OpenAI Insider Talks About the Future of AGI + Scaling Laws of Neural Nets
Summary
TLDRこの動画は、人工知能(AI)の発展と、将来的に人工汎用知能(AGI)に到達する可能性について議論しています。特に、ニューラルネットワークのパラメーター数が人間の脳と同等になった時に、AGIが実現する可能性があることを示唆しています。また、OpenAIの研究員であるScott Aronsonが、AGIの安全性と制御の問題に取り組んでいることにも言及しています。さらに、AGIの実現が人類に与える影響や倫理的課題についても触れられています。
Takeaways
- 🧠 人間の脳には約1000兆のパラメーター(シナプス)があり、AIモデルの能力は一般にパラメーター数に比例する。
- 📈 GPT-3は1750億のパラメーターを持ち、猫の脳に匹敵する規模。完全なAGI(人工般人工知能)を実現するには、人間の脳と同等の1000兆パラメーターが必要とされている。
- ⚡ AIの進化は主にデータ量とコンピューティングパワーの増加によって促進されてきた。AGIの基本的な理論は1950年代から存在していた。
- 🔮 一部の専門家は、AGIを実現するための技術的詳細はすでに解決済みで、あとはハードウェアの進化を待つだけだと主張している。
- 🤖 遠隔労働者が行える作業はほとんどすべてAGIが代替可能になると予測されている。
- 🕵️♂️ スコット・アロンソン氏は量子コンピューター研究者からOpenAIのAI安全性・整合性の研究に携わるようになった。
- 🤔 GPT-4やOpenAIの最新モデルが1000兆パラメーターに達したという噂は、作者自身があまり信用していないようだ。
- 📝 スコット・アロンソンの研究「線形光学の計算複雑性」は、量子コンピューターが古典コンピューターで効率的にシミュレートできないことを示唆している。
- 🔑 AGIを実現する上で重要なポイントは10個程度で、それらはこれまでの様々な研究テキストの中に隠されている可能性がある。
- ✍️ 作者は今回の内容をやや散漫で分かりづらいと認めているが、AGIの話題はますます興味深くなってきている。
Q & A
人工知能はいつ人間レベルの能力に到達すると考えられていますか?
-この記事によると、人工知能のパラメーター数が人間の脳のシナプス数に匹敵する約2*10^14個になると、人間レベルの能力に達すると予測されています。ただし、この予測には幅があり、最低でGPT-3レベル、最高で現在の1万倍のパラメーター数が必要となる可能性があると述べられています。
GPT-3とはどのようなモデルですか?
-GPT-3は2020年にリリースされた大規模な言語モデルで、1750億個のパラメーターを持ち、ある程度の推論や文章生成が可能でした。この能力には多くの人が驚きを示しましたが、人間レベルの知能とはまだ程遠い段階でした。
ScottAronsonはどのような人物ですか?
-ScottAronsonは量子コンピューターの研究者で、2022年半ばにOpenAIの人工知能の安全性と整合性に関する部門に入社しました。彼は人工知能の危険性について警鐘を鳴らす一方で、その進歩を阻止すべきではないと主張しています。
パラメーター数が多ければ多いほど、人工知能の性能は向上するのでしょうか?
-はい、おおむねその傾向があると考えられています。パラメーター数が多ければ、ニューラルネットワークに組み込めるデータの量が増え、より複雑なパターンを認識できるようになります。しかし、単にパラメーター数を増やすだけでは不十分で、適切な学習データとアルゴリズムも重要になります。
人工知能がAGI(汎用人工知能)に到達するための技術的詳細は、すでに解決されていると考えられていますか?
-はい、John Carmackら一部の専門家は、AGIを実現するための技術的詳細は過去数十年の研究で概ね解決されていると主張しています。彫琢が必要なのは、計算能力とデータの確保だけだと述べられています。ただし、この見解には異論もあります。
ニューラルネットワークにおけるパラメーターとは具体的に何を指しますか?
