Quantitative Data Analysis 101 Tutorial: Descriptive vs Inferential Statistics (With Examples)
Summary
TLDRThis video from Grad Coach TV demystifies quantitative data analysis, explaining its definition, the distinction between descriptive and inferential statistics, and their applications. It covers essential concepts like mean, median, mode, standard deviation, and skewness, and delves into choosing the right statistical methods based on data type and research questions. Aiming to build confidence in approaching research, the video offers practical advice for students navigating the complex world of quantitative analysis.
Takeaways
- 📊 Quantitative data analysis involves examining numerical data and can be applied to both categorical and numerical data types.
- 🔍 The process is divided into two main branches: descriptive statistics, which describe the sample, and inferential statistics, which make predictions about the population.
- 📈 Descriptive statistics include measures like mean, median, mode, standard deviation, and skewness, offering a detailed view of the sample data.
- 🔮 Inferential statistics are used for hypothesis testing and making predictions about population parameters based on sample data.
- 🧐 Understanding the difference between population and sample is crucial for choosing the right statistical methods and drawing valid conclusions.
- 📐 Descriptive statistics are essential for getting a macro and micro view of the data, spotting potential errors, and informing the choice of inferential methods.
- 🚫 It's important not to rush through descriptive statistics to get to inferential methods, as this can lead to flawed results.
- 📝 When selecting statistical methods, consider the level of measurement and the shape of the data (e.g., normal distribution vs. skewed).
- 🎯 Align the choice of statistical methods with the research questions and hypotheses to ensure the analysis is relevant and meaningful.
- 🔄 Descriptive statistics should be the first step in any analysis, followed by inferential statistics if the research aims to make predictions about the population.
- 🌐 For further exploration of statistical methods, the Grad Coach blog and other resources are recommended for a comprehensive understanding.
Q & A
What is the main focus of the video?
-The video focuses on explaining the concept of quantitative data analysis, its methods, and how to choose the right methods for research.
What are the two main branches of quantitative analysis discussed in the video?
-The two main branches of quantitative analysis discussed are descriptive statistics and inferential statistics.
What is the purpose of descriptive statistics in research?
-Descriptive statistics serve to describe the data set, helping researchers understand the details of their sample.
What is the purpose of inferential statistics in research?
-Inferential statistics aim to make inferences about the population based on the findings within the sample.
What are some common descriptive statistical metrics mentioned in the video?
-Common descriptive statistical metrics mentioned include the mean, median, mode, standard deviation, and skewness.
What are some common inferential statistical methods discussed in the video?
-Some common inferential statistical methods discussed are t-tests, ANOVA, correlation analysis, and regression analysis.
How does the video suggest choosing the right statistical methods for research?
-The video suggests considering the type of data collected, the level of measurement, the shape of the data, and the specific research questions and hypotheses.
Why is it important to understand the shape of the data when choosing statistical methods?
-Understanding the shape of the data is important because different statistical methods work for different shapes of data, such as symmetrical or skewed distributions.
What is the role of the mean in descriptive statistics?
-The mean serves as the mathematical average of a range of numbers, providing a central value for the data set.
What does the median represent in a data set?
-The median represents the midpoint in a range of numbers when they are arranged in order, indicating the center of the data set.
What is the significance of standard deviation in understanding a data set?
-Standard deviation indicates how dispersed a range of numbers is, showing how close all the numbers are to the mean, thus providing insight into the spread of the data.
Outlines
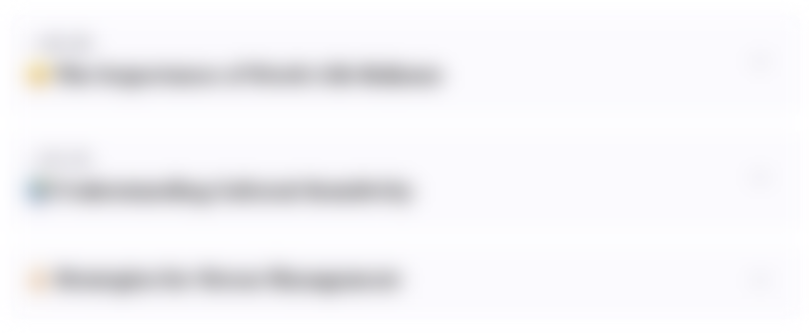
This section is available to paid users only. Please upgrade to access this part.
