Customized Hardware, but for AI
Summary
TLDRThe video script discusses a new startup called Talas that aims to revolutionize AI hardware efficiency. Talas proposes designing dedicated AI chips for specific models, optimized for performance and cost-effectiveness. By combining highly optimized models with structured ASICs (a hybrid between FPGAs and ASICs), Talas claims to achieve 10 to 1000x better efficiency compared to existing solutions. This approach targets edge and embedded devices, enabling AI capabilities in resource-constrained environments where traditional GPUs or internet connectivity may not be feasible. With $50 million in funding, Talas plans to demonstrate a proof of concept by the end of the year.
Takeaways
- π€ The video discusses a new startup called Talas that aims to create highly efficient and dedicated AI chips for specific models, promising 10-1000x better performance and efficiency compared to current hardware.
- π© Talas' approach involves designing custom silicon optimized for individual AI models, rather than using general-purpose hardware like GPUs or CPUs.
- π» Their technology sits between fully programmable hardware (like CPUs/GPUs) and fully configurable hardware (like FPGAs), utilizing a concept called 'structured ASICs' or 'easic' business.
- β‘ By hardening the final few layers of metallization in the chip, Talas can achieve dedicated ASIC-like speeds while retaining some configurability for different models.
- π° According to the video, the cost of AI hardware could become a bottleneck, and Talas' approach aims to reduce this cost, especially for edge devices and embedded systems.
- π The video suggests that AI models will become ubiquitous, present in various devices like smart meters, cars, and electronics, necessitating efficient and dedicated hardware solutions.
- π Talas, founded by former Nvidia executive Lua Bic, has raised $50 million in funding and plans to tape out their first chip by the end of the year, with customer deployments expected in 2024.
- π While AI models and architectures are rapidly evolving, Talas' approach targets models that are well-defined and unlikely to change significantly over 10-30 years.
- π§ The video positions Talas' technology as a potential solution for edge and edge inference applications, rather than large-scale training workloads.
- π Overall, the video presents Talas as an innovative startup aiming to disrupt the AI hardware landscape with highly efficient and dedicated silicon solutions for specific models.
Q & A
What is the main topic discussed in the script?
-The script discusses a new startup called Talis that claims to achieve 10 to 1,000 times better efficiency for AI hardware by developing dedicated AI chips optimized for specific machine learning models.
Why is the development of dedicated AI chips considered innovative?
-The idea of developing dedicated AI chips tailored to specific machine learning models is innovative because it departs from the current approach of using general-purpose hardware (like GPUs) or reconfigurable hardware (like FPGAs) for running various AI models. Dedicated chips can potentially provide better performance and efficiency for specific models.
What is the key advantage of Talis' approach according to the script?
-The script suggests that Talis' approach of developing dedicated AI chips for specific models can lead to better performance, better efficiency, and lower hardware costs compared to using general-purpose or reconfigurable hardware for running AI models.
How does Talis' approach differ from using FPGAs?
-While FPGAs offer fully configurable hardware, Talis' approach involves developing structured ASICs or what Intel calls "eASICs". These chips have a reconfigurable part, but in the final few layers of metallization, some pathways are hardened or fixed, providing ASIC-like speeds while retaining some configurability.
What is the potential market for Talis' dedicated AI chips?
-The script suggests that Talis' dedicated AI chips could be useful for edge and edge inference applications, especially in devices that don't connect to the internet and require efficient, low-power AI processing for tasks like power management, image correction, or voice interaction.
What is the significance of the name 'Talis'?
-The script mentions that 'Talis' means 'locksmith' in Hindi, likely referring to the company's goal of developing dedicated, optimized hardware solutions for specific AI models or 'locks'.
Who is the founder of Talis, and what is their background?
-The script states that Talis was founded by Lua Bic Urein, who was previously the founder of Tenstorrent (a company focused on AI hardware). Urein left Tenstorrent about a year ago, and Jim Keller now runs that company.
What is the current status of Talis' development efforts?
-According to the script, Talis is expecting to have a chip tape-out (a completed chip design ready for manufacturing) by the end of the year, and they aim to have their technology proliferated to customers by next year.
What is the potential impact of widespread adoption of dedicated AI chips?
