Lidar Accuracy Comparison - 13 Different Sensors!
Summary
TLDR本视频对比了16种不同激光雷达传感器数据集,这些数据集来自现实捕捉行业的领先制造商,旨在找出最佳传感器。测试地点位于佛罗里达州中部的一个约8英亩的公园,通过独立检查拍摄来比较每个数据集的水平和垂直精度,以及植被穿透能力和数据密度。结果显示,地面扫描提供最准确的结果,而数据处理和采集方式的重要性超过了传感器本身的价格。
Takeaways
- 🏆 对比了16种不同的激光雷达传感器数据集,旨在找出现实捕捉行业中的佼佼者。
- 📈 研究使用了中佛罗里达一个约8英亩的公园作为测试场地,具有周边道路和内部绿化区域。
- 📊 通过独立的检查射击,比较了每个数据集的水平和垂直精度。
- 🌿 评估了不同数据集在植被穿透和数据密度方面的表现。
- 🔍 使用高精度全站仪进行了12个特征的检查射击,以提取点云中的特征。
- 📝 通过Excel电子表格比较了检查射击的坐标,以确定水平误差。
- 📊 对于垂直误差,通过将点云栅格化并比较平均高程与检查射击的数据来测量。
- ⚙️ 发现了一些数据集的问题,例如Leica RTC360数据集的坐标系统与控制文件不匹配。
- 🌳 地面扫描仪提供了最密集的数据集,而移动扫描仪和无人机(UAV)扫描仪在水平坐标的精度上表现较差。
- 🖼️ 摄影测量数据集在遮挡物下无法提供准确的点,而地面数据集提供了最准确的结果。
- 💡 实验表明,数据的捕获和处理方式比传感器本身的花费更重要。
- 👨💼 经验和正确的现场及办公室程序比昂贵的传感器更为关键,对于获取期望的结果至关重要。
Q & A
视频比较了多少个不同的激光雷达传感器数据集?
-视频比较了16个不同的激光雷达传感器数据集。
这些数据集是关于哪个地区的扫描?
-这些数据集是关于佛罗里达州中部一个约8英亩大小的公园的扫描。
控制点是如何设置的?
-控制点是使用指南针全站仪进行射击的,通过闭合导线调整,高程通过闭合水准环进行校正。
视频作者如何验证点云数据的水平和垂直精度?
-视频作者通过使用高精度全站仪设置并射击12个从点云中提取的特征点,然后将这些检查点带入云比较软件中,与提供的点云进行比较,以确定水平和垂直精度。
在比较中,哪个传感器类型提供了最密集的数据集?
-地面扫描仪提供了最密集的数据集。
在数据集的RGB值方面,有哪些问题需要注意?
-地面扫描仪和无人机扫描在相机看不到的区域没有提供准确的RGB值。移动扫描仪在路边缘后迅速完成扫描,而无人机扫描则错误地将穿透树冠的点着色为绿色。
在比较中,哪个传感器类型在水平精度方面表现最好?
-地面扫描仪在水平精度方面表现最好,平均误差为13毫米。
