一招让你的ChatGPT变聪明|context window原理讲解
Summary
TLDR本次课程由Yen主讲,主题为“AI助力的生产力:从用户到建设者”。课程以Zoom会议形式进行,前20至25分钟为讲座,随后是问答环节。Yen指出,尽管AI是热门话题,但用户在使用Chat GPT时可能会发现其表现不尽如人意。这主要是因为用户对Chat GPT的使用方式存在误区。课程核心是介绍一个关键概念——上下文窗口(context window),它决定了AI的智能表现和使用效果。上下文窗口是AI生成回复时所“看到”的信息,但受限于其有限的容量。通过有效管理上下文窗口,可以显著提升AI的响应质量和智能表现。Yen还介绍了一个简单但有效的技巧:通过编辑当前提示(prompt),而非仅仅追加聊天内容,来重新构造上下文窗口。此外,课程还讨论了如何通过训练和最佳实践来优化AI的使用,提高生产力。最后,Yen回答了关于课程内容、目标受众和课程实用性的问题,并提到了Google提出的无限窗口模型(infinite window model),这可能在未来减少手动管理上下文窗口的负担。
Takeaways
- 📈 **AI 应用技巧**:了解如何更有效地使用 AI,比如通过管理上下文窗口来提升 AI 的表现。
- 🔍 **上下文窗口**:上下文窗口是 AI 生成响应时所看到的内容,它决定了 AI 能够记住的信息量。
- 🚫 **有限的容量**:AI 的上下文窗口有容量限制,这会导致它忘记早期的对话或要求,从而影响其性能。
- 💡 **编辑提示**:通过编辑当前的提示并保存,可以有效地管理上下文窗口,而不是简单地追加新的对话。
- 🔄 **迭代改进**:当 AI 的回答不尽如人意时,可以通过重新组织提示来迭代改进,而不是通过增加更多的对话。
- 🧠 **AI 作为实习生**:将 AI 视作需要指导的实习生,通过精确的提示来引导它完成复杂的任务。
- 📚 **持续学习**:随着 AI 技术的快速发展,持续学习最新的研究和最佳实践对于有效使用 AI 至关重要。
- 🔧 **工具使用**:了解不同工具如 Sandbox 和 Chat Interface 的差异,以及它们如何影响与 AI 的交互。
- 📈 **效率提升**:通过结构化的提示和上下文窗口管理,可以显著提升个人的工作效率。
- 📝 **重用性**:编写的提示不仅用于解释期望的结果,还用于纠正 AI 的非预期行为,这增加了提示的重用性。
- ❓ **问题解决**:在 AI 显得懒惰或健忘时,通过训练和理解其工作原理,可以找到解决问题的有效方法。
Q & A
AI为什么有时会表现得懒惰和健忘?
-AI表现得懒惰和健忘主要是因为它的设计方式。AI如Chat GPT是设计来支持和鼓励对话的,这种形式的产品自然会导致某些使用情况,使得AI忘记事情、失去细节跟踪,并显得笨拙和懒惰。
什么是上下文窗口,它如何影响AI的响应?
-上下文窗口是AI在生成响应时所看到的内容。它是有限大小的,只能处理一定数量的输入。当AI生成回答时,它会将历史对话和最新问题作为上下文窗口的一部分,如果上下文窗口填满,AI可能会忽略旧的请求,导致它忘记之前的要求。
如何有效地管理上下文窗口以提高AI的效率?
-可以通过有意地编辑和组织上下文窗口来提高AI的效率。例如,将所有要求汇总在一个段落中,而不是分散在多个对话中。此外,使用编辑功能而不是简单地追加对话,可以帮助AI更精确地理解和响应。
为什么说对AI的训练很重要?
-对AI的训练很重要,因为它可以纠正我们对AI使用的第一反应,教导我们最佳实践,并帮助我们形成习惯,直到这些做法成为肌肉记忆。通过训练,我们可以更好地理解AI的工作原理,从而更有效地使用它。
课程的目标受众是谁?
-课程的目标受众是IT行业的专业人士,他们需要具备一些基本的Python知识,例如运行Python程序、阅读简单的Python程序和安装Python库。
参加这个课程我们最终能获得什么?
-参加这个课程,我们可以理解AI的基础原理,学习最佳实践,这将极大地提高我们的生产力。个人经验表明,这可以提高两到五倍的生产力。
Sandbox和Chat GPT界面有什么区别?
-Sandbox是OpenAI提供的工具,它直接调用底层GPT API,与Chat GPT界面相比,它没有额外的提示和限制。Sandbox允许使用更长的上下文窗口,并且需要手动管理上下文窗口和聊天历史。
课程是否有一个议程或者大纲?
-是的,课程有一个详细的议程或者大纲,包括课程的日期、课程大纲、预期成果、目标受众等信息,可以通过扫描提供的二维码来获取更多课程信息。
如果我想学习更基础的关于AI的知识,你有什么建议?
-如果你想要学习更基础的知识,我建议直接注册并使用Chat GPT或其他类似的产品。通过实践使用,你可以感受到AI的能力,并提出自己的问题,这将帮助你更好地理解你想要从AI课程中获得什么。
课程内容是否会随着AI模型的迭代而变得过时?
-课程内容分为两部分:一部分是基础研究,这些通常长时间内不会改变;另一部分是基于这些研究得出的技巧和技能,这些可能会随着AI模型的发展而更新。课程会尽量跟上领域的发展,确保内容的时效性。
Google最近是否提出了解决上下文窗口问题的新模型?
