SEM Series (2016) 2. Data Screening
Summary
TLDRThis video script is a comprehensive guide to data screening in statistical analysis. It covers essential steps like identifying missing data, handling unengaged responses, managing outliers, and checking for skewness and kurtosis. The tutorial uses a practical approach with examples from a dataset, demonstrating how to clean and prepare data for analysis in SPSS. It also discusses the implications of each step and provides tips for reporting the findings in research.
Takeaways
- 🔍 The video script discusses a systematic approach to data screening, focusing on handling missing data, identifying unengaged responses, and managing outliers in continuous variables.
- 🗂️ The process starts with organizing the dataset by removing unnecessary variables and keeping track of IDs to ensure data integrity.
- 📊 To detect missing data, the script suggests using Excel to count blank cells and sort the data to identify rows with a high number of missing values, which may be candidates for removal.
- 🚫 The script identifies unengaged responses by looking for patterns such as identical answers across all questions or extremely short survey completion times, indicating a lack of attention from respondents.
- 📈 For continuous variables, the script recommends checking for outliers using scatter plots and replacing extreme values with the mean or median to maintain data integrity.
- 📉 The video also covers the importance of dealing with skewness and kurtosis, suggesting the use of conditional formatting in Excel to highlight values that exceed certain thresholds, indicating potential issues with data distribution.
- 📝 It's advised to report the data screening process in a research paper, including details about missing data imputation, removal of unengaged responses, and handling of outliers.
- ❌ The script emphasizes the need to be cautious when dealing with a high percentage of missing data in a variable, as it can dilute the potency of the variable and affect analysis outcomes.
- 🔢 The process of imputing missing values differs for ordinal and continuous scales, with medians used for ordinal scales and means for continuous scales, to maintain the integrity of the data.
- ⏱️ The video mentions the use of attention traps and reverse-coded items as strategies to identify unengaged respondents, which can help in cleaning the dataset.
Q & A
What is the first step in data screening as described in the script?
-The first step in data screening is to check for missing data in the rows of the dataset.
How does the script suggest handling unengaged responses in a survey?
-Unengaged responses can be detected by visually inspecting data, using attention traps, recording time elapsed for survey completion, employing reverse-coded items, or identifying respondents who give the same response to every question.
What is an 'attention trap' in the context of survey data?
-An 'attention trap' is a question in a survey designed to identify respondents who are not paying attention, such as asking them to select a specific, counterintuitive answer to a straightforward question.
How can outliers on continuous variables be identified and addressed according to the script?
-Outliers on continuous variables can be identified using scatter plots or by calculating the standard deviation of the values. Addressing them may involve removing the outlier if it's due to an erroneous response or imputing a value like the mean or median for the variable.
What is the purpose of using reverse-coded items in a survey?
-Reverse-coded items are used to detect unengaged respondents. They are negatively worded questions where the expected answer should be the opposite of what is typically agreed upon, helping to identify respondents who may not be paying attention.
Why is it important to check for missing data before conducting further analysis?
-Checking for missing data is important because it can affect the validity and reliability of the analysis. Missing data can lead to biased results or require imputation, which should be reported in the study.
What is the recommended approach for handling missing data in ordinal scales versus continuous scales?
-For ordinal scales, the median is recommended for imputation, while for continuous scales, the mean is more appropriate. This distinction is made because ordinal scales do not have actual mean values between the scale points.
How does the script suggest detecting respondents who may not be engaged in the survey?
-The script suggests detecting unengaged respondents by looking for those who provide the same response to every question (liners), those who complete the survey in an unrealistically short amount of time, or those who do not correctly answer attention trap questions.
What is the significance of checking for skewness and kurtosis in the data?
-Checking for skewness and kurtosis is important to assess the normality of the data distribution. Extreme values can indicate that the data may not meet the assumptions required for certain statistical tests, potentially affecting the analysis and its interpretation.
How should the presence of outliers be reported in a research paper according to the script?
-The presence of outliers should be reported by noting the specific variables affected, the nature of the outliers (e.g., extremely high or low values), and the actions taken to address them, such as removal or correction of the values.
