Where We Go From Here with OpenAI's Mira Murati
Summary
TLDRIn this insightful discussion, the guest shares their journey from Albania to working at OpenAI, emphasizing the importance of math and science in their upbringing. They delve into the challenges of building products with AI models, exploring the evolution from theoretical concepts to practical applications in fields like aerospace and automotive engineering. The conversation highlights the significance of AI safety, alignment with human values, and the potential of large language models to transform how we interact with technology, suggesting a future where AI systems could autonomously perform intellectual tasks, raising questions about reliability and the economic implications of AI advancements.
Takeaways
- ๐ The speaker emphasizes the difficulty of building good products on top of AI models, suggesting that while model creation is a focus, the real challenge lies in application.
- ๐ Born in post-Communist Albania, the speaker's early education was heavily focused on math and sciences, which influenced his career path in mechanical engineering and AI.
- ๐ With a background in mechanical engineering and aerospace, the speaker's interest in AI was sparked during his time at Tesla, particularly with the development of autopilot systems.
- ๐ถ๏ธ The speaker explored augmented reality and virtual reality, noting the importance of understanding the practical applications and limitations of these technologies.
- ๐ง The shift in focus from theoretical knowledge to building practical applications is highlighted, with an emphasis on the generality of AI over domain-specific competence.
- ๐ฎ OpenAI's mission attracted the speaker due to its focus on building AGI (Artificial General Intelligence), which he sees as a fundamental and inspiring goal for technology development.
- ๐ค The speaker discusses the importance of AI safety, particularly the development of reinforcement learning with human feedback to align AI systems with human values.
- ๐ The dialogue capability of AI models like Chat GPT is seen as a special form of interaction that allows for the expression of uncertainty and correction of errors, contributing to AI safety.
- ๐ ๏ธ The speaker suggests that we are at an inflection point in redefining human-digital interaction, moving towards a model where AI systems act more as collaborators than tools.
- ๐ The transcript discusses the concept of scaling laws in AI, indicating that there is still significant room for improvement as models scale up in terms of data and compute power.
- ๐ก The future of AI is envisioned with models that encompass multiple modalities (text, images, video) for a more comprehensive understanding, akin to human perception.
Q & A
What was the educational focus in post-Communist Albania and how did it influence the speaker's interests?
-In post-Communist Albania, there was a significant focus on math and physics, which influenced the speaker to develop a strong interest in these fields. The humanities were considered questionable due to the ambiguity of information sources and truthfulness, leading the speaker to pursue math and sciences relentlessly.
How did the speaker's career evolve from mechanical engineering to working with AI?
-The speaker started as a mechanical engineer working in Aerospace and later joined Tesla, where they initially worked on Model S dual motor and then led the Model X program. This experience sparked an interest in AI applications, particularly autopilot, which led them to explore AI in different domains such as augmented reality, virtual reality, and eventually joining OpenAI to focus on general AI (AGI).
What was the speaker's initial attraction to OpenAI?
-The speaker was drawn to OpenAI because of its mission, which they felt was more important than any other technology they could contribute to. They believed that building intelligence is a core unit that affects everything and is inspiring for elevating and increasing the collective intelligence of humanity.
How does the speaker view the role of physics and math backgrounds in the field of AI today compared to 15 years ago?
-The speaker observes that many influential contributors to the AI space today have a physics or math background, which is a shift from 15 years ago when the field was more dominated by engineers from lexical, mechanical, and other engineering backgrounds.
What does the speaker mean by 'building an intuition' in the context of problem-solving in math and theoretical spaces?
-The speaker refers to the process of deeply engaging with a problem over an extended period, often requiring time for reflection and incubation, which leads to the development of a new idea or solution. This process builds an intuition for identifying the right problems to work on and the discipline to persevere until a solution is found.
How does the speaker perceive the current state of AI systems in terms of their capabilities and limitations?
-The speaker sees AI systems as having made significant progress, particularly in representation and understanding of concepts, but acknowledges that there are still substantial limitations, especially regarding output reliability, such as the issue of hallucinations and the need for models to express uncertainty.
What is the speaker's perspective on the future of programming and AI interaction?
-The speaker believes that we are at an inflection point where the way we interact with digital information is being redefined through AI systems. They envision a future where natural language interfaces become more prevalent, allowing for collaboration with AI models as if they were companions or co-workers.
What was the original intent behind creating Chat GPT, according to the speaker?
-Chat GPT was initially created as a means to get feedback from researchers on the dialogue modality of AI, with the goal of using this feedback to improve the alignment, safety, and reliability of more advanced models like GPT-4.
How does the speaker define AGI (Artificial General Intelligence)?
-The speaker defines AGI as a computer system capable of performing autonomously the majority of intellectual work, indicating a level of capability that spans a wide range of tasks beyond specific domains.
What are the speaker's thoughts on the future of AI in terms of economics and the workforce?
-The speaker anticipates that AI systems will take over more tasks, allowing humans to focus on higher-level work and potentially reducing the amount of time spent working. They also suggest that AI could lead to a more powerful and accessible platform for building applications and products.
What is the speaker's view on the potential future of AI models in terms of their capabilities and roles?
-The speaker expects AI models to become incredibly powerful, with the ability to understand and interact with the world across multiple modalities. They also highlight the importance of addressing the challenge of super alignment to ensure these models are safe and aligned with human intentions.
