AI Leader Reveals The Future of AI AGENTS (LangChain CEO)
Summary
TLDR视频脚本讨论了智能代理(agents)的当前状态和未来发展。Lang chain的CEO和创始人Harrison Chase强调,代理不仅仅是复杂的提示(prompts),而是具有工具使用、记忆和规划等多种能力的复杂系统。他提到,通过为代理提供短期和长期记忆,以及允许它们执行计划和行动,可以显著提高代理的性能。Harrison还探讨了用户体验(UX)的重要性,强调了“人类在循环中”的必要性,以及如何通过代理框架减少幻觉(hallucinations)并提高可靠性。最后,他讨论了代理记忆的两个方面:程序记忆和个性化记忆,以及它们如何对下一代代理的发展至关重要。整个讨论突出了在构建生产就绪和现实世界中的代理时,开发者们正在努力解决的一些关键问题和挑战。
Takeaways
- 🤖 **智能代理的复杂性**:智能代理不仅仅是复杂的提示,它们能够使用工具、记忆和执行计划,远超过简单的语言模型提示。
- 🧠 **记忆的重要性**:代理拥有短期记忆和长期记忆,这对于提高代理性能至关重要,长期记忆如使用RAG技术,可以存储信息供以后使用。
- 🛠️ **工具使用**:代理可以使用各种工具,如日历、计算器、网络访问和代码解释器,这些工具极大地扩展了代理的能力。
- 📈 **性能提升**:通过添加短期和长期记忆功能,代理的性能得到了显著提升。
- 🔍 **规划与行动**:代理能够进行规划,包括自我批评、思考链分解和执行动作,这使得它们能够更有效地完成任务。
- 🔁 **循环迭代**:代理的工作方式可以被看作是在循环中运行语言模型,不断询问并执行下一步操作,直到任务完成。
- 🌐 **开发者关注点**:开发者正在将智能代理推向生产就绪和现实世界的应用,特别关注规划、用户体验和记忆。
- 📐 **流程工程**:通过设计良好的流程图或状态机,可以提高代理的效率,这需要人类工程师在开始时进行规划。
- 🔮 **未来展望**:未来的智能代理可能需要全新的架构来实现更深层次的逻辑和推理能力。
- 🤝 **协调一致性**:代理框架在协调不同模型和工具方面非常有价值,即使在未来模型能够更缓慢地思考时,这些框架仍然是必不可少的。
- 🔄 **可逆性与编辑**:用户界面设计中的可逆性和编辑能力,如Devon演示的那样,可以提高用户体验并使代理更加可靠。
- 🧵 **记忆的类型**:代理需要程序记忆(正确执行任务的记忆)和个性化记忆(关于用户的事实,用于个性化体验)。
Q & A
Harrison Chase 是谁?
-Harrison Chase 是 Lang chain 的首席执行官和创始人,Lang chain 是一个流行的编码框架,允许用户轻松地将不同的 AI 工具组合在一起。
什么是 Lang chain?
-Lang chain 是一个开发者框架,用于构建各种大型语言模型(LLM)应用程序,其中最常见的类型之一是代理(agents)。
代理(agents)是什么?
-代理是一种使用语言模型与外部世界互动的工具。它们不仅仅是复杂的提示(prompts),而是拥有访问日历、计算器、网络等工具的能力,还具备短期和长期记忆,能够进行规划和执行动作。
为什么代理不仅仅是大型语言模型的提示?
-因为代理拥有超出大型语言模型本身的能力,如使用工具、记忆和执行规划等,这些能力使得代理能够执行更复杂的任务,远不止生成文本响应。
代理中的规划是什么?
-规划是代理能够进行自我反思、提前计划、将复杂任务分解为子任务的能力,这是目前单独的大型语言模型尚不能有效执行的功能。
什么是“树状思维”(Tree of Thoughts)?
-树状思维是一种允许模型生成对提示的初始响应,然后将该响应反馈给模型并询问如何改进的方法,从而赋予模型自我反思和规划的能力。
代理框架中的“人类在循环中”(Human in the Loop)是什么?
