What REALLY is Data Science? Told by a Data Scientist
Summary
TLDRThe video emphasizes that data science is fundamentally about solving real-world business problems using data, rather than just building complex models or visualizations. It traces the evolution of data science from data mining to the emergence of big data and AI. The speaker, a data scientist at a major tech company, highlights the industry's focus on actionable insights and the importance of analytics over advanced machine learning techniques. He clarifies misconceptions about the role of data scientists and discusses the varying responsibilities across startups, medium, and large companies, encouraging viewers to engage with the content for deeper insights.
Takeaways
- 📊 Data science focuses on creating impact for companies rather than just building complex models or visualizations.
- 🔍 Misconceptions about data science often stem from a misalignment between popular topics and industry needs.
- 🧠 Data science combines computer science and data mining, evolving from data mining to a more technical field that emphasizes statistical analysis.
- 💻 The rise of web 2.0 and big data has created massive amounts of data that traditional technologies can't handle, leading to the emergence of data science.
- 📈 Applications of data science include insights, data products, and product recommendations, with a strong emphasis on real-world impact.
- 🤖 Machine learning and AI are popular in the media but are not the sole focus of data science in industry; traditional analytics play a crucial role.
- 🔄 Companies often seek data scientists to solve ambiguous problems and provide strategic guidance rather than merely crunching numbers.
- 🏢 The responsibilities of data scientists vary by company size, from handling all aspects of data science in startups to specializing in certain areas in larger organizations.
- 🛠️ In medium to large companies, data scientists may focus more on analytics, while data engineers handle data collection and infrastructure.
- 📣 The evolving landscape of data science requires professionals to adapt and specialize based on their company's needs, with various roles such as data analysts, data scientists, and machine learning engineers.
Q & A
What is the primary goal of data science according to the speaker?
-The primary goal of data science is to use data to create as much impact as possible for a company, whether through insights, data products, or product recommendations.
How does the speaker differentiate between the roles of data scientists and traditional analysts?
-Data scientists are described as problem solvers and strategists who tackle ambiguous and complex problems, while traditional analysts may focus more on routine data analysis.
What misconception about data science is highlighted in the video?
-A common misconception is that data science is solely about advanced machine learning and AI, whereas the industry often requires skills in basic analytics, experimentation, and metrics.
What historical developments led to the emergence of data science as a field?
-Data science emerged from the earlier concept of data mining, popularized in 1996, and evolved when William S. Cleveland combined computer science with data mining, leading to the technical expansion of statistics.
How did the rise of Web 2.0 influence data science?
-The rise of Web 2.0 allowed users to interact and contribute to websites, generating vast amounts of data that became challenging to manage with traditional technologies, giving rise to the concept of Big Data.
What are some tools or technologies mentioned that support data science?
-Tools and technologies mentioned include parallel computing technologies like MapReduce, Hadoop, and Spark, which are necessary for handling large datasets.
Why does the speaker believe that AI and deep learning are not the highest priority for companies?
-The speaker argues that AI and deep learning, while heavily covered in the media, often do not yield the most results for the least effort compared to foundational analytics and experimentation.
How do the roles of data scientists vary between startups and larger companies?
-In startups, a data scientist may handle multiple roles due to limited resources, whereas in larger companies, roles are often specialized, allowing data scientists to focus on specific areas like analytics or AI.
What is the significance of A/B testing in the data science process?
-A/B testing is crucial as it allows companies to experiment with different product versions and determine which performs better, providing actionable insights for decision-making.
What advice does the speaker offer for those interested in pursuing a career in data science?
-The speaker encourages aspiring data scientists to focus on understanding the practical applications of data science in solving real business problems, rather than just learning advanced modeling techniques.