Outlines
๐ From Albania to AI: A Journey of Intellectual Curiosity
The speaker reflects on their journey from being born in post-communist Albania to developing a deep interest in math and sciences. This early education in a politically isolated country with a strong emphasis on math and physics led to a career in mechanical engineering and eventually to Tesla, where they contributed to the development of the Model S and X. The speaker's focus on applying knowledge to build practical applications, especially in AI, led them to explore various domains including augmented reality, virtual reality, and eventually to OpenAI, where they became deeply involved in the development of AGI (Artificial General Intelligence).
๐ค The Convergence of Physics and AI: A New Era of Problem Solving
The speaker discusses the increasing intersection between physics and computer science, noting that many influential figures in AI have a background in physics or math. They highlight the unique problem-solving approach required in theoretical fields, which involves deep contemplation and patience. The conversation delves into whether AI development is becoming more of a systems or engineering problem, with the speaker suggesting that while systems and engineering challenges are significant, there is still much to explore in terms of fundamental advancements in AI.
๐ ๏ธ The Evolution of AI Interfaces: From APIs to Natural Language
This section explores the evolution of how humans interact with AI, moving from traditional APIs to more natural language interfaces. The speaker shares their personal experience using AI models and the feeling of using a 'supercomputer with an abacus', indicating the disparity between the power of AI models and the simplicity of current programming interfaces. They speculate on the future of programming, suggesting that it may become less abstract and more collaborative, with AI models acting as partners in the creative process.
๐ The Birth of Chat GPT: A Focus on Safety and Alignment
The speaker recounts the origins of Chat GPT, which was not initially intended as a product but emerged from research into safe AI systems. They discuss the development of reinforcement learning with human feedback to align AI systems with human values and prevent misuse. The speaker also touches on the challenges of hallucinations in AI and the efforts to make models more reliable and safe through fine-tuning and user feedback.
๐ฎ Prognosticating AI's Future: Scaling Laws and Economic Implications
In this paragraph, the speaker addresses the question of AI's future, particularly the concept of scaling laws and whether the pace of progress will continue. They express optimism about the potential for AI to become more capable as models scale up, despite the historical pattern of diminishing returns in AI. The speaker also considers the economic impact of AI, drawing parallels with the silicon industry and speculating on whether general AI models will dominate or if there will be a fragmentation of specialized models.
๐ The Expanding Role of AI: From Text to Multimodal Understanding
The speaker envisions a future where AI models encompass not just text but also images, video, and other modalities, providing a more comprehensive understanding of the world. They discuss the importance of pre-training models to have a broad sense of the world and the challenges of making AI outputs reliable through reinforcement learning with human feedback. The speaker also hints at the potential for AI to solve issues like hallucinations and the importance of building a platform that facilitates collaboration between humans and AI.
๐ The Challenge of Super Alignment: Ensuring AI Serves Human Intentions
In the final paragraph, the speaker discusses the challenge of super alignment, ensuring that increasingly powerful AI models are aligned with human intentions. They acknowledge the fear associated with powerful misaligned AI and the importance of addressing this technical challenge. The speaker also reflects on their personal stance, positioning themselves as something other than a doomer or accelerationist, indicating a nuanced view of AI's potential and risks.
Mindmap
Keywords
๐กAI
๐กAutonomy
๐กModel
๐กOpenAI
๐กAGI
๐กReinforcement Learning
๐กHallucinations
๐กAPI
๐กAlignment
๐กEconomics of AI
๐กProductization
Highlights
Building good products on top of AI models is incredibly difficult.
The speaker's background includes a focus on math and sciences, influenced by the educational environment in post-communist Albania.
Interest in applying mathematical knowledge to build things, leading to a career in mechanical engineering and aerospace.
Experience at Tesla contributed to an interest in AI applications, particularly autopilot technology.
The transition from theoretical to practical applications of AI in domains like augmented reality and virtual reality.
The importance of generality in AI and the appeal of working on AGI at Open AI.
The prevalence of physics and math backgrounds among influential figures in the AI space.
The value of a theoretical approach to problem-solving in AI development.
The difference in user engagement between APIs and more accessible AI technologies like Chat GPT.
The potential for natural language interfaces to replace traditional programming in AI interaction.
The concept of AI as a collaborative partner rather than just a tool to program.
The strategy of making AI technology accessible to discover new use cases and applications.
The development of Chat GPT as a means to gather feedback from researchers on alignment and safety.
The challenge of addressing AI hallucinations and the potential of reinforcement learning with human feedback.
The decision-making process at Open AI regarding what to work on, focusing on safety and alignment.
The economic implications of AI, drawing parallels to the evolution of the silicon industry.
The future of AI as potentially capable of performing the majority of intellectual work, defined as AGI.
The current state of AI capabilities, with a focus on reliability and expanding to new tasks.
The importance of emerging capabilities in AI, even if they are currently unreliable.
The potential trajectory of AI systems becoming autonomous and the need for human oversight.
The vision for AI platforms, allowing people to build on top of pre-trained models.
The challenge of building good products on top of AI models, which is more difficult than model creation.
The future of multi-modal AI models, incorporating text, images, and video for a comprehensive understanding of the world.
The focus on reliability in AI outputs and the introduction of methods to reduce hallucinations.
The concept of AI as a collection of collaborative agents, providing a platform for others to build upon.
The long-term challenge of super alignment in AI as models become incredibly powerful.
