Algorithmic Bias and Fairness: Crash Course AI #18
Summary
TLDRThis CrashCourse AI episode delves into the concept of algorithmic bias, explaining how real-world biases can be mirrored or amplified by AI systems. It outlines five types of biases, including training data issues and feedback loops, and stresses the importance of transparency and critical evaluation of AI recommendations to prevent discrimination and unfair treatment. The episode encourages awareness and advocacy for ethical AI practices.
Takeaways
- 🧠 Algorithmic bias is the reflection or exaggeration of real-world biases in AI systems, which can be problematic if not acknowledged or addressed.
- 🧐 Bias itself is not inherently bad; it's a natural human tendency to find patterns, but it becomes an issue when it leads to unfair treatment of certain groups.
- 📚 Society has laws against discrimination, highlighting the importance of distinguishing between personal bias and systemic discrimination.
- 🔍 There are five main types of algorithmic bias to be aware of: training data bias, lack of diverse examples, difficulty in quantifying certain features, positive feedback loops, and intentional manipulation of training data.
- 📈 Training data can contain societal biases, such as gender stereotypes, which can be unintentionally embedded in AI systems, as seen in Google image search results.
- 🌐 Protected classes like race or gender might not be explicitly present in data, but can emerge as correlated features that influence AI predictions.
- 👥 Insufficient examples of certain classes in training data can lead to inaccurate AI predictions, as seen in facial recognition systems struggling with non-white faces.
- 📊 Quantifying complex human experiences and qualities can be challenging for AI, leading to reliance on easily measurable but less meaningful metrics.
- 🔁 Positive feedback loops in AI can perpetuate and amplify existing biases, as seen with PredPol's crime prediction algorithm and its impact on policing.
- 👾 AI systems can be manipulated, as demonstrated by Microsoft's chatbot Tay, which quickly adopted inappropriate behaviors due to user input.
- 🤖 Human oversight is crucial in AI systems to ensure fairness and adjust algorithms when necessary, emphasizing the role of transparency and critical evaluation.
Q & A
What is algorithmic bias?
-Algorithmic bias refers to the phenomenon where AI systems mimic or even exaggerate the biases that exist in the real world due to the data they are trained on or the way they are designed.
Why is it important to differentiate between bias and discrimination?
-It's important because bias is a natural human tendency to find patterns, but discrimination is an unfair treatment of certain groups which is illegal and can be prevented. Understanding this helps in addressing algorithmic bias ethically.
Can you give an example of how biases in training data can affect AI systems?
-Yes, if an AI is trained on recent news articles or books, it might associate the word 'nurse' with 'woman' and 'programmer' with 'man', reflecting societal stereotypes.
How can protected classes emerge as correlated features in AI algorithms?
-Protected classes like race or gender might not be explicitly in the data but can emerge as correlated features due to societal factors. For example, zip code can be correlated to race due to residential segregation.
What is the issue with training data not having enough examples of each class?
-If the training data lacks sufficient representation of each class, it can affect the accuracy of predictions. For instance, facial recognition AI trained mostly on white faces may have trouble recognizing people of other races.
Why is it challenging to quantify certain features in training data?
-Some features, like the quality of writing or the complexity of relationships, are difficult to quantify because they involve subjective and nuanced qualities that cannot be easily measured with numbers.
How can an algorithm create a positive feedback loop?
-A positive feedback loop occurs when the algorithm's predictions influence the data it receives, amplifying past trends. For example, PredPol's crime prediction algorithm could lead to increased police presence in certain neighborhoods based on biased historical data.
What was the issue with Microsoft's chatbot Tay?
-Tay was manipulated by a subset of people to post violent, sexist, anti-Semitic, and racist Tweets within 12 hours of its release, showing how AI can be influenced by biased input.
Why is transparency in algorithms important?
-Transparency is crucial for understanding why an algorithm makes certain recommendations, allowing us to critically assess AI outputs and ensure fairness and accuracy.
What can be done to monitor AI for bias and discrimination?
-We can start by acknowledging that algorithms will have biases, being critical of AI recommendations, and advocating for transparency and careful interpretation of algorithmic outputs to protect human rights.
What is the role of humans in ensuring fairness in AI systems?
-Humans play a vital role in monitoring, interpreting, and adjusting AI systems to ensure that recommendations are fair and not influenced by harmful biases or discrimination.