Outlines
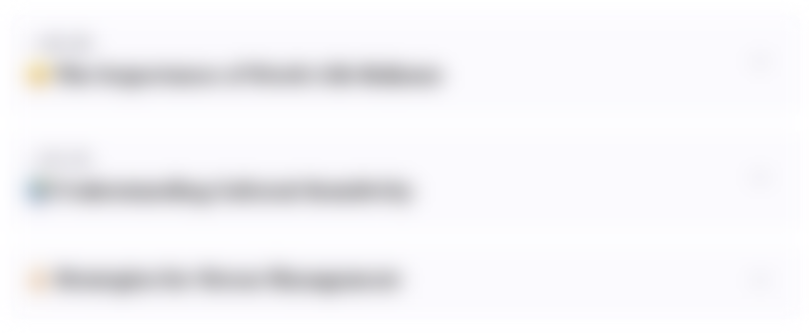
This section is available to paid users only. Please upgrade to access this part.
Upgrade NowMindmap
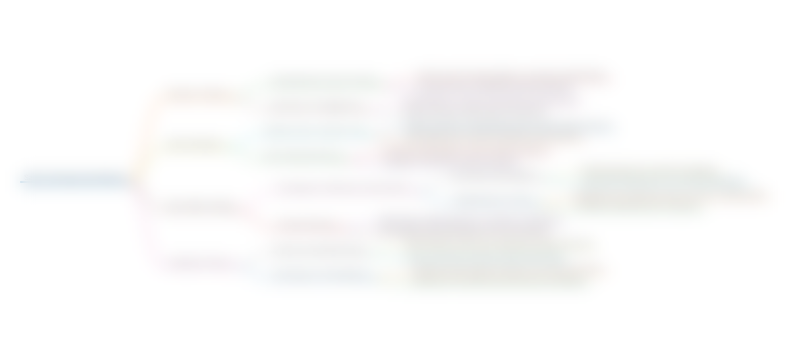
This section is available to paid users only. Please upgrade to access this part.
Upgrade NowKeywords
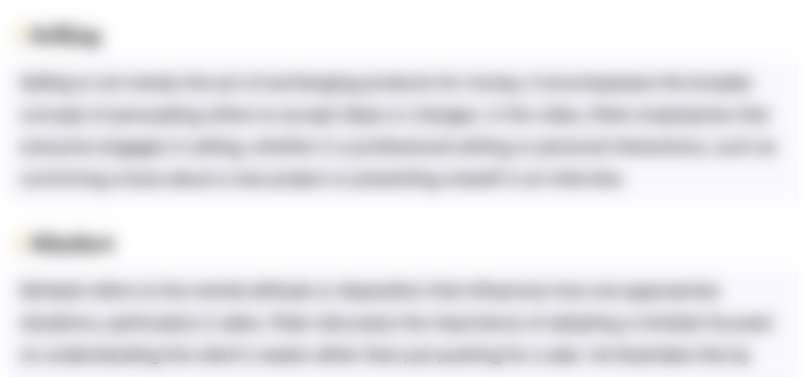
This section is available to paid users only. Please upgrade to access this part.
Upgrade NowHighlights
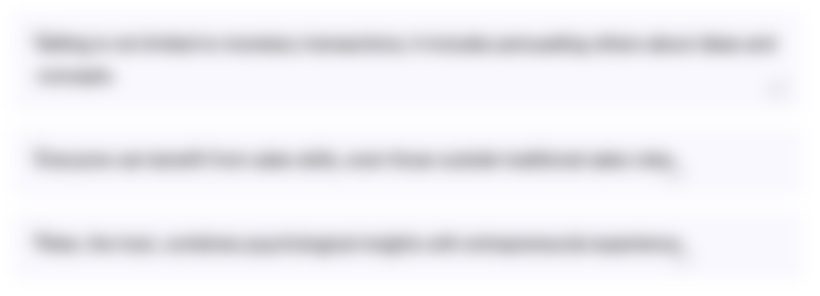
This section is available to paid users only. Please upgrade to access this part.
Upgrade NowTranscripts
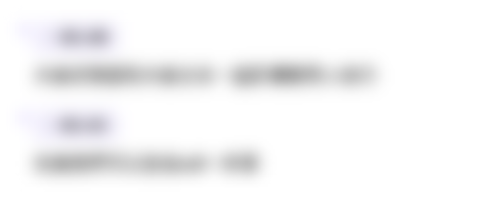
This section is available to paid users only. Please upgrade to access this part.