Upgrade NowMindmap
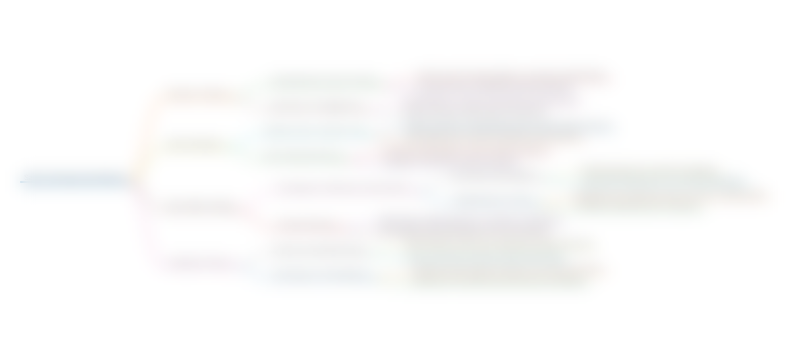
This section is available to paid users only. Please upgrade to access this part.
Upgrade NowKeywords
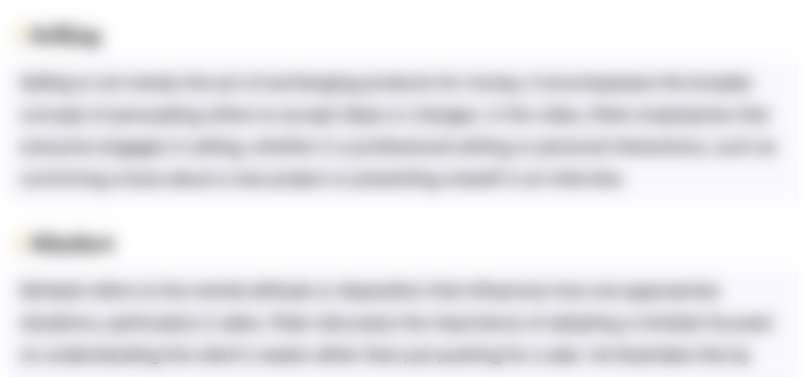
This section is available to paid users only. Please upgrade to access this part.
Upgrade NowHighlights
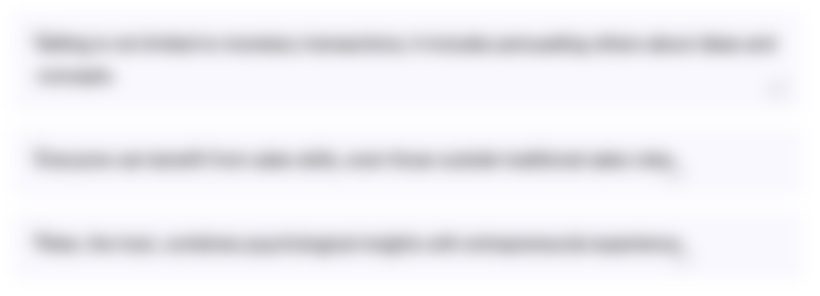
This section is available to paid users only. Please upgrade to access this part.
Upgrade NowTranscripts
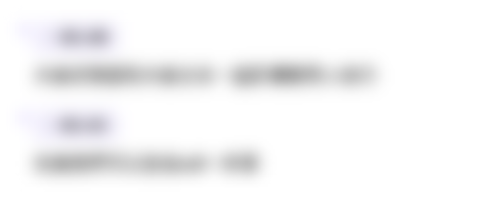
This section is available to paid users only. Please upgrade to access this part.
