems, such as clinical trials, which can slow down development but also protect human safety.
- 😀 The balance between advancing technology and ensuring safety is crucial; while we may be too slow at times, the need for caution is valid.
- 😀 The scaling of AI models could push the boundaries of what we can understand in various domains, especially those that humans are still exploring.
- 😀 In some domains, such as human conflict or materials science, AI might face inherent limitations in its ability to improve further.
Q & A
Why does the speaker believe that bigger models and data lead to more intelligent AI?
-The speaker argues that larger models and datasets allow AI to capture both simple and complex patterns, much like how physical systems with multiple scales produce complex distributions. A small network might only grasp basic structures, but as the model size increases, it can identify more sophisticated and rarer patterns, improving its intelligence and prediction capabilities.
What concept from physics does the speaker refer to when explaining how larger models can capture more patterns?
-The speaker refers to the concept of '1/f noise' or 'one over x distributions,' which describes natural processes with varying scales. Just as physical systems with different scales produce complex noise patterns, larger networks can capture these long-tail distributions of data, allowing them to recognize more intricate and subtle relationships.
What are the challenges that smaller networks face compared to larger networks?
-Smaller networks can capture basic patterns, like sentence structures, but they fail at understanding more complex relationships such as paragraph organization or the thematic structure of text. As networks increase in size, they begin to understand not just simple relationships but also rarer, higher-order patterns, improving their overall performance.
How does the evolution of language relate to the idea of larger models capturing complex patterns?
-Language, as an evolved process, contains both common and rare patterns. There are frequent expressions and basic structures, as well as more complex, novel ideas. Larger networks can capture the entire range of these patterns, from common to rare, which parallels how language has evolved and how AI models with more capacity can process such complexity.
What is the speaker's speculation about the ceiling for model complexity?
-The speaker speculates that there is no inherent ceiling for model complexity, particularly in areas where humans have not fully understood the complexity, such as biology. AI could potentially surpass human understanding in these fields. However, in areas with well-established processes, like speech recognition, the ceiling may align more closely with human limits.
Why does the speaker believe AI could surpass human understanding in biology?
-In biology, the speaker notes that even the most advanced human researchers struggle to understand the full complexity of systems like the immune system. AI, with its ability to process massive amounts of data, might be able to uncover insights that humans cannot easily integrate or comprehend, potentially exceeding human capabilities in this domain.
What does the speaker think about the relationship between human bureaucracy and technological advancement?
-The speaker believes that while technological advancement, particularly in fields like drug development, could be faster, human bureaucracies (such as clinical trials) serve as necessary safeguards. These institutions can slow progress but are important for protecting public health, creating a balance between innovation and safety.
What is the 'long tail' distribution mentioned in the transcript, and how does it relate to AI models?
-The 'long tail' distribution refers to a pattern where a small number of items are very common, while a large number of items are rare but still significant. In the context of AI, larger models can capture not only the common patterns but also the rarer, more complex ones that are part of the 'long tail,' improving the model's ability to predict and understand complex data.
How does the speaker describe the process of AI model scaling in terms of performance?
-The speaker suggests that as AI models scale up, they become better at capturing both simple and complex patterns. Initially, small models understand basic structure, but as the model grows, it can handle more nuanced and intricate relationships, such as thematic organization and contextual understanding in language.
What does the speaker mean when they say there is a balance between speeding up technological progress and ensuring safety?
-The speaker acknowledges that while technological progress, particularly in fields like drug development, could be faster, there must be a balance. Some human institutions, like regulatory systems, may slow down innovation, but they are crucial for ensuring safety and protecting individuals from potential risks associated with new technologies.
