How to Combine Internal & External Training Load Monitoring
Summary
TLDRThe video discusses approaches to combine internal and external training load metrics to gain insights into player fitness and fatigue without additional testing. It covers using simple ratios of the loads and more complex regression models to predict expected internal load based on external load. The video highlights research on training efficiency indexes that scale the loads for comparison. It advocates for individualized analysis, noting machine learning techniques that find different external load metrics most predictive of internal load by player. A case study shows patterns of higher actual than predicted internal load, indicating potential deconditioning to flag for intervention.
Takeaways
- 😀 Combining internal and external training loads may provide insights into fitness and fatigue
- 📊 A simple approach is to calculate the ratio between internal and external loads
- 🔬 Ratios may correlate with fitness measures but become weaker under fatigue
- 🧪 Uncoupling of loads could indicate fatigue without direct testing
- ⚖️ Issues can arise from numbers being on different scales, the training efficiency index aims to address this
- 📈 Training efficiency index plots loads on a logarithmic scale to enable comparison
- 😕 Ratios may oversimplify the complexity of training sessions
- 🤖 Machine learning is being used to relate loads on an individualized basis
- 😃 Case study: Compared actual and predicted internal load based on external load
- 💡 Flagged potential issues through this "invisible monitoring" approach
Q & A
What are internal and external training loads?
-Internal training load refers to the physiological stress imposed on an athlete, often measured by heart rate. External training load refers to the work completed by the athlete, often measured by metrics like distance covered or accelerometry.
Why might combining internal and external loads be useful?
-Combining internal and external loads may provide more insight into an athlete's fitness and fatigue status. This 'invisible monitoring' allows coaches to assess fatigue without extensive additional testing.
What was the first study to investigate ratios of internal to external loads?
-A study by Ebi Acuba and his team first looked at ratios of individualized training impulse (iTRIM) to external load metrics like total distance. They found these ratios correlated with measures of fitness in soccer players.
What did Acuba's next study on internal/external load ratios find regarding fatigue?
-In their next study, Acuba's team found that the relationships between internal/external load ratios and measures of fitness became weaker under fatigue. This suggests uncoupling of loads could indicate fatigue.
What issue needs to be considered when creating ratios of internal to external loads?
-Internal and external loads use different scales - heart rate may be around 300, while distance could be thousands of meters. The numbers need to be scaled before taking a simple ratio.
How does the training efficiency index address the scaling issue?
-The training efficiency index plots internal and external loads on a logarithmic scale before taking the ratio. This scaling allows the comparison of different load metrics.
What does the case study example demonstrate regarding internal/external load analysis?
-The case study shows how regression equations can forecast athlete's internal load based on external load. Comparing predicted and actual internal load over time can indicate changes in fitness and fatigue.
What machine learning approach shows promise for relating internal and external loads?
-A machine learning model developed by John Bartlett demonstrated the need to relate loads on an individualized, athlete-by-athlete basis, with different metrics being most predictive for each athlete.
What is the overall message regarding approaches that combine internal and external training loads?
-There is no clear consensus, but analysis should be done on an individualized basis for each athlete to relate the right external metrics to internal load response.
What is the topic of the next video mentioned?
-The next video will continue the discussion of invisible monitoring - using existing data to monitor fatigue without additional testing.
Outlines
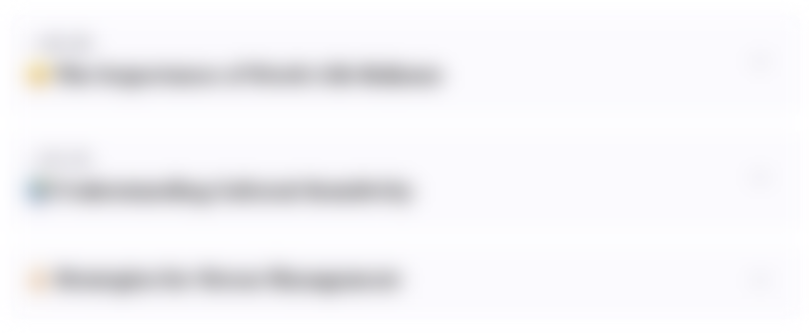
This section is available to paid users only. Please upgrade to access this part.
