0625 Función de densidad conjunta, marginal y condicional
Summary
TLDRThis video script delves into the concepts of joint, marginal, and conditional probability functions for continuous random vectors. It defines and explains the notion of a joint density function, illustrates probability calculations through integrals, and presents a step-by-step example with a specific continuous random vector. The script also covers marginal density functions and how they are calculated, extending the discussion to higher dimensions. Furthermore, it introduces conditional density functions, providing examples and formulas. The final section discusses conditional expectation, concluding the presentation of key concepts in continuous probability theory.
Please replace the link and try again.
Q & A
What is the definition of a joint probability density function (pdf) for continuous random vectors?
-A joint probability density function for a continuous random vector is a non-negative, integrable function defined on R² such that the probability of the random vector X and Y being less than or equal to certain values can be expressed as a double integral of the density function over the desired region.
What are the two main properties of a joint probability density function?
-The two main properties of a joint probability density function are: 1) It is non-negative, and 2) Its integral over the entire plane (R²) must equal 1, ensuring that the total probability is 1.
How do you calculate the probability that a continuous random vector X, Y lies within a certain region?
-The probability that X lies between values a and b, and Y lies between values c and d is calculated as a double integral of the joint pdf, where the limits of integration are from a to b for X, and from c to d for Y.
In the example with the joint density function f(x,y) = 8xy, what condition must X and Y satisfy for the pdf to be positive?
-The joint pdf f(x, y) = 8xy is positive when both X and Y are between 0 and 1, and when X is less than Y.
How is the marginal probability density function of X calculated from a joint pdf?
-The marginal probability density function of X is calculated by integrating the joint pdf f(x, y) with respect to Y over the entire range of Y values.
How can the marginal probability density function of Y be calculated?
-The marginal probability density function of Y is calculated by integrating the joint pdf f(x, y) with respect to X over the entire range of X values.
What is the process for calculating the conditional probability density function of X given Y = y?
-The conditional probability density function of X given Y = y is calculated by dividing the joint pdf f(x, y) by the marginal pdf of Y, f(y). This gives a conditional distribution of X as a function of Y.
What is the formula for the conditional density of X given Y = y in the example?
-The conditional density of X given Y = y is given by the expression f(x|y) = (8xy) / (4y³), which simplifies to 2x / (1 - x³) for 0 < x < y < 1.
How is the conditional density function of Y given X = x calculated?
-The conditional density function of Y given X = x is calculated by dividing the joint pdf f(x, y) by the marginal pdf of X, f(x). This results in a conditional distribution of Y as a function of X.
What is the formula for the conditional density of Y given X = x in the example?
-The conditional density of Y given X = x is given by f(y|x) = (8xy) / (4x(1 - x³)), which simplifies to 2y / (1 - x³) for 0 < x < y < 1.
Outlines
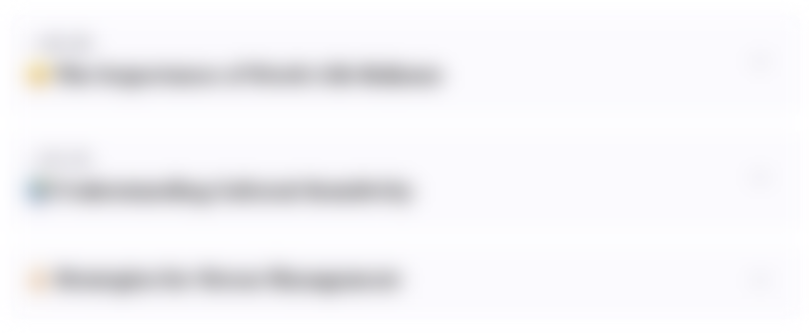
This section is available to paid users only. Please upgrade to access this part.
Upgrade NowMindmap
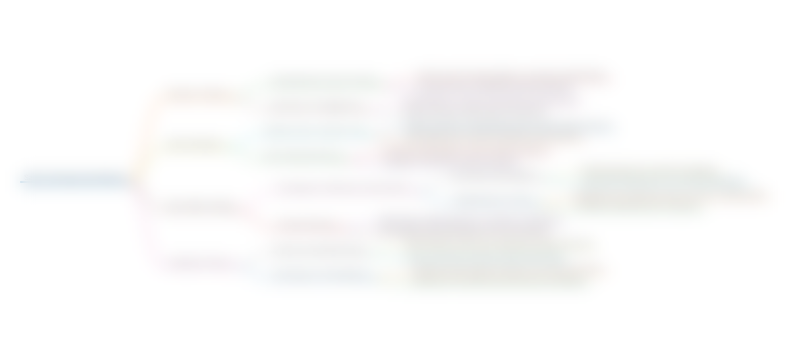
This section is available to paid users only. Please upgrade to access this part.