-是的,Google最近提出了一个新的模型,称为无限窗口模型,但据我所知,它仍处于研究阶段。一旦它投入生产使用,希望我们能减少手动管理上下文窗口的负担。
你个人对Cloud V3有什么体验?
-我个人使用过Cloud V3,发现与GPT相比,管理上下文窗口的难度要小得多。Cloud V3在处理许多提示和上下文窗口时可能不需要那么多的技巧,这表明人们已经意识到上下文窗口的问题,并试图使其更加用户友好。
Outlines
😀 课程介绍与AI使用误区
Yen老师欢迎大家参加关于AI增强生产力的课程。课程将通过半小时的Zoom会议进行,前20至25分钟为讲座,剩余时间用于问答。在讲座期间,鼓励学生在Zoom聊天窗口提问,并使用表情反应来为他人的问题投票。课程结束后,会通过邮件发送视频和音频的下载链接。AI是当下热门话题,但使用中常出现忘记事情、懒惰等问题,这可能是因为我们使用方式不当或AI设计上的缺陷。本节课将介绍核心概念,帮助理解AI的意外行为,并提供简单技巧来提高AI的智能性和任务处理能力。
🔍 理解上下文窗口的重要性
上下文窗口是GPT生成回应时所看到的内容。在对话中,上下文窗口会随着对话的进行而变化,包括聊天记录和最新问题。常见的误解是认为AI有记忆功能,实际上AI通过上下文窗口来模拟记忆。上下文窗口的有限性导致了信息丢失和AI表现不佳的问题。例如,在编写Python程序时,如果上下文窗口已满,AI可能会忽略先前的要求。因此,管理上下文窗口对于有效使用AI至关重要。
🚀 通过训练改善AI的使用
通过学习驾驶的比喻,强调了训练对于掌握技术内部工作原理和最佳实践的重要性。在AI的使用上,我们需要通过训练来纠正第一反应,采用良好实践。管理上下文窗口是解决方案之一,我们应该主动思考上下文窗口应如何配置以使AI更好地工作。通过有意识地编辑上下文窗口,而不是盲目接受AI的设计,可以显著提高AI的效率和智能性。
📝 上下文窗口管理的实际应用
介绍了如何通过编辑当前提示来管理上下文窗口,而不是简单地追加对话。通过将所有要求整理在一段中,可以创建更有效的提示或上下文窗口。这种方法可以使AI更精确地理解任务要求,从而更有效地解决问题。编辑风格与聊天风格相比,可以更有效地利用上下文窗口,并且通过编辑功能,我们仍然保持了易用性。此外,编辑风格还提高了提示的可重用性,有助于纠正AI的非预期行为。
🎓 课程目标与最佳实践
课程的目标是帮助IT行业的专业人士提高生产力,但需要具备一定的Python基础知识。课程内容将涵盖AI的基础和最佳实践,如上下文窗口管理。通过训练,学员将理解AI的工作原理,并学会如何有效地使用AI。课程结束后,学员可以期待生产力的显著提升。同时,讨论了Sandbox与Chat界面的不同,包括安全性、上下文窗口的管理方式和成本等方面。
📈 课程结构与未来发展
课程将提供详细的日程安排,并通过QR码可以访问课程主页以获取更多信息。课程内容将包括如何通过编辑和提问来改进提示的结构。讨论了AI的发展趋势,包括上下文窗口和无限窗口模型。课程内容将分为两部分:基础研究和基于研究的技巧与技能。虽然AI领域发展迅速,但基础原理预计在长时间内保持稳定。课程将不断更新,以保持信息的时效性。
🤖 AI模型的比较与课程总结
Yen老师分享了对Cloud V3和GPT-4的体验,认为Cloud V3在管理上下文窗口方面更为用户友好,而GPT-4则相对更智能。由于时间限制,Yen老师选择了最后一个问题进行回答,并感谢大家的参与,表达了对未来课程的期待。
Mindmap
Keywords
💡AI
💡Chat GPT
💡上下文窗口(Context Window)
💡Token
💡Prompt Engineering
💡迭代(Iteration)
💡训练(Training)
💡Python
💡API
💡Sandbox
💡产品限制(Product Limitations)
Highlights
Yen介绍了AI作为新的热门话题,但指出了当前AI的局限性,比如容易忘记事情和显得懒惰。
提出了AI设计上的缺陷,导致其在对话中容易忽略细节和忘记事情。
课程将介绍一个核心概念,帮助理解AI的意外和不良行为,并提供简单的技巧来改善。
强调了不能再将AI视为一个黑箱,而是需要系统地理解和使用它。
解释了AI的基础模型和如何通过适当的提示和对上下文窗口的理解来有效使用AI。
上下文窗口(context window)的概念,即AI在生成响应时所看到的信息。
讨论了上下文窗口的有限性,以及它如何影响AI的性能和记忆。
提出了管理上下文窗口的技巧,以提高AI的响应质量和智能表现。
通过实际例子展示了如何通过编辑当前提示来改善AI的回答。
强调了训练的重要性,以纠正我们对AI的第一反应,并学习更好的实践方法。
提到了Google提出的无限窗口模型,这可能在未来减少手动管理上下文窗口的负担。
课程的目标受众是IT行业的专业人士,需要具备一些基本的Python知识。
课程旨在提高AI辅助编程的效率,并通过最佳实践提升生产力。
对比了沙盒(Sandbox)和聊天界面(Chat Interface)在使用GPT API时的不同。
讨论了如何通过结构化提示来提高AI的智能性和效率。
建议初学者首先通过使用Chat.com或其他类似产品来感受AI的能力。
提到了课程内容可能会随着模型的迭代而更新,但基础原理将保持不变。
Yen分享了对Google Cloud的新模型的体验,认为它在管理上下文窗口方面更为友好。
Transcripts
all right it's four o'clock now let's
get started hi everyone this is Yen
welcome to the lining lesson for the
course from users to builders AI powered
productivity for T
RS first several Logistics here this is
a half an hour Zoom meeting we will
spend like 20 to 25 minutes on the
lecture and then use the remaining for
the
Q&A um during the lecture feel free to
put your questions in the zoom chat
window and then use the emoji react
reactions to vote for others questions
we will answer the most popular
questions in the end the meeting is
recorded and you will receive an email
from Maven about where to download the
recorded video and
audio now let's jump right into the
topic AI is the new buzz word everyone
is talking about it and big companies
are pouring money in it but if you
really use chbt you may find actually
quite often that chpt is dumb it's lazy
it forgets about things it didn't do
what I asked you to do so we become
upset sometimes painful and curious why
is others char so smart and so
powerful well you're at the right place
this is because we used it wrong and
it's not our fault to some extent chat
gbt is flawed in design this product is
designed to support and encourage
conversations or chats it makes sense
because that's the most intuitive way of
interaction however this form of product
would naturally lead to certain usage
that makes gen forget about things lose
track of details and appear dumb and
lazy in this lesson you will learn about
a core concept from relevant research
that can explain this all it will help
you make sense of the unexpected and
undesired behaviors and naturally leads
to a counterintuitive but simple trick
if you use it you will find the AI to be
smarter and help you better on most
tasks
immediately but in order to do that we
we can't treat chbt as a blackbox
anymore we don't come up with a random
explanation or try out different tricks
and wishing it to work in this lesson we
will tackle the lazy and forgetful
problems of AI systematically beginning
with understanding what is inside
trbt not trbd is still developing very
fast we hope what you learned today will
still be relevant in a few years so we
need to go to the
research the most relevant research of
chbt began about six years ago it began
with a foundation model and then
alignment was added to make it something
similar to today's CH gbt in order to
effectively use this tool we also need
to add proper prompting and a solid
understanding of context Windows this