Upgrade NowMindmap
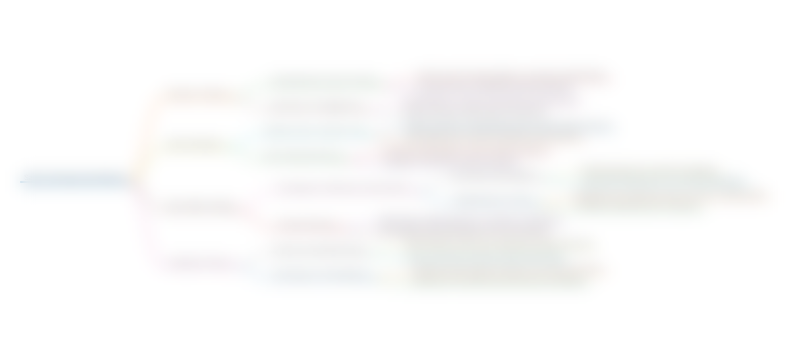
This section is available to paid users only. Please upgrade to access this part.
Upgrade NowKeywords
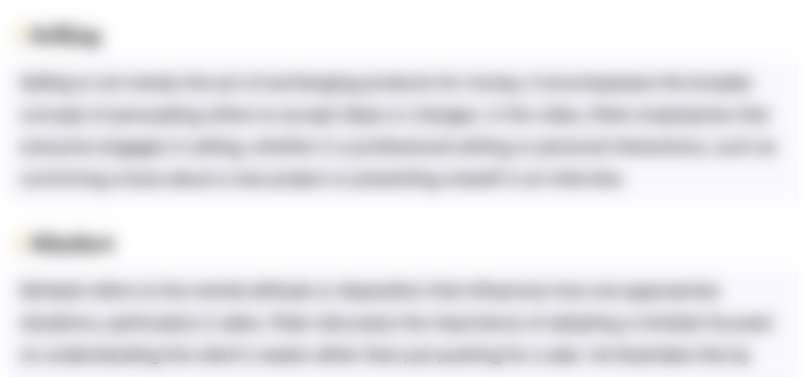
This section is available to paid users only. Please upgrade to access this part.
Upgrade NowHighlights
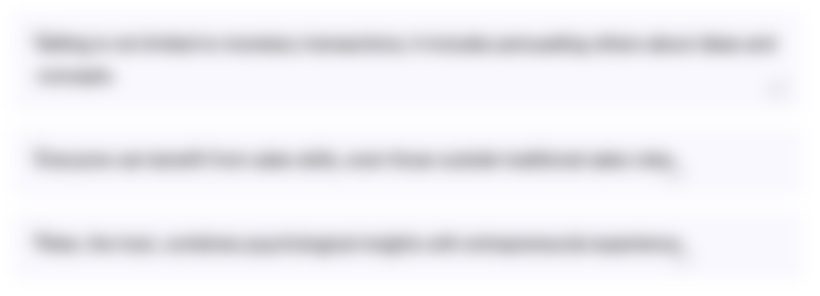
This section is available to paid users only. Please upgrade to access this part.
Upgrade NowTranscripts
