Simple Linear Regression Concept | Statistics Tutorial #32 | MarinStatsLectures
Summary
TLDRThis video serves as an introduction to simple linear regression, illustrating its application through the relationship between gestational age and head circumference in low birth weight babies. It emphasizes the use of regression models to express Y as a function of X and discusses key concepts such as correlation, the regression line, slope, and intercept. While Pearson's correlation indicates a positive association, the video stresses that correlation does not imply causation. Additionally, it outlines the goals of regression models, the significance of estimating effects versus making predictions, and acknowledges the assumptions and complexities involved in linear regression.
Takeaways
- π Simple linear regression models the relationship between one dependent variable (Y) and one independent variable (X).
- π The example used involves gestational age (X) and head circumference (Y) of low birth weight babies, illustrating a positive correlation (0.78).
- π While the correlation coefficient shows the strength of the relationship, it doesn't quantify the effect of X on Yβthat's where regression comes in.
- π The regression equation is expressed as \(\hat{Y} = b_0 + b_1X\), where \(b_0\) is the intercept and \(b_1\) is the slope.
- π The slope \(b_1\) indicates the expected change in Y for each additional unit increase in X, e.g., a 0.11 cm increase in head circumference for each extra day of gestation.
- π The intercept \(b_0\) represents the estimated Y value when X equals zero, though it may lack meaning if zero is outside the observed range.
- π Residuals (errors) are the differences between observed Y values and predicted Y values, crucial for assessing model accuracy.
- π― The two main goals of regression models are estimating the effect of X on Y and making predictions about Y based on X.
- π Understanding assumptions of regression models (linearity, independence, etc.) is essential for effective application.
- π Simple linear regression serves as a foundation for more complex regression models, such as multiple linear regression and logistic regression.
Q & A
What is simple linear regression?
-Simple linear regression is a statistical method used to model the relationship between two numeric or continuous variables, where one variable (Y) is dependent and the other (X) is independent.
What are the variables discussed in the example of simple linear regression?
-In the example, the independent variable (X) is the gestational age of a baby, and the dependent variable (Y) is the head circumference of the baby measured in centimeters.
What does a Pearson correlation coefficient of 0.78 indicate?
-A Pearson correlation coefficient of 0.78 indicates a strong positive association between the gestational age and the head circumference, meaning that as gestational age increases, head circumference tends to increase.
Why is Pearson's correlation coefficient limited?
-Pearson's correlation coefficient only describes the strength and direction of an association and does not provide information about the effect of X on Y or allow for predictions.
What is meant by the term 'model' in regression?
-In regression, a 'model' refers to a mathematical representation that describes the relationship between variables, allowing for predictions of the dependent variable based on the independent variable(s).
What are the roles of the slope (b1) and intercept (b0) in the regression equation?
-The slope (b1) indicates the expected change in the dependent variable (Y) for a one-unit increase in the independent variable (X), while the intercept (b0) represents the estimated value of Y when X is zero.
How is the error or residual in regression defined?
-The error or residual is defined as the difference between the observed value of Y and the predicted value (Y-hat), which can be expressed as (yi - yi^).
What does centering the X variable mean?
-Centering the X variable involves adjusting the variable so that it has a meaningful zero point, which can help provide a more interpretable y-intercept in the regression model.
What are the two broad goals of regression models mentioned in the video?
-The two broad goals of regression models are to estimate the effect of X on Y and to make predictions about Y based on given values of X.
What are some necessary assumptions for building a linear regression model?
-Necessary assumptions for linear regression include linearity, independence of errors, homoscedasticity (constant variance of errors), and normality of error terms.
Outlines
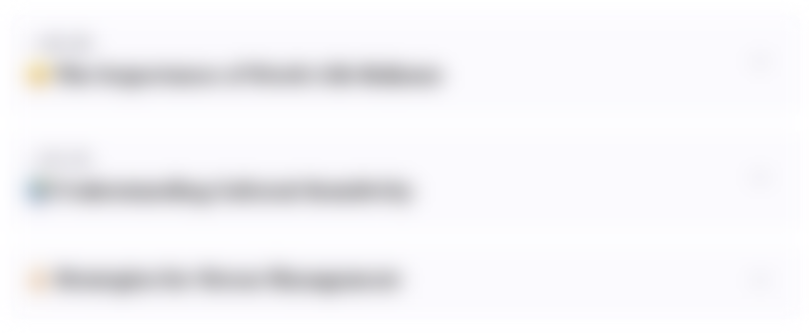
This section is available to paid users only. Please upgrade to access this part.