Upgrade NowBrowse More Related Video
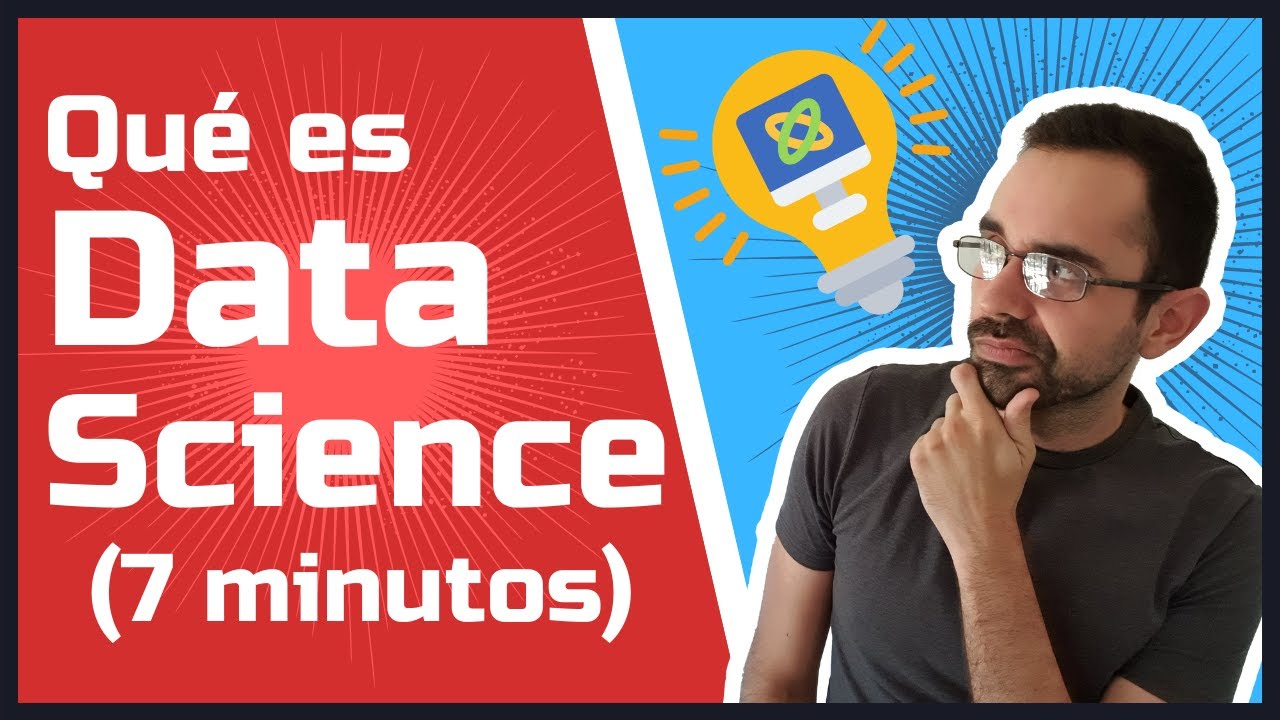
CIENCIA DE DATOS en 7 Minutos ⚡️💻 ¿Qué es Data Science Español?