-人类在循环中是指在代理执行任务的过程中,人类用户可以介入以提供指导或纠正,以提高代理的可靠性和输出质量。
为什么说代理框架对于协调不同的模型和工具非常有价值?
-代理框架可以帮助开发者构建工具和策略,协调不同的模型和代理,提供一致的工作流程,即使在未来模型能够更慢地思考和规划时,这些框架仍将非常有价值。
代理的用户界面(UX)设计为什么很重要?
-用户界面设计影响着用户与代理的交互方式,良好的UX设计可以提高代理的可靠性和用户的使用体验,例如提供“回放和编辑”功能,允许用户回退到代理的某个状态并进行编辑。
代理的短期记忆和长期记忆有什么区别?
-短期记忆指的是在对话或同一对话中代理之间的记忆,而长期记忆则涉及到存储以备后用的信息,如使用检索增强生成(RAG)技术。长期记忆对于个性化和企业环境中的知识保留至关重要。
为什么说记忆管理在代理中非常关键?
-记忆管理对于代理的个性化和适应性至关重要。它需要能够记住正确的操作方式和用户的个性化信息,同时也要能够随着业务需求的变化而演化和更新。
Outlines
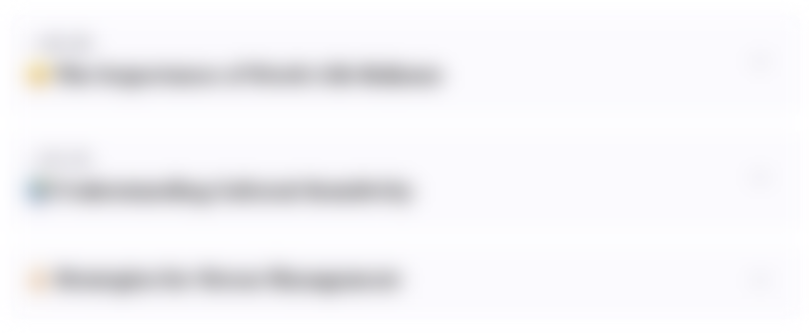
This section is available to paid users only. Please upgrade to access this part.
Upgrade NowMindmap
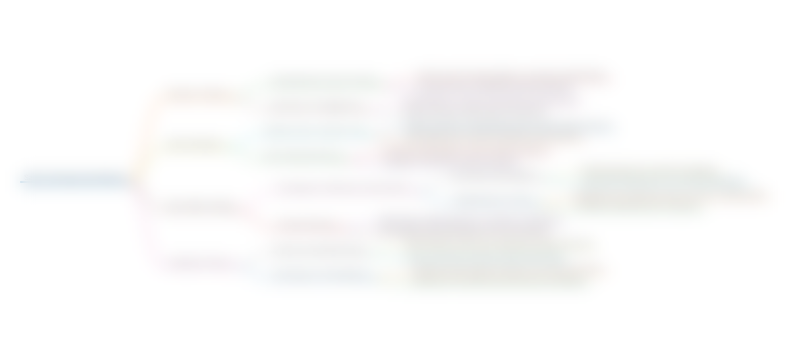
This section is available to paid users only. Please upgrade to access this part.
Upgrade NowKeywords
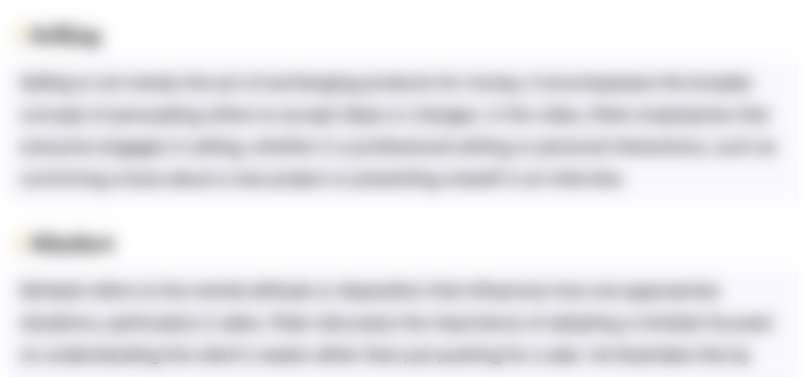
This section is available to paid users only. Please upgrade to access this part.