four are most practical and fundamental
components of using
chbt understanding each component will
help us um will make our AI smarter and
our work more effective in the full
course we we will go over each of them
but today we only have about 30 minutes
so we'll still try to do it but focus on
one thing the contact window because it
can help with all of your prompts it's
often overlooked but it's Central to
explain and correct a lot of the
problems of
jni after understanding the concept we
will then introduce a simple but very
effective trick to effectively manage
the context window you may be familiar
with the G on the left which is often
the case for people not managing their
contact window it gave short answers not
willing to help but with the same model
and even the same prompt after we use
this simple trick the gbt immediately
becomes more willing to help and even
smarter this is the power of contact
window management and let's begin the
journey by first understanding what is a
contact
window contact window is a concept
introduced very early in original GPT
paper it has its meaning derived from
the original along with time even in
open eyes on documents but in this
course a contact window is simply what
trpd sees when it generates
responses what does that mean let's take
a look at basic
example the simplest way to use trbd is
you ask it a question and gives you an
answer in this example it ask it I have
many large files to copy from one
computer to another computer what should
I do this sentence is my question and is
also what chpd sees when generating the
response therefore in this example this
sentence is the context window again
this is a probably 90% but not 100% the
same as original definition in the paper
but in the context of this course a
context window is what chbd sees when it
generates
responses we just saw a simply the case
of we ask a chpd one question things
begin to get more interesting when chpd
generates a response and we ask a
followup question I cannot upload the
file to the cloud storage what are my
options in this case the the context
window will change to the re red
rectangle it would include the chat
history and latest the
question this might be a little bit
counterintuitive for some people so
let's stop here and make a clarification
on a common
misconception trbt is a good product it
gives people an impression that we're
talking with a human so it's natural for
us to imagine oh chbd has a memory
storing its understanding of the world
storing our chat history our requests
and so on and then it reads my question
and tries to answer it by recalling from
the memory and reasoning based on
information in this case the context we
though would be the latest only the
latest question not including the
previous
conversation however this is a natural
and common
misconception open AI here uses a clever
trick to make chb appear to have memory
under the hood what happened is open AI
includes the chat history as part of the
context window and ask gbt to answer the
question based on all the information
including the historical conversations
and the latest question in other words
chat gbt implements memory by appending
chat history to the context window when
we ask CH gbt a new question what
happens is the answer from the last
round and the latest question was added
to the contact window nothing else no
memory no database no state so in the
example above the contact window would
be my first question tr's first response
and my second question
this is a very important clarification
actually it immediately causes two
problems the first problem is that the
contact window is finite chat gbt or any
gen so far can only process a limited
number of
input GPT 3.5 had a limit of 4K tokens
which is about 3,000 words and gradually
expanded to 16k gbd4 had a limit of 8K
tokens and gradually expanded to 120 8K
but still kept a limit of output window
of 4K tokens these contact Windows
appear large but for certain tasks they
can be quickly consumed and causing
issues for example here the user asks
chbt to write a Python program with an
actal requirement that for some reason
don't use the library of npy the AI then
outputs a Python program which can
easily cost hundreds sometimes a
thousand words of tokens
then the user did a few rounds of
iterations to ask the AI to add the
feature of A and B up to this point the
contact window may still hold entire
chat history showing the red rectangle
but assume that it's close to the upper
limit of the context window in this
situation then the user asks can you
also do c in the program then in order
to still fit the contact window and
focus on the latest and potentially the
most important requests try to be PD
drops the first conversation and moves
the contact window down to only keep the
latest
conversations and this causes a problem
