Four Ways of Thinking: Statistical, Interactive, Chaotic and Complex - David Sumpter
Summary
TLDRIn this enlightening talk, the speaker, an applied mathematician, delves into the power and limitations of mathematical thinking in understanding the world. He explores four distinct modes of thinking: statistical, interactive, chaotic, and complex. Through engaging stories from football, science, and everyday life, he illustrates how these approaches can provide insight but also emphasizes the importance of recognizing when statistical significance does not equate to practical importance. The speaker highlights the beauty of chaos theory and the concept of complexity, as defined by Kolmogorov, suggesting that true understanding comes from finding succinct descriptions of complex phenomena.
Takeaways
- 📚 The speaker is an applied mathematician whose motivation stems from a desire to understand the world around them, rather than a love for calculations themselves.
- 🔢 The talk emphasizes four different ways of thinking: statistical, interactive, chaotic, and complex, using stories from football, science, and personal life to illustrate each.
- 👨💼 The speaker discusses the importance of experimental design and the contributions of Ronald Fisher, highlighting the power of statistics in understanding phenomena but also its limitations.
- 🏈 Football is used as a medium to demonstrate how statistics can measure aspects of a player's performance and attitude, challenging the notion that some elements are immeasurable.
- 🧬 The speaker introduces Alfred J. Lotka and his work on differential equations to model ecological interactions, such as predator-prey dynamics, showcasing interactive thinking.
- 🦋 The concept of chaos theory is introduced through the story of Margaret Hamilton and Edward Lorenz, illustrating how small differences can lead to vastly different outcomes, as in weather prediction.
- 🤖 An experiment is conducted during the talk to demonstrate the divergence of outcomes from small initial differences, reflecting the butterfly effect in chaos theory.
- 👨🚀 Margaret Hamilton's story is highlighted to show how a deep understanding of chaos led to meticulous control in critical situations, such as the Apollo moon mission.
- 🌐 The speaker discusses the balance between order (Yang) and chaos (Yin), suggesting that while we can control some aspects of life, we must accept the randomness in others.
- 🌟 The final point touches on complexity theory, suggesting that finding simple rules that capture the essence of complex systems can lead to a deeper understanding of the world.
Q & A
What is the main theme of the speaker's talk?
-The main theme of the speaker's talk is to provide insight into their thinking process in four different stages: statistical, interactive, chaotic, and complex thinking, using examples from football, science, and personal life.
Why did the speaker choose to discuss Ronald Fisher in their talk?
-The speaker chose to discuss Ronald Fisher because he was a significant figure in the development of applied mathematics and experimental design, and his work exemplifies the application of statistical thinking.
What is the significance of the milk-first or tea-first experiment mentioned in the talk?
-The milk-first or tea-first experiment is significant because it demonstrates the application of combinatorics and experimental design to solve a seemingly trivial problem, highlighting the power of mathematical thinking in everyday situations.
How does the speaker use football as an example to illustrate statistical thinking?
-The speaker uses football to illustrate statistical thinking by analyzing player performance metrics, such as how a player's performance changes when their team concedes a goal, and comparing these metrics to evaluate player attitudes and impact on team dynamics.
What is the 'Gary Neville statistic' referred to in the talk?
-The 'Gary Neville statistic' is a measurable statistic developed by the speaker to quantify a player's performance change after their team concedes a goal, which was initially thought to be immeasurable by Gary Neville.
What is the limitation of using statistics to measure concepts like 'grit'?
-The limitation of using statistics to measure concepts like 'grit' is that while it can provide some predictive power, it often only explains a small percentage of the variance in outcomes, indicating that many other factors contribute to success.
What is the role of Alfred J. Lotka in the development of interactive thinking?
-Alfred J. Lotka played a crucial role in the development of interactive thinking by introducing the concept of unbalanced chemical equations to model ecological interactions, such as predator-prey dynamics, which laid the foundation for understanding complex systems.
How does the speaker use the applause experiment to illustrate interactive thinking?
-The speaker uses the applause experiment to illustrate interactive thinking by showing how social behaviors, like clapping, can be modeled using the same principles as ecological interactions, demonstrating the spread of a 'social epidemic'.
What is the concept of chaos theory and how does it relate to the speaker's talk?