Upgrade NowHighlights
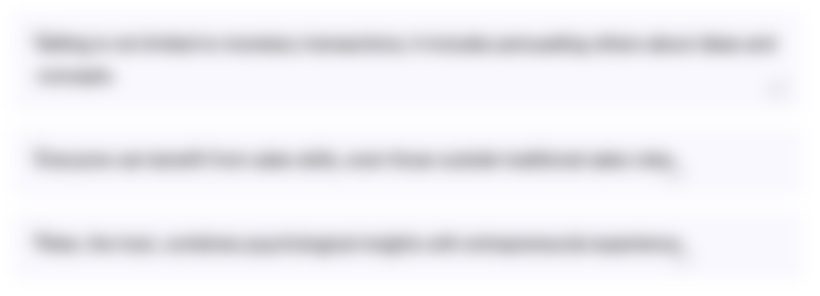
This section is available to paid users only. Please upgrade to access this part.
Upgrade NowTranscripts
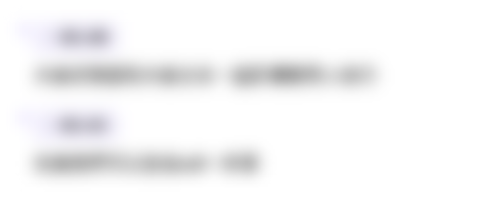