the request of I cannot use npy is now
not in the context window anymore and
chbd has no knowledge on that anymore so
it's totally possible that in the latest
response it may begin using numpy and
from the users perspective trag fails to
satisfy our requirements it forgets
about things it's not as smart as the of
the conversation this is all caused by
the fact that the contact window is
limited and it cannot accommodate
infinite
chat the finite size of the problem of
the context window is easy to recognize
and mitigate when the conversation is
long and AI appears less smart we can
consider restarting a
conversation but a lot of times even
when the conversation is short we still
feel the AI is lazy and dumb and that's
because of another more subtle but
impactful limit gbt has difficulties
processing long or or unorganized
content for this long or unorganized
cont contacts Windows gbt may miss
certain requirements especially those
need attention to the details in the
previous example even if the entire
conversation can fit into the context
window it is still not uncommon that you
gbt may give a a codee doing a and c and
forget about B one way to understand
that is we can treat gbt as an intern
that have limited intelligence when it
spends the bring power on somewhere it
has to spend less power somewhere
else and in this example because the
requirements are scattered everywhere
Sean as the green texts gbt needs to
spend actual Intelligence on recognizing
what are the actual requirements from
this long and Massy taxt and that
distract it so it couldn't spend as much
Intelligence on the actual problem
solving and this is just the one side of
the story another potentially even worse
side is the majority of the contact
window is actually bad answers we were
not satisfied with gpt's previous
answers and that's why we further
iterate to add new requests so from the
perspective I probably answer latest
question all the previous answers are
useless or even wrong to some
extent this again distracts GPT it needs
to figure out oh this taxs are actually
wrong where are the problems and how to
fix them as humans we all know that this
is sometimes even harder than getting
something from scratch so overall it is
this scattered around the green prompts
and the long distracting red promps that
degrades gbt's
Intelligence on unfortunately this is
even not the end of the story when we
see AI BEC dumb and lazy what is our
first reaction we chat more give it more
requests and hope this could correct the
problem but now you understand the
context window and its limits you can
see how this is not only not helping but
actually make things
worse because now we have even longer
chat histor to put in the context window
which means it's more likely to get cut
off and more distracting the
requirements are further scattered
around and the bad previous responses
are even longer that makes everything
worse so it's not self correctable in
the case of AI becomes dumb or forgetful
following our first reaction of chatting
more will make it even
worse I'll pause here to review what
just happened we now learn from L
research that the contact window is a
core concept deciding how smart CH GPT
may appear and how effective we could
use it based on this concept what
previously appears frustrating upset and
even weird behaviors now suddenly begin
to make sense when we chat with chat gbt
is nothing more than appending our chat
history to its contacts window and more
chat ends up with a Messier contact
window which will result in a dumb or
forgetful or lazy AI our first reaction
is to chat more to correct it but it
simply doesn't work it's not really our
fault
is mostly rooted from how chbt is
designed it's designed to encourage us
to chat and that inevitably leads to
this
situation but does that mean that we
have to live with it not necessarily
before touching the actual solution
let's detour a little bit to learning to
drive for a moment when we learn about
driving in many cases our first reaction
is actually wrong for example when the
tire blows humans first reaction is to
hit the brake
but it's wrong the best practice is
actually to live it alone and gradually
decelerate the question is how can we
know what is the best practice and how
can we really do that in real
life the answer is simple through
training training tells you how a
technology Works internally tells you
what's the best to practice and why and
helps you practice until make it a habit
until you remember it in your muscle
memory and it also applies to chbt to
properly use chbt we need training too
we need training to correct our firstly
reaction and use good practice to
replace
it and for our specific problem the
solution based on our previous
understanding is we need to manage our
contact window
intentionally instead of blindly
