AI Learns To Play Golf
Summary
TLDRIn this video, the creator explores the development of a golf-playing AI using four separate neural networks for different golf clubs. Each network is trained with a reward system to optimize swing mechanics. Despite unconventional techniques, the AI achieves impressive results, with the driving AI hitting balls 210 yards. Challenges arise in adapting the algorithm for irons and short game clubs, but the AI shows promise, raising anticipation for its performance in an actual golf course scenario.
Takeaways
- 🤖 The video features an AI-controlled ragdoll named 'Block House' playing golf, utilizing a neural network to simulate a golf swing.
- 🏌️♂️ The AI is split into four separate neural networks, each specialized for driving, irons, chipping, and putting.
- 🚀 The driving AI is trained to swing the club with a specific direction determined by the cross product of vectors from the shoulder line to the club face and the right shoulder.
- 🎯 A reward system is implemented to guide the AI, focusing on club velocity matching the target direction and minimizing deviation from the swing plane.
- 📉 The iron AI is adjusted to account for shots typically taken from the ground, not an elevated tee, and emphasizes keeping the club flush with the ground.
- 🌐 The wedge AI is trained with an extra input to control swing strength and is tested on randomized terrains to improve adaptability.
- 💻 The putting AI focuses on accuracy and control, with a reduced backswing to encourage consistency over speed.
- 🤹♂️ Despite the AI's unconventional techniques, such as one-legged stance and face plants, it can achieve consistent and effective golf shots.
- 📊 The training process involves reinforcement learning, where the AI learns from rewards and punishments based on its performance.
- 🌟 The video concludes with a teaser for a future video where the AI will be tested on actual golf courses.
Q & A
What is the purpose of increasing the size of the golf ball in the AI simulation?
-The size of the golf ball is increased to overcome limitations in the physics engine where small objects tend to phase through solid surfaces. This also helps to better simulate how the club interacts with the ground during golf shots.
How many separate neural networks are used for the AI golfer, and what are they responsible for?
-Four separate neural networks are used for the AI golfer: one for driving, one for irons, one for chipping, and one for putting. Each network is specialized to handle the unique swing requirements for each type of golf shot.
What is the swing plane and how is it calculated?
-The swing plane is defined as the axis around which the golf club needs to be tilted to hit a perfect golf shot. It is calculated using the cross product of two vectors: one from the shoulder line to the club face and the other from the shoulder line to the right shoulder.
How does the reward system for the driver AI work?
-The reward system for the driver AI compares the velocity of the club to a target direction using the dot product. It also measures how far the club has deviated from the swing plane and gives a punishment that scales with that deviation.
What changes are made to the AI's swing when it needs to come back the other way after the backswing?
-The swinging reward is reversed once the club is past a certain point relative to the ball, and the swinging reward is squared to emphasize the importance of moving the club fast.
How does the AI's iron swing differ from its driver swing?
-The iron swing is similar to the driver swing, but with a slight tweak to the reward system to keep the club flush with the ground. The backswing is also shortened to reduce the swing speed, leading to a more precise swing.
What additional input is added to the neural networks for the wedge and putting AI?
-An extra input is added to control how hard the AI swings, shifting the focus from rewarding speed to rewarding how close it gets to the target speed.
How does the AI handle uneven terrain during wedge shots?
-The AI learns to hit shots on uneven terrain by simulating random platforms for the ragd doll to stand on during training, which makes it more resilient to uneven terrain on actual courses.
What adjustments are made to the putting AI to improve its performance?
-The putting AI has its backswing greatly reduced to focus more on consistency than speed. It also follows a set of rules to ensure proper putting technique, such as keeping the leg straight and hitting the ball at a 45° angle.
What are the results of the AI's training for different types of golf shots?
-The AI's training has been successful for driving and iron shots, with the AI capable of hitting long distances and maintaining a consistent swing. The wedge AI shows versatility in hitting from various surfaces, while the putting AI, despite some issues, is functional and can putt effectively.
Outlines
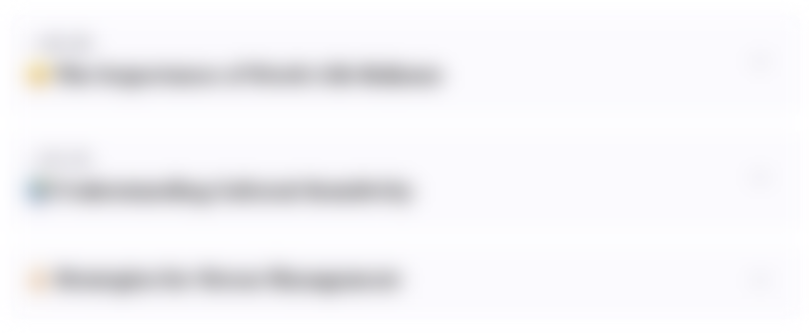
This section is available to paid users only. Please upgrade to access this part.