Upgrade NowBrowse More Related Video
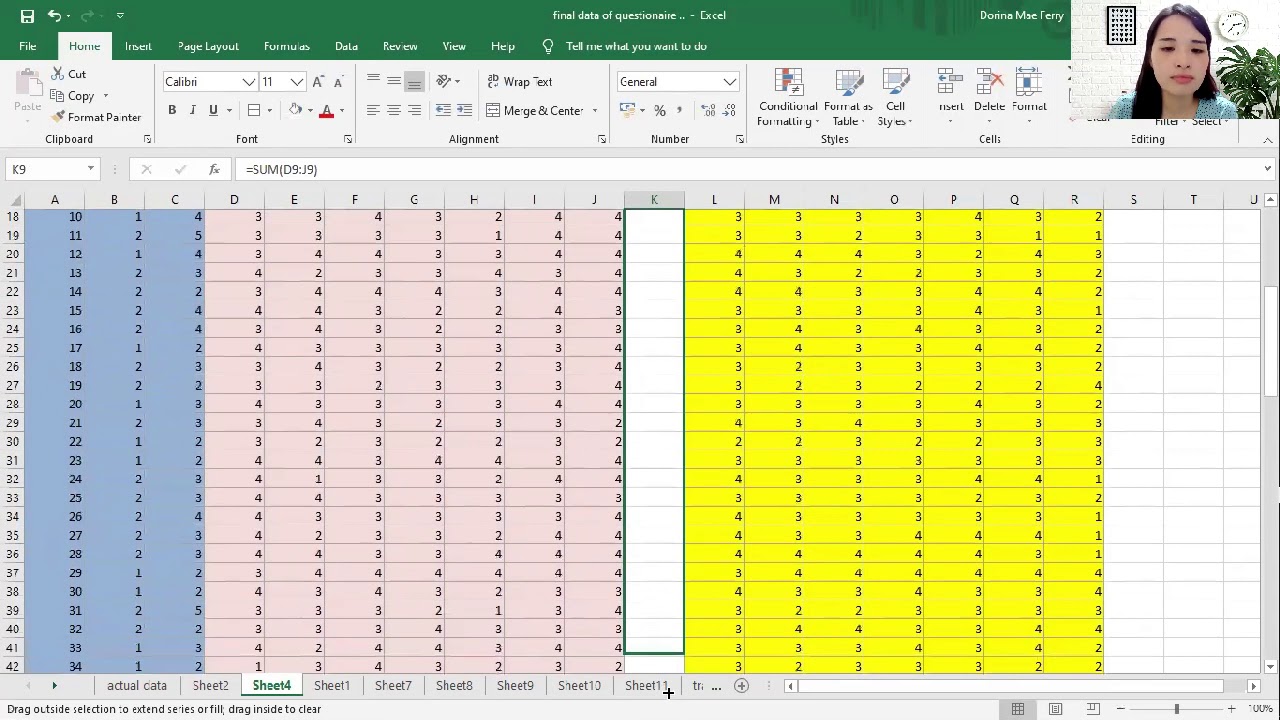
How to Tally, Encode, and Analyze your Data using Microsoft Excel (Chapter 4: Quantitative Research)
