5 Ways Data Science Changed Finance
Summary
TLDRThis 365 Data Science special explores the top 5 ways data science is revolutionizing finance. It covers fraud prevention, anomaly detection, customer analytics, risk management, and highlights algorithmic trading as the most impactful. The script delves into how machine learning and deep learning enhance security, predict consumer behavior, and automate trading to minimize human error, emphasizing data as the new competitive edge in the financial industry.
Takeaways
- 🔒 Data Science has revolutionized Fraud Prevention in finance by using algorithms to detect unusual activities and protect against identity theft and credit card fraud.
- 🕵️♂️ Anomaly Detection is employed to identify post-facto unusual trading patterns, particularly to catch illegal insider trading, using advanced deep learning models.
- 📊 Customer Analytics allows financial institutions to segment consumers based on behavioral trends and socio-economic characteristics, helping to predict and manage client value and financial strategies.
- 💰 Risk Management in finance has been enhanced by data science through the development of real-time scoring models that assess and mitigate uncertainties and vulnerabilities in investments.
- 🤖 Algorithmic Trading represents a significant leap, with machines making high-speed trades based on algorithms, reducing human error and exploiting market opportunities efficiently.
- 📉 The 2008 financial crisis highlighted the need for better risk models, leading to the adoption of data science to create more reliable credit scoring systems and prevent similar downturns.
- 🚫 The script mentions a downside of algorithmic trading where imprecise algorithms can lead to significant market losses, as seen in the Dow Jones plummet in February 2018.
- 🛑 Human intervention may still be necessary in unprecedented events, such as the drone strike on Saudi Arabia's oil refinery in 2019, to prevent algorithmic trading models from causing market chaos.
- 📈 The scarcity of arbitrage opportunities and the even playing field due to algorithmic trading have led financial institutions to seek a competitive edge through exclusive data access.
- 📊 Data has become a highly valued commodity in the finance industry, with institutions investing heavily to gain insights and construct superior models for market advantage.
- 🌐 The script emphasizes the importance of Machine Learning and Deep Learning as integral parts of Data Science, enabling the construction of self-evolving algorithms for future predictions.
Q & A
How has Data Science transformed the financial industry?
-Data Science has transformed the financial industry by introducing automated algorithms and complex analytical tools that work hand-in-hand to provide insights and predictions, leading to advancements in areas such as fraud prevention, anomaly detection, customer analytics, risk management, and algorithmic trading.
What is the role of Machine Learning (ML) and Deep Learning (DL) in data science?
-Machine Learning and Deep Learning are aspects of data science that use modeling algorithms to find links between data, extract insights, and make future predictions. They allow for the construction of self-evolving algorithms given enough time and information.
How does data science aid in fraud prevention within financial institutions?
-Data science aids in fraud prevention by using methods like random forests to determine if there are sufficient factors to indicate suspicion. It also supports security advancements with biometric recognition and additional authentication layers to lower the chances of identity theft.
What is anomaly detection in finance, and how does deep learning contribute to it?
-Anomaly detection in finance is the process of identifying unusual or suspicious activities, such as illegal insider trading, after they occur. Deep learning, through Recurrent Neural Networks and Long Short-Term Memory models, helps create algorithms that can spot trading histories that are well above the norm.
How does customer analytics in finance benefit from data science?
-Customer analytics benefits from data science by using unsupervised ML techniques to segment consumers into clusters based on socio-economic characteristics. Predictive models are then constructed to determine the expected worth of each client, allowing financial institutions to allocate resources efficiently.
What is the significance of risk management in the financial industry, and how does data science support it?
-Risk management is crucial for ensuring stability and minimizing uncertainty in financial deals. Data science supports this by developing risk analytics, which involves identifying, ranking, and monitoring uncertain interactions to prioritize and address vulnerabilities in investments.
What is algorithmic trading, and how does it impact the financial markets?
-Algorithmic trading is the process where a machine makes trades on the market based on a predefined algorithm. It can execute trades multiple times per second across various markets without human intervention, reducing opportunity costs and human errors. It has led to high-frequency trading and increased market efficiency.
How have trading algorithms evolved to prevent market instability?
-Trading algorithms have become more complex to prevent market instability, incorporating reinforced learning models where mistakes are penalized, and hyperparameters are adjusted based on performance to make better estimations.
What was the impact of the 2018 Dow Jones plummet on trading algorithms?