Outlines
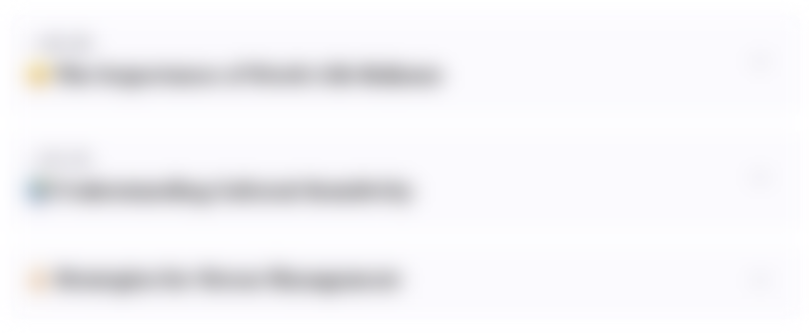
This section is available to paid users only. Please upgrade to access this part.
Upgrade NowMindmap
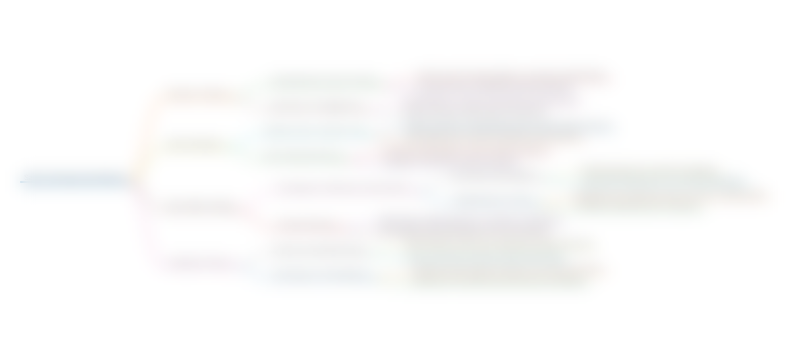
This section is available to paid users only. Please upgrade to access this part.
Upgrade NowKeywords
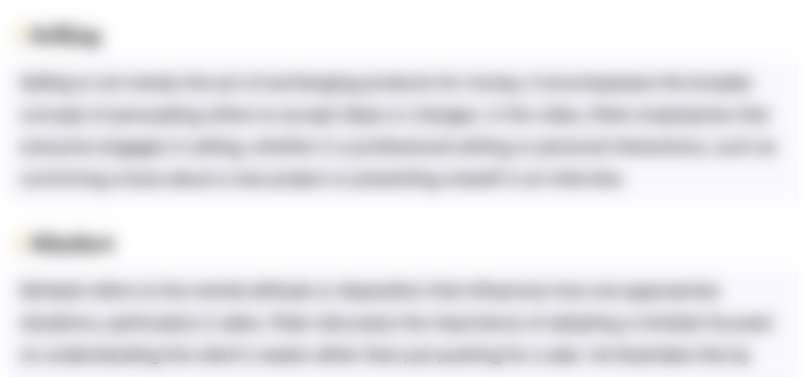
This section is available to paid users only. Please upgrade to access this part.
Upgrade NowHighlights
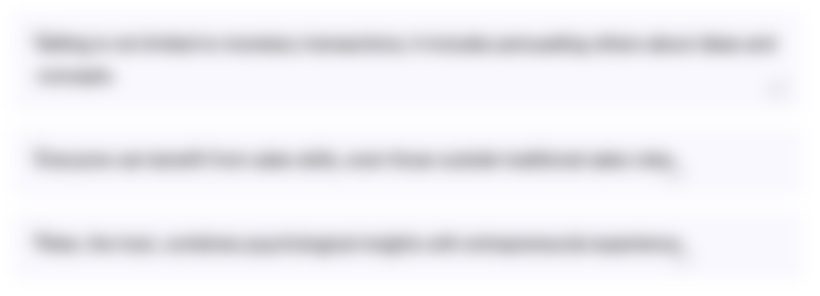
This section is available to paid users only. Please upgrade to access this part.
Upgrade NowTranscripts
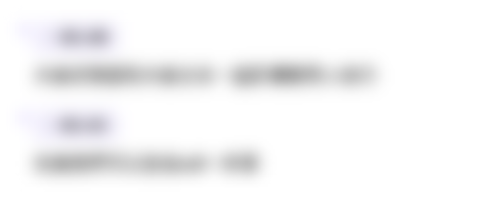
This section is available to paid users only. Please upgrade to access this part.
