Giulio Biroli - Generative AI and Diffusion Models: a Statistical Physics Analysis
Summary
TLDRこのスクリプトは、最近の扩散モデルに関する研究を紹介し、生成的AIの進歩とその物理的な意味を探求しています。扩散モデルは、画像やテキスト生成の最先端に位置しており、興味深いトピックです。スクリプトでは、扩散モデルの仕組み、特にその逆プロセスとその中的での物理的な性質について詳細に説明し、データの次元数やデータの量がどのようにモデルの性能に影響を与えるかについても議論します。また、扩散モデルの理論的な背景と、その精度に関する数学的な結果も触れています。最後に、研究者が取り組んでいるいくつかの重要な課題についても触れています。
Takeaways
- 🌟 拡散モデルは非常に興味深く、特に物理学からの多くの知見が適用されています。
- 🔍 拡散モデルは生成的AIの分野で重要な突破を果たしており、画像やテキスト生成の分野で結果を出すことが示されています。
- 📈 拡散モデルは時間の経過とともに画像を白ノイズに変化させ、逆に白ノイズを画像に戻すことで新しい画像を生成します。
- 🔄 拡散プロセスは前向プロセスと逆のプロセスであり、その時間スケールが重要です。
- 🎯 拡散モデルの学習には、スコア関数の近似に関する重要な役割があります。
- 📚 理論的には、十分に近似されたスコア関数を持つ拡散モデルは、データの分布を適切に近似できます。
- 📈 データの次元とデータの量のバランスが重要であり、異なる時間スケールでの拡散モデルのパフォーマンスが異なります。
- 🧠 データの分布が特定のデータセットに関連する場合、拡散モデルはデータの重みを正しく捉えることが難しい可能性があります。
- 🔧 拡散モデルの学習には、データ次元とデータの量の関係、および近似クラスの選択などの重要な要素があります。
- 🌐 拡散モデルは高次元のデータにも適用され、その次元とデータの量の関係が複雑な問題を解く上で重要な役割を果たします。
- 🚀 拡散モデルの研究は進化し続けており、より複雑な問題や実際の応用にも取り組むようになっています。
Q & A
ディフュージョンモデルとは何ですか?
-ディフュージョンモデルは、画像やテキストの生成に使用される方法であり、特に最近の進歩の中で突破的な成果を生み出しているとされています。
ディフュージョンモデルが注目される理由は何ですか?
-ディフュージョンモデルは、高品質な画像やテキストを生成することができ、その能力が多くの分野で価値があると認識されているため注目されています。
ディフュージョンモデルがどのように動作するか説明してください。
-ディフュージョンモデルは、最初にノイズを含む画像を生成し、徐々にそのノイズを減少させることで、最終的にクリーンな画像を生成するプロセスを経て動作します。
ディフュージョンモデルの数学的な美点は何ですか?
-ディフュージョンモデルの数学的な美点は、そのシンプルさにあります。モデルを理解し、実装することが比較的容易で、効果的な結果を生成することができるためです。
ディフュージョンモデルを学習させるためにはどのような手順が必要ですか?
-ディフュージョンモデルを学習させるためには、時間逆転のプロセスを理解し、スコア関数を学習する必要があります。深度学習技術を用いて、データからスコア関数を近似することが一般的です。
ディフュージョンモデルが生成する画像の品質はどのように評価されるのですか?
-ディフュージョンモデルが生成する画像の品質は、視覚的に評価されることが一般的です。また、画像の鮮明さ、解像度、リアルな表現力などの観点から評価することもできます。
ディフュージョンモデルを使用する際の課題は何ですか?
-ディフュージョンモデルを使用する際の課題としては、データの次元性、データの量、そしてモデルの適切な訓練などが挙げられます。特に、高次元でのデータ処理には計算コストが高くなり、適切な近似技術が必要です。
ディフュージョンモデルの応用分野にはどのようなものがありますか?
-ディフュージョンモデルは、画像生成、テキスト生成、音声合成、データの復元や增强など、多くの分野で応用されています。また、アート作品の作成や新しい資料の発見など、創造的な分野にも活用されています。
ディフュージョンモデルの研究が進むことで、どのような影響が期待されますか?
-ディフュージョンモデルの研究が進むことで、より高品質な画像やテキストの生成が期待されます。また、データの欠如やノイズの多いデータを扱う能力が向上し、医療分野や自然災害の迅速な対応など、社会的な課題に対する貢献が期待されます。
ディフュージョンモデルと他の生成モデルとの比較において、どのような特徴がありますか?
-ディフュージョンモデルは、生成モデルの一種であり、他のモデルと比較して、特に画像や音声の高品質な生成能力が特徴です。また、データの前処理や後処理が比較的少ないため、シンプルな構造を持ち、計算コストを抑えることができます。
ディフュージョンモデルを実装する際に注意すべき点は何ですか?
