Chat with Squirrel Ai Learning | ASU+GSV Summit 2024
Summary
TLDRこのスクリプトは、 scoro AIという会社が提供するAIアダプティブ学習システムについて紹介しています。scoro AIは、数学や科学など、子供たちに幅広い科目でアダプティブ学習を提供しています。彼らのプラットフォーム「L」は、学習内容の構築とハードウェアの提供を含む大きなアダプティブモデルです。その主な製品であるスマート学習タブレットは、ディストリビューターを通じて市場に販売されており、学生が自己学習を通じて学ぶためのシステムです。また、scoro AIは、中国の2,000以上のディストリビューターと60,000以上の公的な学校を支援し、2,400万人以上の学生がそのシステムを使用しているとされています。さらに、 scoro AIは、AI技術の進歩を最大限に活用し、教育の難しさに対処する取り組みを行っています。
Takeaways
- 🌟 スコロAIは、子供たちに適応型学習を提供し、数学、科学などの科目があります。
- 📚 scoro AIは、AI適応学習を9年前に開始し、今年で10周年を迎えます。
- 💡 Lという新しいプラットフォームは、大規模な適応モデルです。コンテンツビルディングとハードウェアを提供しています。
- 📈 scoro AIは、中国の2,000人のナショナルディストリビューターと60,000以上の公立学校を支援し、2,400万人以上の学生がシステムを使用しています。
- 🏆 scoro AIは、Deoの研究によりトップ50の急速成長テック企業の一つであり、MITテクノロジーレビューによって最も賢い企業の50社にランクされました。
- 📝 scoro AIは、AI適応学習と大規模モデルに関する研究で50以上の論文を発表し、さらに50を書く予定です。
- 🔍 scoro AIは、カリフォルニア大学、スタンフォード研究インstitute、中国のアカデミーと共同研究ラボを設立しています。
- 🧠 AIエージェントは、オープンAIのシステムと適応システムの間で動作し、評価、推奨、予測などの機能を提供します。
- 📊 scoro AIは、学生の学習行動データをリアルタイムで収集し、学習目標のナノレベルの分解を使用して、学生の学習ギャップを特定します。
- 🌐 scoro AIは、中国とアメリカの両方のデータセンターを使用し、データの安全性とプライバシーを重視しています。
- 🏢 scoro AIは、中国にサービスセンターを持ち、米国市場に進出し、数学のコンテンツを今年、来年は科学などの他の科目のコンテンツを構築予定です。
- 📱 スマート学習タブレットとデジタルパッドを使用して、学生は手書きの数学解答を入力する必要がなく、システムはすべての学習セッションを内部で処理します。
- 👩🏫 scoro AIの物理センターでは、デバイスの販売と学生の自己学習の監視、データ分析が行われ、オンラインスーパーバイズも提供しています。
Q & A
スクーロAIのサービスは何を提供していますか?
-スクーロAIは、子供たちに合わせた学習を提供しています。数学、科学などの科目があり、さらに他の科目にも拡大していく予定です。また、AIアダプティブ学習を用いて、学生が自分に合った学習パスを見つけることができます。
スクーロAIのプラットフォーム「L」とは何ですか?
-「L」はスクーロAIの新しいプラットフォームで、大きなアダプティブモデルをシステムに取り入れています。コンテンツのビルディングが行われ、さらにハードウェアも提供しています。
スクーロAIのスマート学習タブレットはどのような機能を備えていますか?
-スマート学習タブレットは、AIアダプティブ学習モデルを搭載し、学習コンテンツを提供します。また、ペンやスピーカーなどの周辺機器も用意されており、学習をサポートする機能が集約されています。
スクーロAIのビジネスモデルはどのようなものか説明してください。
-スクーロAIは、中国には2,000人のナショナルディストリビューターがあり、60,000以上の公立学校がアダプティブ学習システムを使用しています。また、スマート学習タブレットは過去1年間に200,000台以上の販売実績があります。
スクーロAIはどのようにして学生の学習効果を向上させていますか?
-スクーロAIは、AIアダプティブ学習を用いて学生が自分に合った学習パスを見つけることができます。また、教師の役割は、学習内容との直接的な対話よりも、監督とデータ分析に変わっています。
スクーロAIの学習システムはどのようにして学生の学習を個別化していますか?
-スクーロAIは、学生がテストを行うことで学習状態を把握し、強みや既に習得している学習目標、未習得のギャップを特定します。その後、個々の学生に合わせた学習コンテンツが提供され、学習セッションが進むごとに学習目標が動的に変化します。
スクーロAIの学習データはどのように活用されていますか?
-スクーロAIは、学生の学習データを収集し、学習行動や歴史を記録、リアルタイムでの分類と予測を行い、学習内容の最適化に活用しています。また、学習データは学生の感情的な表情や学習プロセスを理解するのに役立ちます。
スクーロAIの学習システムはどのようにして学生の間違いを特定し、フィードバックを提供していますか?
-学生が解答を提供する際には、デジタルパッドを使用して解答を記入します。システムはこれらの解答を分析し、学生が問題を正しく答えられない理由に基づいてフィードバックを提供します。また、問題解決の方法を示すビデオも提供されます。
スクーロAIはどのようにして学生の学習を監督していますか?
-スクーロAIは、学習センターでの自習を監督するとともに、オンラインでの監督も行っています。学習セッション後、スーパーバイザーは学生の進捗や学習状況をダッシュボードから確認し、必要に応じてサポートを提供します。
スクーロAIはどのようにして学生の学習効果を予測していますか?