Upgrade NowBrowse More Related Video
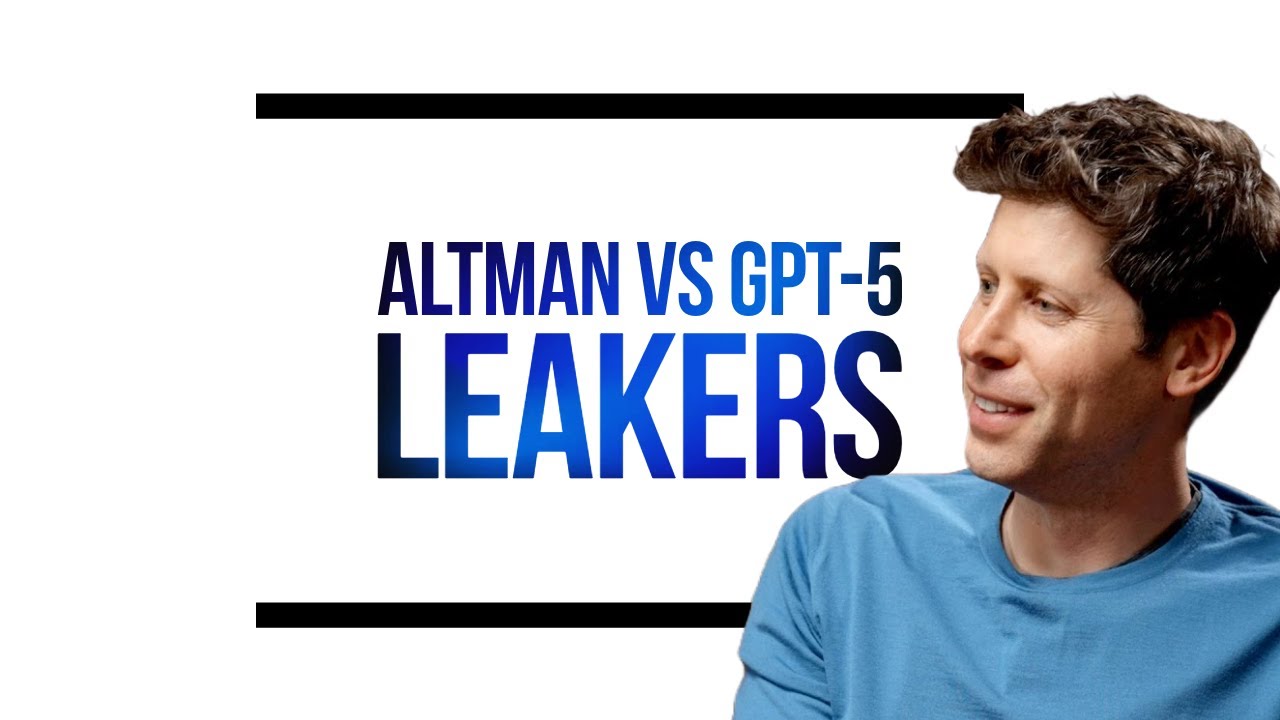
Leak: ‘GPT-5 exhibits diminishing returns’, Sam Altman: ‘lol’