视频作者在处理数据时遇到了哪些问题?
-视频作者在处理数据时遇到了一些问题,例如Leica RTC360数据集的坐标系统与控制文件不匹配,Asai传感器的数据集没有很好地捕捉到周围环境,而Navis VLX3数据集的水平误差异常高,可能是由于操作员的错误。
视频作者得出的最重要的结论是什么?
-视频作者得出的最重要的结论是,数据的捕获和处理方式比购买的传感器的价格更重要。即使拥有顶级的传感器,如果没有正确的训练和经验,也可能无法获得预期的结果。
视频作者建议在进行激光雷达扫描时应该重视什么?
-视频作者建议在进行激光雷达扫描时应该重视现场和办公室的程序,这比在传感器上的投资更为重要。
为什么视频作者没有公布每个传感器的精确度数值?
-视频作者没有公布每个传感器的精确度数值,因为数据捕获方式存在很大变化,如果由不同的工作人员进行数据捕获和处理,很可能会得到不同的结果。
Outlines
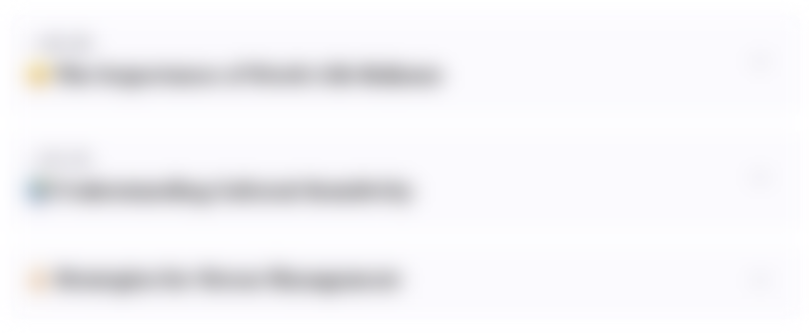
This section is available to paid users only. Please upgrade to access this part.
Upgrade NowMindmap
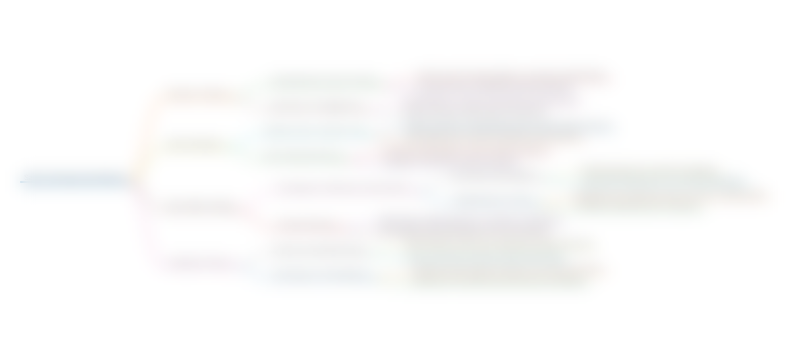
This section is available to paid users only. Please upgrade to access this part.
Upgrade NowKeywords
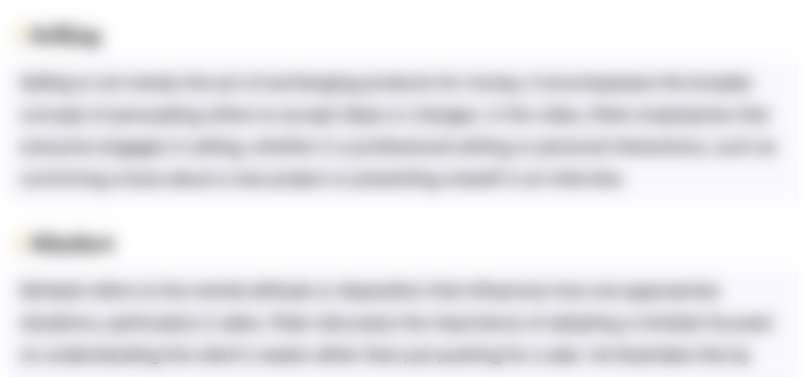
This section is available to paid users only. Please upgrade to access this part.
Upgrade NowHighlights
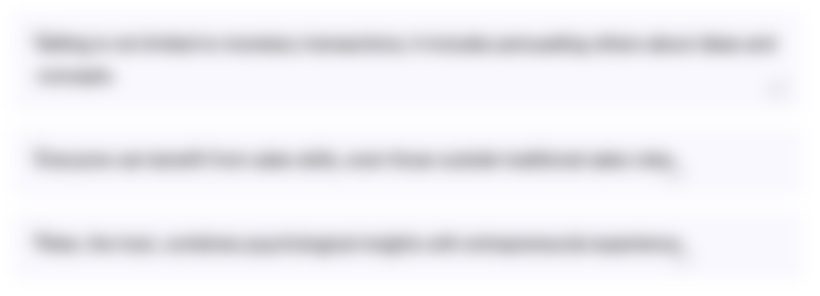
This section is available to paid users only. Please upgrade to access this part.
Upgrade NowTranscripts
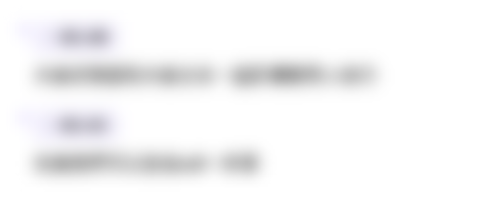
This section is available to paid users only. Please upgrade to access this part.
Upgrade NowBrowse More Related Video

