【早く気付け】今すぐやめるべきデータサイエンス学習法TOP5
Summary
TLDRThis video script addresses common pitfalls in learning data science and presents a top 5 list of inefficient methods to avoid. It emphasizes the importance of practical experience over theoretical knowledge, especially for beginners. The speaker shares personal experiences and success stories of his students to illustrate the effectiveness of focusing on real-world data analysis projects. The script promotes a learning roadmap that prioritizes gaining practical skills and building a portfolio through competitions like Kaggle to enhance employability in the field of data science.
Takeaways
- 📚 Avoid focusing solely on theoretical knowledge early in the learning process, such as deep learning theories, as it may not directly translate to practical skills needed for data science roles.
- 🔢 It's not necessary to study mathematics in-depth initially, especially for beginners. Instead, focus on gaining practical experience that can be applied in data analysis tasks.
- 🚀 Skip learning Docker for data science unless it's specifically required for a job you're targeting, as it's not typically a prerequisite for entry-level data science positions.
- 🛠 Prioritize learning only what is essential for the immediate goal of entering the field, rather than acquiring a broad range of skills that may not be immediately useful.
- 📈 Start building practical data analysis experience by participating in data analysis competitions like Kaggle, which closely mimic real-world data science tasks.
- 🏆 Kaggle competitions are an excellent way to gain tangible achievements, such as medals, that can showcase your skills to potential employers.
- 💡 The speaker emphasizes the importance of learning by doing, rather than memorizing libraries or syntax, which can be looked up as needed.
- 🚫 Avoid spending excessive time on foundational learning without progressing to practical application; this can lead to inefficiency and wasted time.
- 🕒 Recognize that time is a limited resource, and focus on learning in a way that minimizes waste and maximizes efficiency.
- 💼 For those looking to transition into data science, aim to gain practical experience as quickly as possible to make yourself more attractive to employers.
- 🌟 The speaker shares personal experiences and success stories of individuals who have overcome initial struggles and achieved rapid progress in data science by adopting the right learning methods.
Q & A
What is the main focus of the video script?
-The video script focuses on introducing the top 5 data science learning methods that one should avoid, and then suggests the most effective way to learn data science.
What is considered the most important factor when learning data science, according to the script?
-The script suggests that the most important factor is not the materials or the time spent studying, but rather the learning method itself.
Why does the script suggest avoiding the study of machine learning theory at the beginning of data science learning?
-The script argues that understanding machine learning theory is not the best starting point for beginners because knowing the theory and being able to apply it are separate issues, and practical application is more important initially.
What is the script's stance on studying mathematics for data science learning?
-The script advises against focusing on mathematics at the beginning of data science learning because it's not immediately applicable and can lead to wasted effort without a clear understanding of its use.
What is the script's opinion on learning Docker for data science beginners?
-The script discourages beginners from learning Docker because it is not a high priority skill for most job positions, and there are more urgent and important areas to focus on for someone new to data science.
Why does the script warn against memorizing Python libraries and syntax?
-The script suggests that memorizing Python libraries and syntax is not necessary because programming can be looked up on Google, and it's more important to focus on practical application and problem-solving.
What is the script's view on continuous foundational learning in data science?
-The script criticizes continuous foundational learning because it can be time-consuming and may not lead to practical skills that can be applied immediately in the field of data science.
What is the most effective method to learn data science according to the script?
-The script recommends participating in data analysis competitions like Kaggle as the most effective method because it closely resembles real-world tasks, helps build analytical skills, and allows for tangible achievements.
What are the three main reasons why participating in Kaggle competitions is beneficial for learning data science?
-The three main reasons are: it allows for learning in a format that closely resembles real-world tasks, it helps in building practical analytical skills, and it enables the creation of tangible achievements.
What kind of support does the speaker offer for those who wish to learn data science efficiently?
-The speaker offers a complete roadmap for learning data science in 3 months, available on their official website, which includes slides and other resources to help beginners efficiently learn data science.