The speaker's perspective on AI's future, neither a doomer nor an accelerationist, but something else.
Transcripts
there is a lot of Focus right now on
building more models but you know
building good products on top of these
models is incredibly difficult
[Music]
thank you
I would love it if you're comfortable
it's giving kind of the longer form of
your background what brought you to open
AI you know just kind of bring us up to
speed and then we'll go from there yeah
so um
it was born in Albania just after the
fall of Communism very interesting times
in this you know very isolated countries
sort of similar to North Korea today and
I bring that up because it was I think
very Central to sort of my
education and focus in math and sciences
because there was a lot of focus on
maths and physics
in in Coast communist Albania and you
know the humanities like history and
sociology and these type of topics they
were a bit questionable like the source
of information and truthfulness was uh
was hard it was ambiguous so anyway I I
got very interested in math and sciences
and that's what I pursued relentlessly
until you know still still working
fundamentally in mathematics
and over time my interests grew more
from this theoretical space into
actually building things and figuring
out how to
apply that knowledge to build stuff and
I studied mechanical engineering
and went on to work in Aerospace as an
engineer and then joined Tesla shortly
after where I spent a few years
initially I joined to work on Model S
dual motor and then I went on to model X
from the early days of the initial
design and eventually led the whole
program
um to to Launch
and this is when I got very interested
in applications of AI and
specifically with autopilot and so
I started thinking more and more about
different applications of AI okay what
happens when you when you you're using
Ai and computer visually in a different
domain instead of autopilot
and after Tesla I went on to work on
augmented reality and virtual reality
because I just wanted to get experience
with different domains and I thought at
the time that it was the right time to
actually work on special computing
obviously in retrospect too early
back then but anyways I learned a lot
about the limitations of pushing this
technology to the practicality of using
it every day and at this point I started
thinking more about what happens if you
just focus on the generality like forget
the competence in different domains and
just focus on generality and there were
two places at the time there were laser
focused on this issue and open Ai and
deepmind
uh and I was very drawn to open AI
because of its Mission and I felt like
there's not going to be a more important
technology that we all built than than
AGI
um
back then I certainly did not have the
same conviction about it as I do now
but I thought that fundamentally if
you're building intelligence it's such a
it is such a core unit then the
university it affects everything and so
you know what what else is there is
there to do more inspiring than than
Elevate and increase Collective
collective intelligence of humanity
whenever I meet somebody that's a like a
real
um uh influencer and has done major
contributions to the space they almost
invariably have a physics background or
a math background which is actually very
different than it was 15 years ago like
15 years ago I was like you know the
kind of you know it was like engineers
and you know they came from lexical
engineering mechanical engineering but
it does feel like
um you know there's something and I
don't know if it's like some like quirk
in the network or like it's it's more
fundamental like systemic and I mean do
you think that this is kind of the time
for the physicist to step up and kind of
contribute to computer science and
there's something about that or do you
think it's just more of a coincidence
so I think maybe one thing to draw on
from the theoretical space of math
but also the kind of the natural
problems with math is that you know you
kind of need to sit with a problem for a
really long time and you have to think
about it sometimes to sleep and you wake
up and you have a new idea and over the
course of maybe a few days sometimes or
weeks you get to the final solution and
so it's not like a quick reward
and sometimes it's not this iterative
thing and and I think it's almost like a
different way of thinking where you're
building an intuition but also a sort of
discipline to sit with the problem and
have faith that you're going to solve it
and over time you build an intuition on
what problem is the right problem to
actually work on so do you think it's
now more of a systems problem more like
kind of more of an engineering problem
or do you think that we still have a lot
of like kind of pretty real kind of
signs to unlock
um both I think the systems and the
engineering problem is massive
um is as we're deploying these
Technologies out there
um and we're trying to scale them we're
trying to make them more efficient we're
trying to make them easily accessible so
you don't need to have you know to know
the intricacies of ml in order to use
them and actually you can see sort of
the contrast between making these models
available through an API and making the
technology available through child GPT
it's fundamentally the same technology
maybe with with a small difference with
reinforcement learning with human
feedback for chat GPT but it's
fundamentally the same technology and
the reaction and the ability to to grab
people's imagination and to get them to
just use the technology every day is
totally different I also think the API
for example PPT is such an interesting