This section is available to paid users only. Please upgrade to access this part.
Upgrade NowBrowse More Related Video
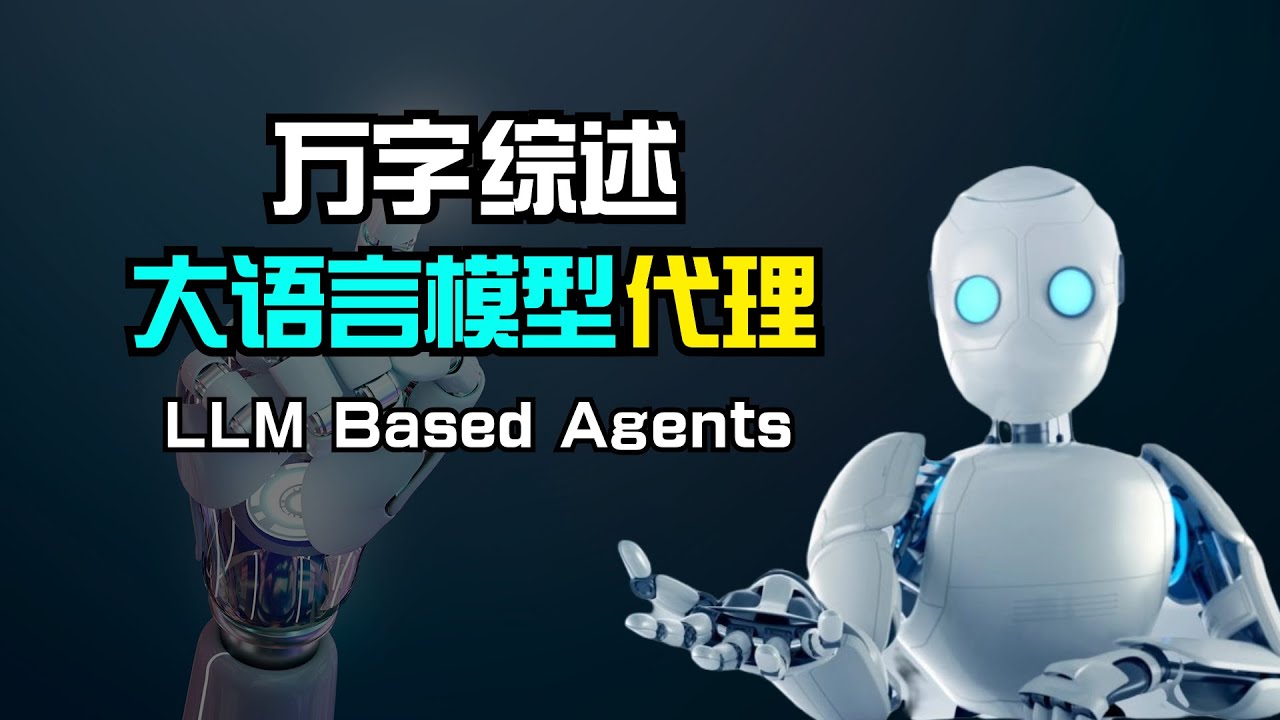
