Introduction to Neural Networks with Example in HINDI | Artificial Intelligence
Summary
TLDRThis video introduces neural networks, a key component of artificial intelligence. It explains how artificial neural networks mimic the human brain's interconnected neurons to learn from data, enhancing recognition and processing capabilities. Unlike traditional computers, which require extensive input data and struggle with tasks like facial recognition, neural networks can adapt and improve over time. The video uses relatable examples, such as Google Assistant and facial recognition, to illustrate the advantages of neural networks. It concludes with a promise to delve deeper into neural network architectures and their functionality in future discussions.
Takeaways
- 😀 Neural networks are a fundamental concept in artificial intelligence, essential for many modern applications.
- 🤖 They function similarly to the human brain, using interconnected artificial neurons to process information.
- 💡 Unlike traditional computers, neural networks can learn from experience and adapt their outputs accordingly.
- 🔍 The human brain excels in recognizing patterns and faces, while traditional computers often struggle with such tasks without predefined data.
- 👥 Neural networks can identify individuals even with variations in their appearance, demonstrating their learning capabilities.
- 📊 The structure of neural networks includes inputs, weights, and functions that help generate outputs based on learned data.
- ⚙️ Different mathematical functions, such as linear and sigmoid, are used to process inputs and produce results in neural networks.
- 🛠️ The performance of neural networks improves as they are trained with more data, allowing for better accuracy over time.
- 🎥 Real-world applications like Google Translate and Google Assistant utilize neural networks to provide immediate and accurate responses.
- 🔄 Various architectures of neural networks exist, including single-layer, multi-layer, and recurrent networks, each serving different purposes in AI.
Q & A
What are neural networks?
-Neural networks are computational models inspired by the human brain, designed to recognize patterns and learn from data through interconnected artificial neurons.
Why are neural networks important in artificial intelligence?
-They are crucial because they enable machines to learn from experiences and improve their performance on tasks, making them suitable for various applications in AI.
How do neural networks compare to traditional computers?
-Traditional computers excel in speed and calculations, but they struggle with tasks requiring learning and adaptability, which neural networks handle effectively.
Can you provide an example of neural networks in action?
-An example is facial recognition, where a neural network can identify faces despite changes in appearance, while traditional computers would fail without specific pre-loaded data.
What role do learning algorithms play in neural networks?
-Learning algorithms allow neural networks to improve over time by training on data, enabling them to adapt and make more accurate predictions.
How do artificial neurons mimic biological neurons?
-Artificial neurons have a structure that resembles biological neurons, with inputs (dendrites), processing elements (cell body), and outputs (axons) to transmit information.
What are some common functions used in neural networks?
-Common functions include linear, sigmoid, and quadratic functions, which help in processing inputs and generating outputs based on mathematical computations.
What is the basic aim of neural networks in AI?
-The main aim is to replicate the learning capabilities of the human brain in machines, enabling them to learn from experience and improve their outputs.
How does Google Assistant utilize neural networks?
-Google Assistant uses neural networks to process user queries by picking relevant keywords and generating accurate responses based on trained data.
What are some architectures of neural networks mentioned?
-Different architectures include single-layer, multi-layer, and recurrent neural networks, each designed to handle specific types of data and tasks.
Outlines
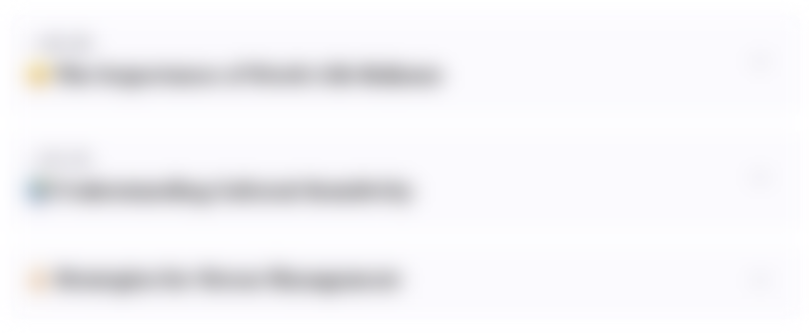
This section is available to paid users only. Please upgrade to access this part.