-スクーロAIは、学生が行ったテストの結果や学習行動をもとに、学習効果を予測します。また、学習データアナリストは、データに基づいて学生が今後どれだけの学習時間を必要とするかを予測し、フィードバックを提供します。
スクーロAIの学習システムはどのようにして学生の学習目標を細分化していますか?
-スクーロAIは学習目標をナノレベルまで細分化し、学生が習得していない学習目標を特定します。これにより、学生は小さな学習目標に焦点を当て、より効率的に学習することができます。
スクーロAIはどのようにして学生が異なる科目間のつながりを学ぶことができますか?
-スクーロAIは、異なる科目間のつながりを学ぶために、学習目標の間の関係性を理解し、クロス科目の知識を組み合わせる知識グラフを用います。これにより、学生は異なる科目間のつながりを理解し、総合的な学習を深めることができます。
Outlines
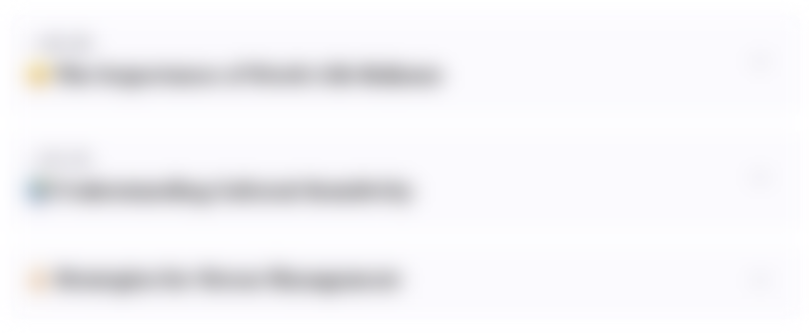
This section is available to paid users only. Please upgrade to access this part.
Upgrade NowMindmap
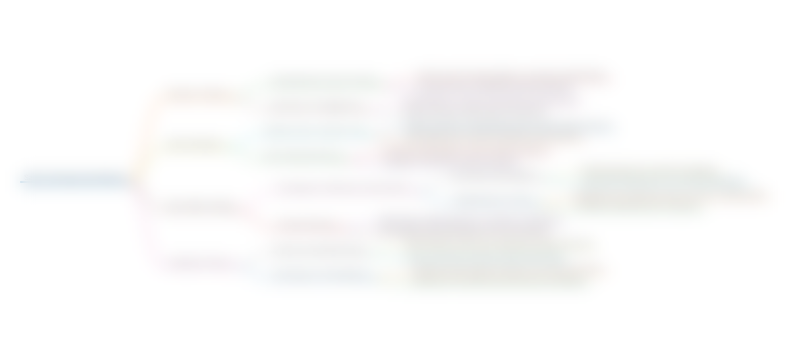
This section is available to paid users only. Please upgrade to access this part.
Upgrade NowKeywords
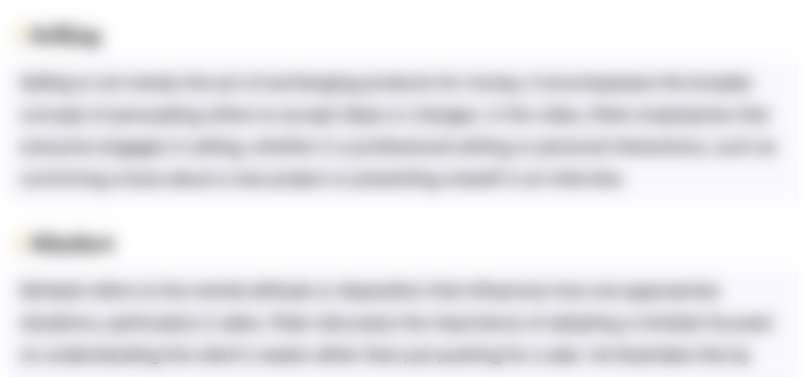
This section is available to paid users only. Please upgrade to access this part.
Upgrade NowHighlights
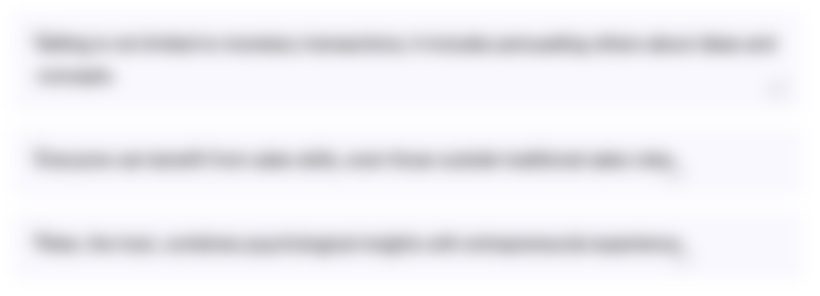
This section is available to paid users only. Please upgrade to access this part.
Upgrade NowTranscripts
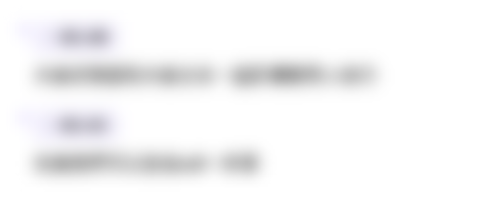
This section is available to paid users only. Please upgrade to access this part.
Upgrade Now5.0 / 5 (0 votes)