【人工智能】万字综述大语言模型代理 Agent | 研究背景 | 通用框架 | 控制、感知、行动 | 应用场景 | 代理社会 | 前瞻问题
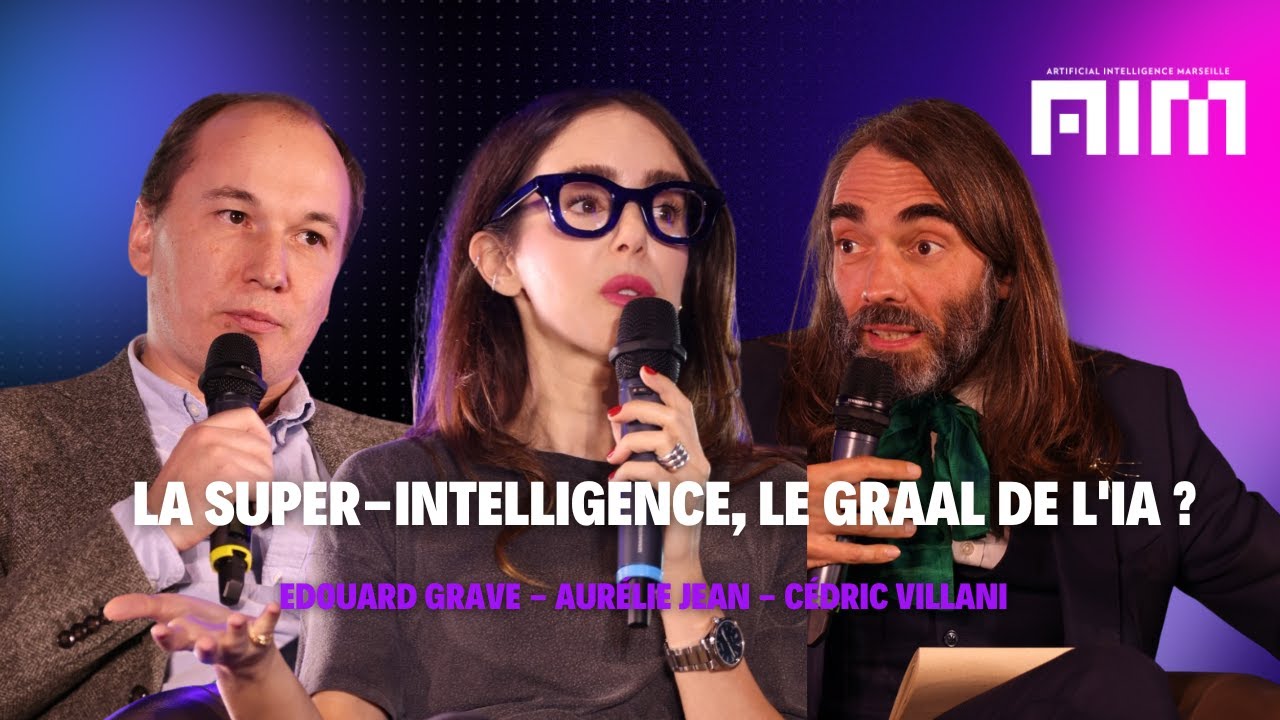
La super-intelligence, le Graal de l'IA ? | Artificial Intelligence Marseille
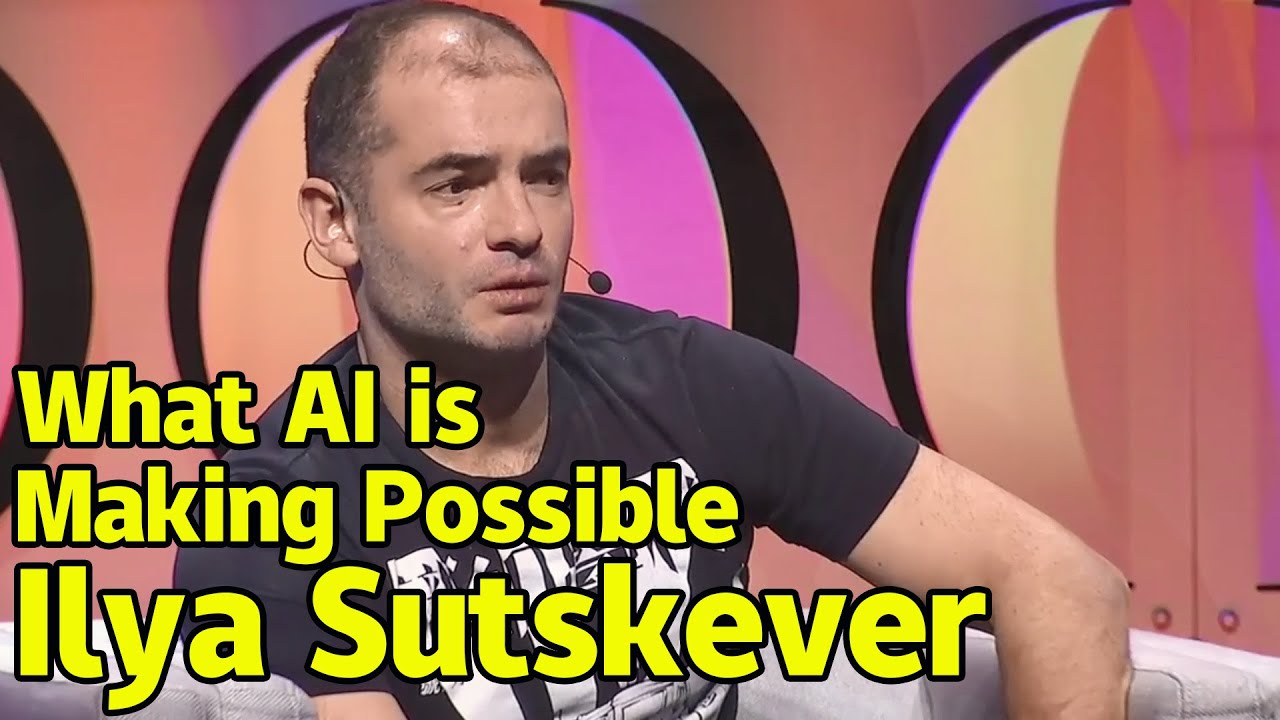