Upgrade NowMindmap
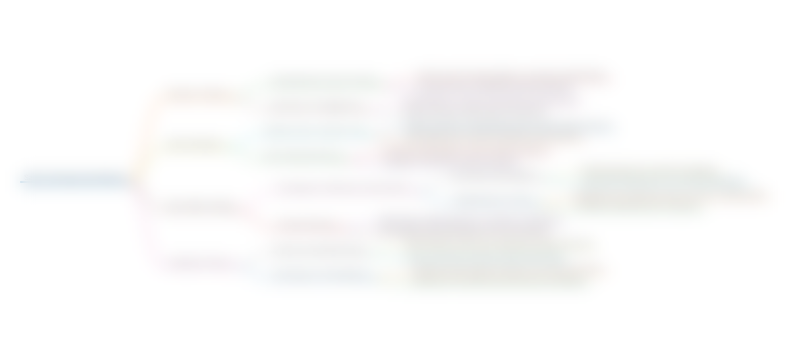
This section is available to paid users only. Please upgrade to access this part.
Upgrade NowKeywords
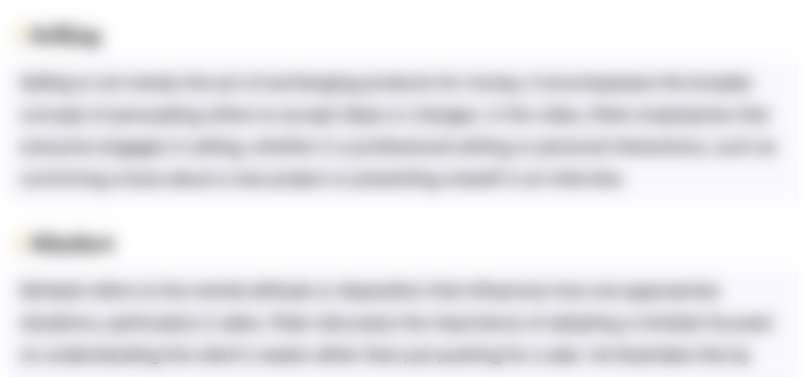
This section is available to paid users only. Please upgrade to access this part.
Upgrade NowHighlights
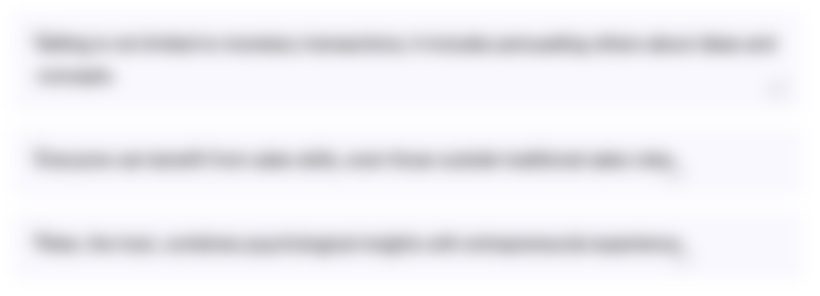
This section is available to paid users only. Please upgrade to access this part.
Upgrade NowTranscripts
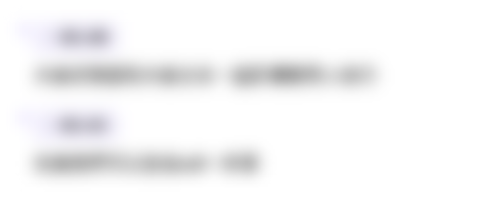
This section is available to paid users only. Please upgrade to access this part.