-The script suggests that if dedicated AI chips become ubiquitous, they could be used in various devices like smart meters, cars, and electronic devices for tasks like advanced power management, image correction, or voice interaction, even in devices that don't connect to the internet.
What is the significance of the statement "AI and machine learning is still such a rapidly developing market"?
-This statement highlights the rapidly evolving nature of the AI and machine learning field, suggesting that the need for dedicated, optimized hardware solutions may arise as new models and applications continue to emerge and evolve over time.
Outlines
π€ The Landscape of AI Hardware and the Emergence of a New Startup
The video script discusses the current state of AI hardware, with various companies offering different chips like TPUs, GPUs, CGAs, and specialized cores. It then introduces a startup called Talas (meaning 'locksmith' in Hindi), funded by Lua Bic, the founder of Tencent. Talas proposes a novel approach to AI hardware design, aiming to create dedicated AI chips tailored for specific models, with the potential to achieve 10 to 1000 times better efficiency compared to existing solutions.
π Talas' Innovative Approach to Dedicated AI Chips
The script delves into Talas' approach, which involves creating dedicated AI chips optimized for specific models that are not expected to change for an extended period (10-30 years). This approach aims to address the high costs associated with designing chips for frequently changing models. Talas proposes a middle ground between standard AI accelerators and fully reconfigurable hardware, using a technique called structured ASICs or eASICs. This technique combines the benefits of dedicated ASICs and the flexibility of reconfigurable hardware, potentially reducing costs and improving efficiency for applications like edge and edge inference, where dedicated, low-power AI chips could be ubiquitous.
Mindmap
Keywords
π‘AI Chips
π‘Machine Learning Models
π‘Dedicated AI Chips
π‘Taras
π‘FPGAs
π‘Structured ASICs
π‘Edge Inference
π‘Ubiquitous AI
π‘Hyper-optimized Models
π‘Tape Out
Highlights
The startup Talas, which means 'locksmith' in Hindi, aims to create dedicated AI chips per model, with the goal of achieving 10 to 1,000x better efficiency for AI hardware.
Talas is proposing a solution that sits between a standard AI accelerator and fully reconfigurable hardware, called a structured ASIC or 'eASIC', which combines the configurability of FPGAs with the dedicated, hardened pathways of ASICs.
The key benefit of Talas' approach is the ability to extract dedicated ASIC-like speeds while retaining some configurability, potentially reducing hardware costs and improving efficiency.
Talas believes their approach is the future of machine learning, especially for edge and edge inference applications, where dedicated, ultra-low power, and cheap AI hardware is needed.
Talas is aiming to have their first chip tapeout by the end of 2023 and customer proliferation in 2024, showcasing a proof-of-concept for their technology.
The company has received $50 million in funding from Lua Bic, the former founder of Tenstorrent, who left the company about a year ago.
Designing a chip for each AI model is typically expensive, but Talas' approach aims to reduce costs by leveraging structured ASICs.
AI models are expected to become ubiquitous, appearing in devices like smart meters, cars, and electronic devices for tasks like power management and image processing.
The founder of Talas, Lua Bic, previously founded Tenstorrent and left the company about a year ago, with Jim Keller now running Tenstorrent.
Talas is based in Toronto, Canada.
The transcript discusses the rapid pace of development in machine learning and AI, with new models, architectures, and optimizations being introduced frequently.
The need for configurable and programmable hardware has increased to handle the diversity of AI models and optimizations.
GPUs and dedicated AI accelerators have been used to handle various AI workloads, but Talas aims to create specialized hardware for specific, well-defined models.
FPGAs offer fully configurable hardware but with overhead, while Talas' structured ASICs aim to combine configurability with dedicated ASIC-like speeds.
The transcript mentions the potential for machine learning in various devices, such as cameras, ring lights, and other electronic devices, for tasks like power optimization and image correction.