-Chaos theory is the concept that small changes in initial conditions can lead to vastly different outcomes in complex systems, making long-term prediction impossible. It relates to the speaker's talk by illustrating the limitations of control and predictability, even with advanced mathematical models.
How does the speaker's personal life example of moving a sofa with friends relate to interactive thinking?
-The speaker's personal life example of moving a sofa relates to interactive thinking by demonstrating how social interactions and cooperation can lead to a desired outcome, which is a simple model of how collective behavior can be understood and predicted.
What is the significance of the experiment involving doubling numbers and subtracting from 100 in the context of chaos theory?
-The significance of the experiment involving doubling numbers and subtracting from 100 is to demonstrate how small differences in initial conditions can quickly lead to divergent outcomes, illustrating the concept of sensitive dependence on initial conditions in chaos theory.
What is the 'butterfly effect' in chaos theory and how was it discovered?
-The 'butterfly effect' in chaos theory refers to the idea that the flap of a butterfly's wings in Brazil could set off a chain of events leading to a tornado in Texas. It was discovered by Edward Lorenz in the context of weather prediction models, where small differences in initial data led to vastly different forecasts.
Who is Margaret Hamilton and what is her contribution to the field of mathematics?
-Margaret Hamilton was a mathematician who worked on the Apollo moon mission, creating the software that helped navigate the spacecraft and control the thrusters. She is known for her attention to detail and her work in ensuring error-free computation in critical systems.
What is the fourth way of thinking introduced by the speaker and how does it relate to complexity?
-The fourth way of thinking introduced by the speaker is complex thinking, which is related to the concept of complexity as defined by Kolmogorov. It involves finding the shortest description that can produce a pattern, capturing the essence of complexity without losing detail or nuance.
What is the significance of cellular automata models in understanding complexity?
-Cellular automata models, like the Game of Life, are significant in understanding complexity because they demonstrate how simple local interaction rules can lead to the emergence of complex patterns and behaviors, showcasing the self-organizing nature of complex systems.
Outlines
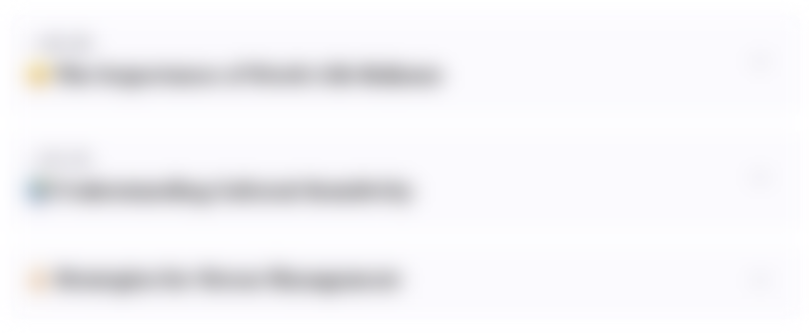
This section is available to paid users only. Please upgrade to access this part.
Upgrade NowMindmap
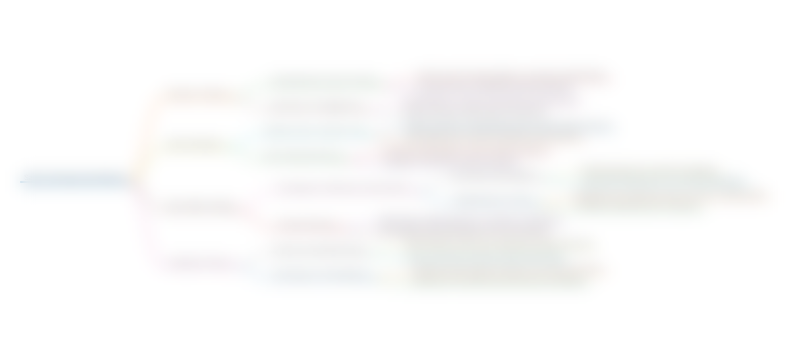
This section is available to paid users only. Please upgrade to access this part.