Upgrade NowBrowse More Related Video
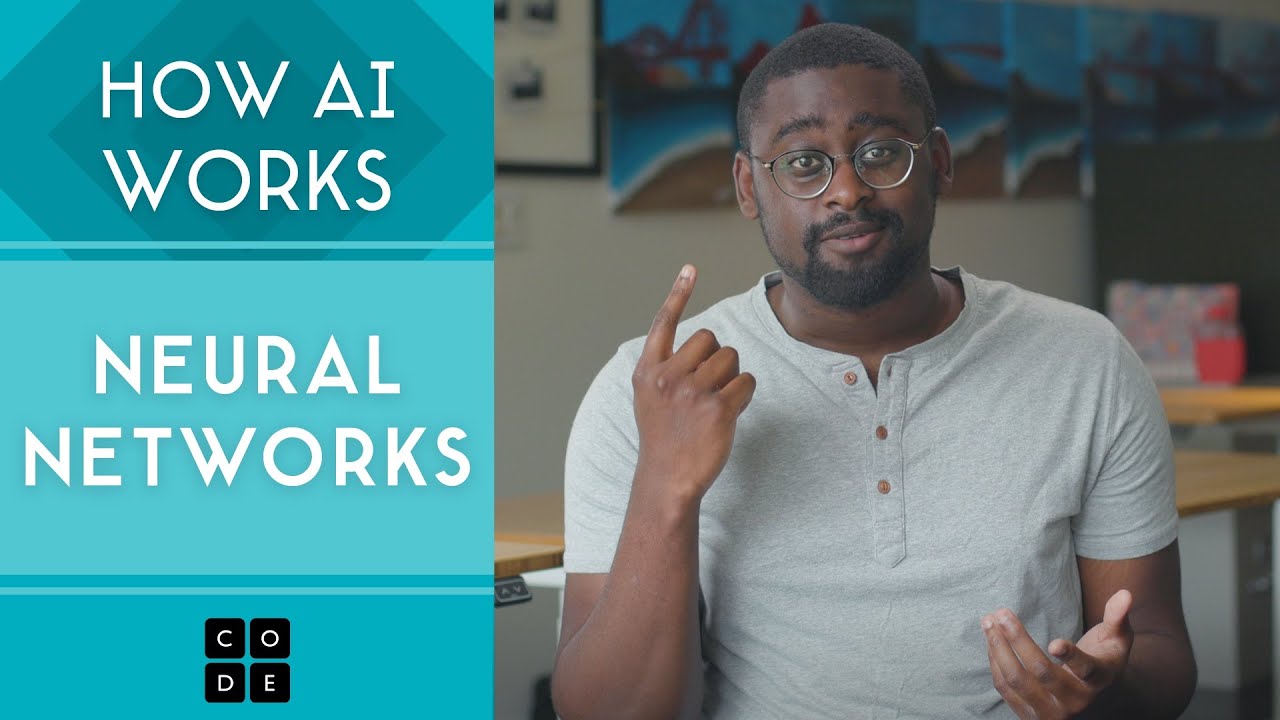
How Neural Networks Work
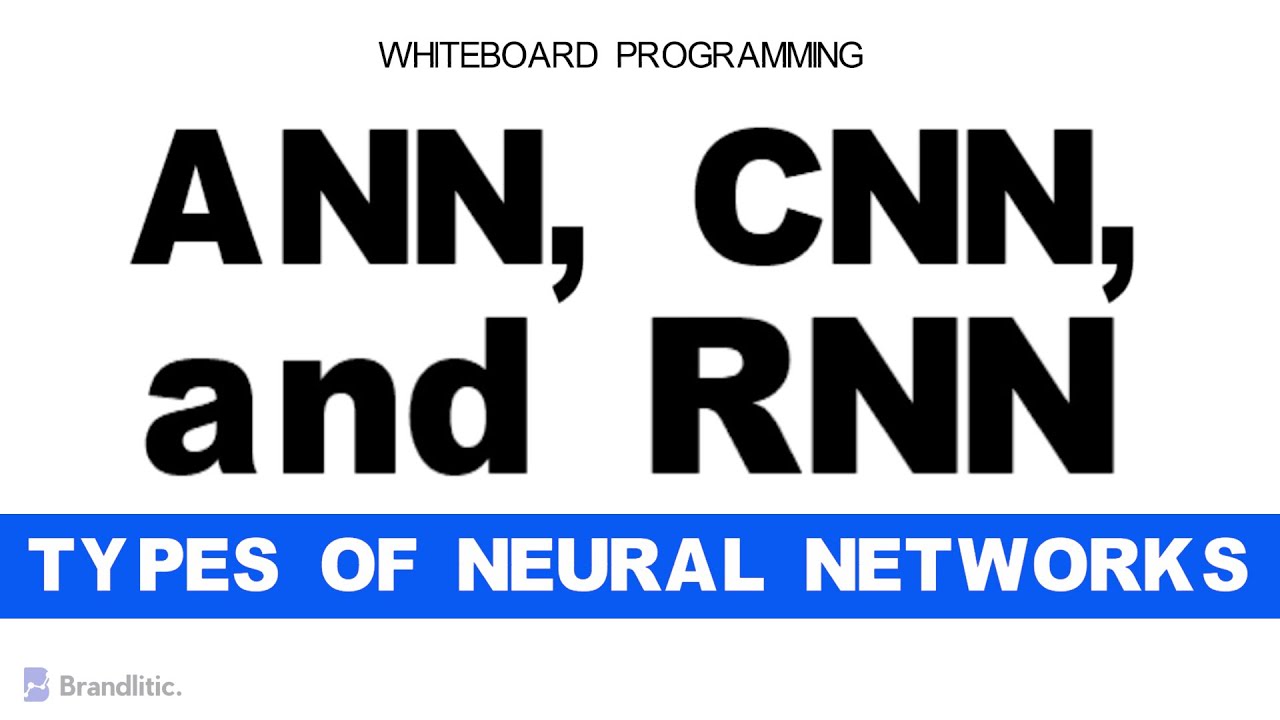