accepting trb suboptimal design we
should proactively think about what our
contact window should look like is it in
the best shape for G to work well if we
follow this mindset it will be easy to
come up with a much more effective
prompt or context window for example
simply put all of the requirements in
one paragraph with nothing else write a
Python program to do a b and c I can I
cannot use
npy but how do we do that does that mean
that we need to start a new conversation
every time when I need to chat that's
not stupid fortunately we have a hidden
feature in the chat gbd UI helps us to
do this contact window
management and that is this small pencil
button below our question it means edit
we can click here to edit the current
prompt and there will be a button say
save and submit clicking it will change
this prompt in the context window rather
than append it to the context window
let's take a look at example here a side
note is we are using gbd 3.5 here
because it has a smaller contact window
and it's easier to trigger those
behaviors gbd4 has the same issue and
benefits from the same trick it's just
not that friendly for this 30 minute
long lining
course come back to our example it's
following the previous example of
copying files from a computer to another
here we further ask chbt oh I cannot
upload the files to the cloud storage
what are my options chbt gives a very
short answer without any detailed
instructions but if we use the addit
trick not how we change the prompt here
we simply copy paste the second prompt
after the first prompt making it a
complete request oh I want to copy files
from computer to another and I cannot
upload the files to the cloud then
suddenly Char becomes more diligent and
intelligent it gives details and
specific and organized answers this is
very different behavior from before and
note here we still use the same model
even the prompt is the same
what was changed is the content of
contact window we intentionally make it
precise and brief and this is the core
of context window
management we can continue the
conversation back to the chat style if
we further ask chbt following the
previous conversations I'm copying files
from a Mac to a PC what shall I do then
chbt gives a bunch of solutions the
third of them is clock storage and
actually we literally just said I cannot
upload files to the cloud in the
immediate previous round stupid right
how to fix it you tell me contact window
management instead of relying on chbt to
append everything and construct its own
contact window we constructed by
ourselves simply append I'm copying the
files from a Mac to a PC to our previous
prompt and that's it gbd suddenly become
smart just like a different Ai No cloud
storage anymore
so let's reflect on this example why did
it work what is the internal mechanism
so that I can use it somewhere
else in the chat style we have the asks
or requirements scattered everywhere and
GPT needs to spend its intelligence on
recognizing and understanding the
requirements but in the added style the
context is well organized with claim
statements on what needs to be done so
GPT could save the intelligence to
really focus on solving the problem in
the chat style Cha's imperfect or
incorrect answer becomes a major part of
the prompt and that's both a waste and
sometimes a distraction of the context
window on the contrary this is not
included in the edit style and we have
efficient use of the context window
meanwhile thanks to the editing feature
we still keep the same ease of
use actually there's the bonus benefit
of the editing style that is the
reusability when we write prompt to help
chbt accomplish some task The Prompt is
not only to explain what we expect from
it but more importantly to correct its
undesired behaviors for example due to
some reason we cannot use numpy in the
company's computer but trb likes to
solve the problem using this Library so
we need to include this requirement in
the prompt however when we use the chat
style it's nearly impossible for us to
use the prompt because such kind of
Correction of unexpected behaviors are
scattered all around the place across
many conversations but for the addit
style use we always have the latest and
greatest The Prompt at hand summarizing
all the requirements and Corrections
ready to use this is especially helpful
in AI assisted programming which we will
cover in the full
course and what we just experienced is
not only a journey to effective use of
chbt but also a great example of
training before training we Face a lazy
forgetful and dumb AI rely on our first
reaction to use it and blame open AI for
when we see unexpected
behaviors after the training we
understood how it works internally we
made made sense of all those behaviors
and learned about the best practices
which is addit not chat the training
