Geoffrey Hinton Unpacks The Forward-Forward Algorithm
Summary
TLDR在这段视频脚本中,Craig Smith采访了深度学习领域的先驱Jeffrey Hinton,探讨了他提出的前馈-前馈(forward-forward)算法。Hinton教授对大脑如何处理信息充满好奇,尤其是他所提出的算法,旨在模拟大脑皮层的学习方式。该算法分为在线(清醒时)和离线(睡眠时)两个阶段,在线阶段网络通过高活动性来识别真实数据,而离线阶段则生成自身的数据并尝试低活动性。Hinton认为,这种方法比传统的反向传播算法更符合大脑的工作机制,因为它不需要完美的前向系统模型,也不需要在时间上反向传播。此外,他还讨论了如何生成负数据,以及如何利用这种算法进行有效学习。整个讨论技术性很强,但即便对于那些不熟悉技术细节的听众,Hinton教授的洞察力也提供了宝贵的视角。
Takeaways
- 🤖 神经网络和深度学习先驱杰弗里·辛顿(Geoffrey Hinton)提出了一种新的学习算法——前馈前馈(forward-forward)算法,他认为这可能是大脑皮层学习的一种更合理的模型。
- 🧠 辛顿认为传统的反向传播(back propagation)算法并不能完全解释大脑是如何处理信息的,因为大脑中并没有证据表明信息是以反向传播的方式进行处理的。
- 🔁 前馈前馈算法包含在线(在线相位对应于清醒状态)和离线(离线相位对应于睡眠状态)两个阶段,分别用于处理真实数据和生成数据。
- 🌐 在线阶段,网络尝试让每一层对真实数据有高活动性,而在离线阶段,网络生成自己的数据并尝试让每一层的活动性降低。
- 📉 该算法的一个关键特点是它不需要对前向系统有一个完美的模型,这与传统的反向传播算法不同,后者需要精确的前向系统模型来进行权重更新。
- 🚀 辛顿讨论了将静态图像转换为“视频”,以便在时间维度上实现自上而下的效应,这有助于处理动态视觉信息。
- 👶 辛顿提到婴儿在很短的时间内就能学习到结构从运动中获得的3D感知,这表明大脑能够快速学习到3D结构与运动之间的关系。
- 🔧 辛顿在Matlab上进行了大量的实验,以测试和证明前馈前馈算法的有效性,尽管Matlab并不是处理大型系统的最有效工具。
- 📈 辛顿认为,如果我们能够理解大脑的工作原理并复制它,那么创建具有推理能力的模型是有可能的,但他对于意识(consciousness)的本质持谨慎态度。
- 🤔 辛顿强调,我们对意识的理解可能类似于100年前的“生命力”概念,即试图用简单的精华来解释复杂机制,而这种理解可能并不准确。
- 💡 辛顿提到,他的知识很大程度上来自于与人交谈,而不是阅读,他认为与人交谈是获取知识的高效方式。
Q & A
什么是深度学习中的前馈前向(forward-forward)算法?
-前馈前向算法是杰弗里·辛顿提出的一种新的学习算法,它被认为比反向传播(backpropagation)算法更可能是大脑皮层学习信息的一个合理模型。该算法将学习过程分为在线(清醒时)和离线(睡眠时)两个阶段,在线阶段网络通过输入真实数据并尝试让每一层都保持高活动水平来区分真实数据和假数据;离线阶段网络生成自己的数据,并尝试让每一层的活动水平降低,以此来学习生成模型。
为什么杰弗里·辛顿认为反向传播算法不是大脑处理信息的方式?
-辛顿认为反向传播算法需要一个完美的前向系统模型,并且在大脑中没有证据表明信息会以反向传播所需的方式反向流动。此外,反向传播在递归网络中的实现会遇到时间上的挑战,例如在处理视频时无法简单地停止并反向传递。
在前馈前向算法中,如何生成负数据(negative data)?
-在前馈前向算法中,负数据是在网络运行在负阶段时输入的数据,目的是在所有隐藏层中获得低活动水平。负数据可以由模型自身生成,也可以通过手动输入不正确的标签或图像来提供。最终目标是让模型自己生成负数据,当模型足够好时,负数据看起来就像真实数据一样,此时学习过程结束。
前馈前向算法中的“在线阶段”和“离线阶段”是如何工作的?