ANN vs CNN vs RNN | Difference Between ANN CNN and RNN | Types of Neural Networks Explained
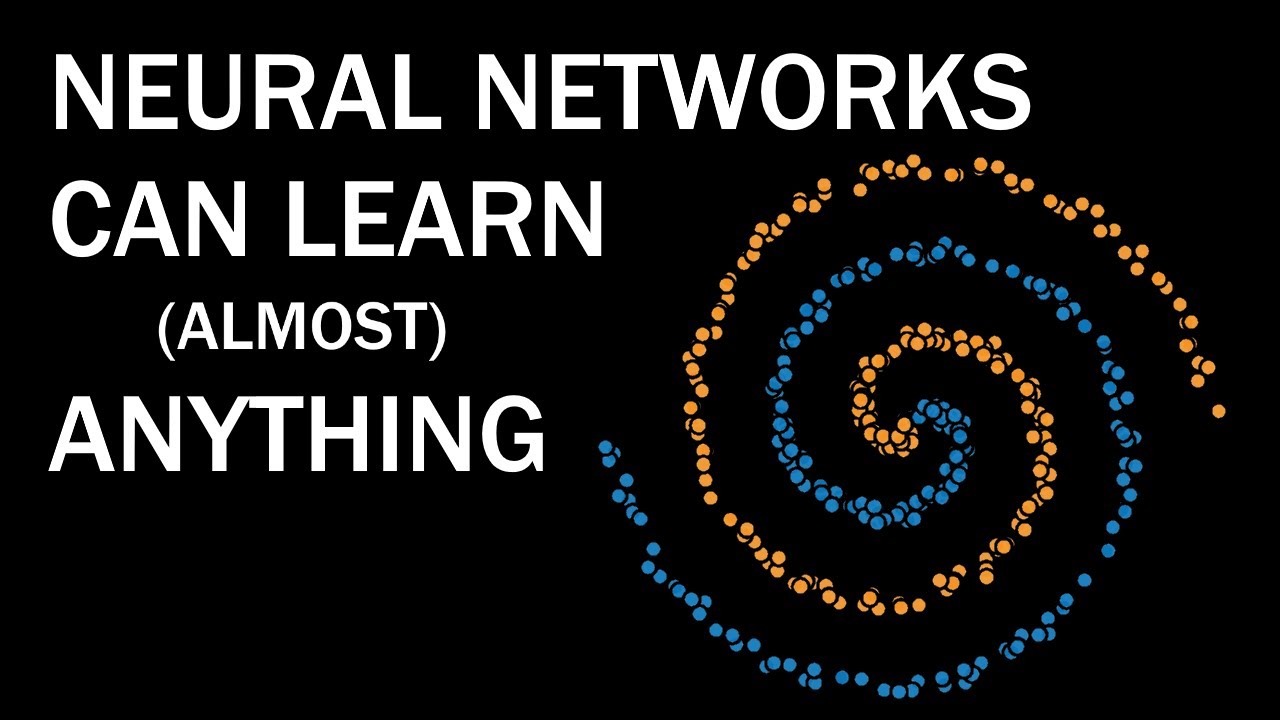
Why Neural Networks can learn (almost) anything