Upgrade NowMindmap
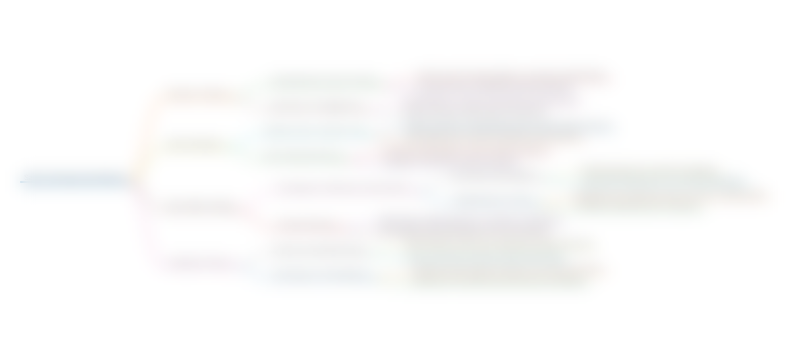
This section is available to paid users only. Please upgrade to access this part.
Upgrade NowKeywords
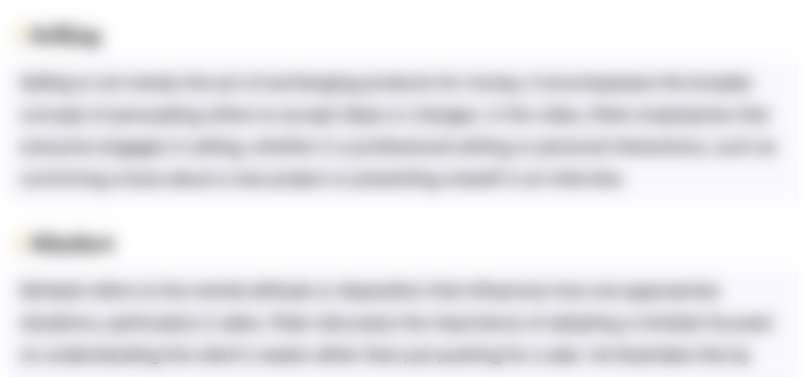
This section is available to paid users only. Please upgrade to access this part.
Upgrade NowHighlights
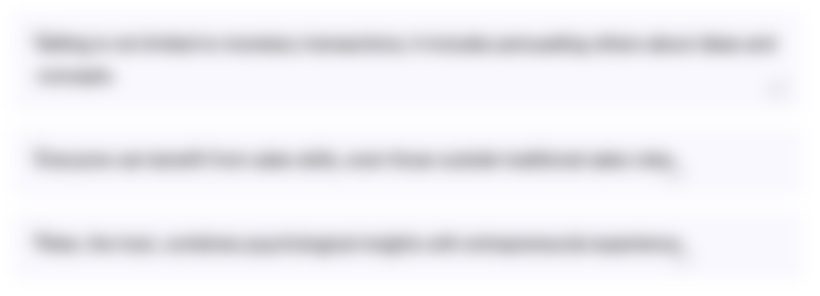
This section is available to paid users only. Please upgrade to access this part.
Upgrade NowTranscripts
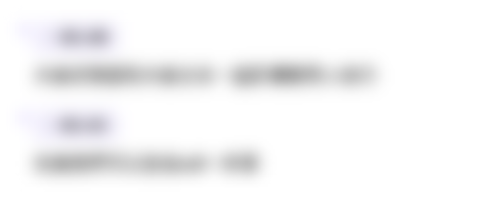
This section is available to paid users only. Please upgrade to access this part.
Upgrade NowBrowse More Related Video

GAME IMPROVEMENT IRONS vs PLAYERS IRONS | Which SHOULD You PLAY?