-在线阶段,网络接收输入并处理真实数据,目标是让每一层的活动水平足够高,以便于区分真实数据和假数据。而在离线阶段,网络尝试生成自己的数据,并希望在给定自己生成的数据作为输入时,每一层的活动水平降低。这样可以训练网络学习一个生成模型,并区分真实数据和假数据。
为什么杰弗里·辛顿认为前馈前向算法可能更适合低功耗计算机架构?
-前馈前向算法不需要对前向系统有一个完美的模型,它只需要对单个神经元的行为有一个足够好的模型,以便能够改变进入该神经元的权重,使其更活跃或不那么活跃。这种算法可以在包含未知黑盒的系统中学习,这使得它在低功耗硬件上更具潜力。
杰弗里·辛顿是如何开发和测试他的新学习算法的?
-辛顿通过在Matlab中编写和测试小规模的模型来开发和测试他的新学习算法。他会花费时间思考概念,并在Matlab中实现这些想法,以检验它们是否有效。对于大多数原始想法,他发现它们是错误的,而Matlab的便利性在于能够快速证明这些想法的错误。
为什么杰弗里·辛顿认为意识不是一个简单的概念?
-辛顿认为意识是一个极其复杂的概念,人们常常将其混为一谈,试图用简单的“本质”来解释复杂的机制。他认为,意识的讨论类似于过去的“生命力”概念,后者是一个无法具体定义的概念,用来模糊地解释生物为何是活的。辛顿强调,意识的感知和体验实际上是一种关于假设性状态的陈述,而不是某种特殊的内部本质。
在前馈前向算法中,网络如何处理静态图像和视频数据?
-在处理静态图像时,前馈前向算法可以将其视为视频数据的一个特例,其中视频帧没有随时间变化。而在处理视频数据时,网络会在时间维度上进行前向传递,允许顶层的预测影响底层的表示,从而实现对动态场景的理解。
杰弗里·辛顿是如何与他人交流和学习新知识的?
-辛顿通过与不同领域的专家交谈来交流和学习新知识。他与认知科学家、神经科学家和心理学家等人交流,从他们那里获取信息。由于阅读速度较慢,且在阅读方程时会受到阻碍,他认为与人交谈是获取知识的有效方式。
为什么杰弗里·辛顿认为在当前阶段,将前馈前向算法扩展到大型系统还为时过早?
-辛顿认为,由于前馈前向算法在实现时需要一些特定的技巧,而这些技巧比反向传播算法更为复杂,因此在基本算法的基本原理和最佳实践方法得到充分理解和验证之前,将其扩展到大型系统是不成熟的。
杰弗里·辛顿是否认为前馈前向算法在未来有可能超越基于反向传播的模型?
-辛顿并不确定前馈前向算法是否会超越基于反向传播的模型。他认为反向传播在给定数量的连接中可以压缩更多的知识,而前馈前向算法则在处理大量连接时可能不那么有效。但他也指出,大脑的主要问题不是知识压缩,而是如何有效地利用经验来获取信息。
在前馈前向算法中,网络是如何区分真实数据和假数据的?
-网络通过每一层的活动水平来区分真实数据和假数据。在线阶段,网络处理真实数据时,每一层都试图保持高活动水平;而在离线阶段,网络生成自己的数据(假数据)时,每一层都试图降低活动水平。通过这种方式,网络可以学会区分真实数据和假数据。
Outlines
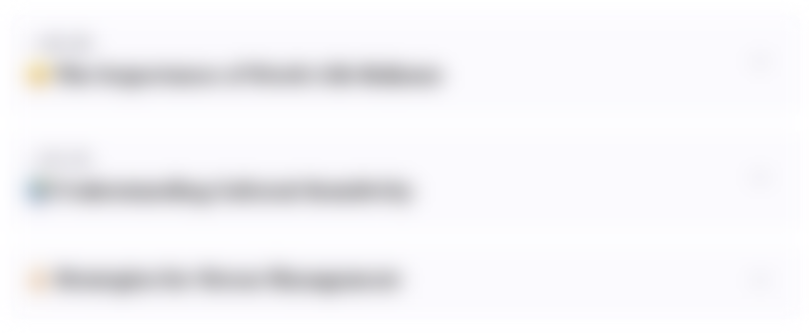
This section is available to paid users only. Please upgrade to access this part.