-ニューラルネットワークにおけるパラメーターとは、ノード間の結合の重みや、各ノードへの入力に対する閾値を表すバイアス値のことを指します。これらのパラメーターを調整することで、ネットワークの出力を最適化できます。生物の脳のシナプス結合に相当します。
AA (Anthropic AI)のTIIモデルとは何ですか?
-TIIは、Anthropic AIによって開発されているAGIモデルの名称です。このモデルは、リモートワーカーが行える作業のほとんど全てを人間レベルで実行できることを目標としています。パラメーター数の予測値も示されています。
AIの性能予測において、パラメーター数以外に重要な要素はありますか?
-パラメーター数だけでなく、学習データの質や量、使用されるアルゴリズム、モデルアーキテクチャなども、AIの性能に大きな影響を与えます。単にパラメーターを増やすだけでは、必ずしも性能が向上するわけではありません。適切な設計と学習が重要です。
AGI実現の鍵は、新しい技術ブレークスルーにあるのでしょうか?
-この記事では、AGIを実現するための重要な技術的詳細はすでに過去の研究で明らかになっている可能性があると示唆されています。新しいブレークスルーよりも、既存の知識を組み合わせて適用することが鍵となるかもしれません。
この動画の主な主張は何ですか?
-この動画の主な主張は、人工知能の進化はパラメーター数の増加と適切なデータの組み合わせによって加速する可能性があり、AGIに到達するための技術的詳細はすでに存在している可能性があるということです。ただし、具体的な時期予測には不確定要素が多いことも示唆されています。
Outlines
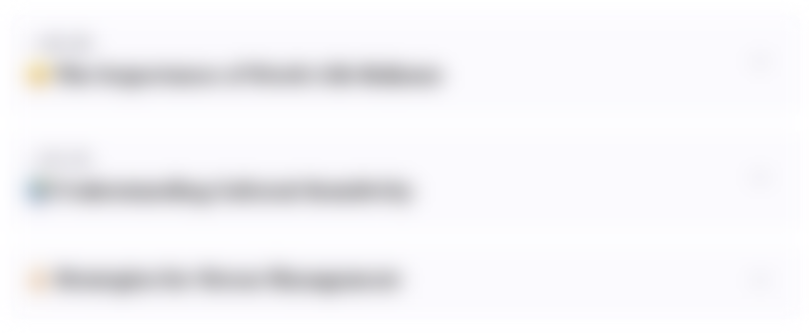
This section is available to paid users only. Please upgrade to access this part.
Upgrade NowMindmap
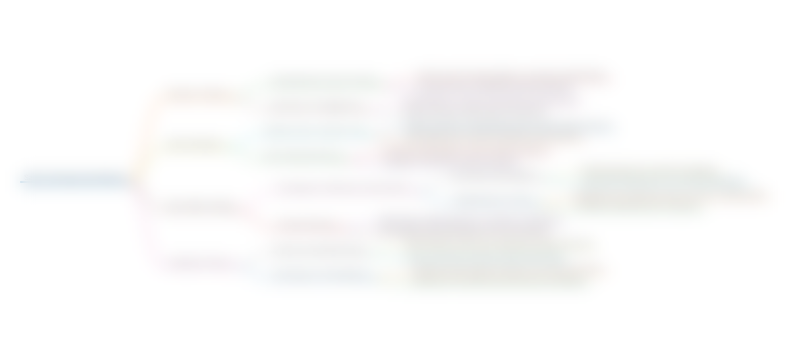
This section is available to paid users only. Please upgrade to access this part.
Upgrade NowKeywords
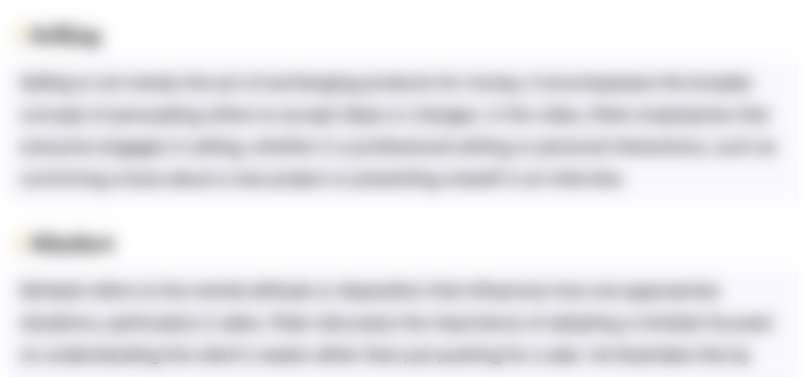
This section is available to paid users only. Please upgrade to access this part.