Deep Learning for Music Generation - Training GPT-2 on a large music dataset - Lakh MIDI Dataset
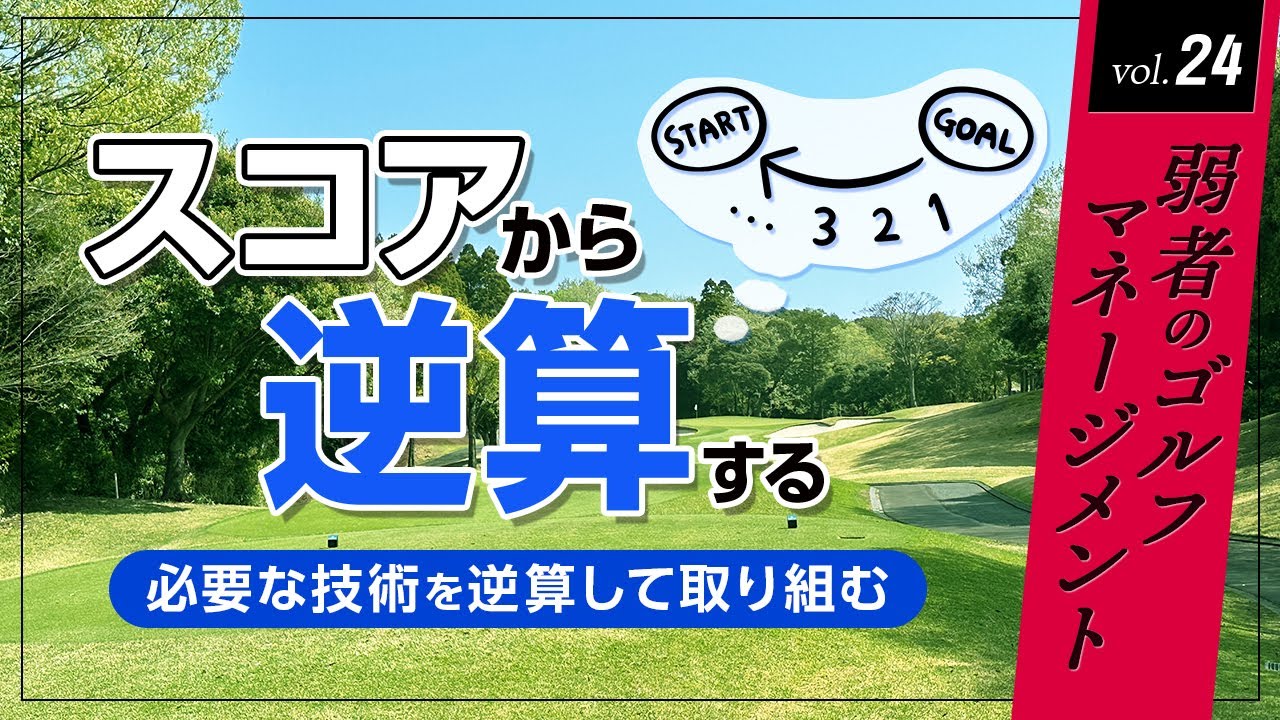
スコアから逆算する! 目的に必要な技術を逆算して取り組む練習とクラブ選び【ゴルフトレンドウォッチャー】
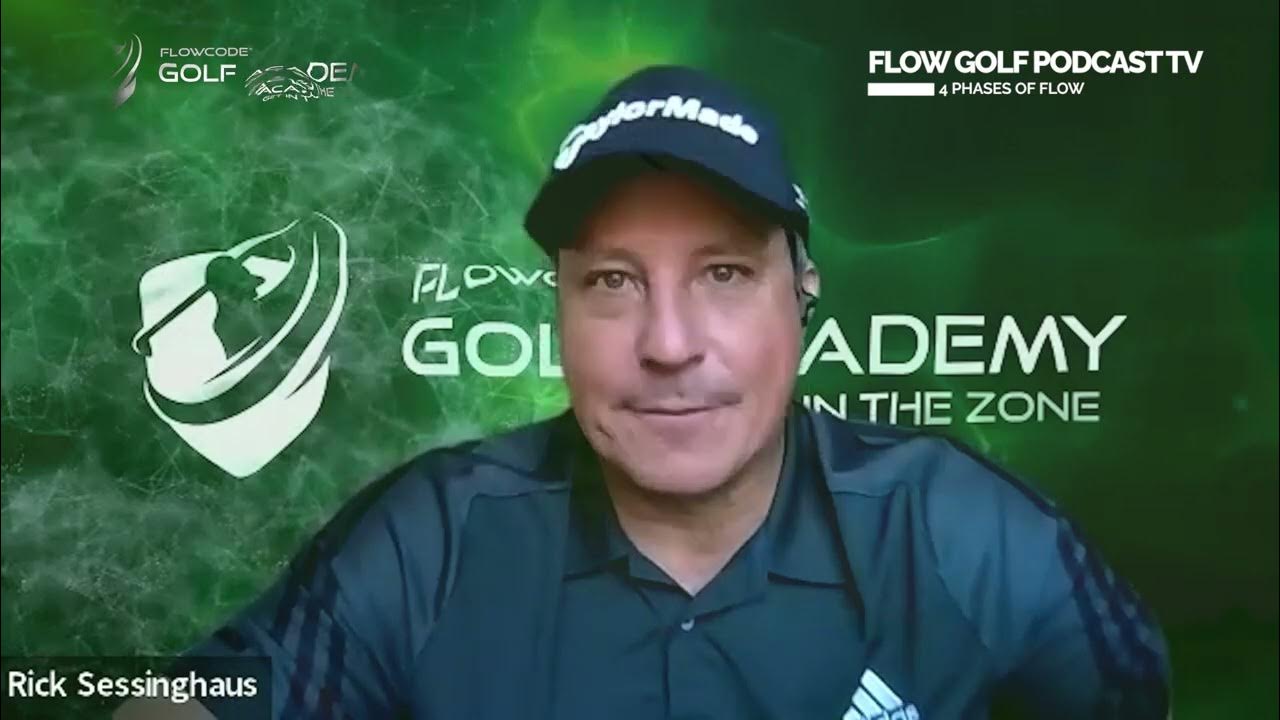