thing so it's my program against these
models myself for fun right and it
always feels like whenever I'm using one
of these models in a program I'm like
I'm wrapping a super computer with an
abacus it's like the code itself just
seems so kind of flimsy compared to the
model that it's wrapping sometimes I'm
like listen I'm just going to give the
model
like a keyboard and a mouse and like and
let it do the programming and then
actually the API is going to be English
and I'll just tell it what to do and
it'll do all the programming and I'm
just kind of curious as you designed
stuff like chat GPT do you view that
over time the actual interface is going
to be like the like natural languages or
do you think that there's still a big
role for programs the programming is
becoming less abstract where we can
actually talk to computers in high
bandwidth in natural language but
another Vector is one where we're using
the technology and the technology is
helping us understand how to actually
collaborate how to collaborate with it
versus program it and I think there is
definitely the layer of programming
becoming easier more accessible because
you can program things in natural
language but then there is also this
other side which we've seen with child
GPT that you can actually collaborate
with the model as if it was a companion
a partner Co worker you know that's the
interesting thing like it'll be very
interesting to see what happens over
time like you've made decision to have
an API and whatever but like you don't
like have an API to a co-worker right
like you talk to a co-worker so it could
be the case that like over time these
things evolve into like you just speak
natural languages or do you think it
will always be a component of a finite
State machine a traditional computer
that's it yeah I think this is right now
an inflection point where we're sort of
you know redefining how we interact with
with digital information and it's it's
through you know the form of this AI
systems that we collaborate with and uh
maybe we have several of them and maybe
they all have different competences and
maybe we have a general one that kind of
follows us around everywhere knows
everything about uh you know my context
what I've been up to today what my goals
are
um sort of in life at work and kind of
guides me through and coaches me and so
on and you know you can imagine that
being super super powerful so I think it
is we are right now at this inflection
point of redefining what this looks like
um but you know
but there is also
we don't know exactly what the future
looks like and so
we are trying to make these tools
available and the technology available
to a lot of other people so they can
experiment and we can see what happens
it's a strategy that we we've been using
from the beginning and also with child
GPT where you know the week before we
were worried that it wasn't good enough
and we also what happened you know we
put it out there and then people told us
it is good enough to discover new use
cases and you see all this emergent use
cases that I know you've written about
um and that's what happens when you make
this stuff accessible and easy to use
and put it in the hands of everyone so
this leads to my my next question which
is
um so you invent cold fusion and then
part of it you're like okay listen I'll
just give people like electrical outlets
and they'll use the energy but like I
think when it comes to AI people don't
really know how to think about it yet
and so like there has to be some
guidance like you have to make yeah some
choices and so you know you're an
opening you know you have to decide what
to work on next well if you could like
walk through that decision process like
how do you decide like what to work on
or what to focus on or what to release
or how to position it if you consider
how Chad GPT was born it was not born as
a product that we wanted to put out
there in fact the real roots of it go
back to like more than five years ago
when we were thinking about how do you
how do you make this safe AI systems
um and you know you don't necessarily
want humans to actually write the the
goal functions because you don't want to
use proxies for complex call functions
or you don't want to get it wrong it
could be very dangerous this is where
reinforcement learning with human
feedback was was developed where you
know
but what we were trying to really
achieve was to align the the AI system
to human values and get it to receive
human feedback and based on that human
feedback it would be more likely to do
the right thing less likely to do the
thing that you don't want it to do
and uh you know after we developed gpt3
and we put it out there in the API this
was the first time that we actually had
Safety Research become practical into
the real world and this happened through
instruction following models so we use
this method
to basically take
prompts from customers using the API and
then we had contractors
generate feedback for the model to learn
from and we fine-tuned the model
on on this data and build the
instruction following models there were
much more likely to to follow the intent
of the user and to do the thing that you
actually wanted to do and so this was
very powerful because AI safety was not
just this theoretical concept that you
sit around and you talk about but it it
actually became you know was sort of
going into AI Safety Systems now like
how do you integrate this into into the
real world
and obviously with large language models
we see great representation of Concepts
ideas of the real world but on the
output front there are a lot of issues
um and one of the biggest ones is