Transcripts
we're currently in a fast-paced world
where there are tons of AI chips in the
market we have Google with tpus we have
Nvidia with gpus we have companies like
sambanova with cgas and cerebrus with
massive wafer scale down to T tent with
106 cores and then all the embedded
markets with all the little analog and
neuromorphic and all those different
sorts of cores that you've seen on this
channel before what if I have to tell
you that there is another way in this
video we're going to speak about a new
startup who says that they can go
forward another 10 to 1,000x in
efficiency for AI
Hardware so what this company is doing
is actually quite Innovative um it's not
a necessarily a brand new idea but it's
definitely being applied in a brand new
context now machine learning and AI
currently whether it's Hardware or
software Ware is pretty fast placed
we've got new models being developed all
the time new architectures for those
models how they're applied how they're
used and how they perform how they're
optimized everything is being moving at
roughly a fairly fast pace so as a
result we've needed a lot of
configurable Hardware in order to do
that or at least programmable Hardware
the ability to use it in different ways
in order to extract performance
regardless of whether it's doing this
little niche or that little niche or
this new optimization uh the big changes
are things like
Transformers uh that's required almost a
new dedicated sort of hardware on top of
that but the whole point is if you use a
GPU you can pretty much do anything if
you use one of these new dedicated AI
as6 as long as you're using tens FL pie
torch it's usually pretty okay what if
some of those models were very well
defined the ability to look at a model
and say this isn't going to change for
10 20 30 years it's highly optimized we
know the code paths we know exactly how
it's going to perform what if we had
silicon dedicated to that exact model
and this this is what this startup is
going to do it's called talas which
means locksmith in Hindi and it's a new
startup with about $50 million in
funding by Lua Bic you reain remember
Lua from being the founder of ten
torrent he left tenens torrent about a
year ago Jim Keller now runs that
company and I do a bit of work with them
but his new startup based in Toronto
Canada is called talas I keep wanting to
call it Talis but it's talas and their
whole point is what if you had dedicated
AI chips per model now this seems a bit
far-fetched I mean designing a chip
takes millions and millions and millions
and millions of dollars um which means
if you have one per model and the models
are changing frequently then that's
going to be Millions for that chip
Millions for that chip Millions for that
chip now now there is one sort of
solution here you could go down Route
it's called the fpga route fpga is fully
configurable hardware and in that
instance you get essentially a fully
optimized version of your code path and
you can change it with a different Co