Upgrade NowMindmap
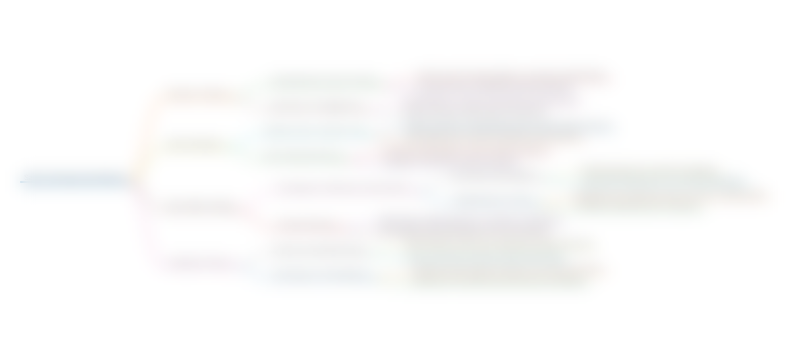
This section is available to paid users only. Please upgrade to access this part.
Upgrade NowKeywords
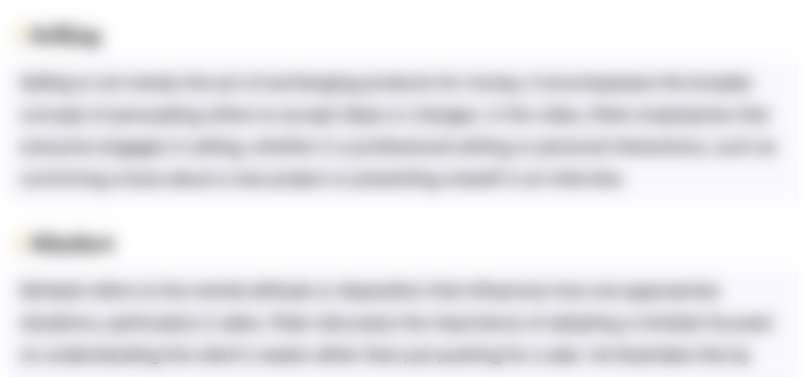
This section is available to paid users only. Please upgrade to access this part.
Upgrade NowHighlights
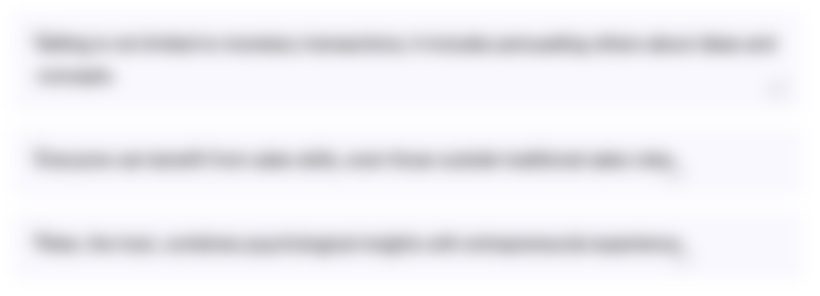
This section is available to paid users only. Please upgrade to access this part.
Upgrade NowTranscripts
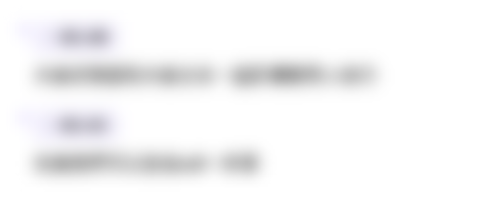
This section is available to paid users only. Please upgrade to access this part.
Upgrade NowBrowse More Related Video
5.0 / 5 (0 votes)