obviously hallucinations
and so
we we had been studying the issue of
hallucinations truthfulness
um how do you get these models to
express uncertainty the precursor to
child GPT was actually another
project that we called Web GPT
and it used retrieval to be able to get
information and cite sources and so this
project then eventually turned into
child gbt because we thought the
dialogue was really special because it
allows you to sort of you know ask
questions to correct the other person to
express uncertainty there's just
something down the error because you're
interacting exactly there is this
interaction and you can get to a deeper
truth
um and and so anyway we we started going
down this path and at the time we were
doing this with gpt3 and ngpts 3.5
um and and we were very excited about
this from a safety perspective
um but you know one thing that
people forget is that actually at this
time we had already trained gbd4 and so
internally at open AI we were very
excited about gbt4 and sort of put
chagibit in the rear view mirror
and uh then you know we kind of realized
okay we're gonna take six months
to focus on alignment and safety of gbt4
and we started thinking about things
that we could do and uh one of one of
the main things was actually to put
charge ubt in the hands of researchers
out there that could give us feedback
since we had this dialogue modality and
so this was the original intent to
actually
get feedback from researchers and use it
to make contributive form or aligned and
safer and more robust more reliable I
mean just for clarity when you say align
and safety do you actually
do you include in that like correct and
does what it wants or do you mean
safety like actual like protecting from
some sort of harm by alignment I
generally mean that it aligns with the
user's intents so it does exactly the
thing that you wanted to do
but safety includes other things as well
like misuse where the user is you know
intentionally trying to use the model to
generate to create harmful outputs
um
so yeah you can we were trying in this
in this case with charge GPT we were
actually
um trying to make the model more likely
to do the thing that you wanted to do to
make it more lines and uh we also wanted
to figure out the issue of
hallucinations which is obviously an
extremely hard problem but I do think
that with this method of
to reinforcement learning with human
feedback maybe that is all we need if we
push this hard dinner so there's no
grand plan it was literally like what do
we need to do to like get to AGI and
it's just one step after right yes and
it's you know all the little decisions
that you make along the way but
maybe what made it more likely to happen
is the fact that we did make a strategic
decision a couple of years ago to pursue
products yeah and we did this because we
thought it was actually crucial to
figure out how to deploy these models in
the real world and it would not be
possible to just you know sit in a lab
and develop this thing in a vacuum
without feedback from users from The
Real World so there was there was a
hypothesis and and I think that that
helped us along the way make some of
these decisions build the underlying
infrastructure so that we could actually
eventually deploy things like
I would love if you would Riff on
scaling laws I think this is the big
question that everybody has like I mean
like the pace of progress has been
phenomenal and you would love to think
that the the graph always does this but
like the history of AI seems to be that
like you hit diminishing returns at some
point and it's not parametric it kind of
like tapers off and so
from your standpoint was probably like
the most informed vantage point in the
entire industry do you think the scaling
laws are going to hold and we're going
to continue to see advancements or do
you think we're hitting diminishing
returns
so there isn't
any evidence that we will not get much
better much more capable models as we
continue to scale them across the access
of data and compute whether that takes
you all the way to AGI or not that's a
different question there are probably
some other breakthroughs and
advancements needed along the way but I
think there's still a long way to go
in in the scaling laws and to really
gather a lot of benefits from from these
larger models how do you define AGI
um in our chart Opening Our Charter we
we Define it as a computer system
basically that is able to perform
autonomously the majority of
intellectual work okay I am was that I
was at a lunch and Robert nishihara from
any scale was there and um and he asked
what I called it Robert nishihara
question which I thought was actually
very good characterization he said okay
so like you've got to continue in
between like say a computer and Einstein
so you can go from a computer to a cat
you know from a cat to an average human
and you go from an average human to
Einstein then they ask a question of
okay so where are we on the continue
what problem have we solved and the
consensus was we know how to go from a
cat to an average human like we don't
know how to go from like a computer to a
cat because like that's you know that's
the general perception problem or very
close but we're not quite there yet and
then we don't really know how to do the
Einstein which is kind of set to set
reasoning with fine tuning you can get a
lot obviously
um but in general I think we're sort of
the most tasks kind of like in turn
level I would say that's what I I
generally say the issue is reliability
right of course you know you can't fully
rely on the system to do the thing that
you wanted to do all the time and you