Upgrade NowHighlights
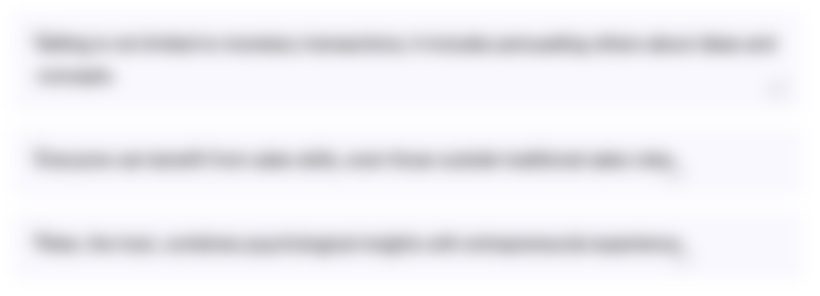
This section is available to paid users only. Please upgrade to access this part.
Upgrade NowTranscripts
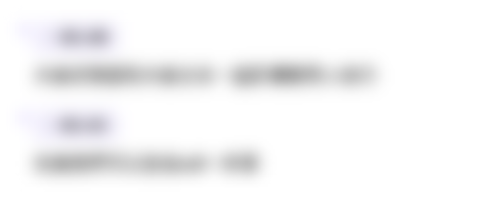
This section is available to paid users only. Please upgrade to access this part.