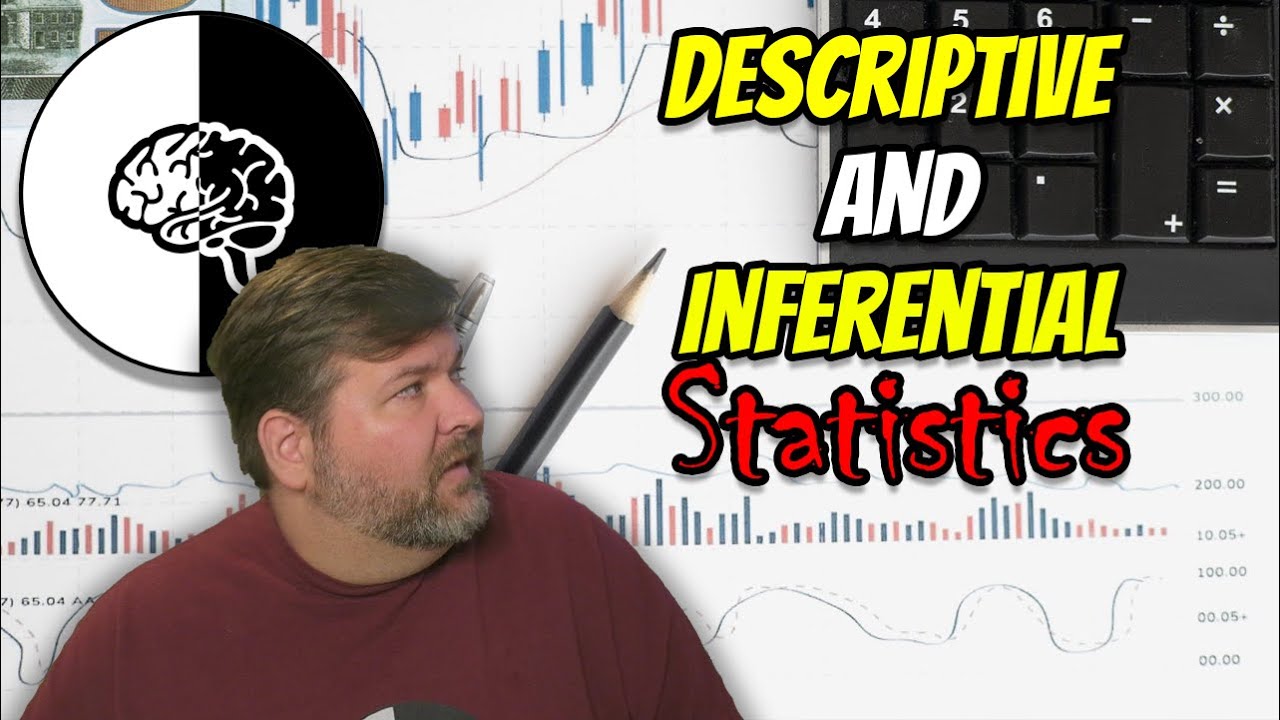
What is the difference between Descriptive Statistics and Inferential Statistics?
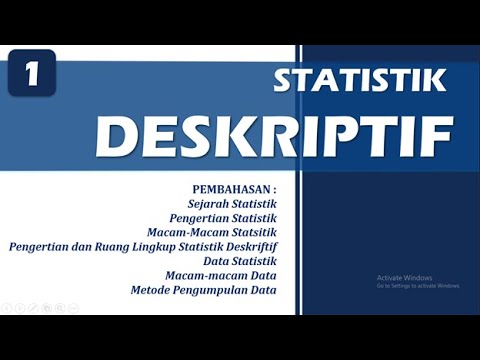
SEJARAH DAN PENGERTIAN STATISTIK - STATISTIK DESKRIPTIF | BAB 1
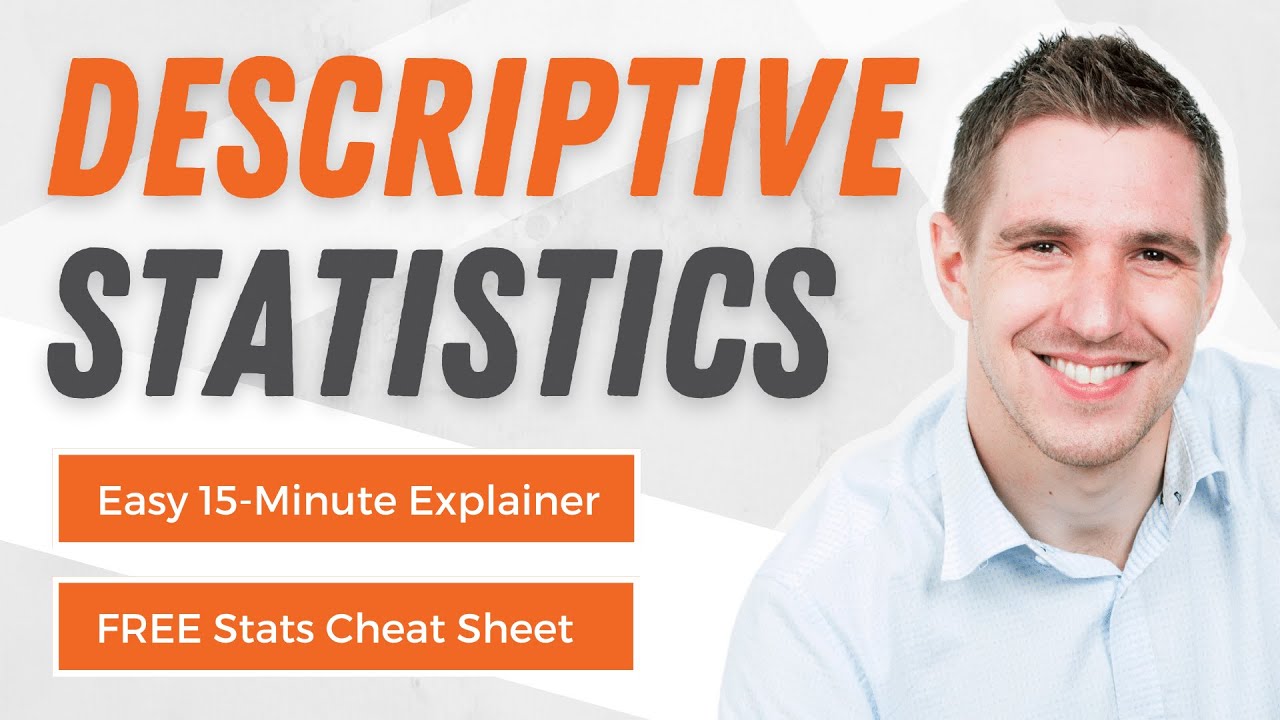
Descriptive Statistics: FULL Tutorial - Mean, Median, Mode, Variance & SD (With Examples)
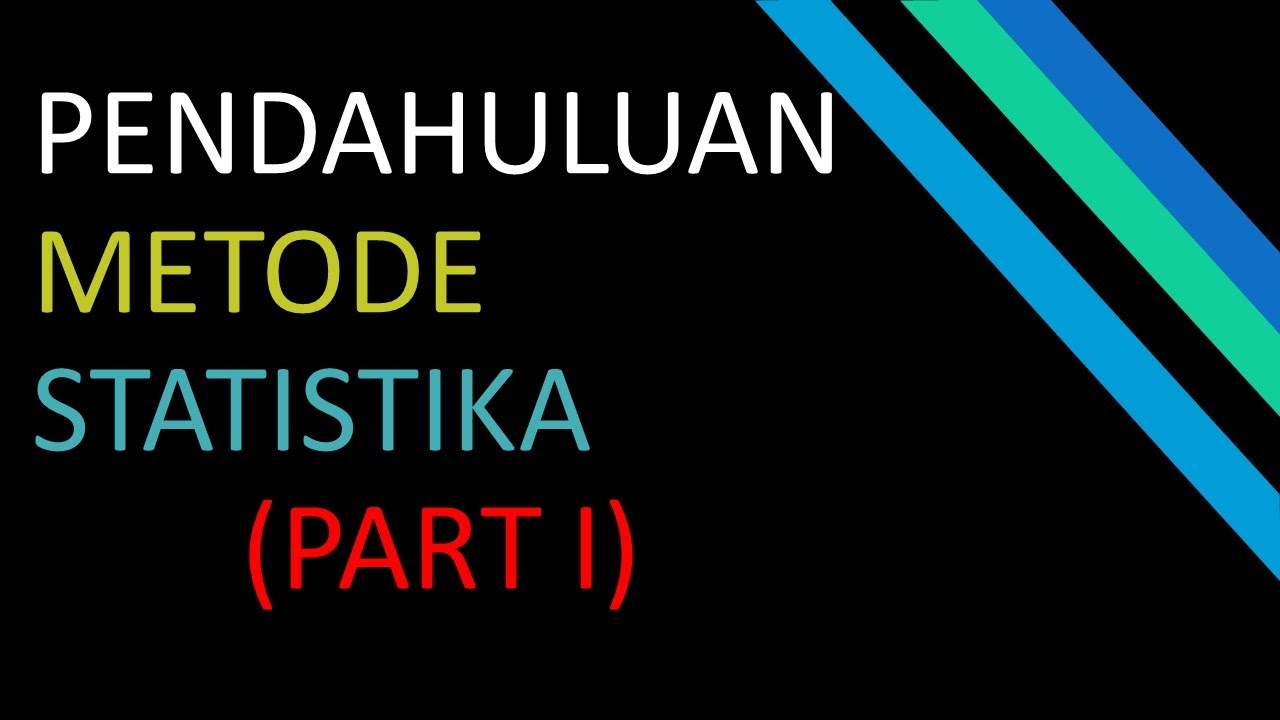