Upgrade NowKeywords
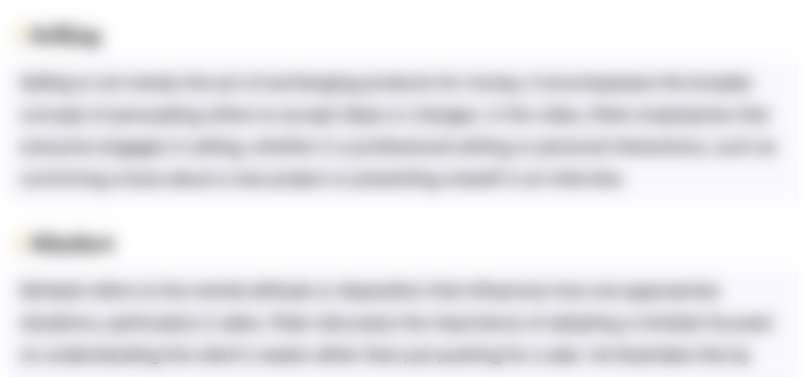
This section is available to paid users only. Please upgrade to access this part.
Upgrade NowHighlights
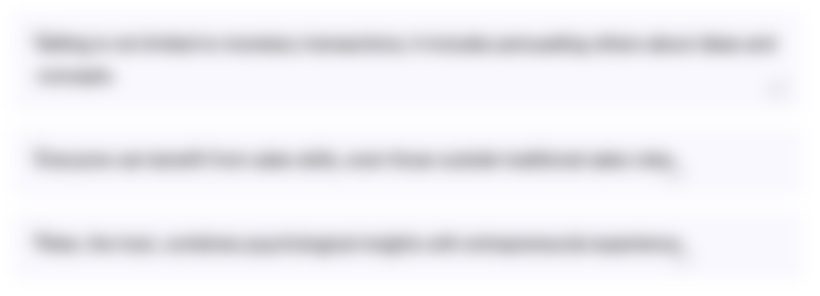
This section is available to paid users only. Please upgrade to access this part.
Upgrade NowTranscripts
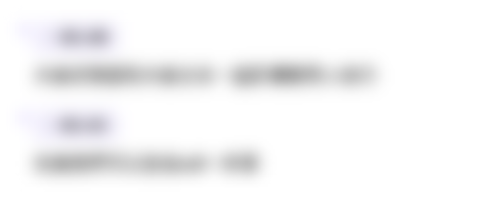