Upgrade NowKeywords
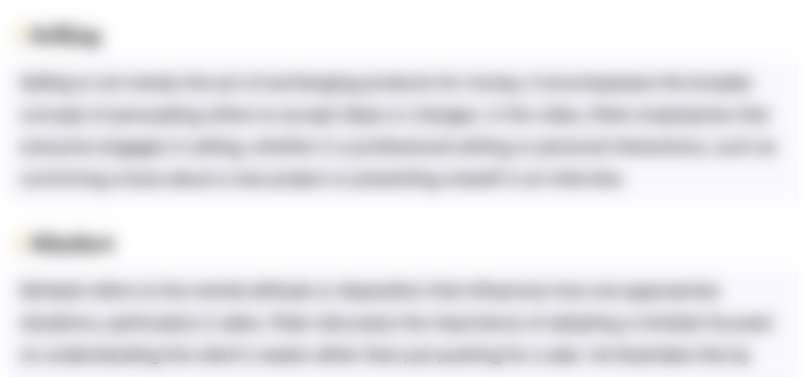
This section is available to paid users only. Please upgrade to access this part.
Upgrade NowHighlights
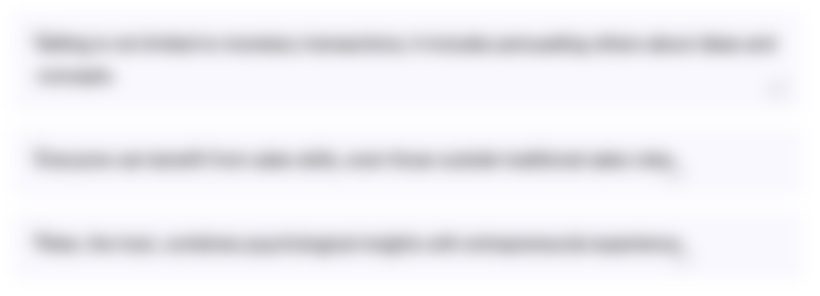
This section is available to paid users only. Please upgrade to access this part.
Upgrade NowTranscripts
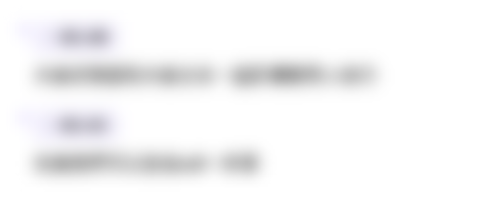
This section is available to paid users only. Please upgrade to access this part.
Upgrade NowBrowse More Related Video

Paul Erdős: The Man Who Loved Only Numbers [1998]