Pendahuluan Metode Penelitian Part I
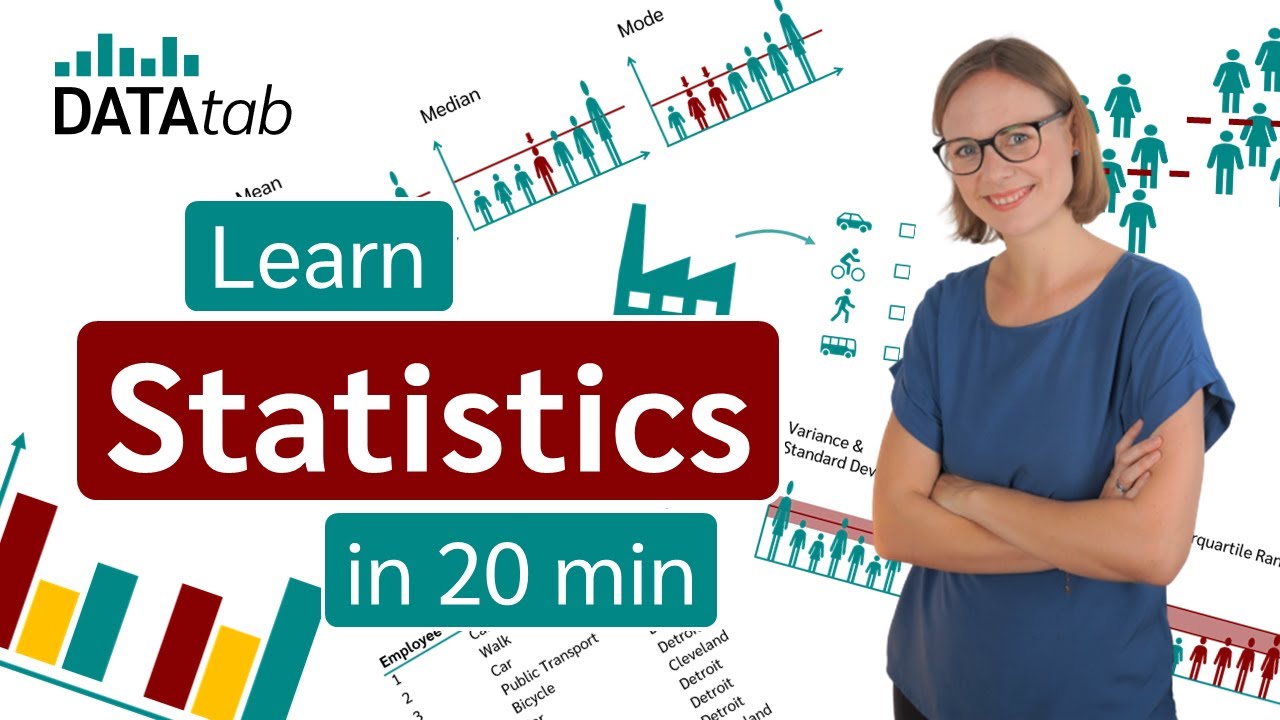
What is Statistics? A Beginner's Guide to Statistics (Data Analytics)!
5.0 / 5 (0 votes)