Outlines
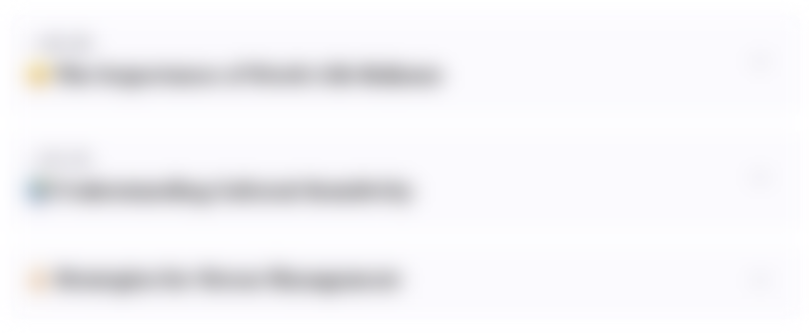
This section is available to paid users only. Please upgrade to access this part.
Upgrade NowMindmap
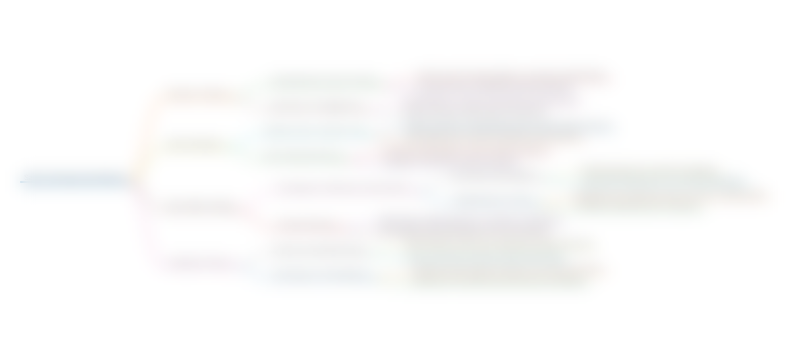
This section is available to paid users only. Please upgrade to access this part.
Upgrade NowKeywords
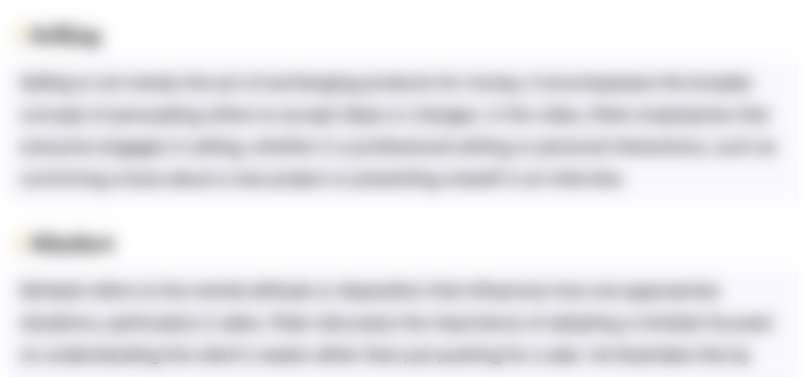
This section is available to paid users only. Please upgrade to access this part.
Upgrade NowHighlights
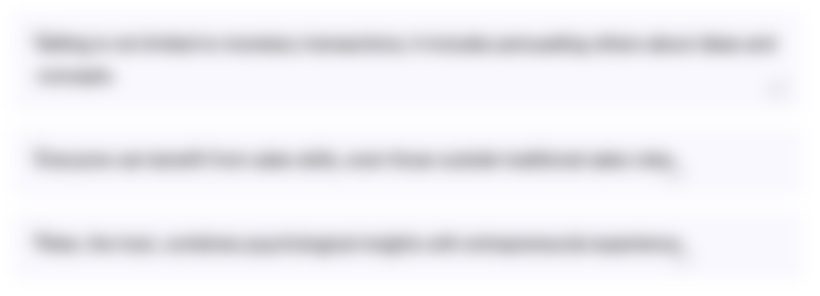
This section is available to paid users only. Please upgrade to access this part.