Upgrade NowBrowse More Related Video
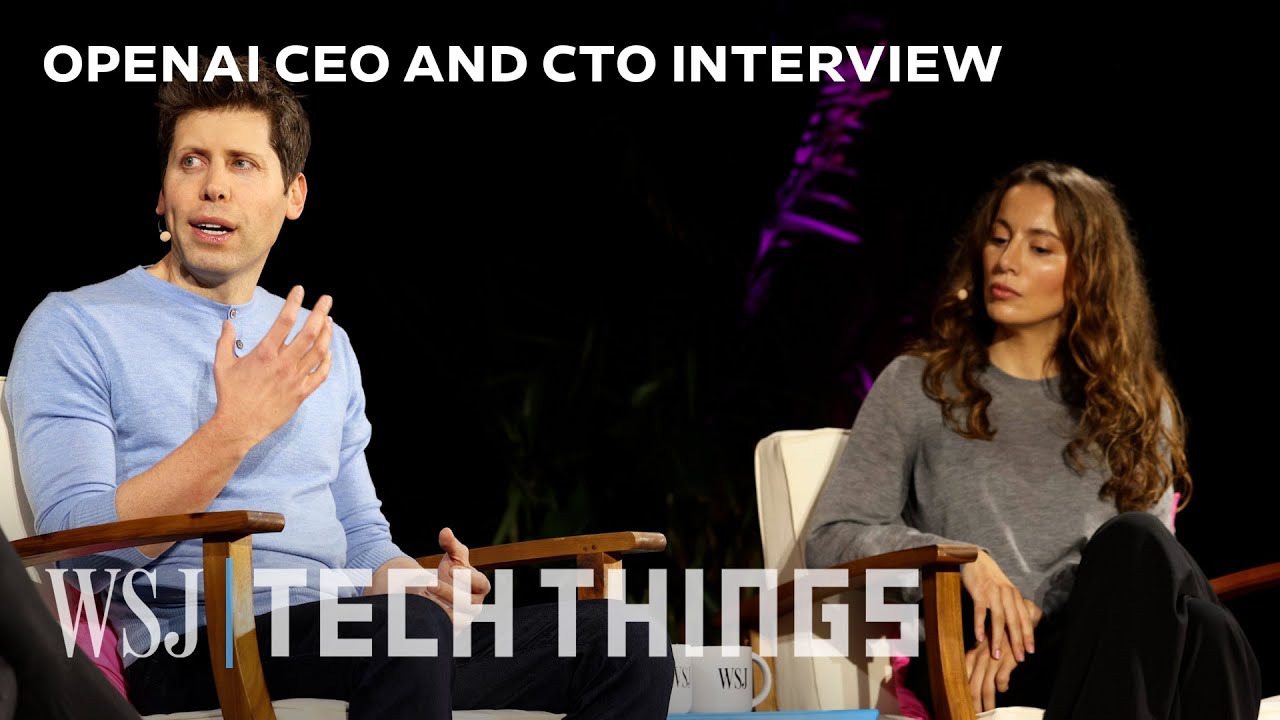
OpenAI CEO Sam Altman and CTO Mira Murati on the Future of AI and ChatGPT | WSJ Tech Live 2023
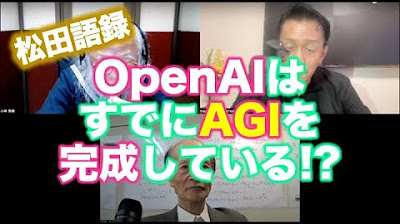
松田語録:OpenAIはすでにAGIを完成している!?
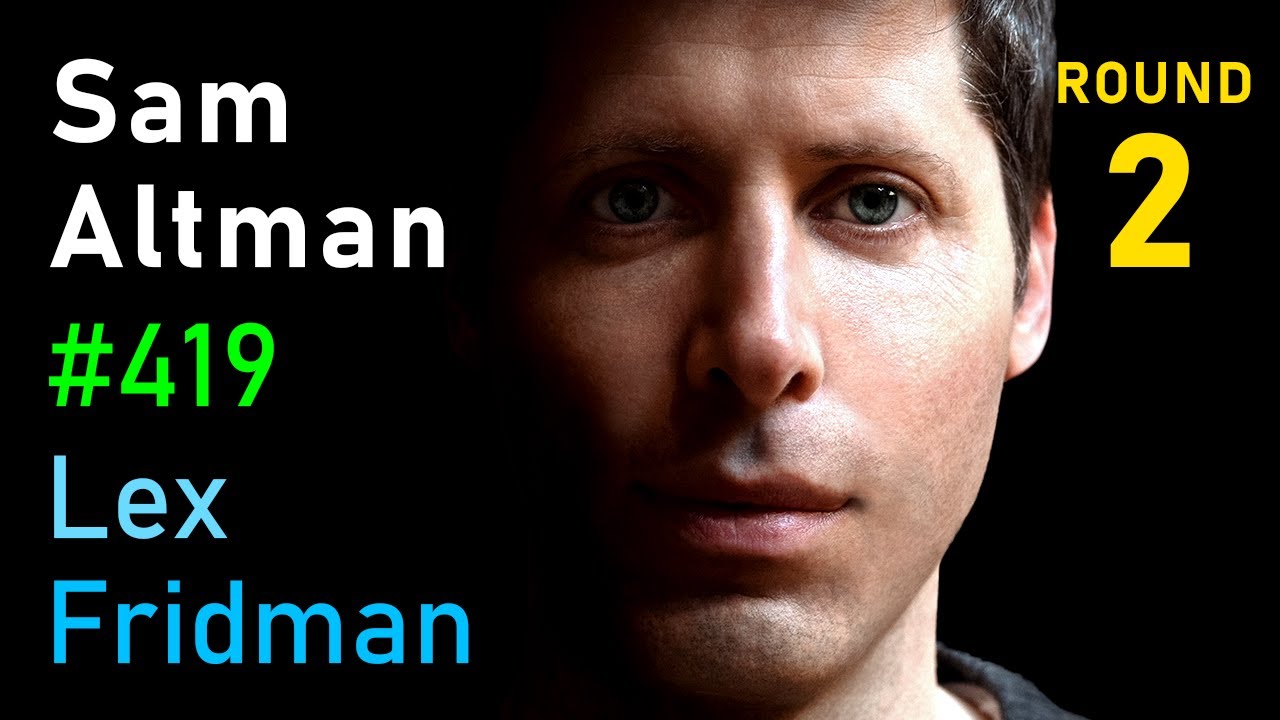