Upgrade NowMindmap
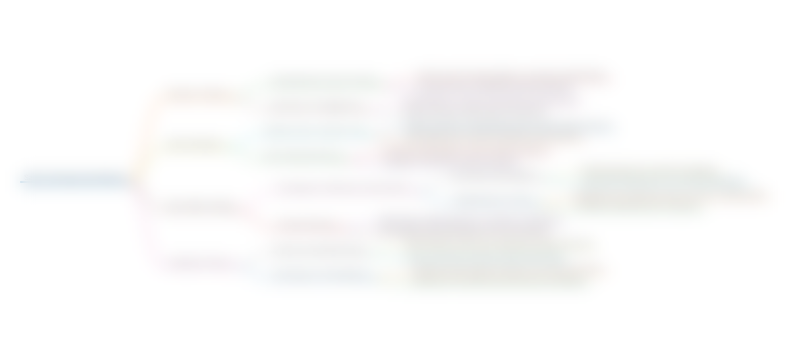
This section is available to paid users only. Please upgrade to access this part.
Upgrade NowKeywords
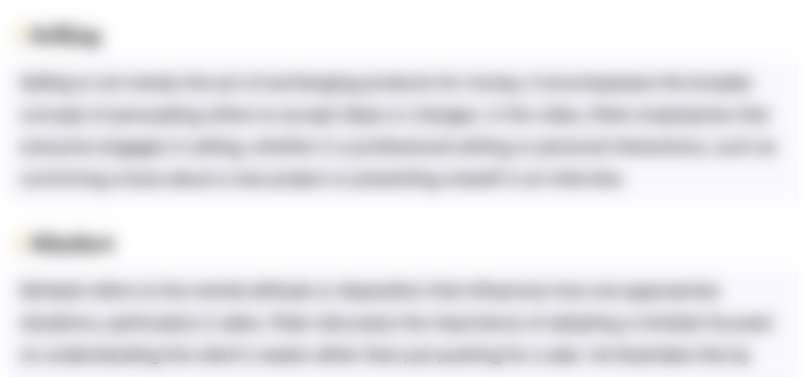
This section is available to paid users only. Please upgrade to access this part.
Upgrade NowHighlights
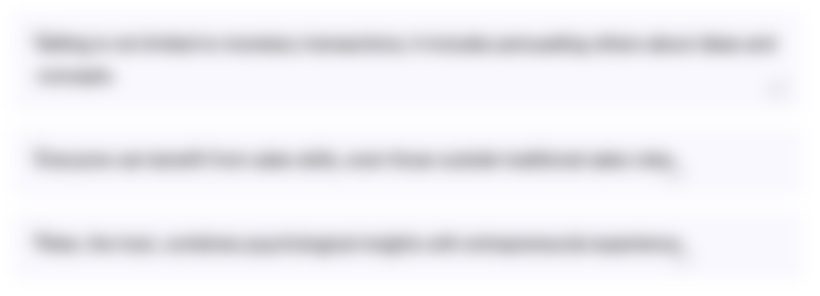
This section is available to paid users only. Please upgrade to access this part.
Upgrade NowTranscripts
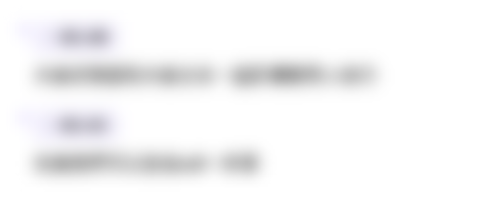
This section is available to paid users only. Please upgrade to access this part.