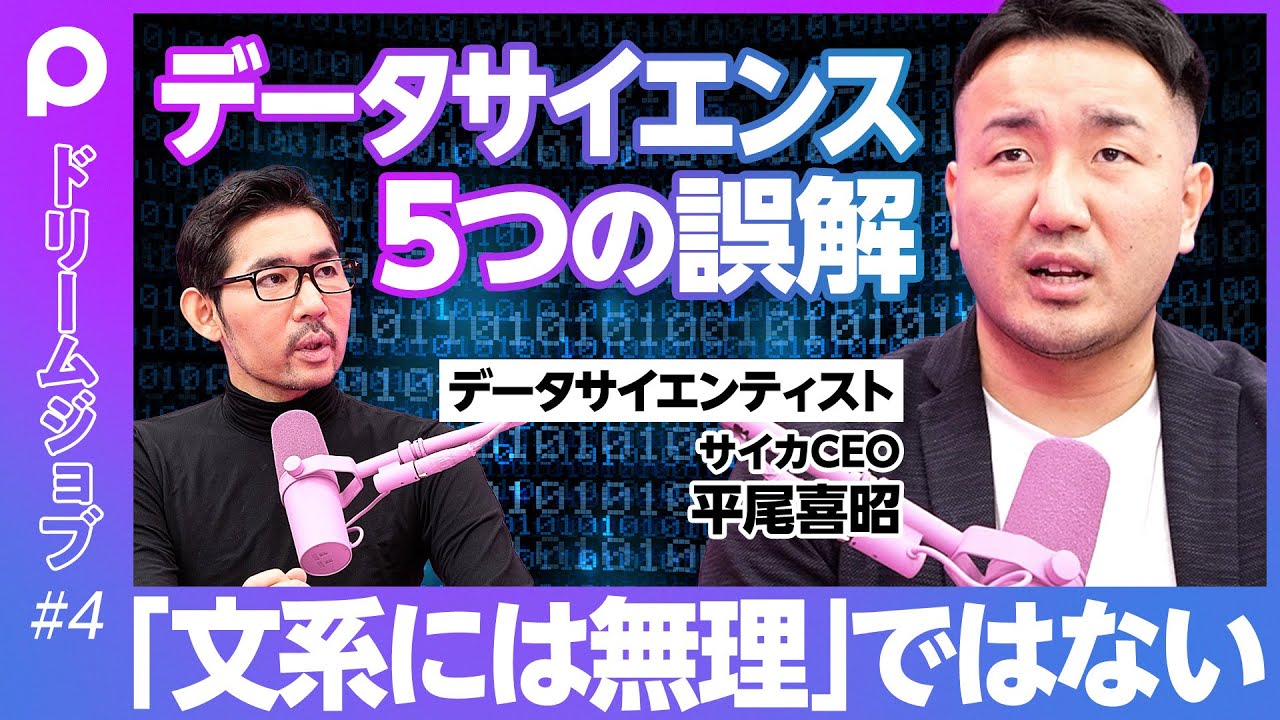
【データサイエンス5つの誤解】①データサイエンス=データを分析すること/②データサイエンスは「+α」/③データサイエンスは専門家に任せるべき/④分析結果=答え/⑤文系には無理/独習におすすめの書籍
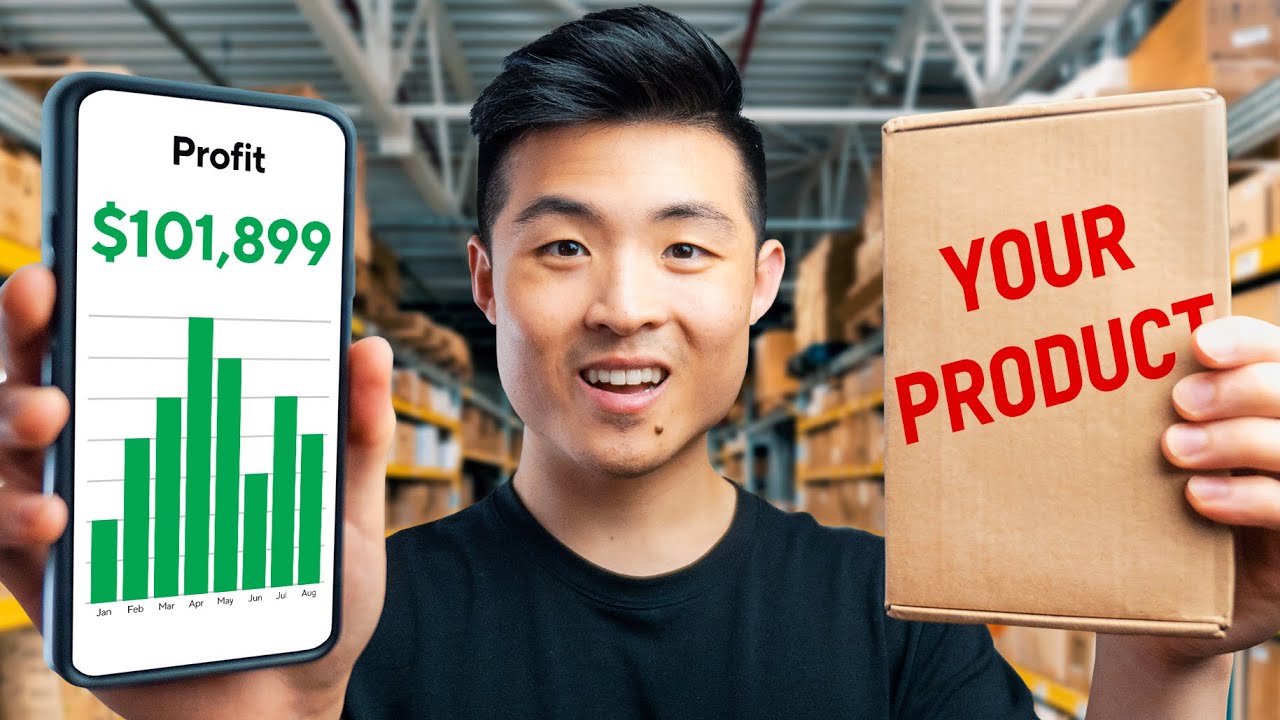