The 4 Phases of Flow | Flow Golf Podcast | Rick Sessinghaus & Hallam Morgan

My Game: Tiger Woods | Episode 8: My Mental Game | Golf Digest
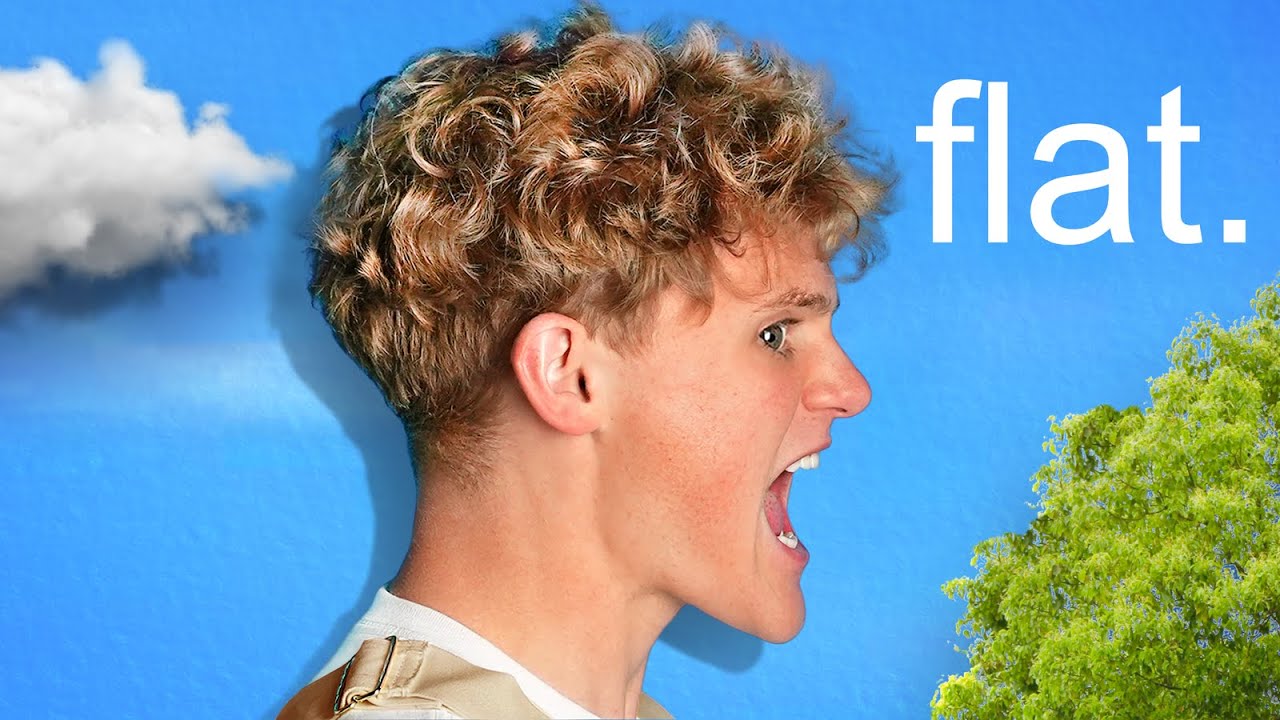
I Survived 50 Hours in 2D
5.0 / 5 (0 votes)