Ilya Sutskever | AI will be omnipotent in the future | Everything is impossible becomes possible
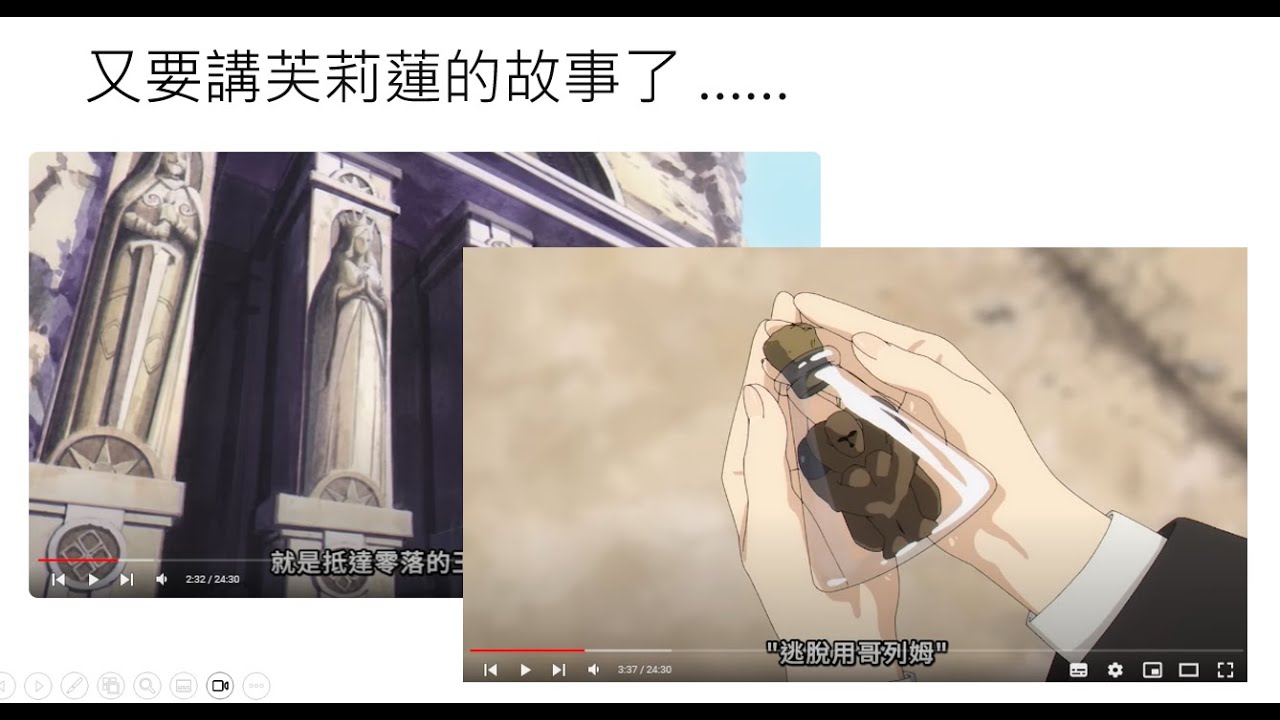
【生成式AI導論 2024】第9講:以大型語言模型打造的AI Agent (14:50 教你怎麼打造芙莉蓮一級魔法使考試中出現的泥人哥列姆)
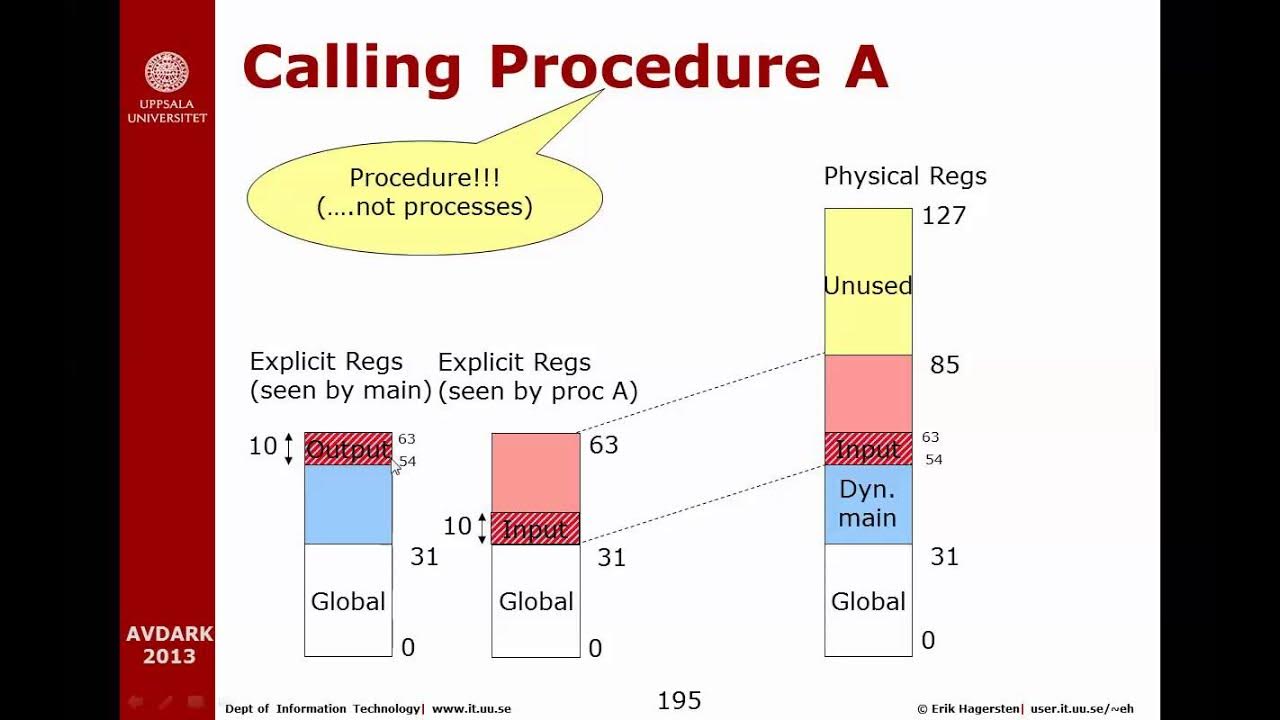
Itanium 2013 11 14 12 03 41
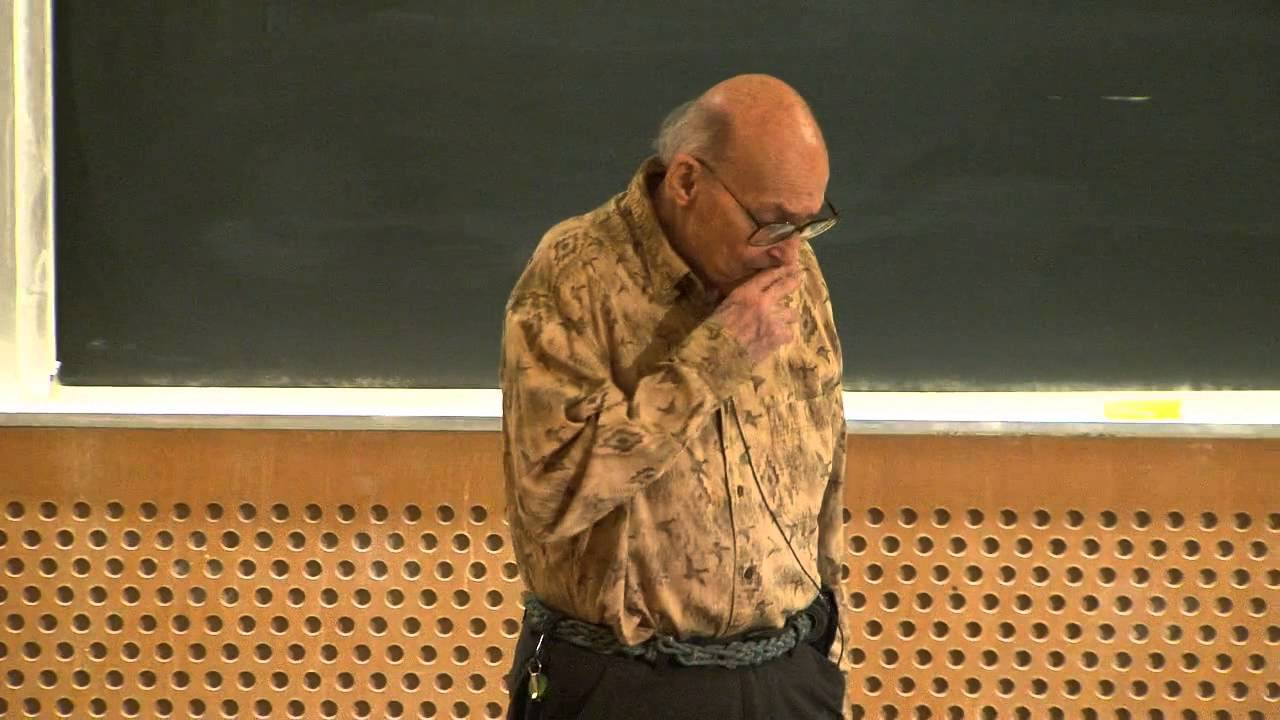
6. Layers of Mental Activities
5.0 / 5 (0 votes)