This section is available to paid users only. Please upgrade to access this part.
Upgrade NowBrowse More Related Video
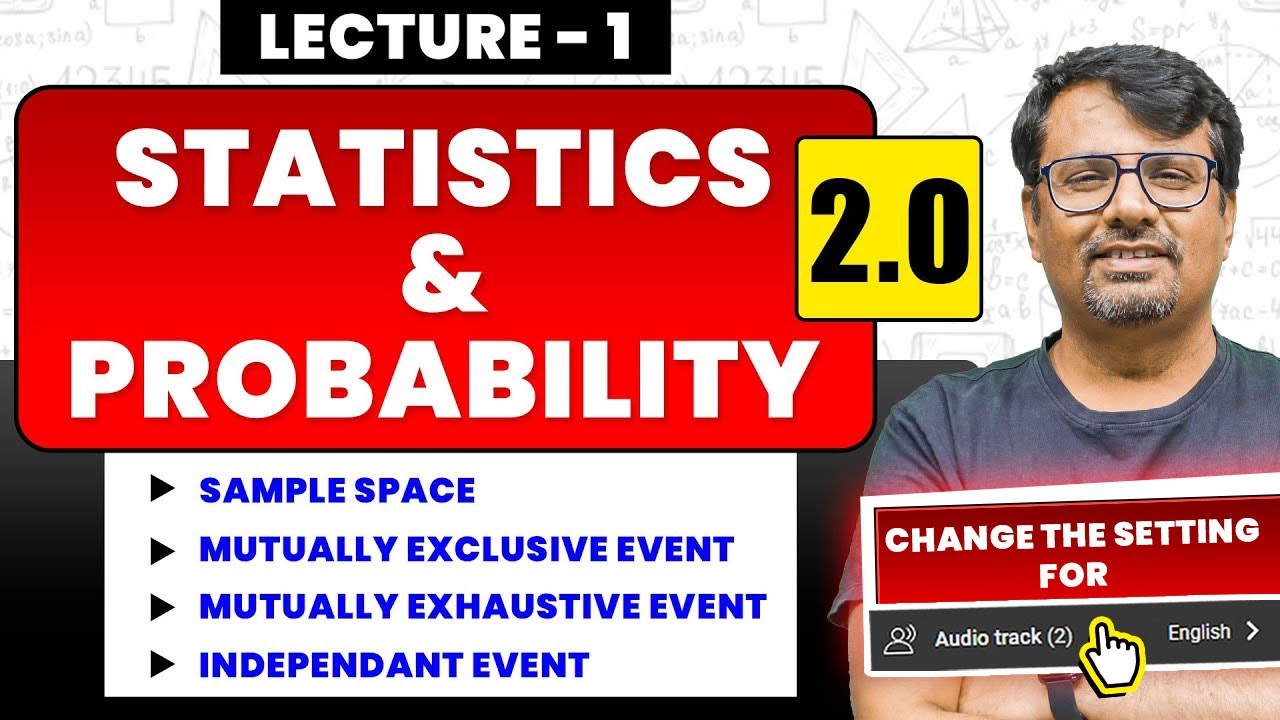
Overview, Sample Space & Types of Events | Statistics and Probability | By GP Sir

0625 Independencia de variables aleatorias
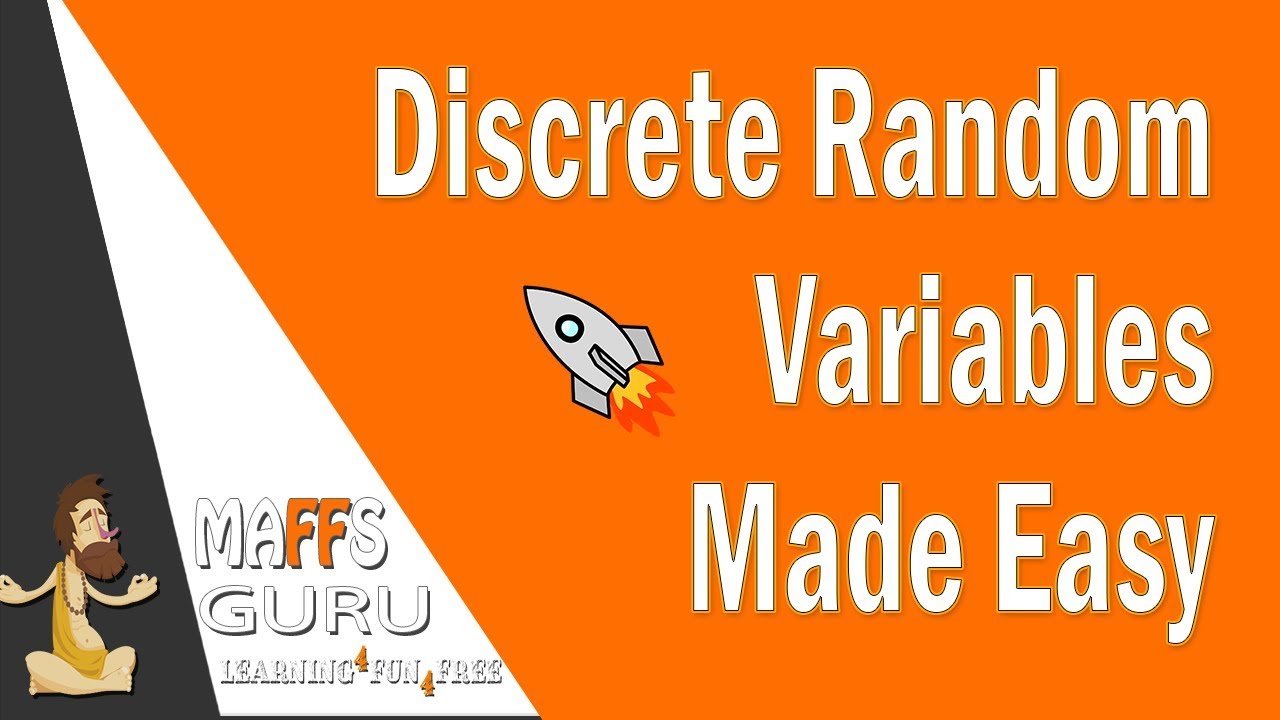
