T-Tests: A Matched Pair Made in Heaven: Crash Course Statistics #27
Summary
TLDRIn this Crash Course Statistics episode, Adriene Hill explores the concept of test statistics through a coffee tasting experiment. Comparing two local coffee shops, she uses a two-sample t-test to determine if there's a significant difference in scores. Initially, no significant difference is found, but after considering individual preferences, a paired t-test reveals a preference for one shop. The lesson emphasizes the importance of controlling for variability in experiments and the power of statistical tests to reveal patterns amidst noise.
Takeaways
- π The script introduces the concept of test statistics and how they can be generalized for different situations without memorizing a new formula for each.
- π It uses a coffee tasting experiment to illustrate the process of hypothesis testing, comparing two coffee shops, Caf-fiend and The Blend Den.
- π The experiment involves a two-sample t-test, which is also known as an independent or unpaired t-test, to determine if there's a significant difference in the mean scores of coffee ratings.
- π― The null hypothesis is that there is no difference between the two coffee shops, while the alternative hypothesis suggests that one is better than the other.
- π The standard error is used as a measure of average variation in the two-sample t-test, taking into account the sample variance of both groups.
- π The script explains how to calculate the critical t-value and p-value to determine statistical significance, using an alpha level of 0.05.
- π It discusses the limitations of the initial experiment, such as not accounting for individual preferences for coffee, which can introduce variability.
- π The concept of a paired t-test is introduced as a solution to control for individual differences in coffee preference by comparing scores from the same individuals.
- π The paired t-test involves creating difference scores for each person, which allows for the comparison of the two coffees while controlling for individual preferences.
- π The script emphasizes the importance of reducing variability in experiments to better identify true differences and patterns.
- π It concludes by highlighting that statistical tests help in understanding and comparing observed data with expected outcomes, a fundamental principle in statistics.
Q & A
What is the main topic discussed in this Crash Course Statistics episode?
-The main topic discussed in this episode is the application of the general formula for creating test statistics in various situations, with a focus on how to use statistical tests to determine if there is a significant difference between two groups, exemplified by comparing coffee scores from two shops.
Why is it important not to have to memorize a new formula for every statistical test?
-It's important not to memorize a new formula for every statistical test because it would be inefficient and overwhelming. The general formula for test statistics allows for adaptability across different situations, making the process of statistical analysis more streamlined and manageable.
What is the null hypothesis in the coffee tasting experiment described in the script?
-The null hypothesis in the coffee tasting experiment is that there is no difference between the two coffee shops, meaning the mean scores for coffee from Caf-fiend and The Blend Den are the same.
What statistical test is used to analyze the difference in coffee scores between Caf-fiend and The Blend Den?
-A two-sample t-test, also known as an independent or unpaired t-test, is used to analyze the difference in coffee scores between Caf-fiend and The Blend Den.
How is the standard error calculated for a two-sample t-test?
-The standard error for a two-sample t-test is calculated by taking into account the sample variances of the two groups and their sizes (n1 and n2). It involves squaring the standard deviation to get the variance and then using the formula specific for two-sample t-tests.
What are the two methods mentioned to determine if there is a statistically significant difference between the two coffee shops?
-The two methods mentioned are calculating the critical t-value and comparing it with the t-statistic, and calculating the p-value from the t-statistic and comparing it with the chosen alpha level.
Why is an alpha level of 0.05 commonly used in statistical tests?
-An alpha level of 0.05 is commonly used because it means that in the long run, only 5% of tests done on groups with no real difference will incorrectly reject the null hypothesis, providing a balance between avoiding false positives and detecting true effects.
What is the significance of the p-value in the context of this coffee experiment?
-The p-value in the context of the coffee experiment represents the probability of observing a difference in sample means as extreme as the one observed, assuming the null hypothesis is true. A p-value greater than the alpha level indicates a failure to reject the null hypothesis, suggesting no significant difference between the coffee shops.
Why is a paired t-test considered a better approach for the coffee experiment after considering individual preferences?
-A paired t-test is considered better because it accounts for individual preferences by comparing the scores of the same individuals for both coffees, thus controlling for variability due to personal coffee affinity and making it easier to detect true differences between the coffees.
What does the t-statistic of -3.212 in the paired t-test indicate about the difference in coffee scores?
-The t-statistic of -3.212 in the paired t-test indicates that the observed mean difference in scores is 3.212 standard errors away from the expected mean difference under the null hypothesis, suggesting a statistically significant difference between the coffees from Caf-fiend and The Blend Den.
How does the script illustrate the concept that 'absence of evidence is not evidence of absence'?
-The script illustrates the concept by showing that even though the initial experiment did not find a significant difference between the coffee shops, it doesn't mean there is no difference. It could be due to variability in the sample or other factors not accounted for, hence the need for a more controlled experiment like the paired t-test.