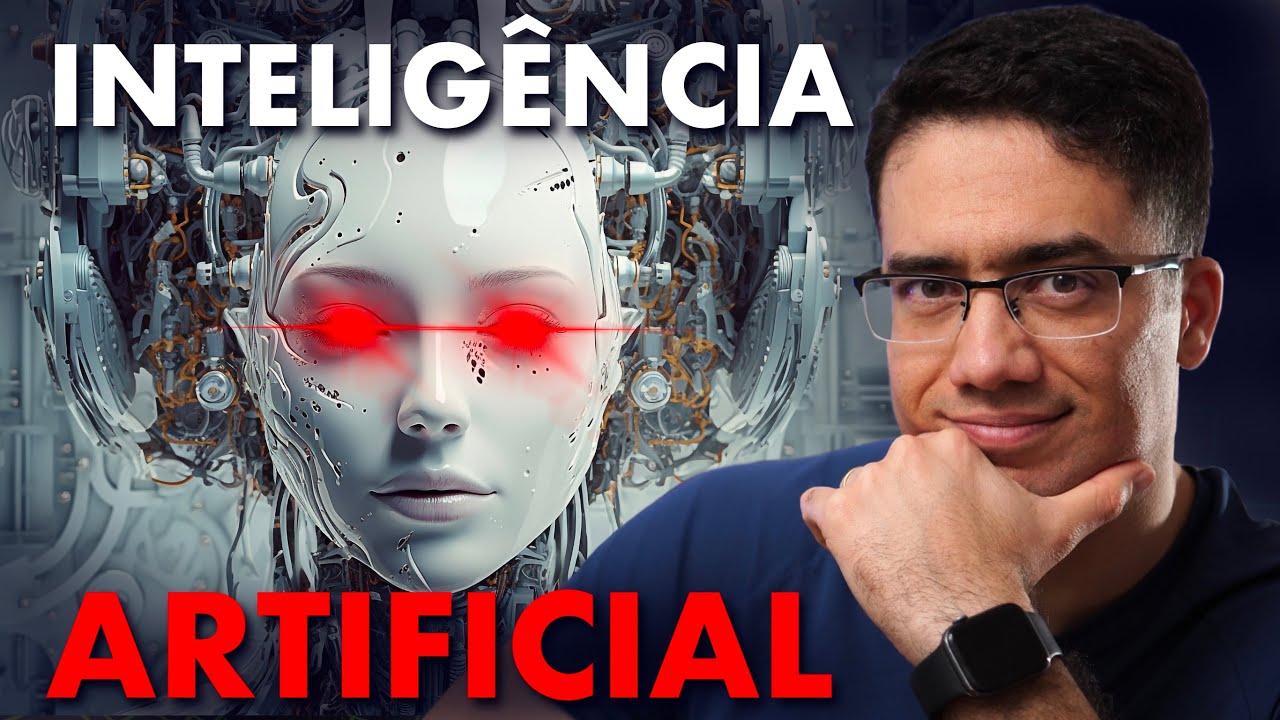
COMO a INTELIGÊNCIA ARTIFICIAL realmente FUNCIONA?
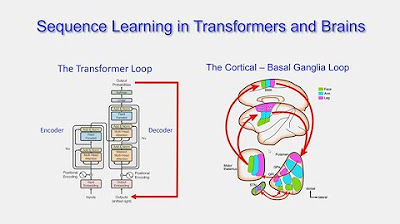
CARTA: Computational Neuroscience and