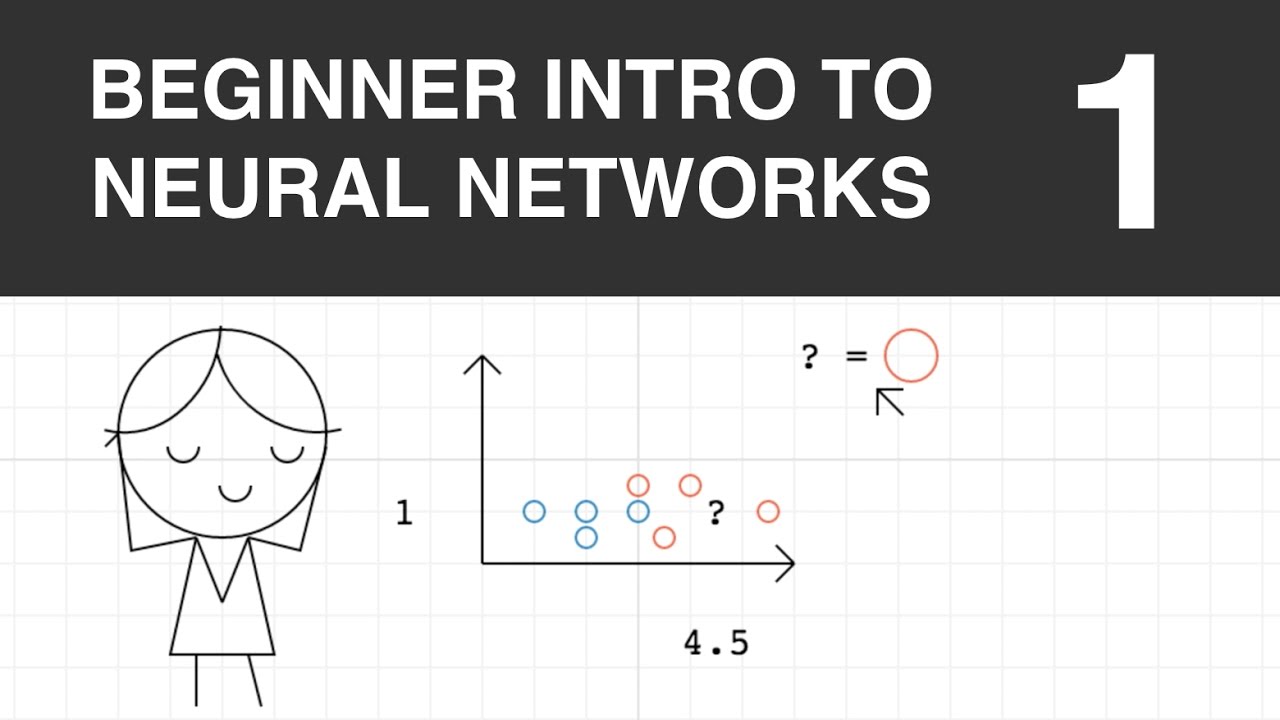
Beginner Intro to Neural Networks 1: Data and Graphing
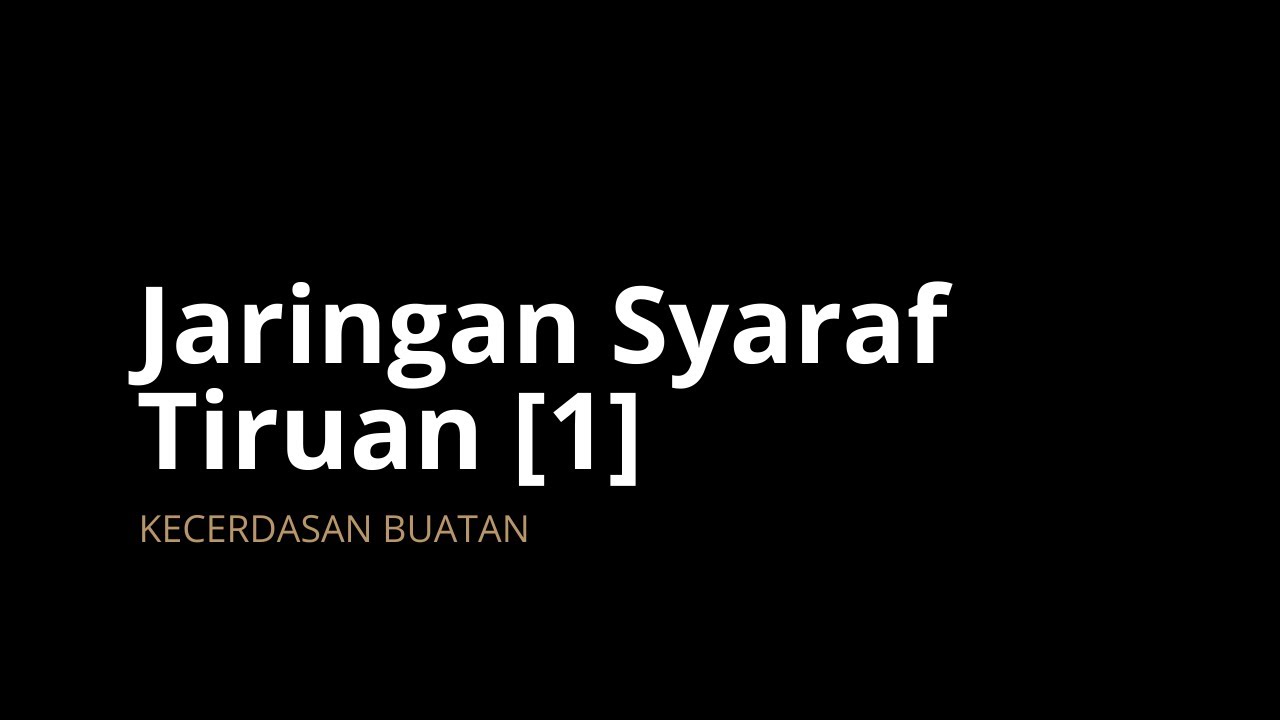
Jaringan Syaraf Tiruan [1] : Konsep Dasar JST