-The 2018 Dow Jones plummet, caused by trading algorithms misinterpreting a false signal, led to a quick market crash. This incident prompted the development of more sophisticated algorithms to prevent similar market freefalls.
How has data science influenced the value of data in the financial industry?
-Data science has made data the most valuable commodity in the financial industry. Financial institutions are investing heavily in exclusive data rights to construct better models and gain a competitive edge.
What is the role of data in the competition among financial institutions today?
-In today's financial industry, data plays a crucial role in gaining a competitive edge. With more information, institutions can develop superior models, leading to an increased focus on data acquisition and utilization over traditional analyst or quant roles.
Outlines
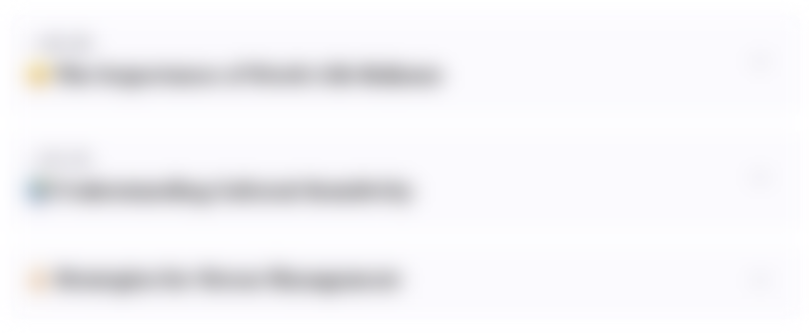
This section is available to paid users only. Please upgrade to access this part.
Upgrade NowMindmap
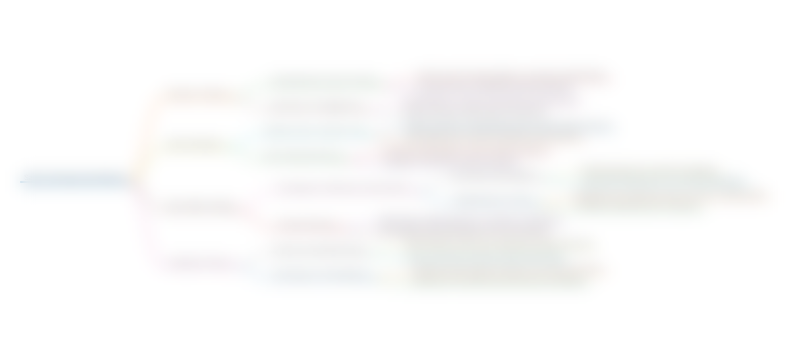
This section is available to paid users only. Please upgrade to access this part.
Upgrade NowKeywords
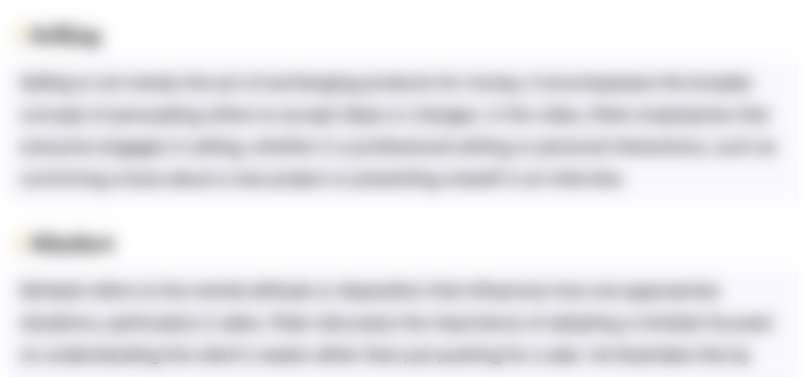
This section is available to paid users only. Please upgrade to access this part.
Upgrade NowHighlights
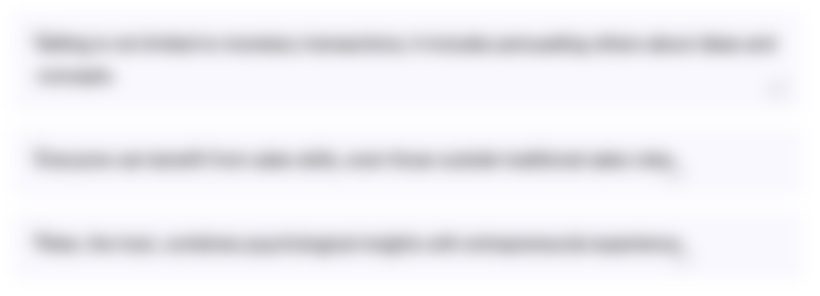
This section is available to paid users only. Please upgrade to access this part.