lets us know the best way to use chbt is
actually to not chat and in the full
course we will also help you to
exercises to form a habit to make it a
muscle
memory overall the general principle is
for more complicated tools you need more
training it applied to bikes cars planes
and AIS and you need people who knows
the ins and outs and people who know how
to teach to train
you in the full course we will also go
over different components of chbt and
have a comprehensive overview on how to
prepare yourself in terms of mindset
knowledge and practical tricks like
addit not chat we already had the first
two cohorts sold out and the current
cohort will begin from July
22nd all right this is it for linning
course hope you enjoy this and I'm happy
to take any questions let me go over the
chat window to figure out what are the
questions about uh Google so the
question is didn't Google recently void
this issue with the infinit window model
uh yes in the recent I think that's in
last a few days Google proposed this um
but it's my understanding is still in
the pretty research phase after it it
gets into production hopefully we will
have less burden in manually managing
all this context window but before that
this is a pretty easy easy to use and
effective
trick uh and I saw that some of the
questions are already answered and Eugen
is also online to help answer the
questions and the question could you
point us to some resources where we can
dig more into the foundation of L
understand more critical Concepts like
concept Windows this is a pretty new
field and I will say most of resources
online is pretty not unsystematic and uh
at least for this field the this this
makes us painful and we like to have a
systematical overview of what was
important from our perspective so to
direct answer Nick your question um the
best place probably is our our course
but if we see some other resources they
are not a very systematic one on top of
my mind um if I see in the future
probably we can post it somewhere um
such as in the community of our course
what is the target audience of this
course what can we get out of this
course in the end if we are uh talking
about the main course the target
audience of this course is uh the
Professionals in the IT industry by that
I mean we need to have some not basic
knowledge of python such as we know how
to you need to know how to run python
programs you need to know how to read
simple python programs and install
python libraries you don't need to be a
professional developer um for example
PMS TPMS engineering managers uh
analysts the or the target audience of
this course um but we do require some
basic python knowledge so if uh you're
out of the it Prof industry that might
not be the best course for
you and what we can get out of this
course in the end is the general idea is
Gen gen is a pretty effective tool to
boost our productivity but it's actually
there are a lot of petful and nuances as
we just demonstrated in this process if
we understand better from the foundation
and know about the best practices this
will help us a lot in boosting our
productivity my personal experience is
Boost like two times to five times of my
productivity and we' like to also share
this with our the course audience that
is the target outcome of this
course so on us sandbox versus chat
interface that's a great question I I
actually I think using sandbox is a
pretty good way of using uh the gbt API
or chat gbt uh some background is
sandbox is a tool provided by openai
it's developer oriented instead of
showing you a chat interface it in
directly invokes the underlying GPT API
so it's a little bit different from the
chat interface in a few ways
uh difference one is chpt is a product
built on top of GPT API so it has quite
some additional prompts and limits and
features but the sandbox doesn't have
that which means you may not have the
alignment or Safeguard of chbt or other
limits of char BT so you may be able to
get into the dark side of GPT which
means it may not be that safe and the
second is second difference is there is
also some limit POS on the CH on the
chbt for example gbd4 has a contact
window of
128k but in chbt you cannot use that
long before that you will be cut by Char
saying that oh your message is too long
but in the sendbox you are able to use
that the third difference is the uh
maintenance of chat history in chbt web
interface chbt will maintain the context
window as well as the chat history for
you but in the sendbox a little bit more
manual process
which is good thing and from the
perspective of of this course because it
forces you to manually manage the
context window and the fourth uh
potential difference is the price charb
is a subscription based $20 per month
but sendbox is uh per
token so overall it's a pretty
interesting idea to use sendbox I if for