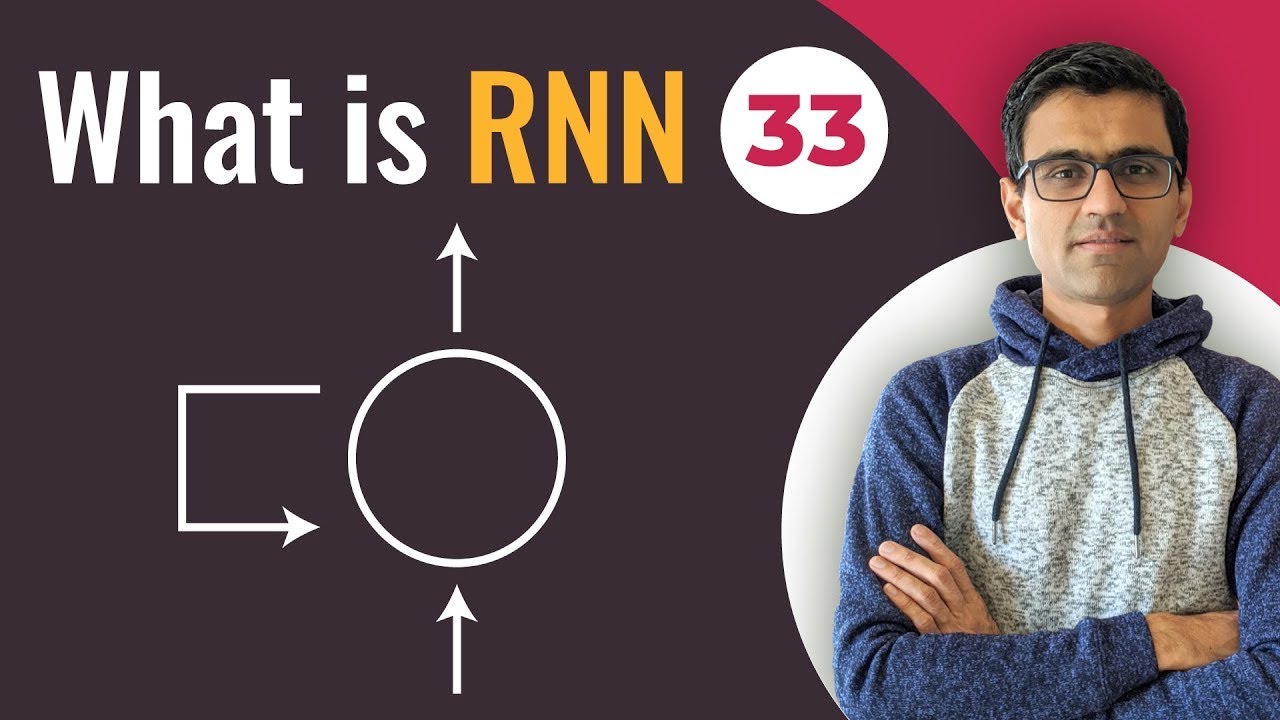
What is Recurrent Neural Network (RNN)? Deep Learning Tutorial 33 (Tensorflow, Keras & Python)
5.0 / 5 (0 votes)