Outlines
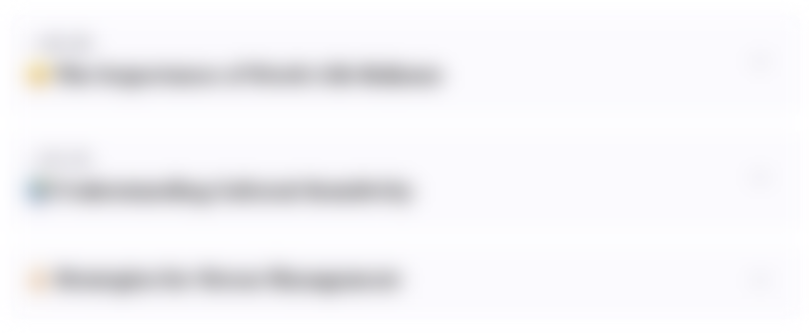
This section is available to paid users only. Please upgrade to access this part.
Upgrade NowMindmap
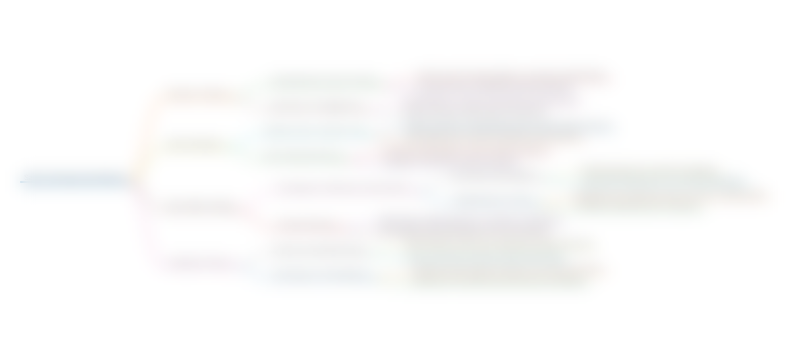
This section is available to paid users only. Please upgrade to access this part.
Upgrade NowKeywords
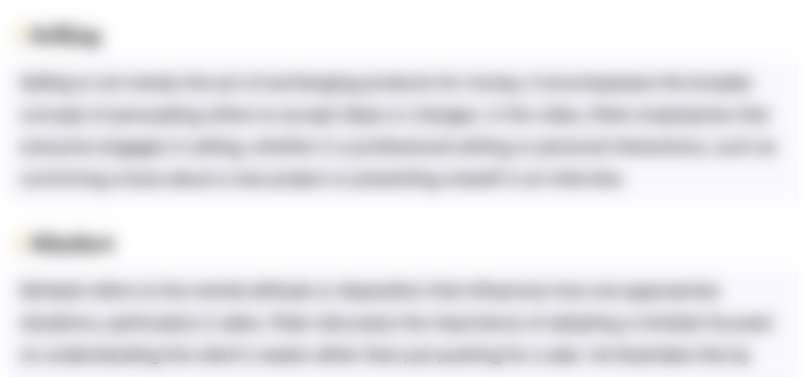
This section is available to paid users only. Please upgrade to access this part.
Upgrade NowHighlights
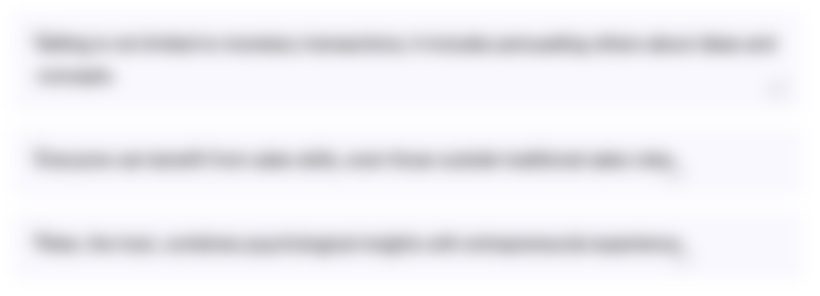
This section is available to paid users only. Please upgrade to access this part.
Upgrade NowTranscripts
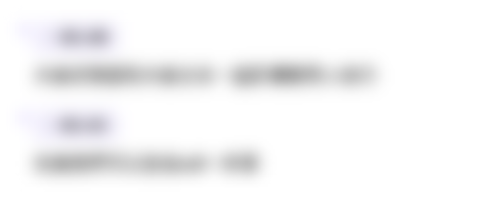
This section is available to paid users only. Please upgrade to access this part.
Upgrade NowBrowse More Related Video
5.0 / 5 (0 votes)