anyone having haven't tried that before
I encourage you to try that it's quite
unique and fun experience and that might
be the right solution to to the context
meal
management do we have an agenda of this
course yes uh in terms of cohort dates
we have that in the latest slide and
feel free to also scan the QR code so
that you get to uh the cord homepage and
know more about the course such as the
uh the the uh syllabus the expected
outcome target audience we have every
everywhere we have everything
there s on using iterations of a highly
structured prompt by edit and or having
start by asking questions to improve
context of prompt then incorporating
into
prompt um that's more about prompt
engineering that's my understanding of
this question um and we do have some
tricks around that but that may be out
of the scope of this context window but
overall yes having the prompt formulated
in a more structured way is definitely
helpful for making trbt smarter as we
mentioned before tra is like it's pretty
much like an intern actually I really
like this analogy it's it's energetic it
doesn't know what is tiest and it can
work 247 but it needs some handh holding
and this kind of PR engineering is a
great example of handholding you cannot
assign a very complicated task to an
intern and expect him or her to
accomplish the task perfectly you have
to do some handholding uh and
potentially even babysitting and prompt
engineering and contact window
management are very good examples of
star
Point um and what might be a more
beginner noice oriented geni
course that really depends on your
background and the intention of what do
you want want to learn and my suggestion
is actually if you want even more
beginner or novice um course probably of
course is not the best option directly
go to the the chat.com or other similar
products begin using that and begin to
feel the capability envelope of geni is
probably the best um thing to do to to
begin with because this will give you a
feeling and
then you also give you some questions
with the questions it will be easier for
you to figure out oh what I want from
this a course like that what are the
pinpoints what can the AI bring to me
what I I think getting those questions
might be more important than figing out
the answers so to directly answer a
question probably the best course is
sign up a account using chbd from today
it seems that the price of course
increases per cohort will that be a
trend um it sounds like a you already
answered
that what are your thoughts on the
content of the course potentially
becoming outdated in mod models iterate
for example context window and
information infinite window model that's
that's a great question uh sounds like a
e already addressed that but generally
the idea are two folds the first is the
content of course can be be basically
divided into two things one is the
underlying research and another is based
on those research that derve the tricks
and skills um for a lot of fundamental
things they will not change in a long
time for example the initial com initial
gbt model has been proposed like six
years but it's the basic PR principle
Still Remains the Same and another side
is we do see this field is growing very
fast that's also reason why ourselves
are Inus as in Inus as of this field and
we keep up with the field every day um
we try to figure out what are the most
important tricks and principles and
distill them into the course to make it
up to
dat um due to the limit of time we will
take the last question here um Yen have
you played around cloud. a pick out of
curiosity yes I played with it and it's
it's quite interesting uh in terms of
two aspects the first is I found that
the difficulty of managing my contact
window is much less for cloud V3
especially Opus more more um
specifically for a lot of prompts and
contact Windows gbt may be lazy so we
need to do more tricks to make to like
massage those prompts and contact
Windows to make it work but for cloud V3
we don't need to do that tricks from
this perspective we can see a trend that
people also realize the problem with
contact window and Make it try to make
it more user friendly and from another
perspective I also feel gbts 4 is still
a little bit smarter than Cloud a floud
V3 but that may be just my feeling based
on limited
tests so due to the limit of time uh
that's it for the Q&A and really
appreciate everyone's time and interest
for attending this course we look
forward to see you in the actual course
thanks a lot bye
Browse More Related Video
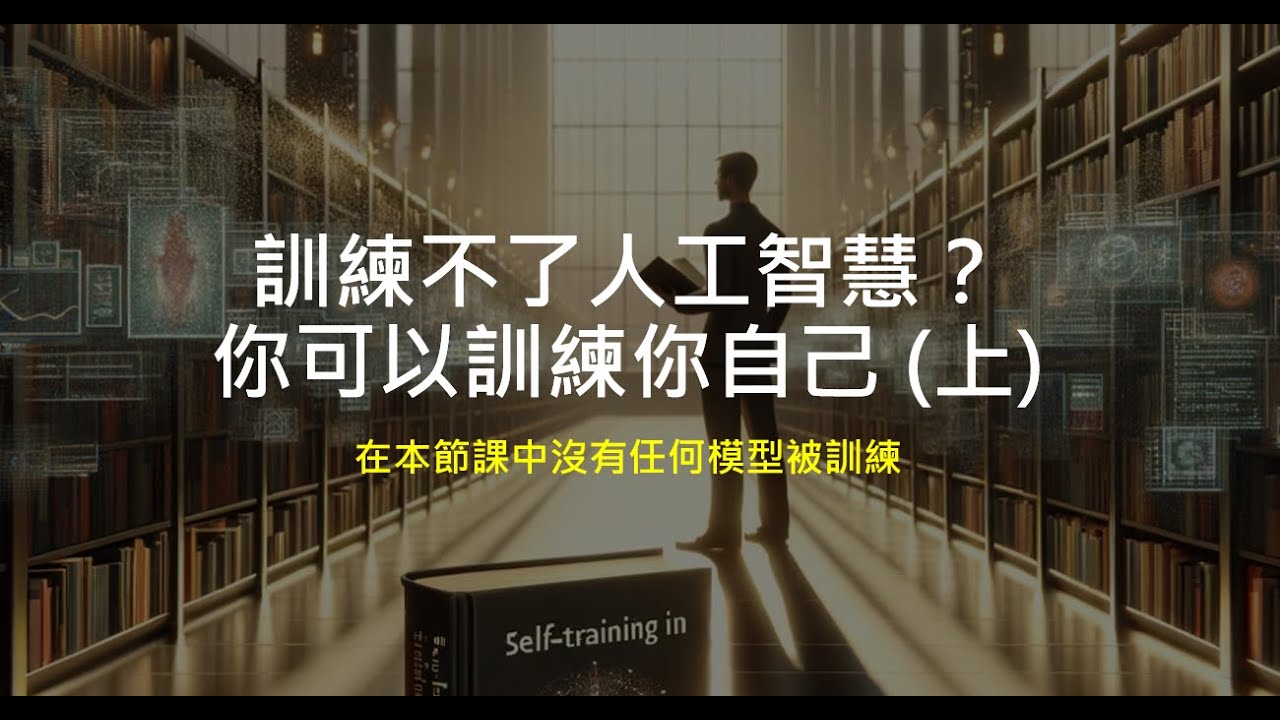
【生成式AI導論 2024】第3講:訓練不了人工智慧?你可以訓練你自己 (上) — 神奇咒語與提供更多資訊

Live demo of GPT-4o coding assistant and desktop app

Self-reflective RAG with LangGraph: Self-RAG and CRAG
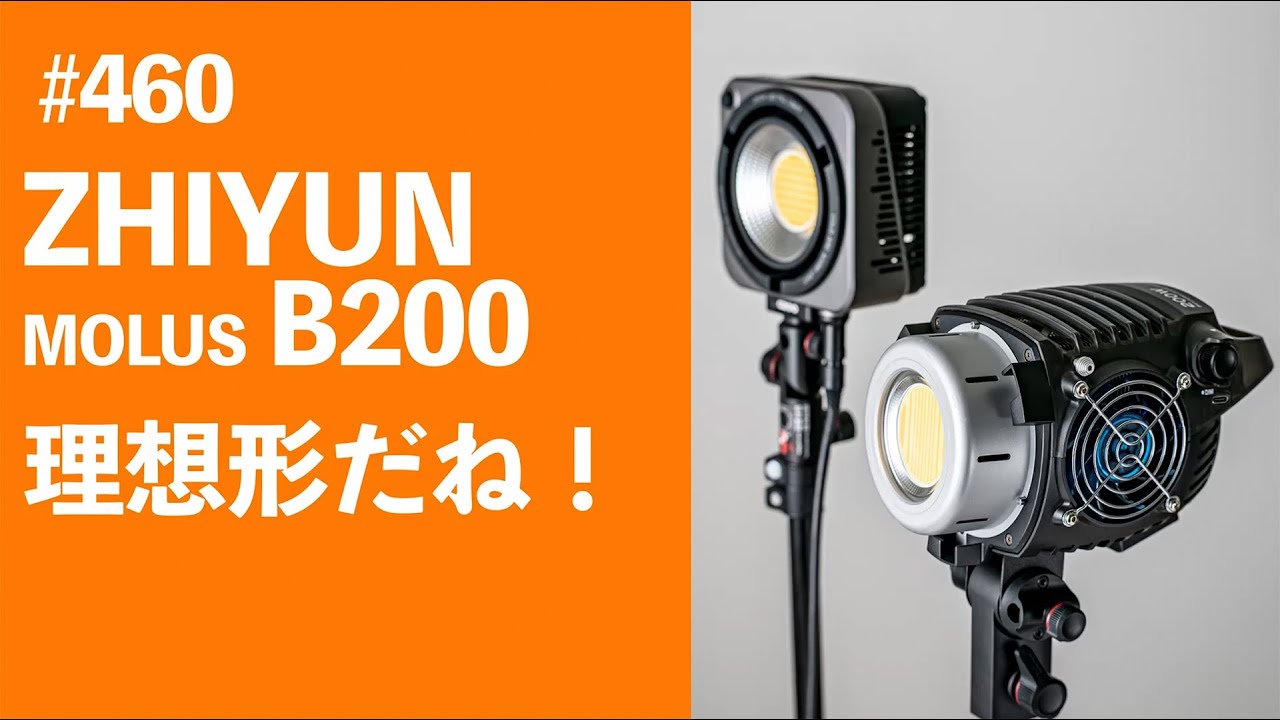
#460 ZHIYUN MOLUS B200 理想形だね!