Upgrade NowTranscripts
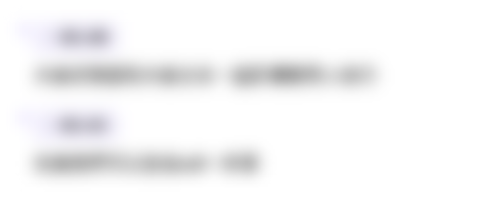
This section is available to paid users only. Please upgrade to access this part.
Upgrade NowBrowse More Related Video
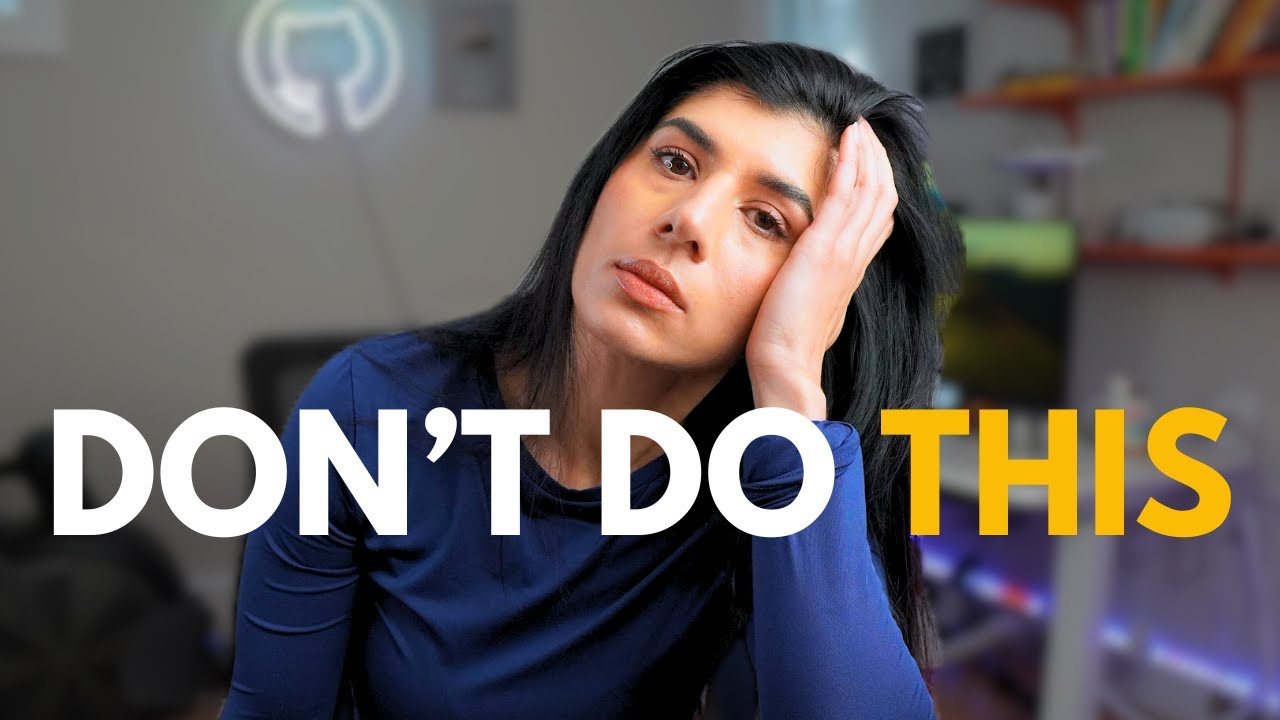
Starting a Career in Data Science (10 Thing I Wish I Knew…)
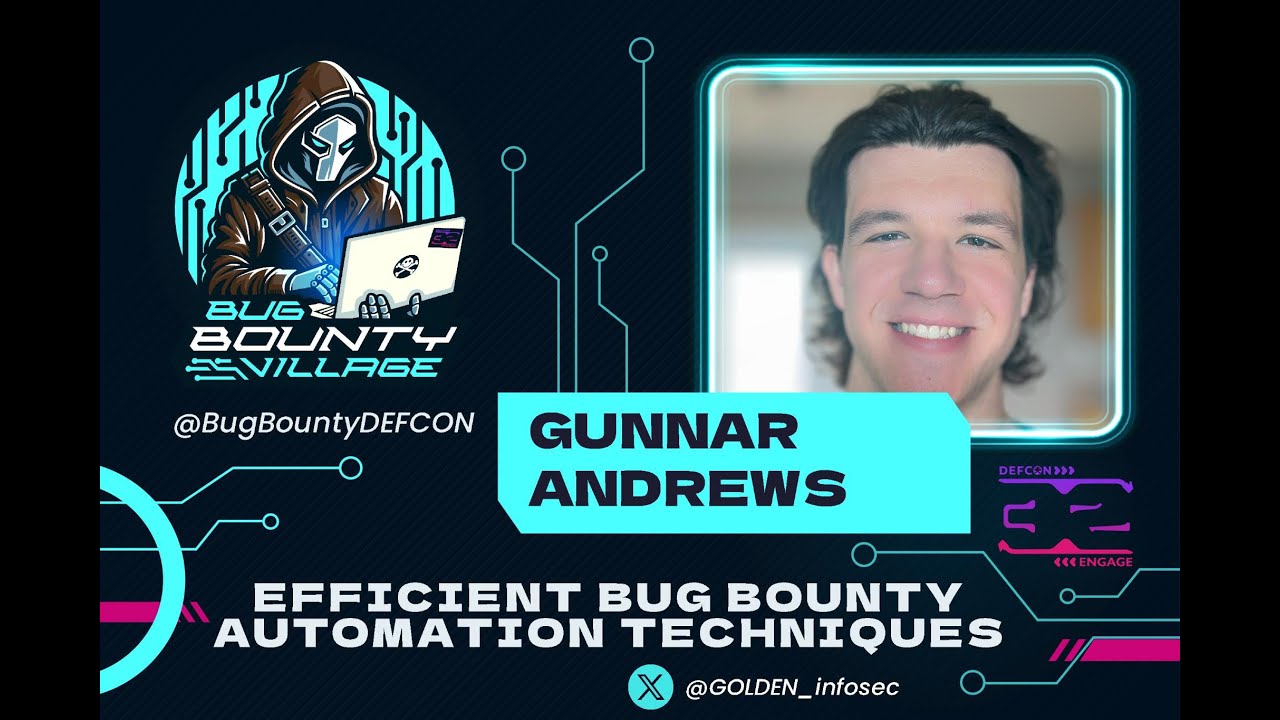
@g0lden1: Efficient Bug Bounty Automation Techniques | DEF CON 32, Bug Bounty Village
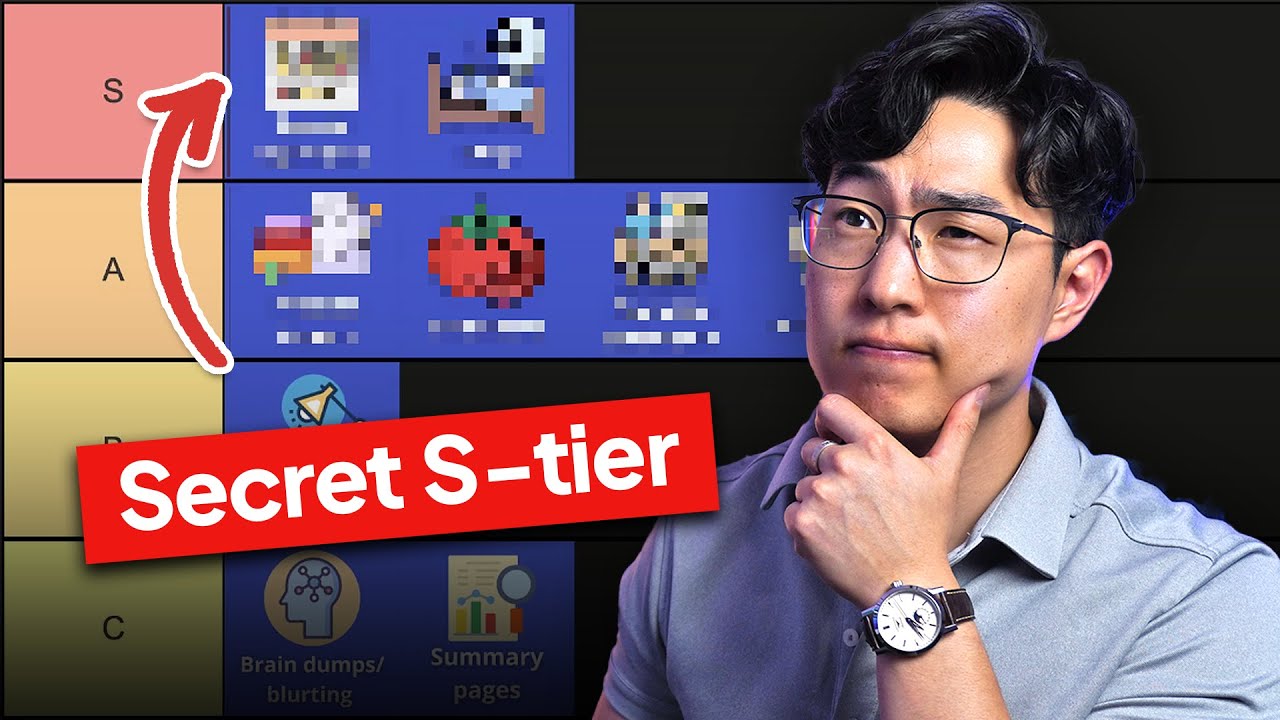
Ultimate Study Technique Tier List (Learning Coach Edition)
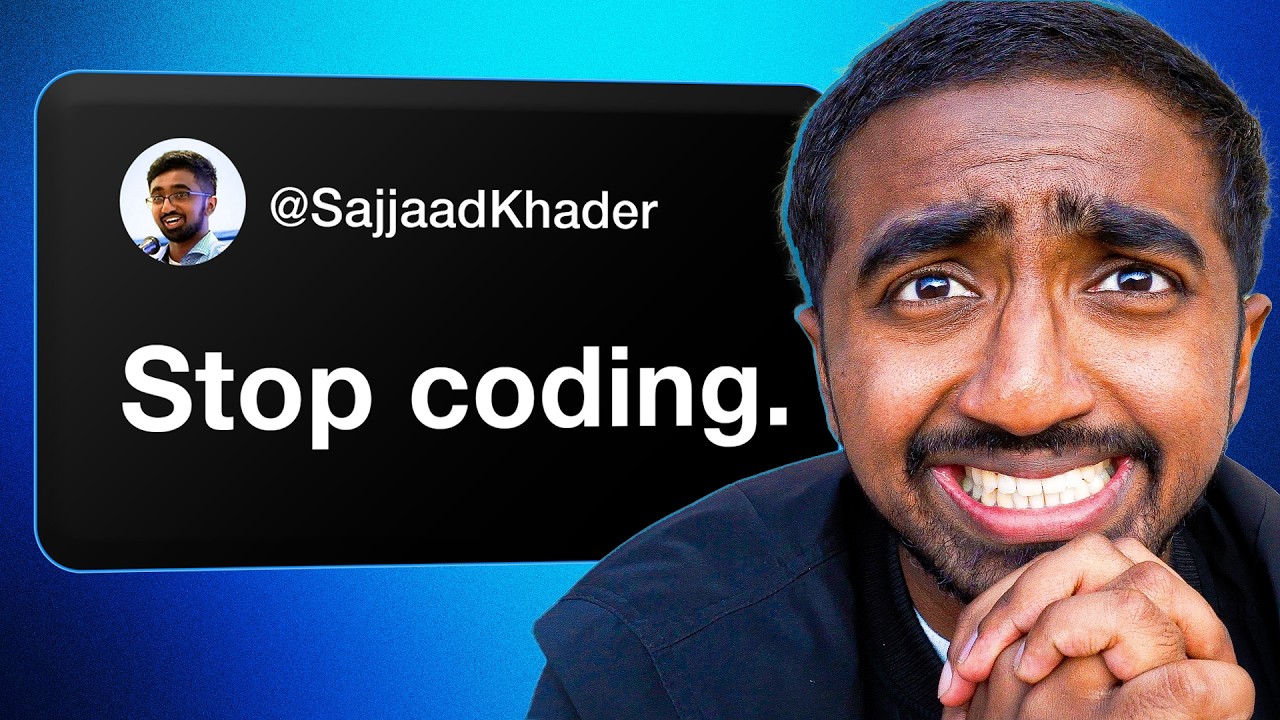
