Unpopular Opinion: LLMs Didn't Add Value to AIs—They Just Made Them More Accessible
Summary
TLDRこのトークは、LMS(Language Models)が技術的に新しいテーブルを持ち出しているか、それとも単に技術をよりアクセス可能にし、利用を拡大したにすぎないかという議論の中心に立脚しています。パネルは、LMSがビジネスや一般大衆の理解にどのように影響を与えたか、そしてこれらのモデルが将来どのように進化し、どのよう活用されるかについて洞察を提供します。彼らは、LMSが特定の業界でどのように使用され、その利点と課題をどのようにバランスを取るかについても議論します。
Takeaways
- 🤖 AIは、人間のように行動するコンピュータシステムの総称であり、特に機械学習がその大きな分野の中で中心的な役割を果たしています。
- 📊 LMS(Language Models)は技術的には新しいものではなく、RNNやCNNなどの既存技術に基つていますが、より高いアクセス性と広範な利用をもたらしました。
- 🚀 Transformerアーキテクチャは、より大きなテキストや文脈を処理できるようになり、自然言語処理の分野で大きな進歩を遂げました。
- 🧠 LMSの進化は技術的な進歩だけでなく、ビジネス価値の発見と適用面での革新も重要です。
- 💡 LMSは現在、単純なタスクを自動化し、生産性を向上させることができていますが、複雑な意思決定やビジネスプロセスを完全に takeover することはまだ達成されていません。
- 📈 LMSの成功事例は、医療や法律などのテキストに基づく分野で特に高いポテンシャルがあり、専門用語や規則に基づいたタスクを効率的に処理できるようになりました。
- 🔍 LMSの信頼性については、まだ完璧ではありません。実際の専門家と比較して、LMSは試験で高いスコアを獲得しても、実際の業務では不十分な場合があります。
- 🌐 LMSが将来的にシステムとの最初の接触点となる可能性があるとの視点があるが、効率的なユーザーインタラクションを実現するために、様々な方法が検討され続けています。
- 🔗 LMSの発展は、多様な言語や文化を考慮に入れた包括的なアプローチが求められ、これにより技術の普及と利用が促進されます。
- 📚 LMSの今後の進化は、応答の正確さと信頼性を向上させることで、より多くのビジネス分野で実装され、より広範に受け入れられるようになるでしょう。
Q & A
LMSが既存のAIシステムにどのような価値を提供したと思いますか?
-LMSは、技術的には新しい価値を提供していないものの、よりアクセスしやすいインターフェースを提供し、既存のAIシステムをより广く利用できるようにしました。
LMSが技術的にどの分野で進化を遂げたと感じますか?
-LMSは、Transformersアーキテクチャを基にしており、より大きなテキストデータセットを扱えるようになり、自然言語処理のコンテキストを大幅に改善しました。
LMSがビジネス価値を提供するための課題は何だと思いますか?
-LMSがビジネス価値を提供するためには、技術的な課題だけでなく、適用層でのイノベーションも重要です。特定のビジネスニーズに合ったLMSの活用方法を見つけることが鍵となります。
LMSが医療や法務などの規制された分野でどのように役立つでしょうか?
-LMSは、医療や法務などの分野で、豊富なテキストデータの処理やルールベースの分析を行い、専門家の仕事をサポートすることができます。ただし、LMSは最終的な決定を下すわけではなく、専門家の判断を補完する役割を果たします。
LMSの応用において、どのような技術的な課題があると感じますか?