Outlines
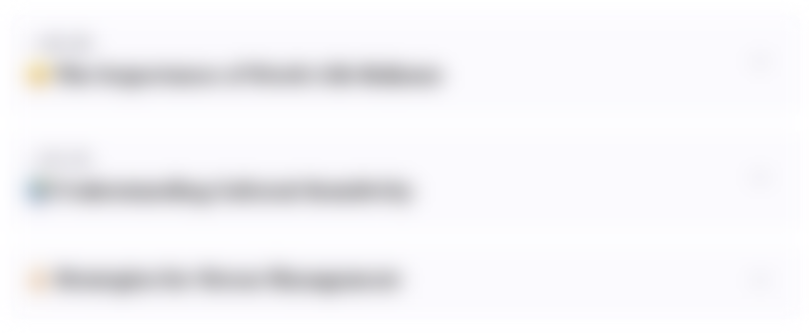
This section is available to paid users only. Please upgrade to access this part.
Upgrade NowMindmap
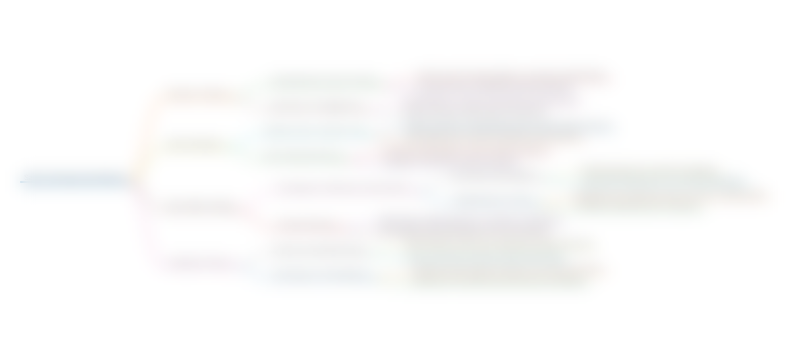
This section is available to paid users only. Please upgrade to access this part.
Upgrade NowKeywords
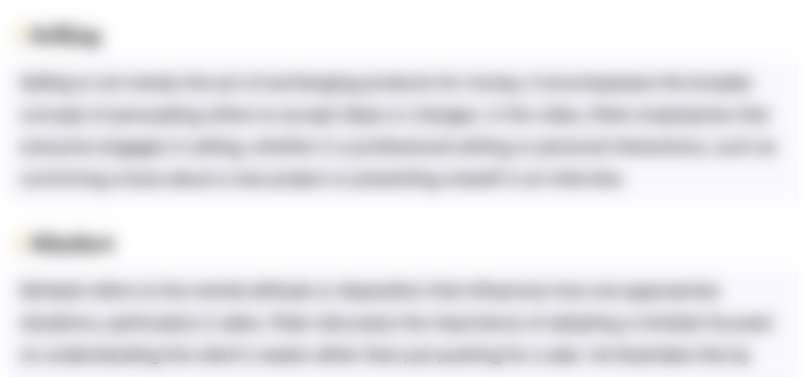
This section is available to paid users only. Please upgrade to access this part.
Upgrade NowHighlights
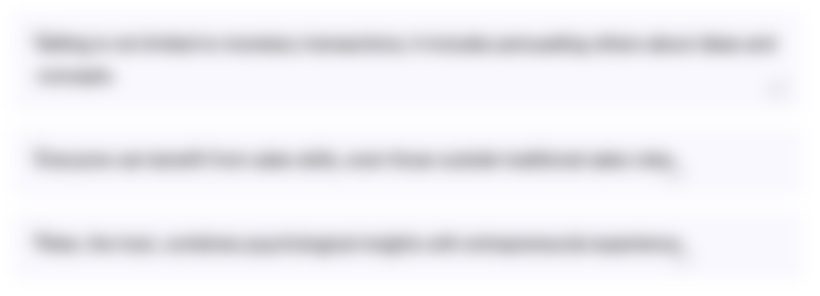
This section is available to paid users only. Please upgrade to access this part.
Upgrade NowTranscripts
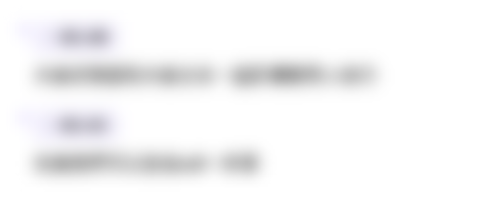
This section is available to paid users only. Please upgrade to access this part.