Outlines
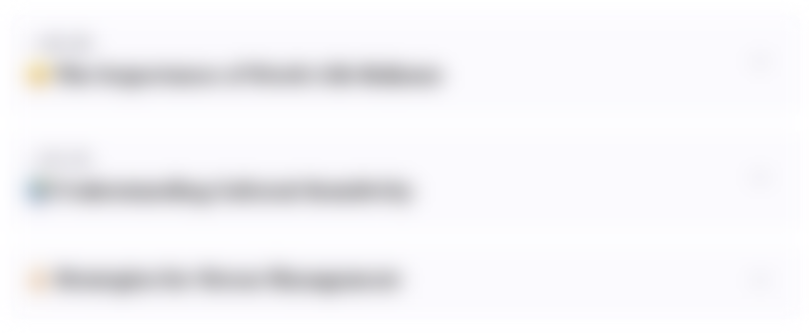
This section is available to paid users only. Please upgrade to access this part.
Upgrade NowMindmap
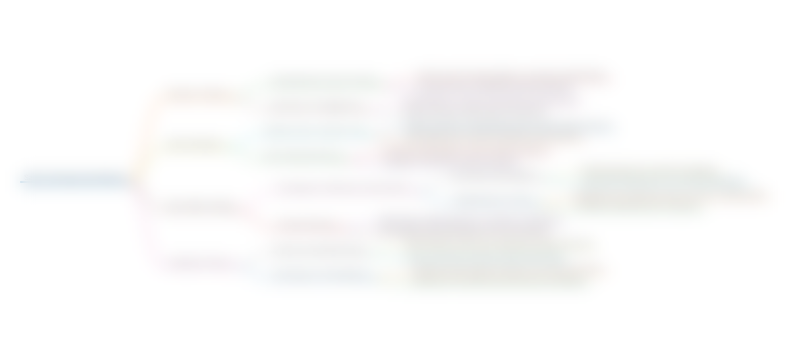
This section is available to paid users only. Please upgrade to access this part.
Upgrade NowKeywords
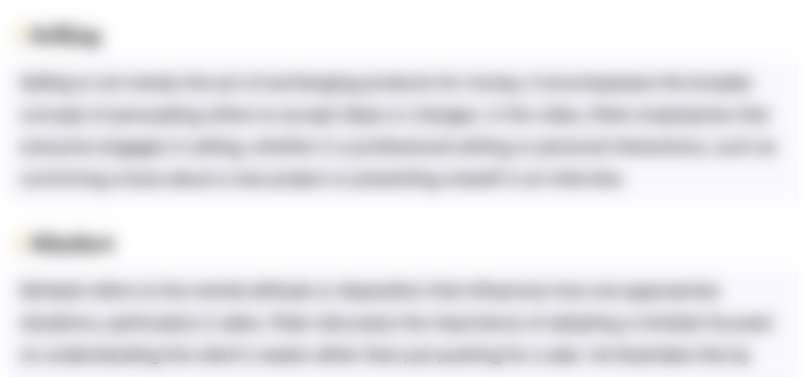
This section is available to paid users only. Please upgrade to access this part.
Upgrade NowHighlights
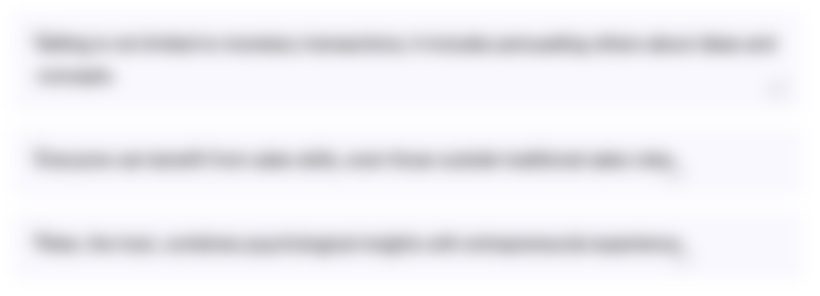
This section is available to paid users only. Please upgrade to access this part.
Upgrade NowTranscripts
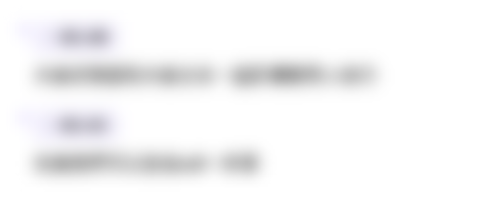
This section is available to paid users only. Please upgrade to access this part.
Upgrade Now5.0 / 5 (0 votes)