Sam Altman: OpenAI, GPT-5, Sora, Board Saga, Elon Musk, Ilya, Power & AGI | Lex Fridman Podcast #419

シンギュラリティの世界|「AI」は神になれるのか?それとも、、【未来予測 2045】人工知能

松田語録:イリア・サツケバーがOpenAIを退社
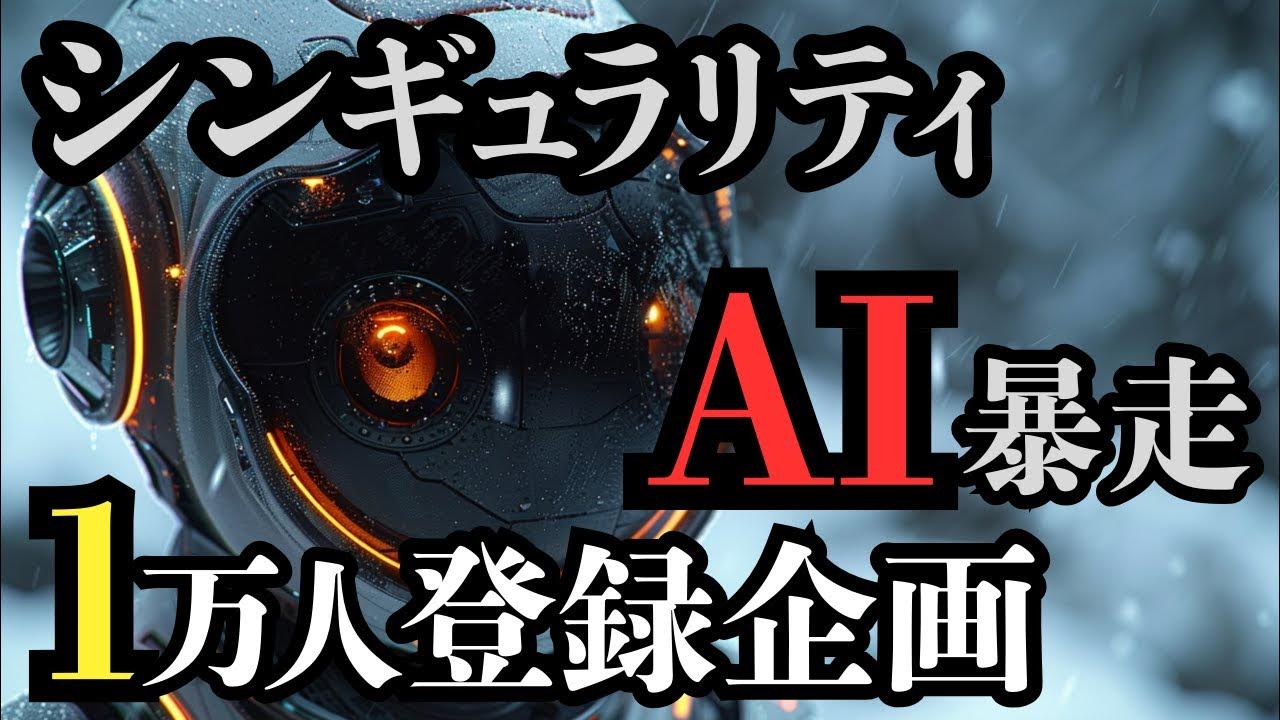
シンギュラリティ×暴走×完全なる仮想世界|希少種となるオリジナル、そして、、【未来予測 2045】人工知能 AI AGI
5.0 / 5 (0 votes)