bit stream in order to change those
Pathways for your optimized model what
we think talas is proposing here is
something that sits in between you know
a standard AI accelerator and that fully
reconfigurable Hardware something called
a structured Asic or what Intel calls
this easic business what you have here
is a reconfigurable hardware like an
fpga but in the final few letter layers
of metallization you fix some of those
Pathways to be what's called hardened
that means that there's no overhead for
reconfigurable logic uh you get
dedicated Asic like speeds but the
configurability of having different of
having the same
uh chip being manufactured but then
being optimized in different ways it's
not something that we necessarily speak
about a lot in the industry just because
we either have things like dedicate we
have like CPU cores and gpus which are
fully programmable uh logic and then you
have fpga which is reconfigurable
Hardware this is essentially trying to
meet in the middle with that the
benefits of having an Asic on top what
Talis and the beer are saying here is
that they can extract a 10 to a,x better
performance better or better efficiency
as well um and may bring the cost of the
hardware down what they say is the
what's going to be an issue moving
forward with some of this machine
learning stuff is the cost of the
hardware not everybody can buy gpus
perhaps you don't want a GPU in your
small embedded device you needed a
dedicated AI accelerator with dedicated
AI Pathways that's super efficient maybe
the device it's going in will never
connect to the internet for 10 20 30
years you know what code is going to be
on there and as a result you can have
dedicated Hardware just for the model
you're running on that piece of Hardware
now we could go into a conversation here
about the proliferation of AI models um
we I expect AI models to be almost as
ubiquitous as electricity it's going to
be in the small chips in your smart
meters in your cars in your or any any
electronic device it's going to be doing
things like Advanced power management it
may not be doing things like large
language models uh they maybe you do
have a small handheld device that
doesn't connect to the internet that
will have to interact uh with voice or
with language in some way or you know
image generation that's like I say Ai
and machine learning is still such a
rapidly developing Market it depends
what you're going to be using these
devices for I mean right now I've got a
camera in front of me the camera doesn't
connect to the internet but it could use
machine learning for power optimization
or for uh image correction because
goodness knows this image is probably
terrible but then behind it I've got a
ring light and maybe the ring light
needs machine learning again for
adapting some of the LEDs perhaps the
performance of the LEDs changes over
time and perhaps you can put that into a
dedicated Asic that's cheap to make
cheap to proliferate ultra low power and
could potentially become ubiquitous this
is essentially what Talis are doing