Easiest Way To Start An Online Business From $0
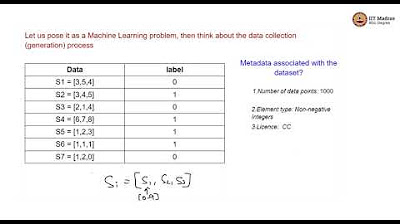
To ML or not to ML
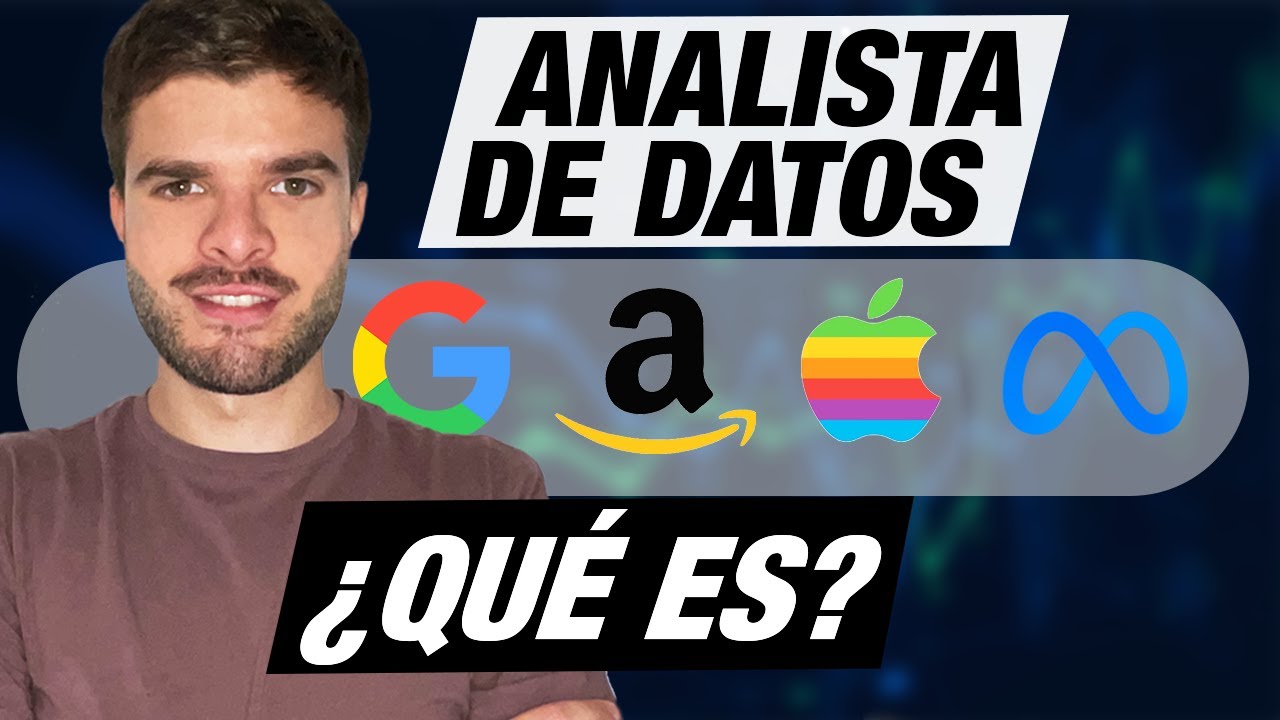