Upgrade NowBrowse More Related Video

Heroes of Deep Learning: Andrew Ng interviews Geoffrey Hinton
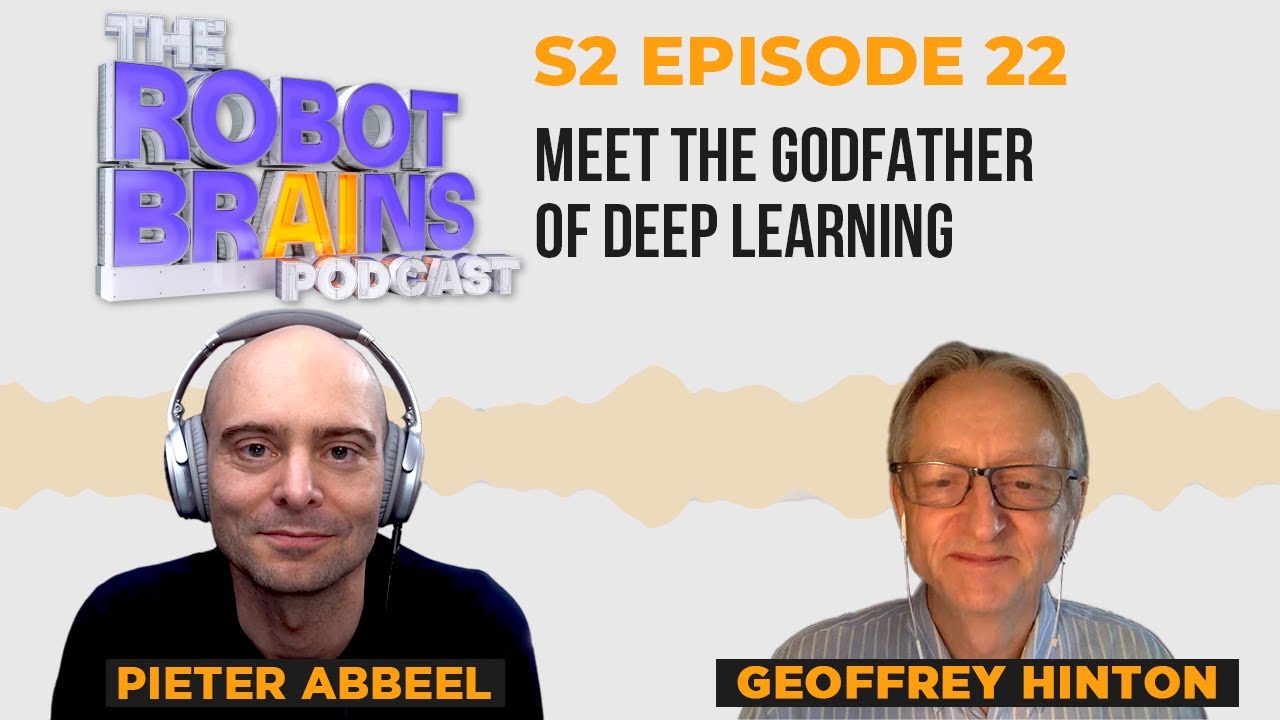
Season 2 Ep 22 Geoff Hinton on revolutionizing artificial intelligence... again
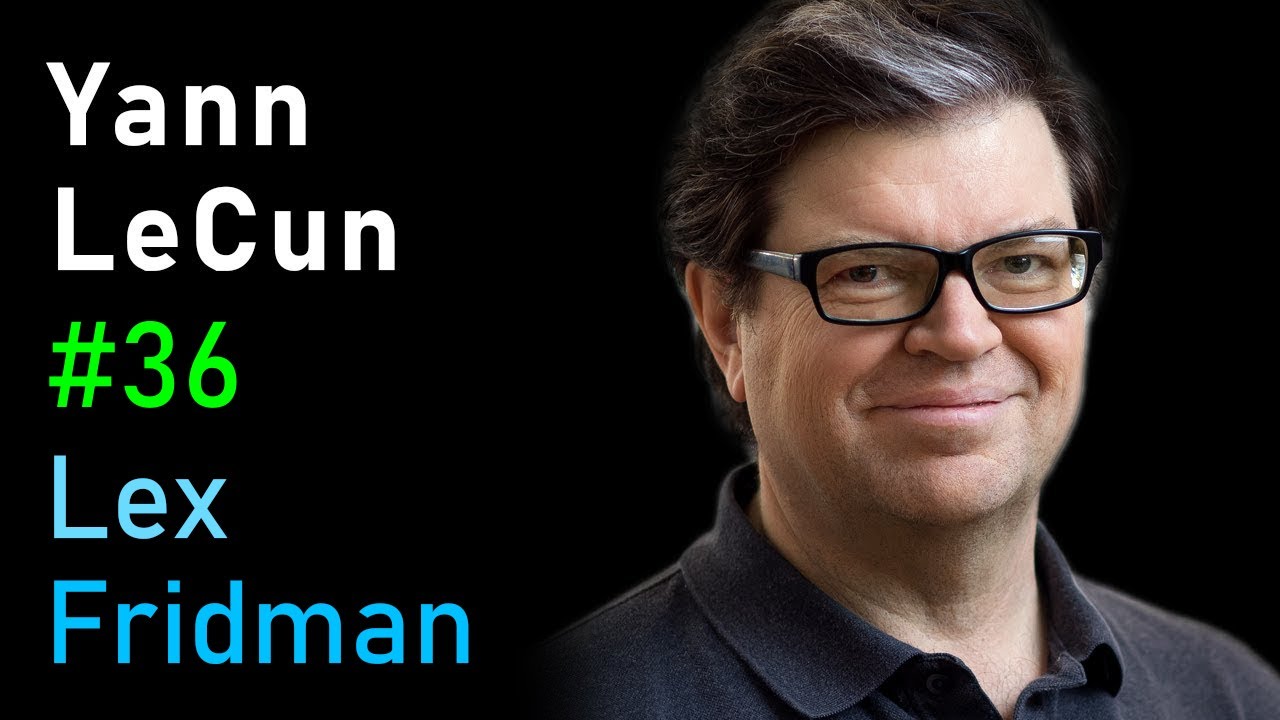
Yann LeCun: Deep Learning, ConvNets, and Self-Supervised Learning | Lex Fridman Podcast #36