Discrete random variables | Methods 3 and 4 | MaffsGuru
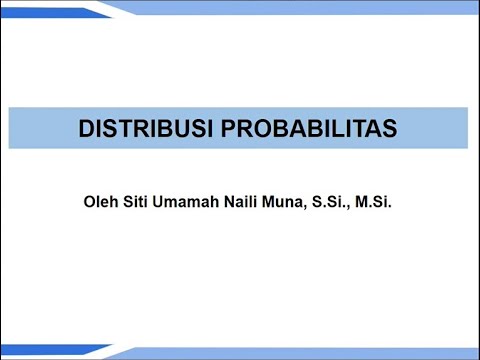
Pertemuan 1 - Distribusi Probabilitas (Part 2)
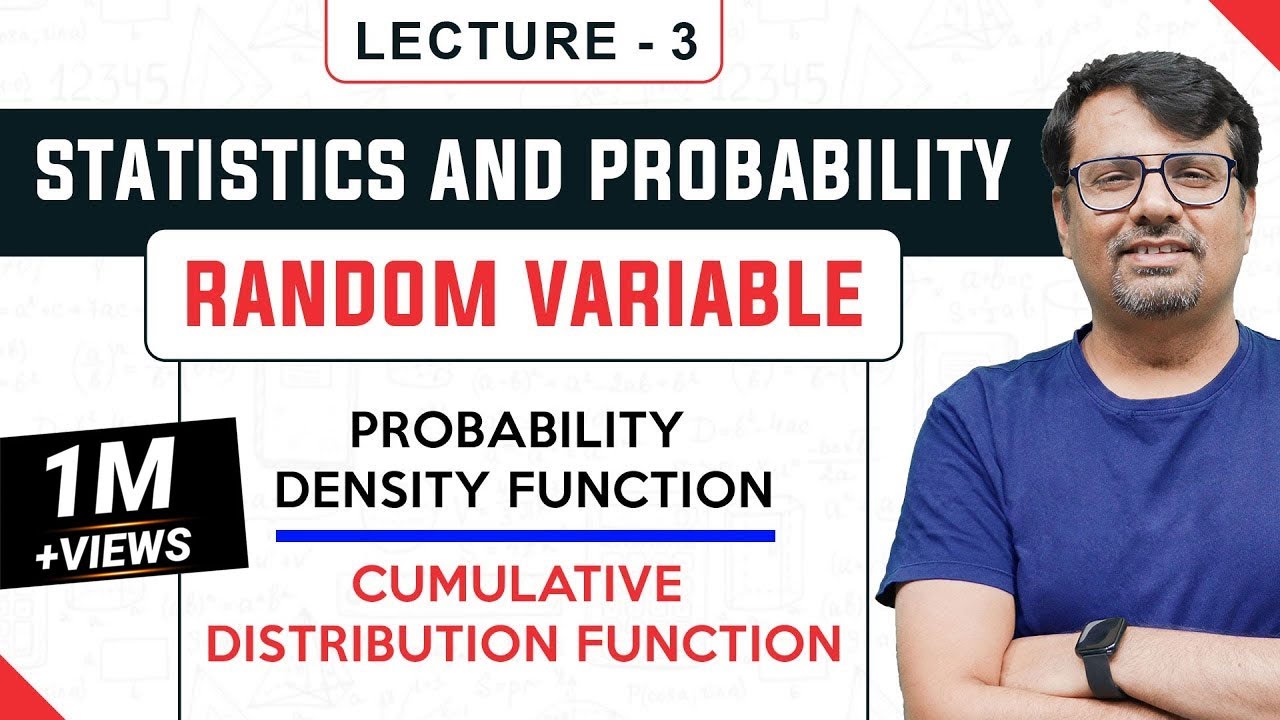
Random Variable, Probability Density Function, Cumulative Distribution Function
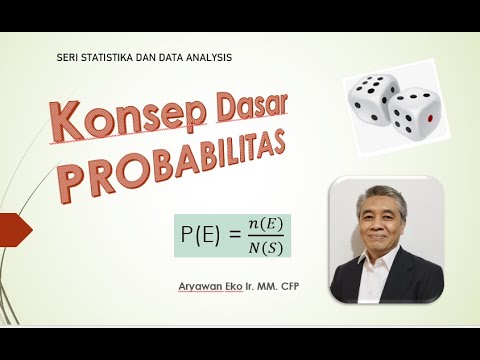
STATISTIKA | KONSEP DASAR PROBABILITAS
5.0 / 5 (0 votes)