Upgrade NowTranscripts
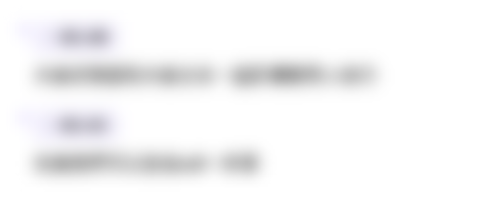
This section is available to paid users only. Please upgrade to access this part.
Upgrade NowBrowse More Related Video
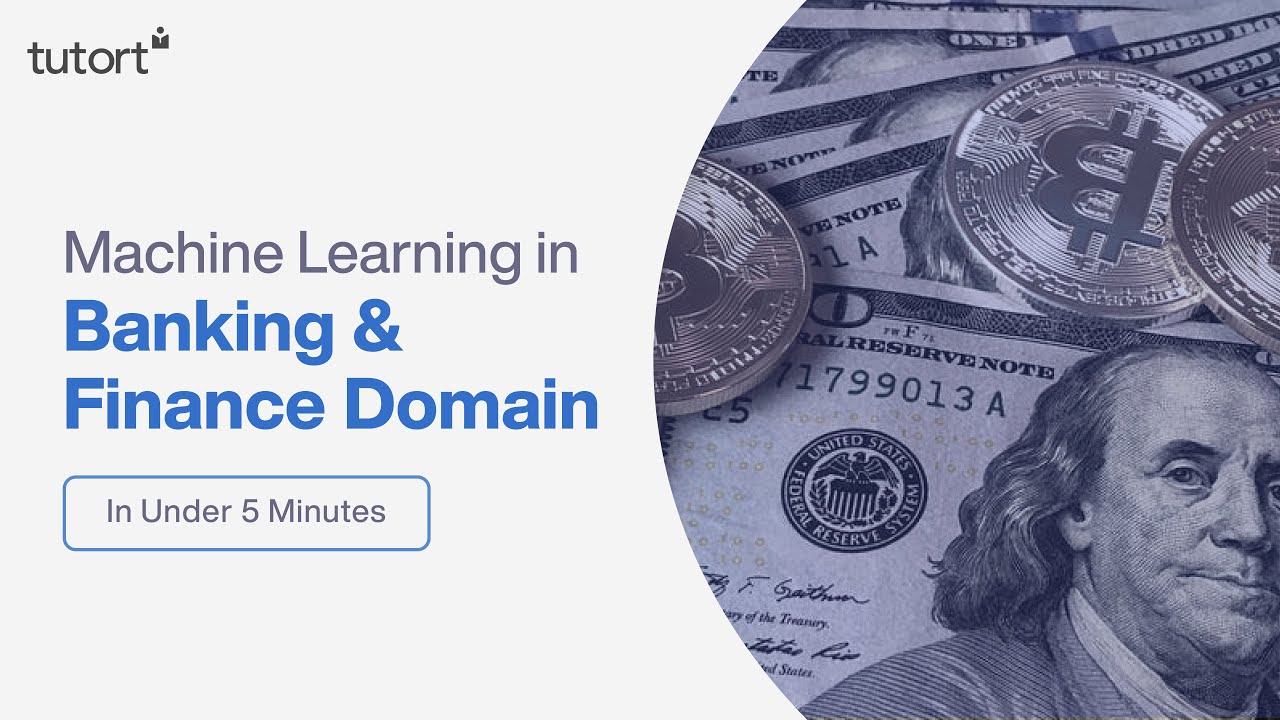
How Banking & Finance Industry use Machine