know how do you increase that
reliability over time and then how do
you obviously expand to the the
capabilities
um the new the emergent capabilities the
new things that these models can do I
think though that it's important to pay
attention to these emerging capabilities
even if if they're highly unreliable and
especially for people that you know are
building companies today you really want
to think about okay what what's somewhat
possible today
um what do you see glimpses of today
because you know very quickly this could
actually become
these models could become reliable so
I'd love I've been asking just a second
to prognosticate on what that looks like
but before very selfishly I've got uh
I've got a question
on on how you think the economics of
this are going to pencil out which is
I'll tell you what it reminds me of it
reminds me very much of the Silicon
industry
so I remember in the 90s when you buy a
computer there are all these weird
co-processors there's like here's like
string matching here's a floating Point
here's crypto and like all of them got
consumed into basically the the CPU it
just turns out generality was very
powerful and that created a certain type
of economy one where like you had you
know Intel and AMD and like you know it
all went in there
and of course because a lot of money to
build these chips and so like you can
imagine two Futures there's one future
where like you know generality is so
powerful that over time the large models
basically consume all functionality
and then there's another future where
there's going to be a whole bunch of
models and like things fragment and you
know different points of the design
space do you have a sense of like
is it open Ai and nobody or is it
everybody it kind of depends what you're
trying to do so obviously the trajectory
is one where these AI systems will be
doing will be doing more and more of the
work that we're doing and they'll be
able to operate autonomously but we will
need to provide Direction and guidance
and oversee but I don't want to do a lot
of the repetitive work that I have to do
every day I want to focus on other
things and maybe we don't have to work
you know
10 12 hours a day and maybe we can work
less and Achieve even higher
outputs and so that's sort of what I'm
hoping for but in terms of like how this
how this works out with with the
platform
um
you can see even today you know we make
a lot of models available through our
API and from the various from the very
small models to to our Frontier models
and people don't always need to use the
most powerful the most capable model
sometimes they just made the model that
actually fits for their specific use
case and it's far more economical so I
think there's going to be a range
um but yeah in terms of how we're
Imagining the platform play
um we definitely want people to build on
top of our models and we want to give
them tools so to make that easy and give
them more and more access and control so
you know you can bring your data you can
customize these models and you can
really focus on the layer beyond the
model and defining the products which is
actually really really hard there is a
lot of Focus right now on building more
models but you know building good
products on top of these models is
incredibly difficult okay we only have a
couple more minutes sadly I would love
for you to prognosticate a little bit
unlike where you think this is all going
like yeah like three years or five years
or ten years
I think that um the the foundation
models today obviously have this great
representation of the world in text and
we're adding other modalities like
images and video and various other
things so these models can get a more
comprehensive sense of the world around
us similar to how we understand and
observe the world the world is not just
in text it's also in images so I think
that will certainly expand in in that
direction and we'll have these bigger
models that will have all these
modalities
um and that's kind of the pre-training
part of the work where we really want to
get
these pre-trained models that understand
the world like we do and then there is
the output part of the model where we
introduced reinforcement learning with
human feedback and we want the model to
do the actually the thing that we ask it
to do and we want that to be reliable
and there is a ton of work that needs to
happen here and maybe introducing
browsing so you can get fresh
information and you can cite information
and solve hallucinations I don't think
that's impossible I think that's
achievable on the product side I think
we want to put this all together in this
collection of agents that people
collaborate with and you know really
provide a platform where people can
build on top of and you know if you
extrapolate really far out these models
are going to be incredibly incredibly
powerful and with that obviously comes
fear of them being misaligned having
this very powerful model that are
misaligned with our intentions so then a
huge challenge becomes the the challenge
of of super alignment which is a
difficult technical Challenge and we've
we've assembled an entire team at open
AI um
to just focus on on this problem so very
very very last question are you a Doomer
an accelerationist or something else
let me say something else all right
perfect thank you so much fantastic
[Applause]
[Music]
Browse More Related Video
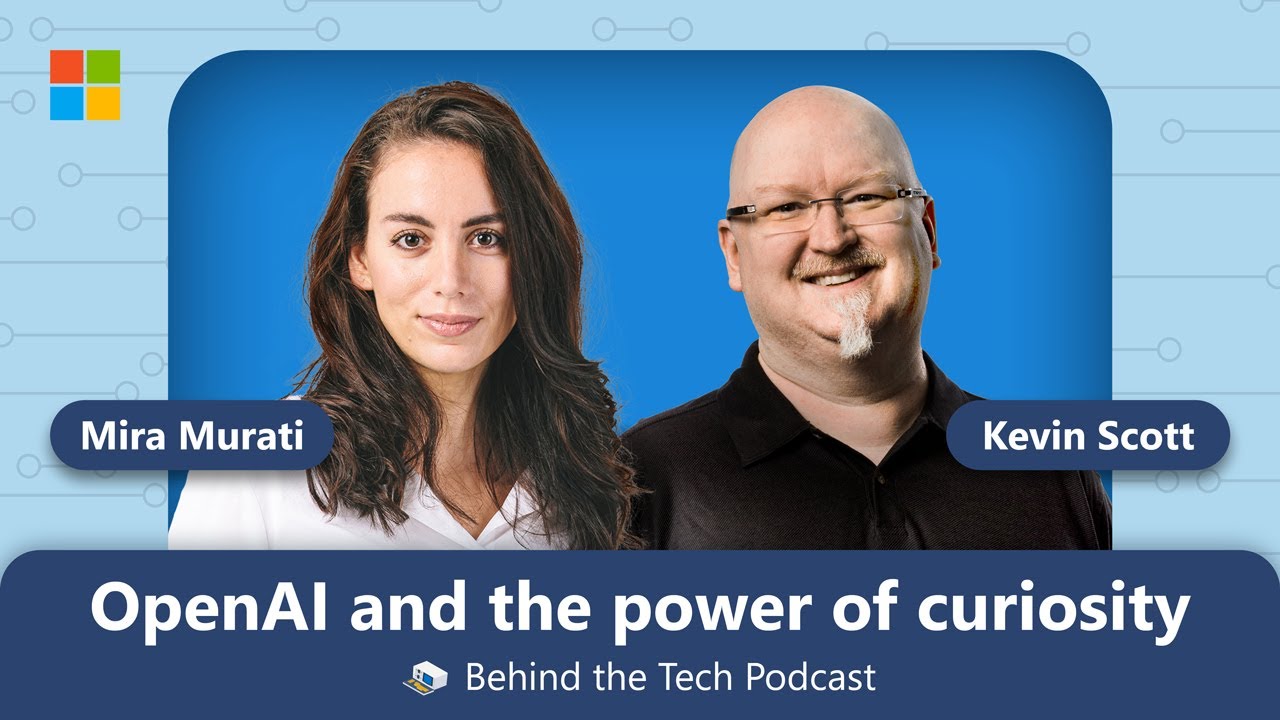
OpenAI's Mira Murati on ChatGPT and the power of curiosity | Behind the Tech with Kevin Scott
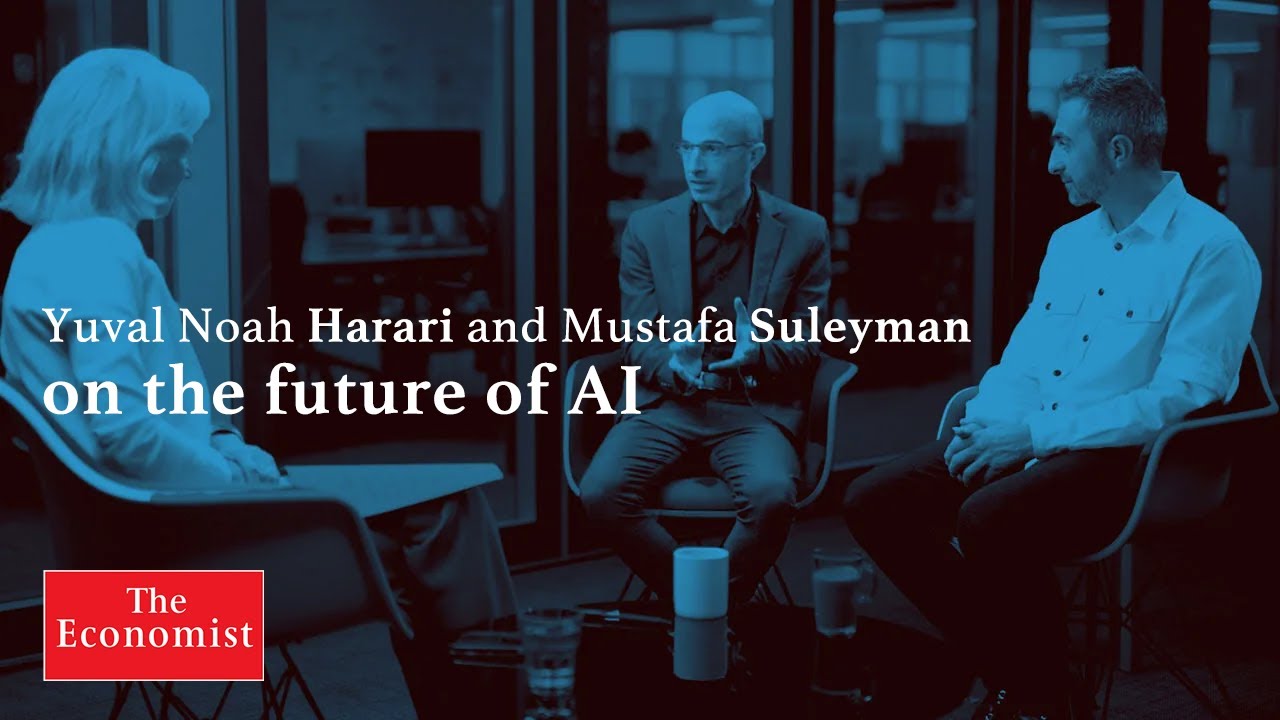
Mustafa Suleyman & Yuval Noah Harari -FULL DEBATE- What does the AI revolution mean for our future?