Anthropogeny with Terry Sejnowski
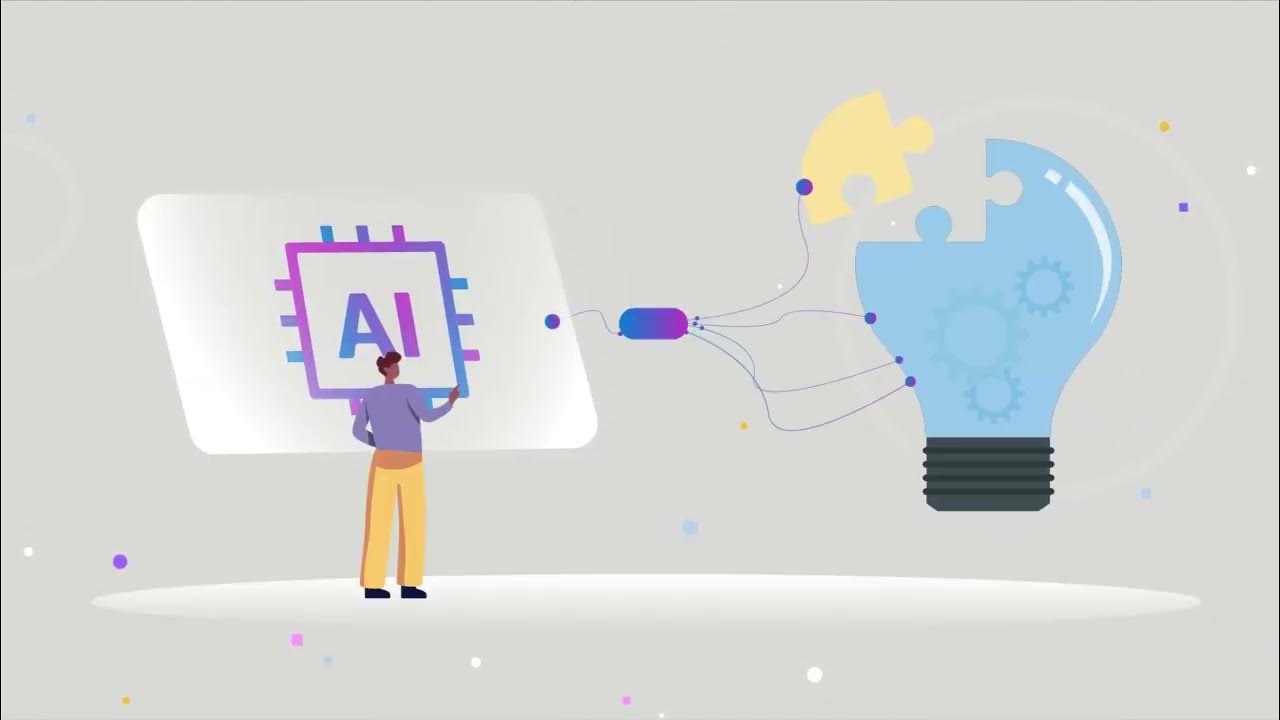
Course 1 Video 1 Introduction to AI
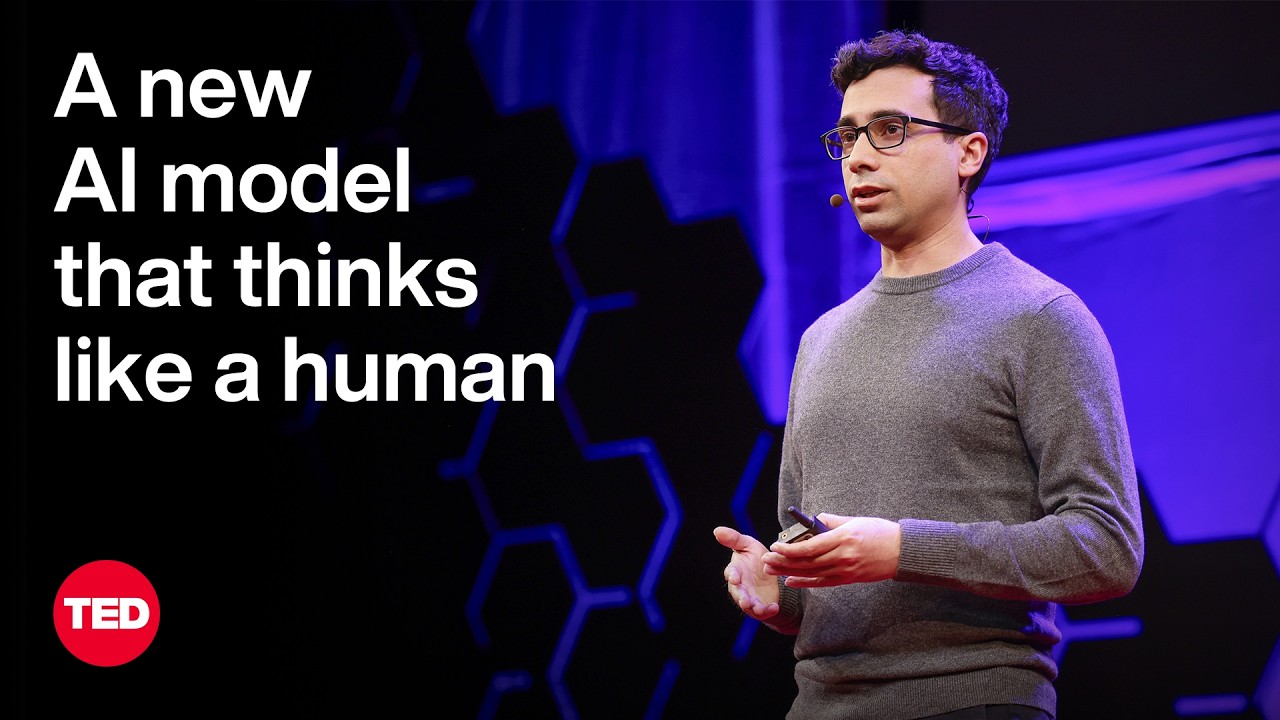