¿Qué es ser un Data Analyst? Contado por un Analista de Datos
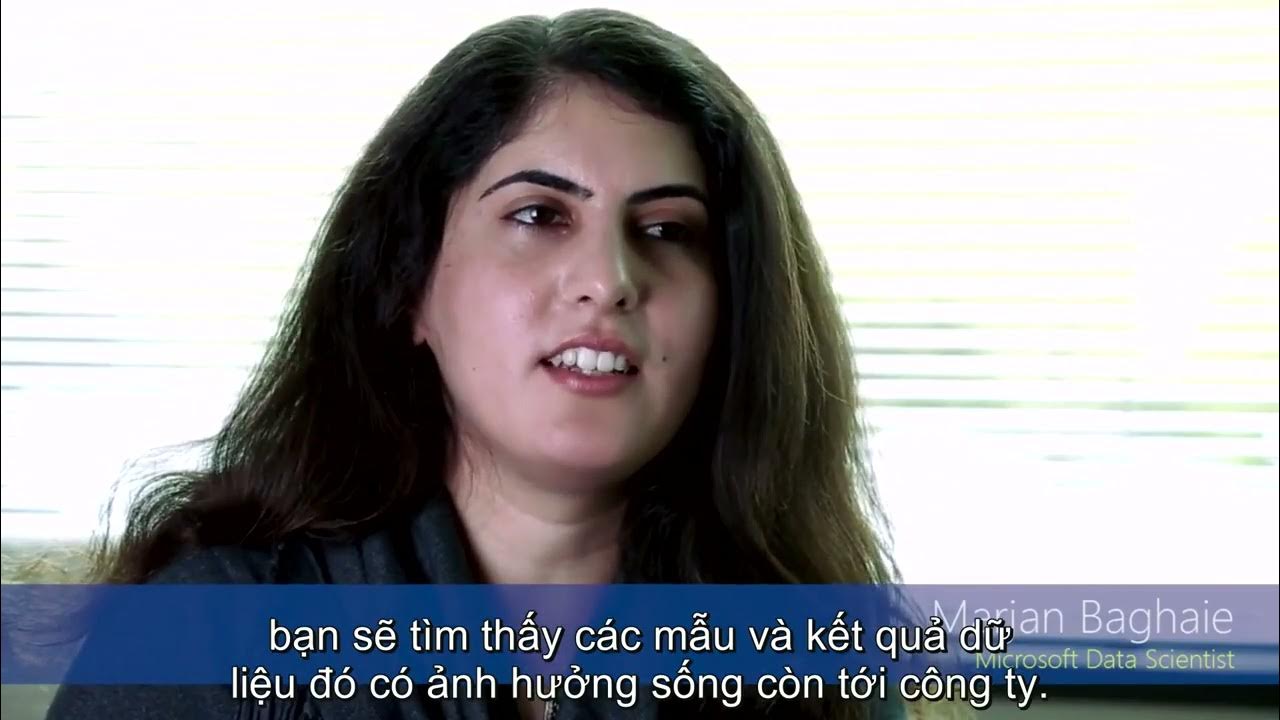
01 Điều gì khiến tôi mong muốn trở thành một nhà khoa học dữ liệu
5.0 / 5 (0 votes)