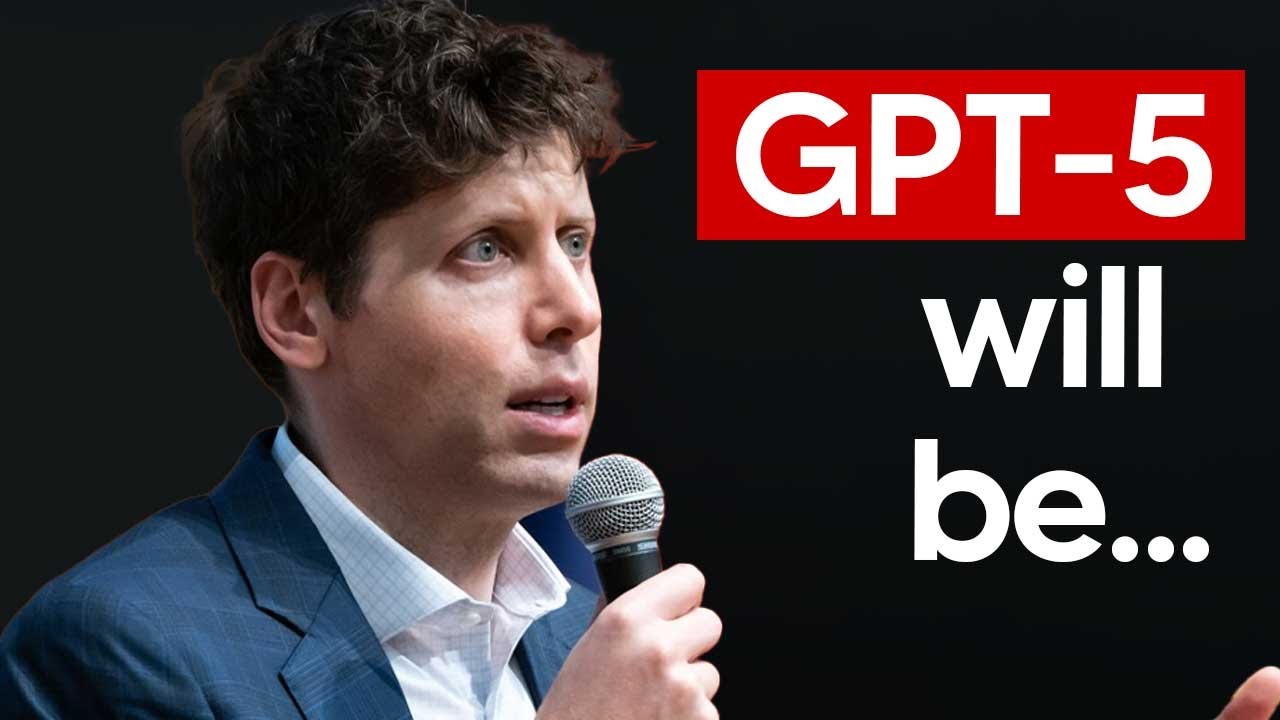
The AI Hype is OVER! Have LLMs Peaked?

How Will We Know When AI is Conscious?