Learning and Artificial Intelligence | Tutort Academy
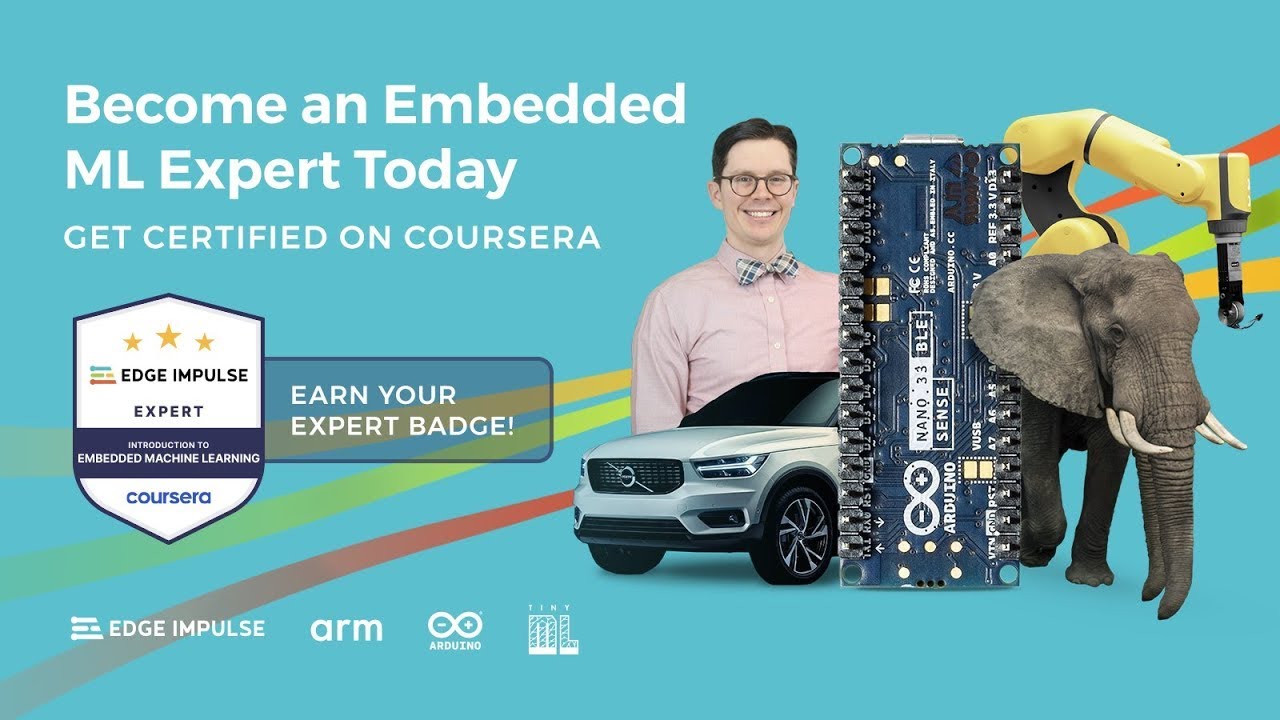
Introduction to Embedded Machine Learning 2.4.1 - Anomaly Detection

#7 Machine Learning Specialization [Course 1, Week 1, Lesson 2]
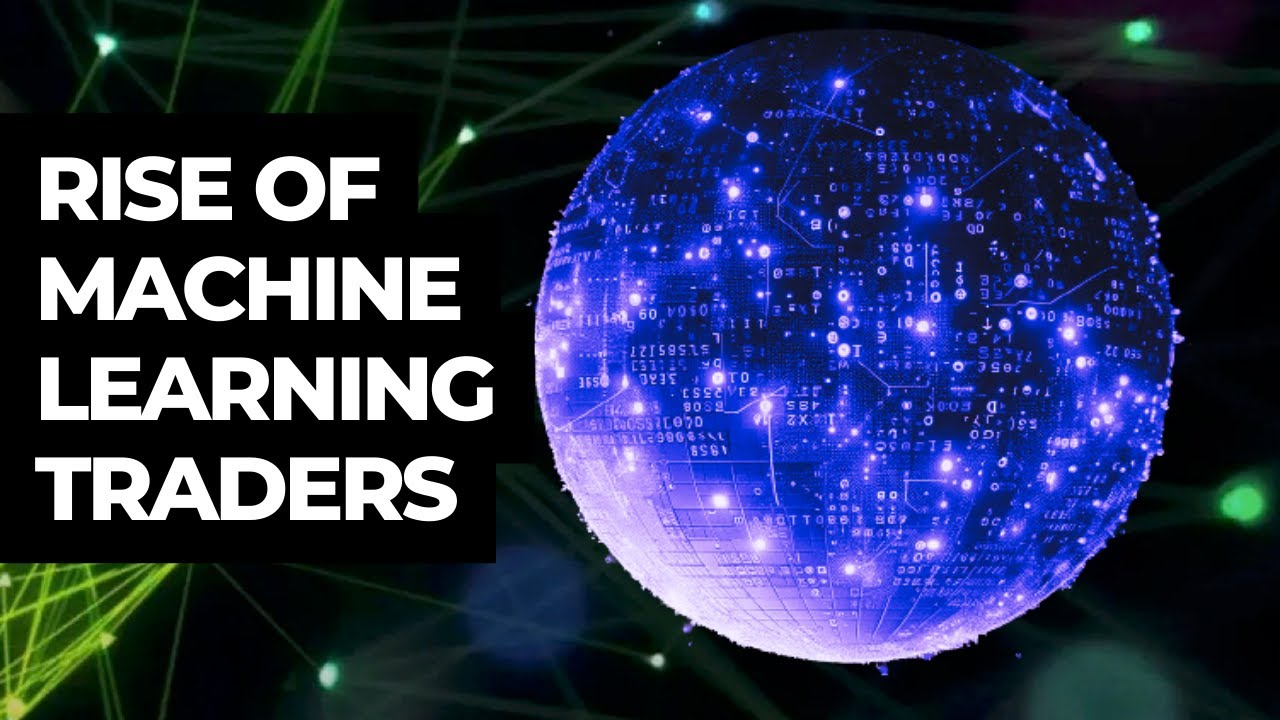