AI Won’t Plateau — if We Give It Time To Think | Noam Brown | TED
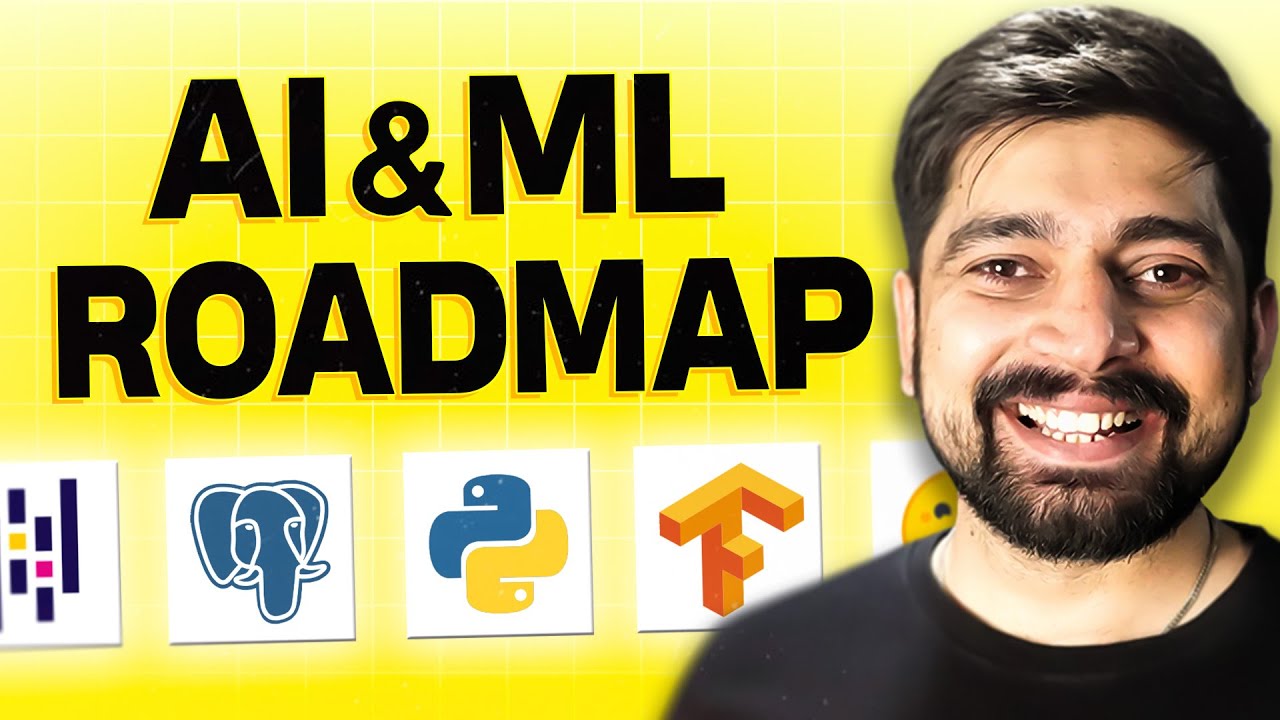
Ultimate AI ML Roadmap for beginners
5.0 / 5 (0 votes)