7 Years of Software Engineering Advice in 10 Minutes
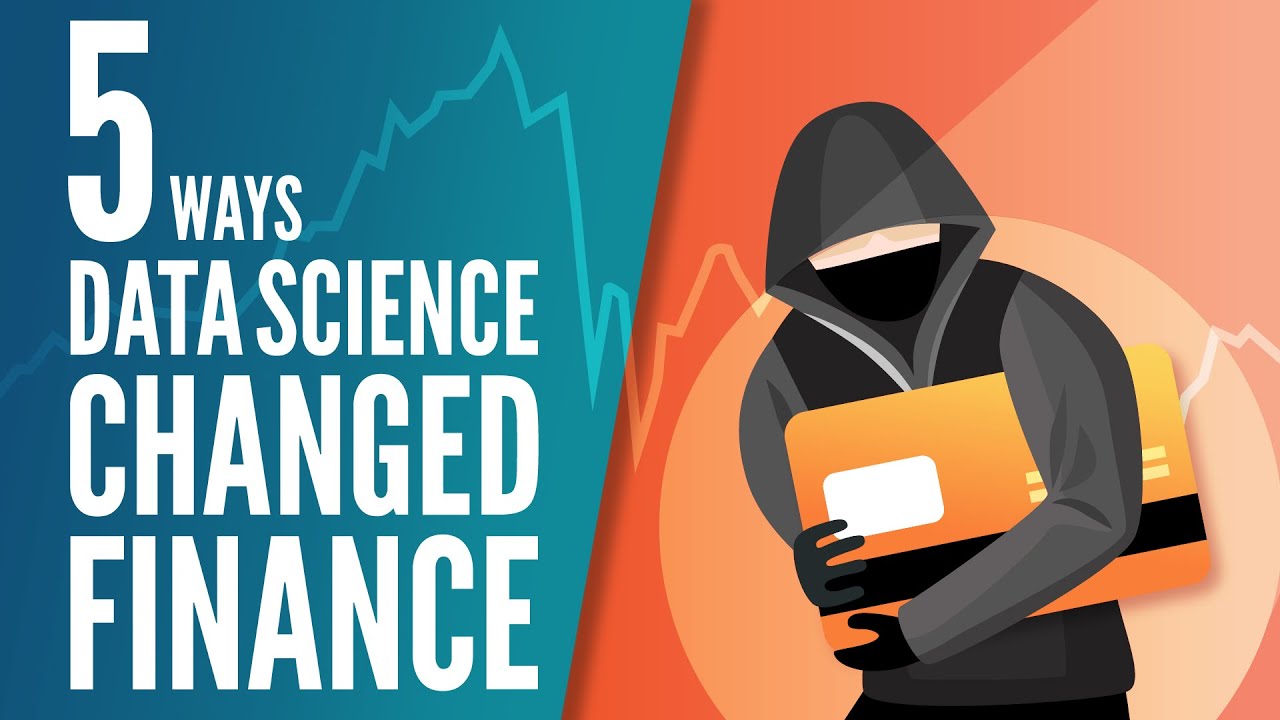
5 Ways Data Science Changed Finance

Top 17 BEST Data Science & Analytics Certificates (2024)
5.0 / 5 (0 votes)