-ディフュージョンモデルを実装する際には、適切なデータセットの選択、適切なネットワーク構造の設計、そして適切な学習率やパラメータの調整など特别注意が必要です。また、過学習や欠学習を避けるために、適切な正則化技術を用いることも重要です。
Outlines
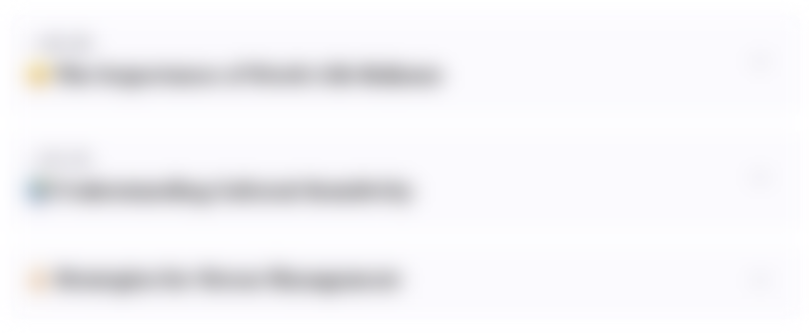
This section is available to paid users only. Please upgrade to access this part.
Upgrade NowMindmap
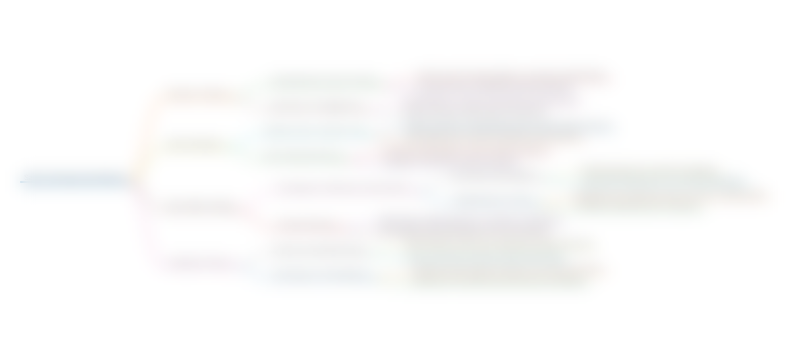
This section is available to paid users only. Please upgrade to access this part.
Upgrade NowKeywords
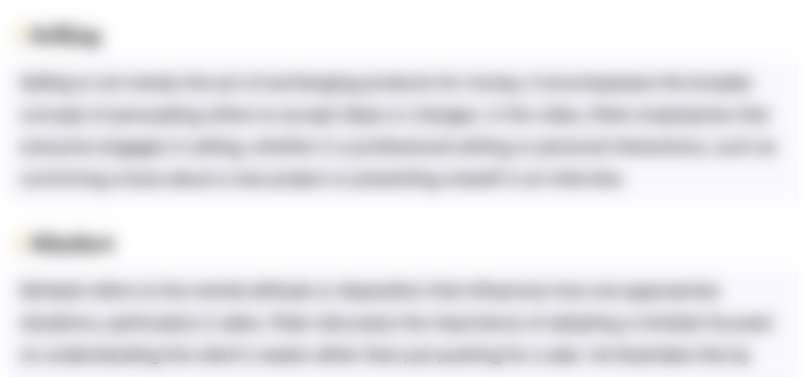
This section is available to paid users only. Please upgrade to access this part.
Upgrade NowHighlights
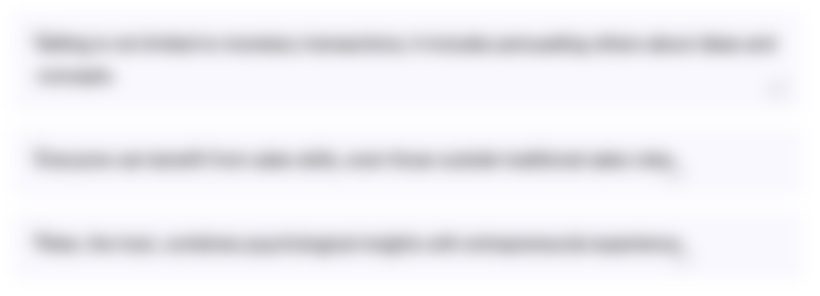
This section is available to paid users only. Please upgrade to access this part.
Upgrade NowTranscripts
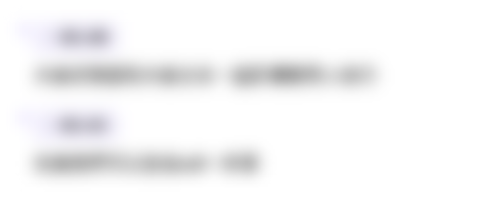
This section is available to paid users only. Please upgrade to access this part.
Upgrade Now5.0 / 5 (0 votes)