-LMSの応用においては、技術的な正確性と信頼性の向上、そして実際の現場での適用可能性の確認が課題となっています。また、LMSが誤った情報を提供するリスクにも注意が必要です。
LMSが自然言語処理の分野でどのような進化を見せましたか?
-LMSは自然言語処理の分野で、より長いテキストや複雑な文脈を理解し、応答する能力を向上させました。これにより、より自然な人机交互が実現し、さまざまな業界で応用が可能となりました。
LMSを導入する際、どのようなビジネスのニーズを考慮すべきですか?
-LMSを導入する際には、ビジネスプロセスの効率化、カスタマーサービスの改善、データ分析の支援など、企業が抱える課題やニーズに応じた適切な活用方法を検討する必要があります。
LMSが提供する自然言語処理能力を最大限に活用するためには、どのようなアプローチが効果的ですか?
-LMSを効果的に活用するためには、適切なデータセットで事前学習を行い、特定のタスクや業界に関連するデータをファインチューンすることが重要です。また、ユーザーとのインタラクションを通じてLMSを徐々に改善していくことも効果的です。
LMSの開発において、今後どのような進化が期待されています?
-LMSの開発において、今後期待される進化には、より高精度な自然言語理解能力の向上、多様なデータタイプとの連携能力の強化、そして倫理的な問題に対する対処などが含まれます。
LMSが誤った情報を提供するリスクがあることはどのように対処すべきですか?
-LMSが誤った情報を提供するリスクに対しては、適切なデータセットで学習させ、定期的な評価とファインチューニングを行い、また、重要な決定においては人間の審査や介入を確保することが重要です。
LMSを導入する際のユーザーエクスペリエンスの改善について、どのようなポイントが重要ですか?
-LMSを導入する際には、ユーザーが自然で使いやすいインターフェースを提供し、不明瞭なプロンプトや質問を最小限に抑えることが重要です。また、フィードバックメカニズムを設けることで、ユーザーの声を取り入れながらLMSを改善していくことも効果的です。
Outlines
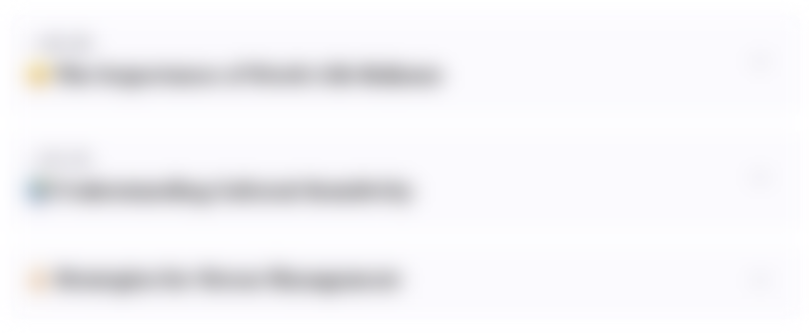
This section is available to paid users only. Please upgrade to access this part.
Upgrade NowMindmap
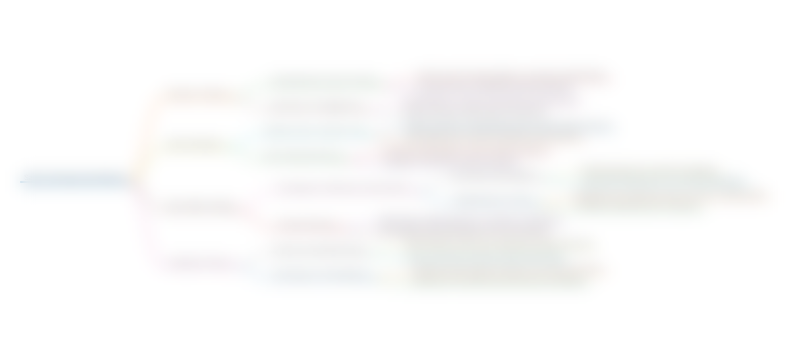
This section is available to paid users only. Please upgrade to access this part.
Upgrade NowKeywords
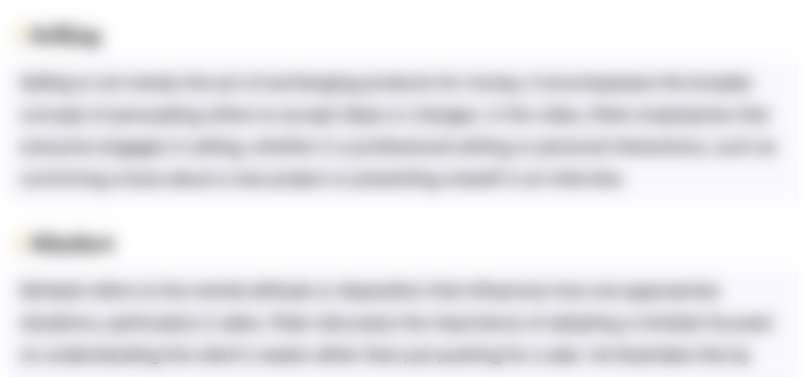
This section is available to paid users only. Please upgrade to access this part.
Upgrade NowHighlights
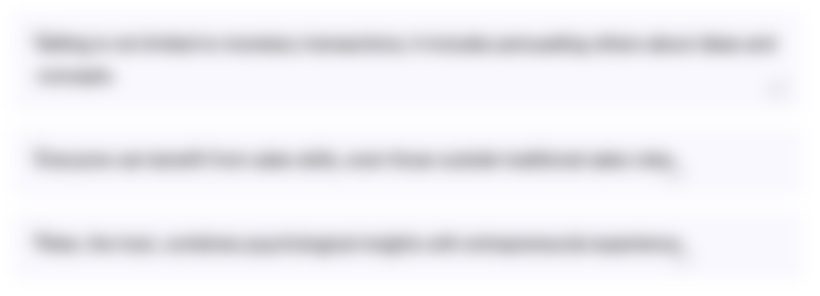
This section is available to paid users only. Please upgrade to access this part.
Upgrade NowTranscripts
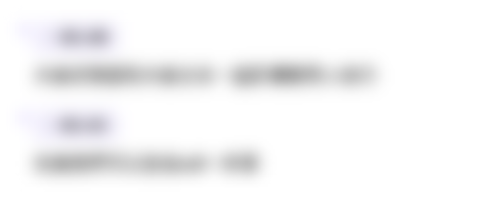
This section is available to paid users only. Please upgrade to access this part.
Upgrade NowBrowse More Related Video