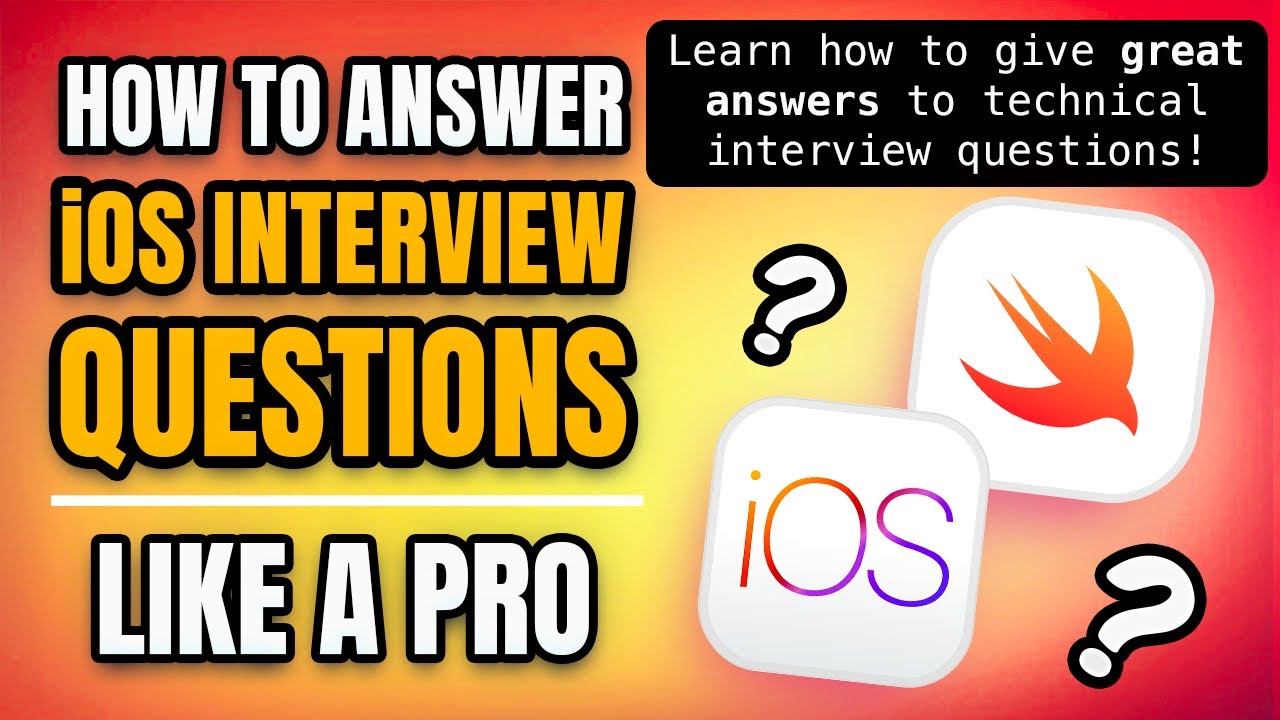
How to Answer iOS Interview Questions Like a Pro 👩🏽💻👨🏻💻 (free training course)
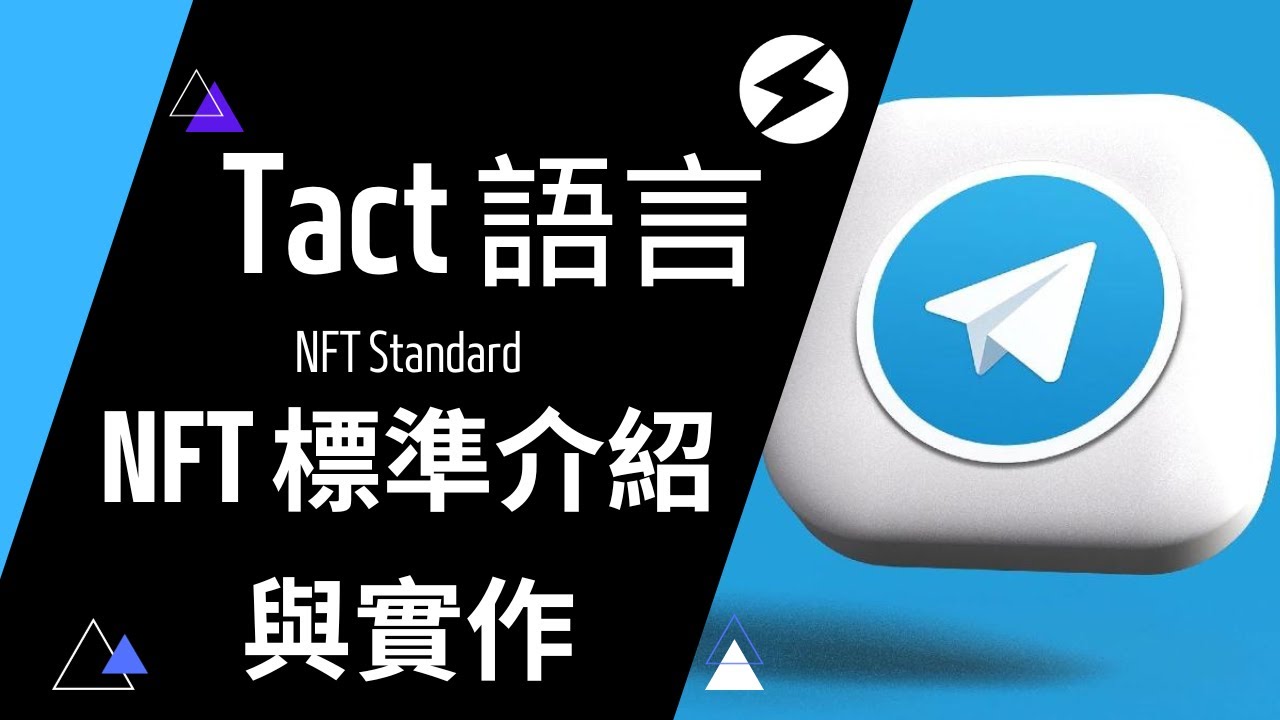
快速上手了解智能合約(NFT標準) | TON Blockchain, TEP62
5.0 / 5 (0 votes)