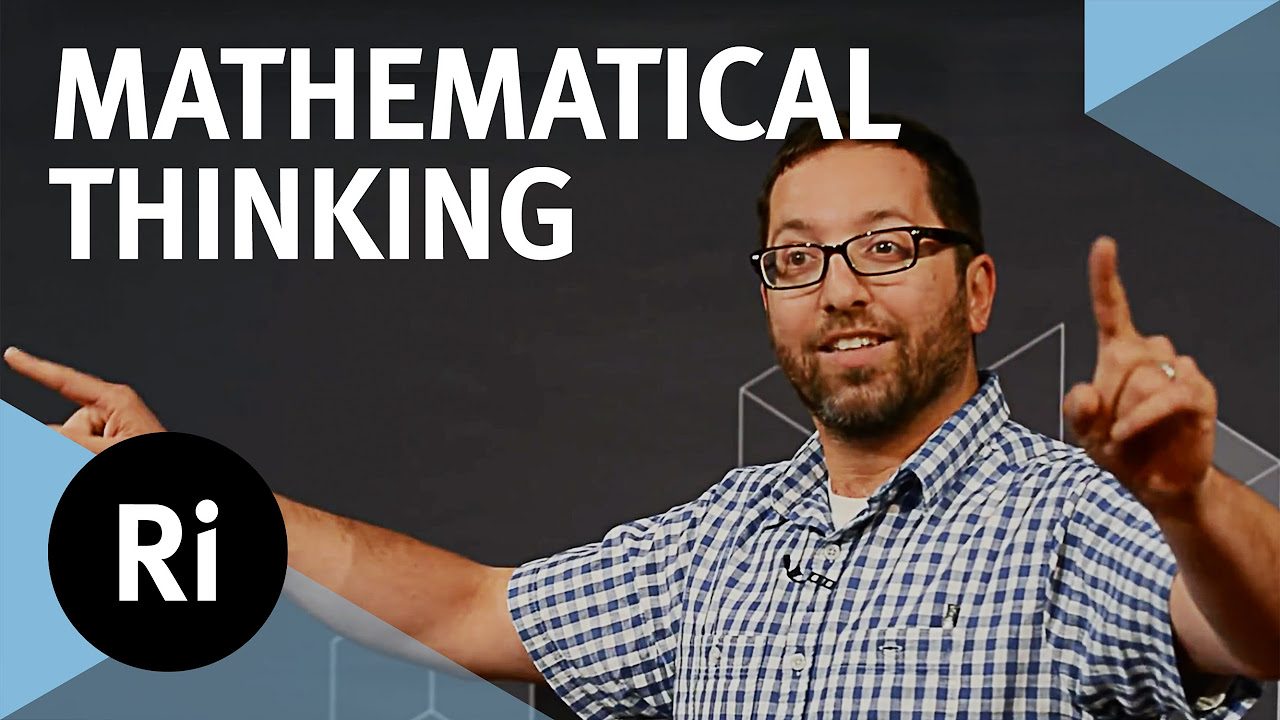
How Not to Be Wrong: The Power of Mathematical Thinking - with Jordan Ellenberg

Decoding the Secret Patterns of Nature - Fibonacci Ratio & Pi - Full Documentary

Soccermatics: how maths explains football | David Sumpter | TEDxUppsalaUniversity
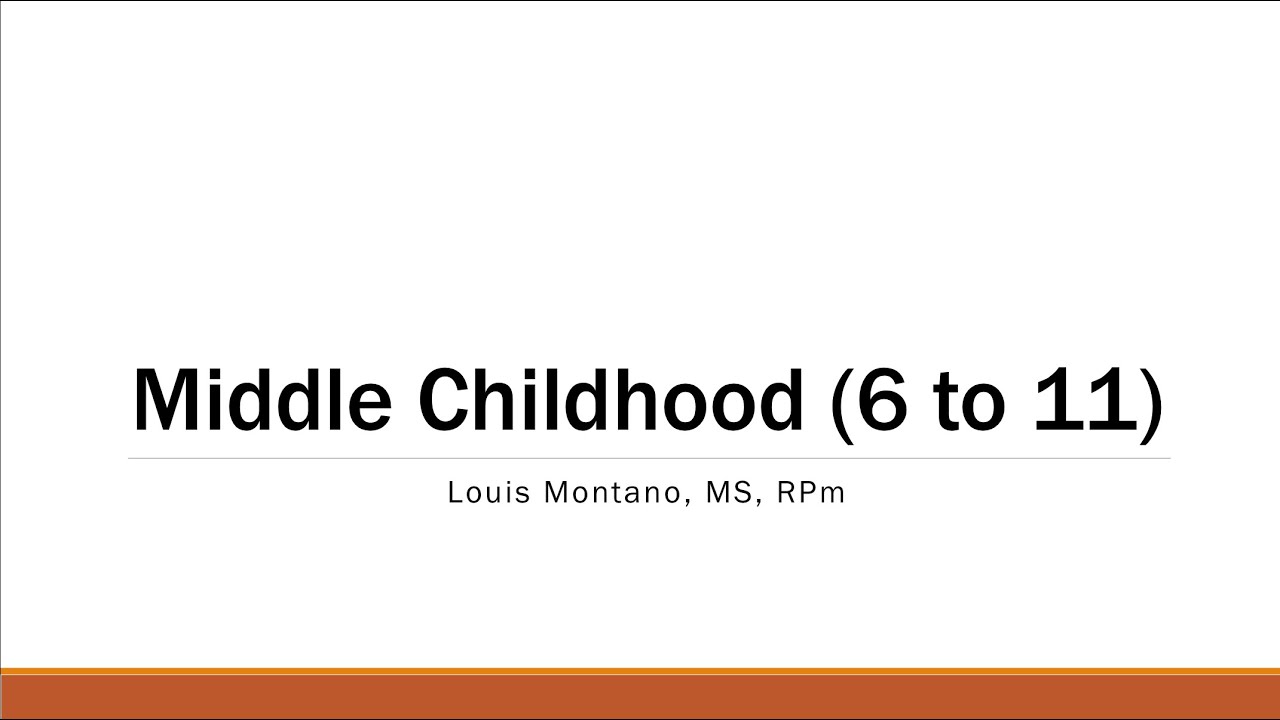
[DEVPSY] Middle Childhood Part 1

Bakit Kailangan ng Calculus Para sa Time Rates? Differential Calculus Explained In Tagalog/Filipino
5.0 / 5 (0 votes)