NASA | TIRS: The Thermal InfraRed Sensor on LDCM

Crop Water Use Dynamics & Water Budget Parameters/High Plains Aquifer - Gabriel Senay, USGS EROS

[CVPR 2024 Highlight] 4D-DRESS: A 4D Dataset ofReal-World Human Clothing With Semantic Annotations

[ML2021] Pytorch Tutorial 2

Field-scale Actual ET Estimation using SSEBop | Gabriel Senay, Ph.D., P.E.
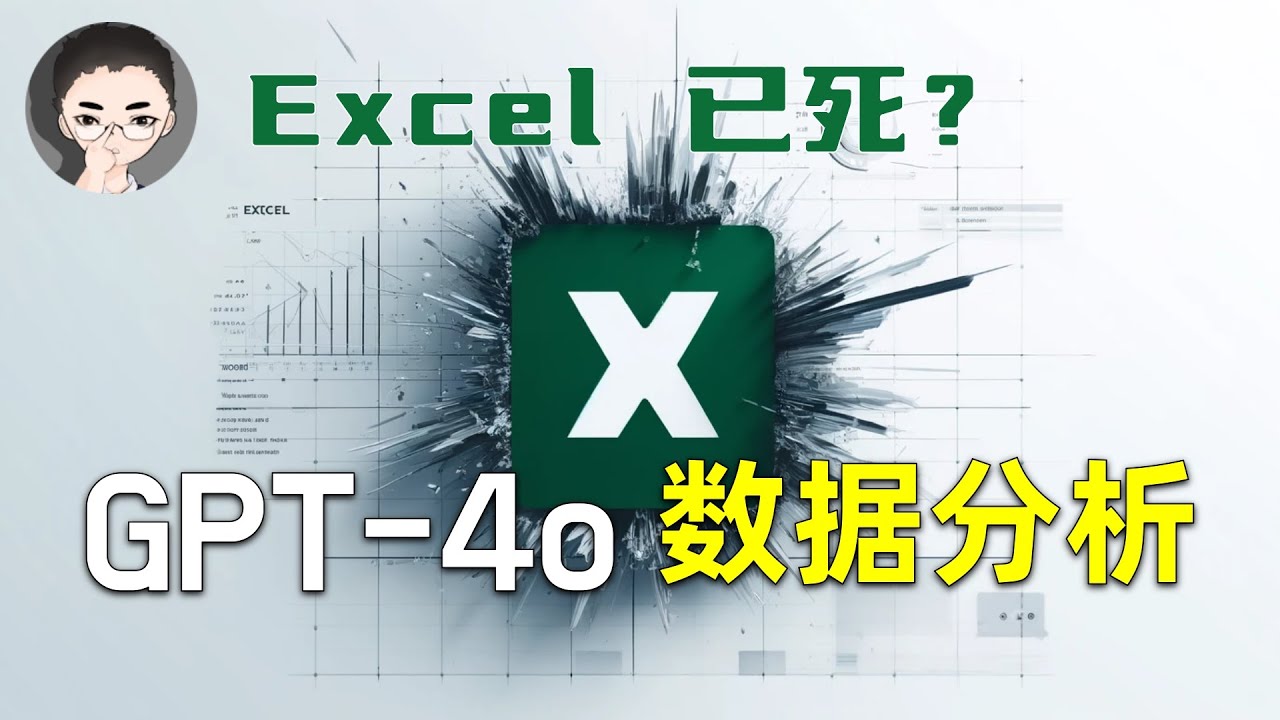
Excel 已死? 有了 GPT-4o 从此不愁 Excel 公式和数据透视表!免费、强悍的高级数据分析 | 回到Axton
5.0 / 5 (0 votes)