Upgrade NowBrowse More Related Video

Test Statistics: Crash Course Statistics #26

STATISTIKA - Uji T Sampel Bebas (Independent Samples T Test) Perhitungan Manual
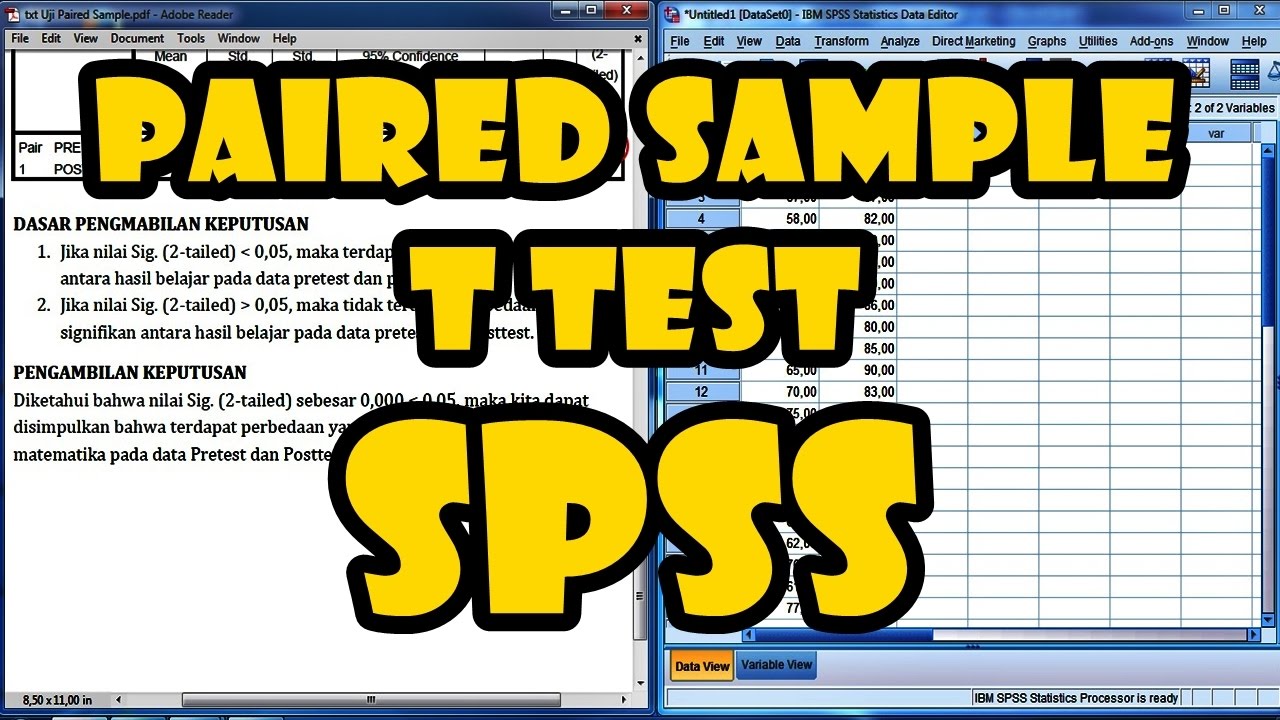
Cara Uji Paired Sample T Test dengan SPSS Full
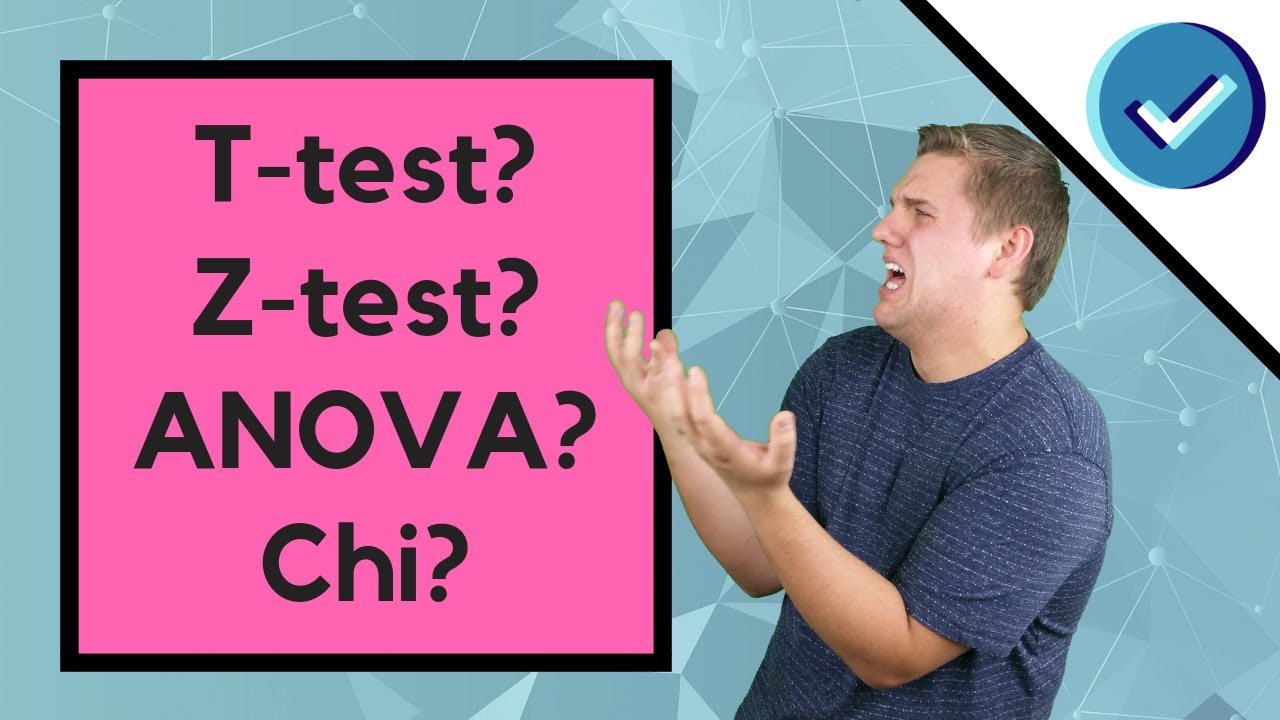