they're calling it Talis Foundry so it's
a combination of hyper optimized models
and then hyper optimized silicon
producing this net benefit and they
think that's the future of where machine
learning has to go especially when we're
talking about Edge and Edge inference
we're not necessarily talking about the
big training right now this is mainly an
edge and Edge inference um so good luck
to Leia and his team they're expecting a
chip tape out by the end of the year and
proliferation into customers by next
year um as a generalized language model
uh chip I say generalized it's going to
be dedicated for a specific customer I'm
pretty sure but they've got to show off
a proof of concept and they got to show
that this technology works we'll be
staying tuned and we'll be keep we'll
keep on top of what announcements
they'll make in the
future
Browse More Related Video
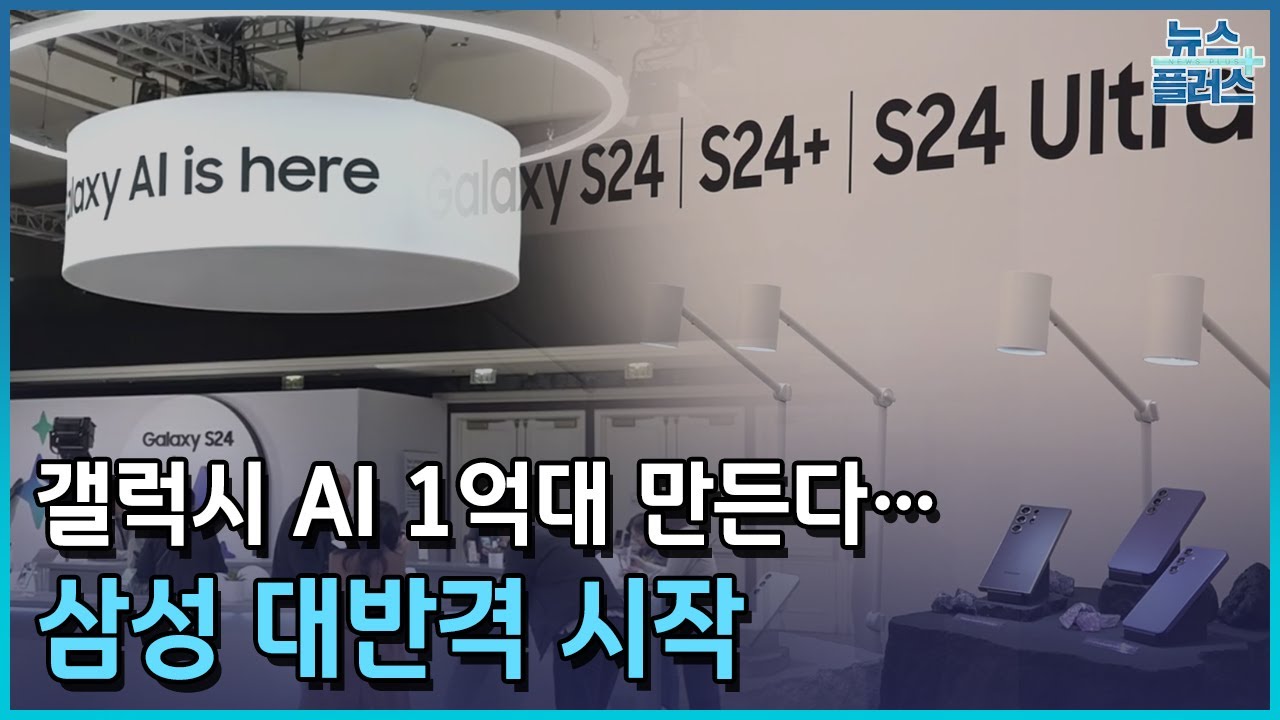
κ°€λμ AI 1μ΅λ λ§λ λ€β¦μΌμ± λλ°κ²© μμ/νκ΅κ²½μ TVλ΄μ€
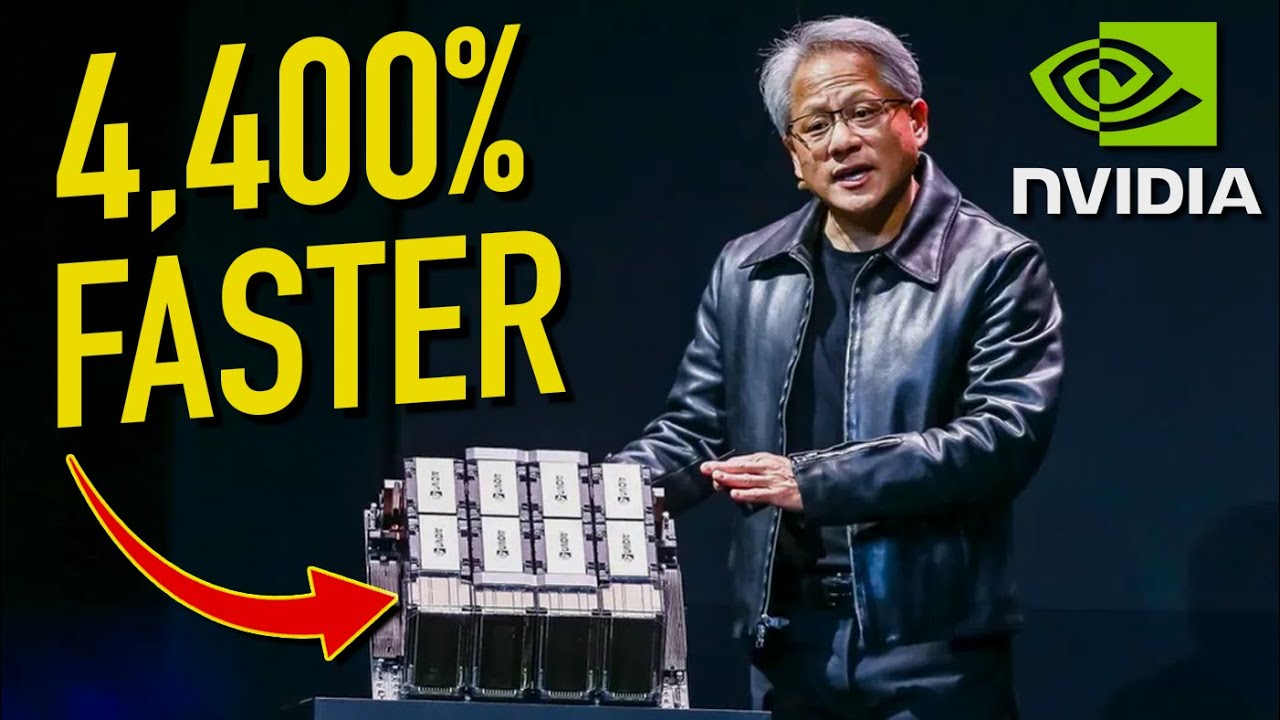
NVIDIA'S HUGE AI Chip Breakthroughs Change Everything (Supercut)

How Google Makes Custom Cloud Chips That Power Apple AI And Gemini
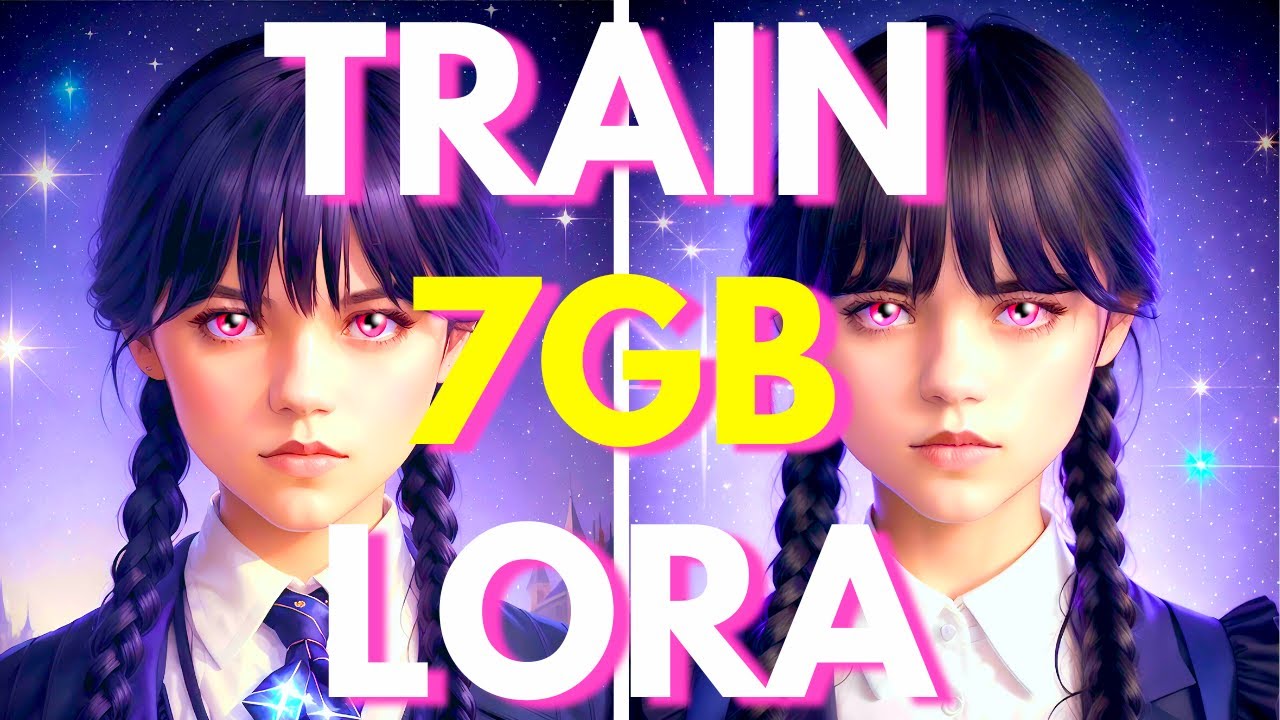
ULTIMATE FREE LORA Training In Stable Diffusion! Less Than 7GB VRAM!
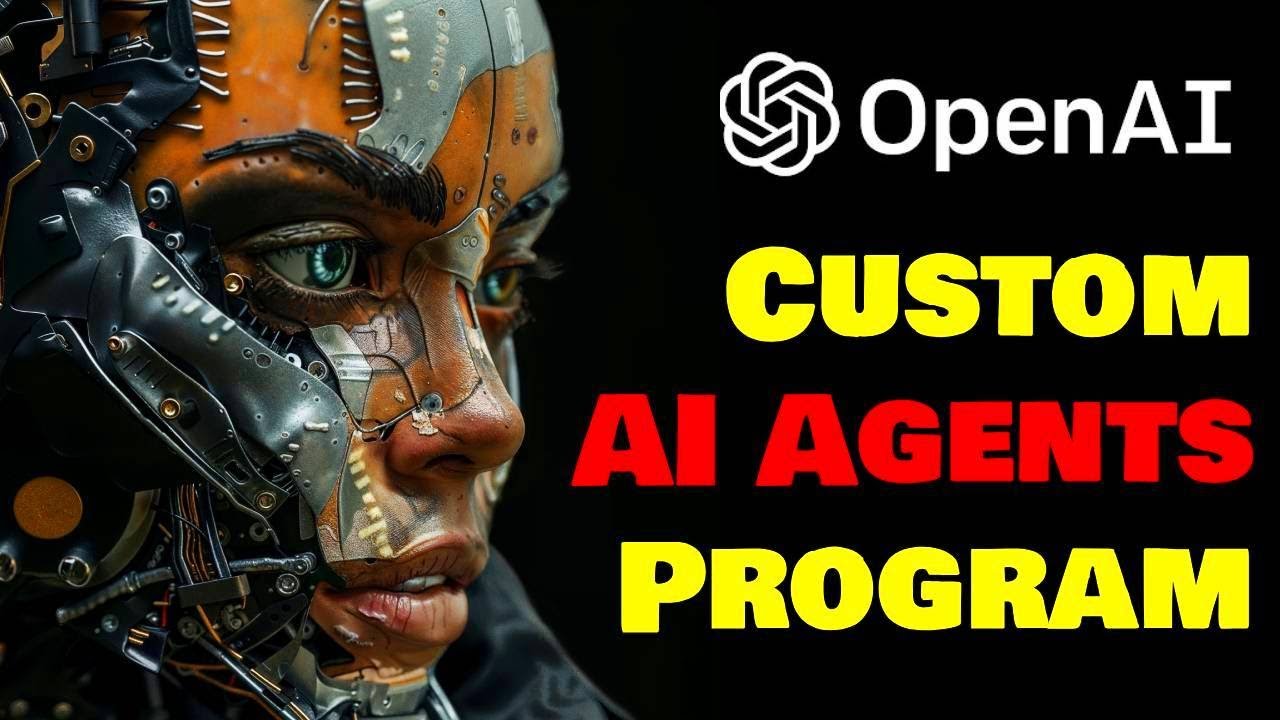
OpenAI's STUNNING "GPT-based agents" for Businesses | Custom Models for Industries | AI Flywheels
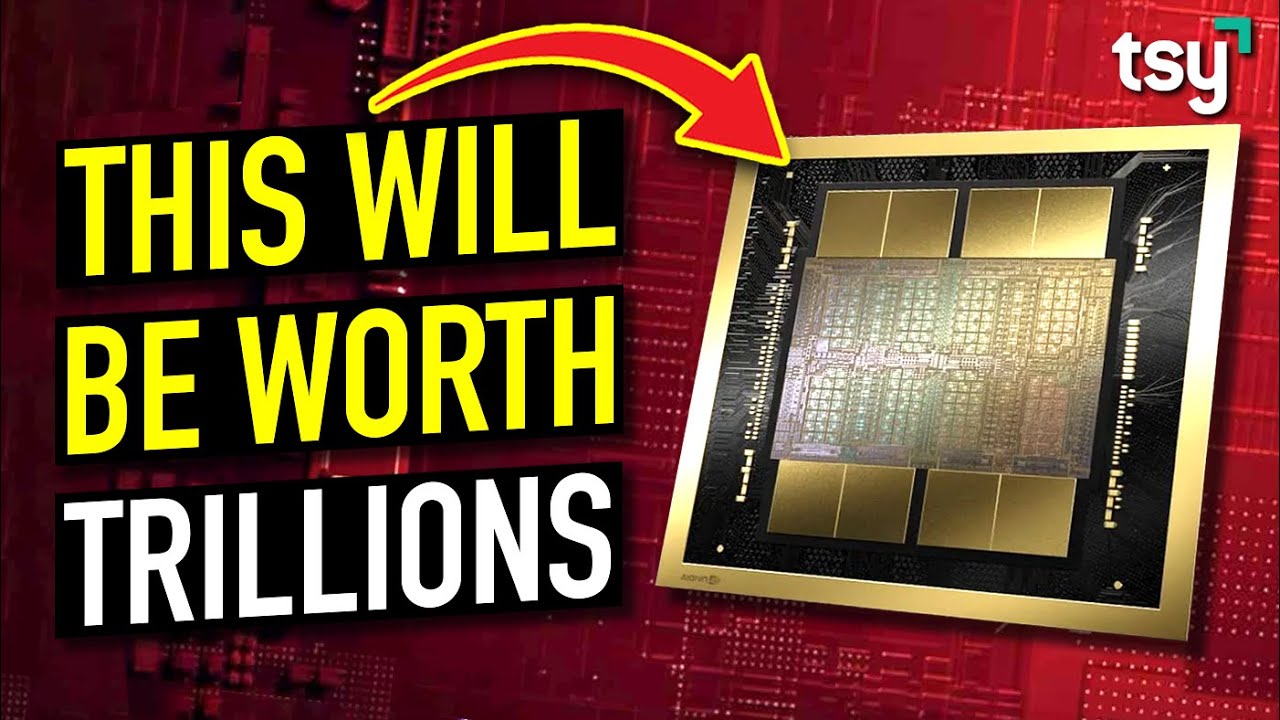
GET IN EARLY! I'm Investing In This HUGE AI Chip Breakthrough
5.0 / 5 (0 votes)