How Machine Learning/AI Traders Beats Retail Traders with Example Strategy for Beginners

Top 17 BEST Data Science & Analytics Certificates (2024)
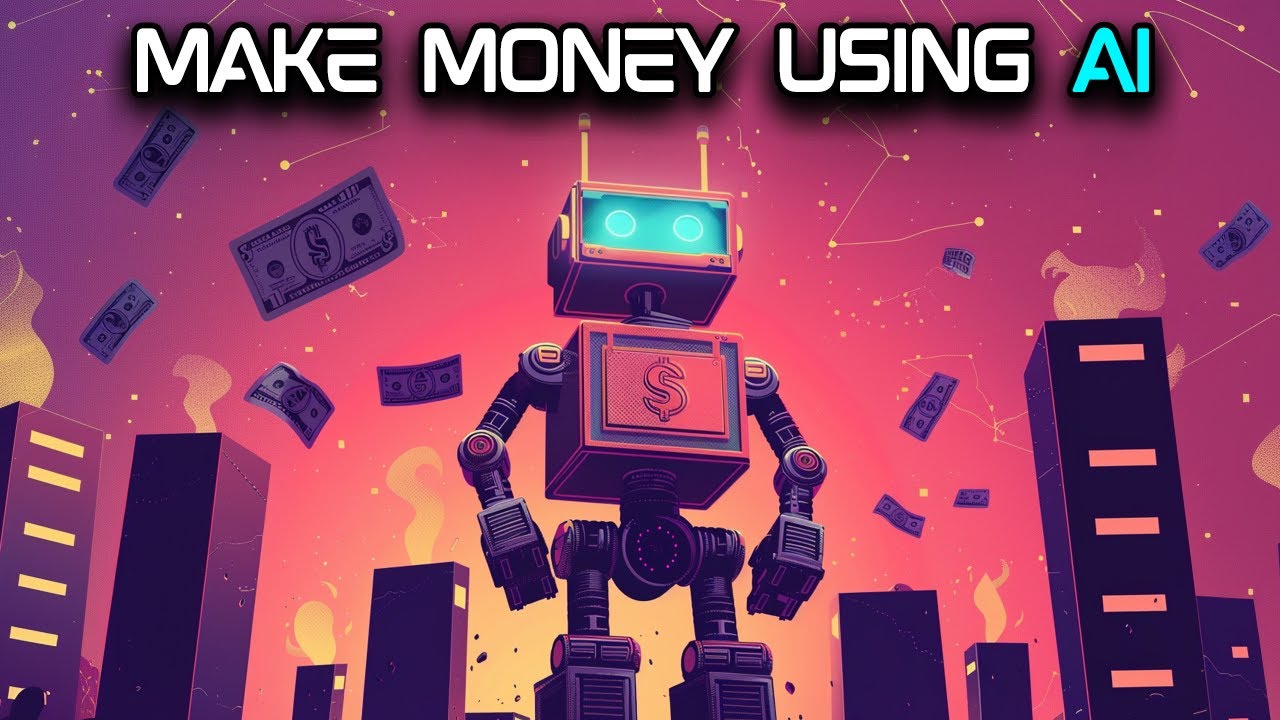
10 Ways People Are Using AI to Get Rich Quickly (in 2024)
5.0 / 5 (0 votes)