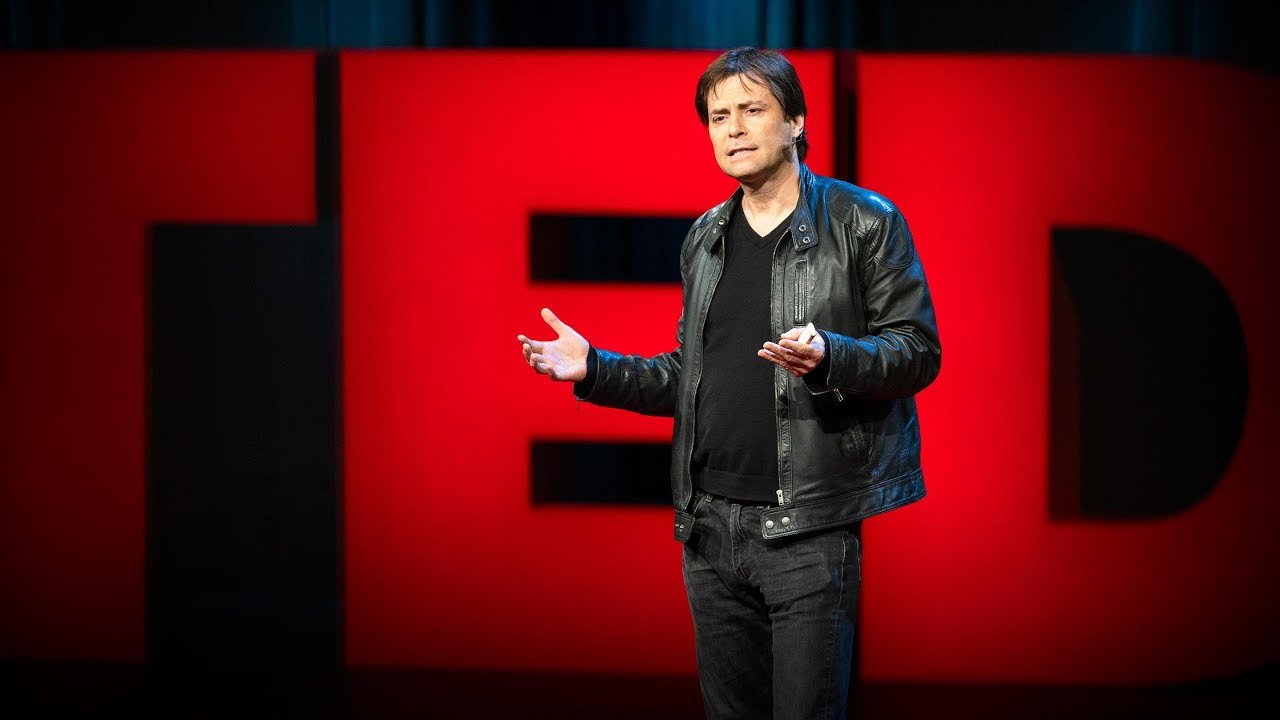
How to get empowered, not overpowered, by AI | Max Tegmark
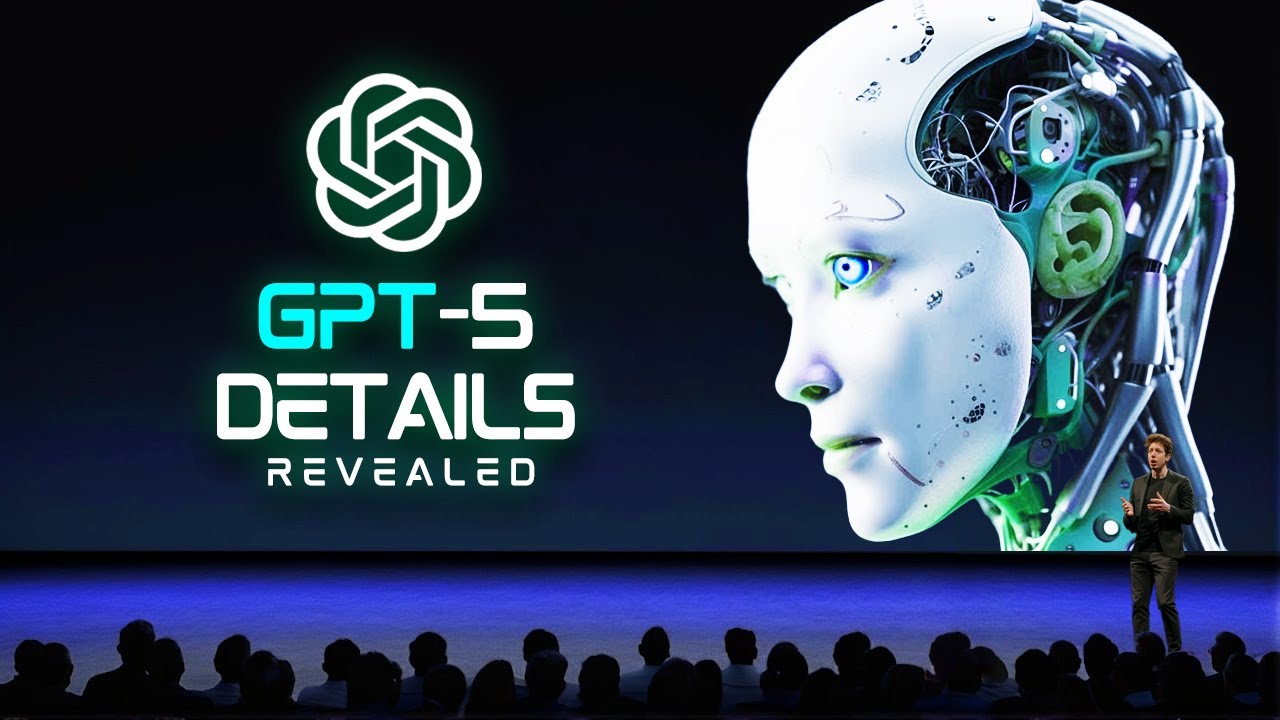
OpenAI Reveals New ChatGPT-5 Details
5.0 / 5 (0 votes)