【AIで画像が喋る】リップシンクが可能なサービス3つを比較【EMOっての凄い】

IoT: Beyond the Smart Gadgets | Gina Martinez | TEDxLewisUniversity

2019 Keynote Discussion Sam Altman and Vinod Khosla
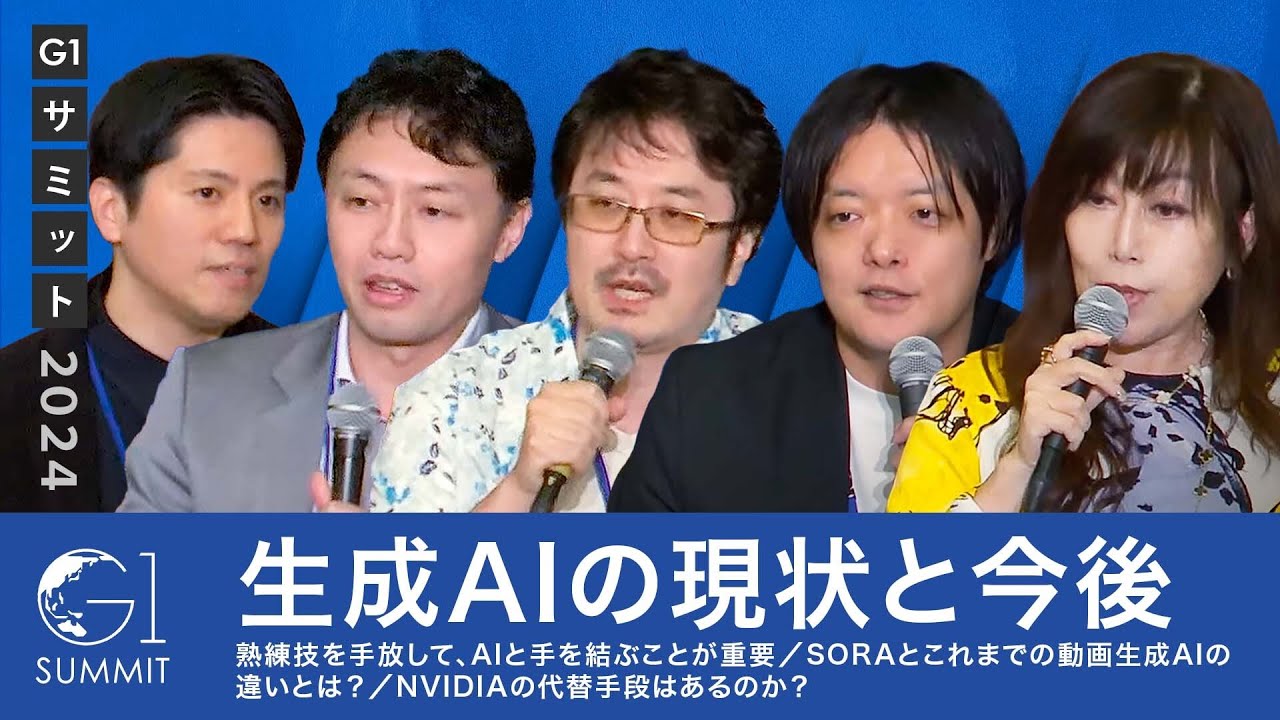
生成AIの現状と今後/熟練技を手放して、AIと手を結ぶことが重要/Soraとこれまでの動画生成AIの違いとは?/NVIDIAの代替手段はあるのか?【松尾豊×岩村水樹×上野山勝也×川上英良×関灘茂】

'AI Superpowers': A Conversation With Kai-Fu Lee

Billionaire BLOWN Away By Tesla FSD v12
5.0 / 5 (0 votes)