How To Know Which Statistical Test To Use For Hypothesis Testing

Two-sample t test for difference of means | AP Statistics | Khan Academy
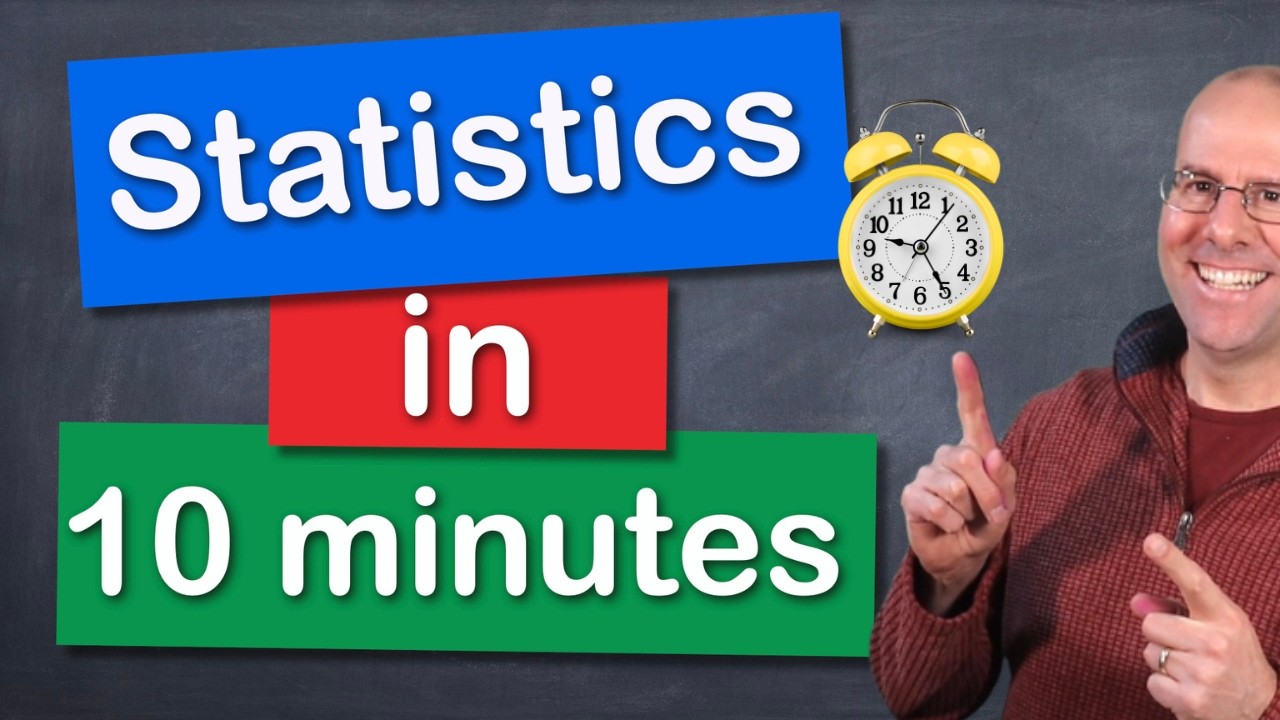
Statistics in 10 minutes. Hypothesis testing, the p value, t-test, chi squared, ANOVA and more
5.0 / 5 (0 votes)