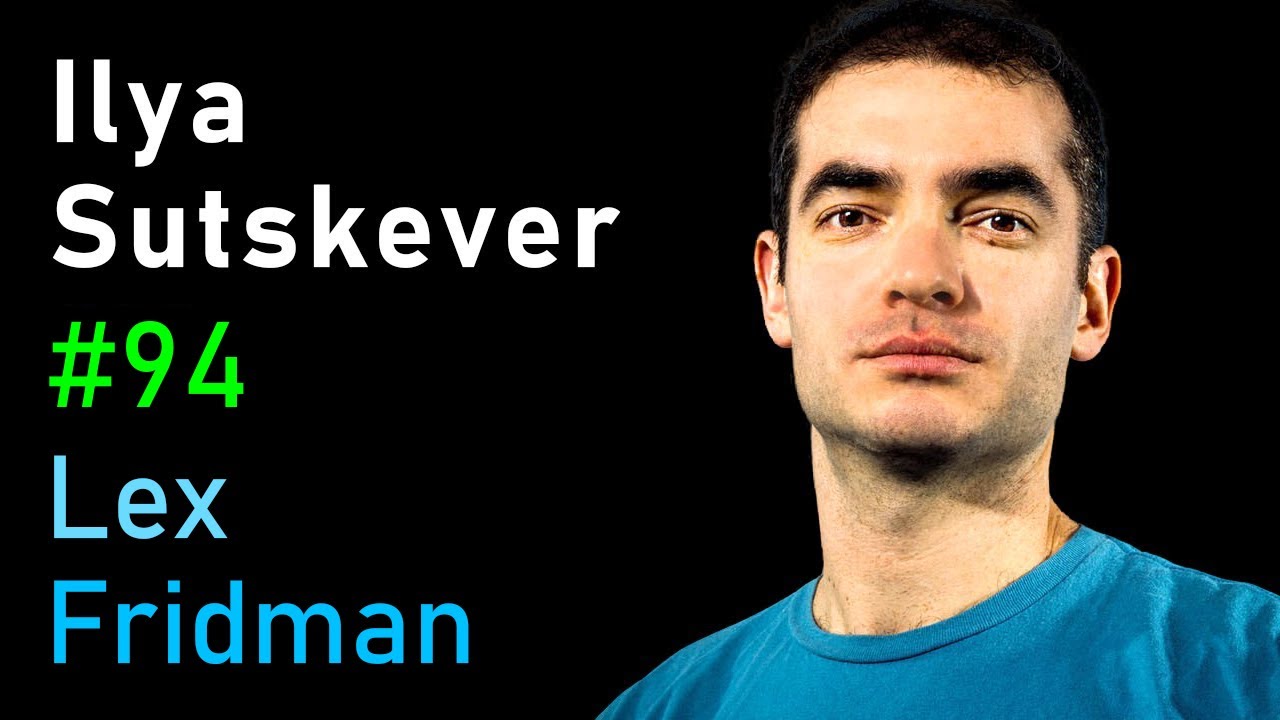
Ilya Sutskever: Deep Learning | Lex Fridman Podcast #94

Ilya sutskever | Humanity will eventually move towards AGI | The intelligent body will soon appear

The Most Important Algorithm in Machine Learning
5.0 / 5 (0 votes)