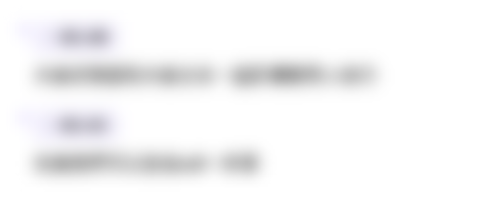
This section is available to paid users only. Please upgrade to access this part.
Upgrade NowBrowse More Related Video
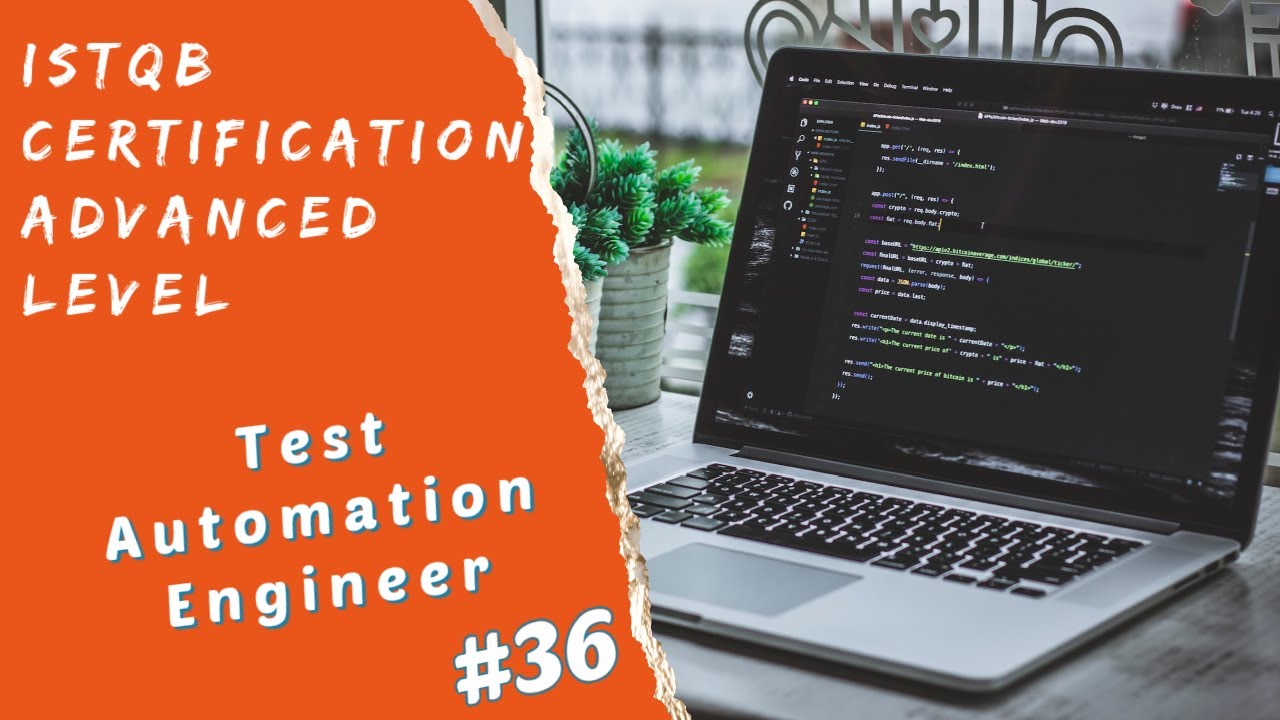
ISTQB CTAL TAE Session 36 - 5.1 - Selection of TAS Metrics
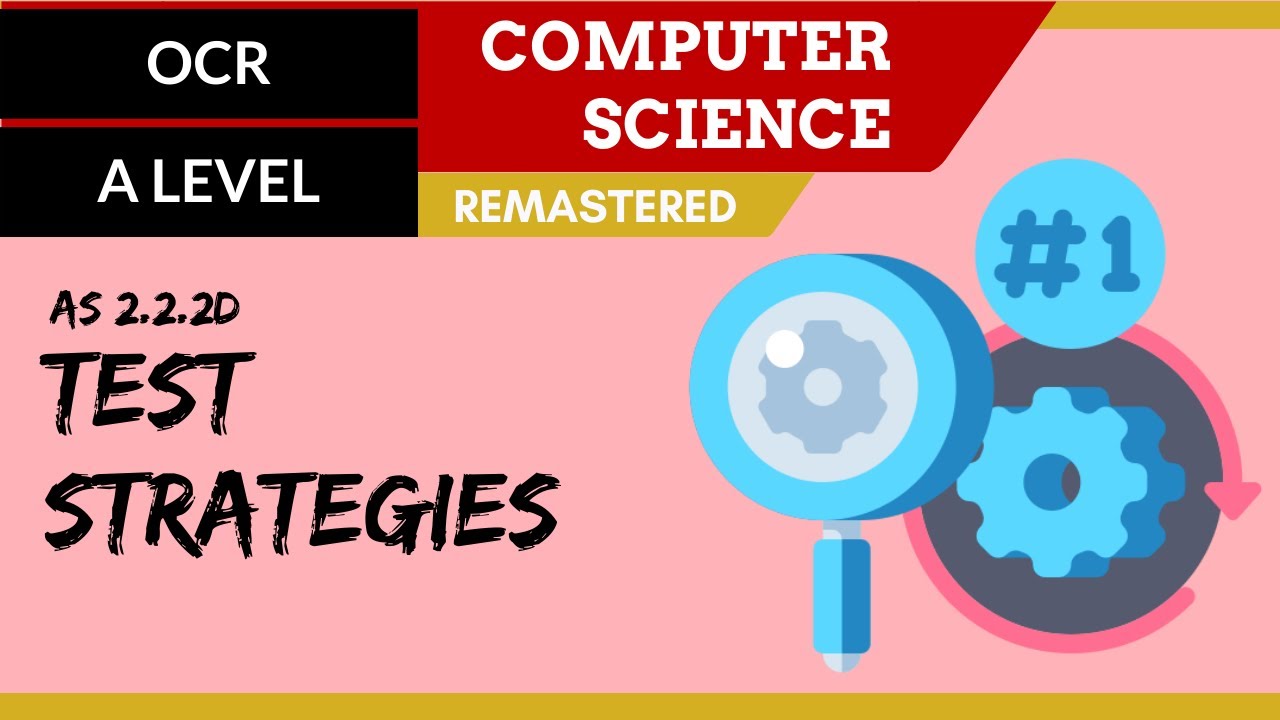
30. OCR A Level (H046) SLR6 - 2.2 Test strategies

My Hybrid Training Routine to Lose Fat and Gain Muscle

I Hate Cycling For This, But Cyclists Should Try It
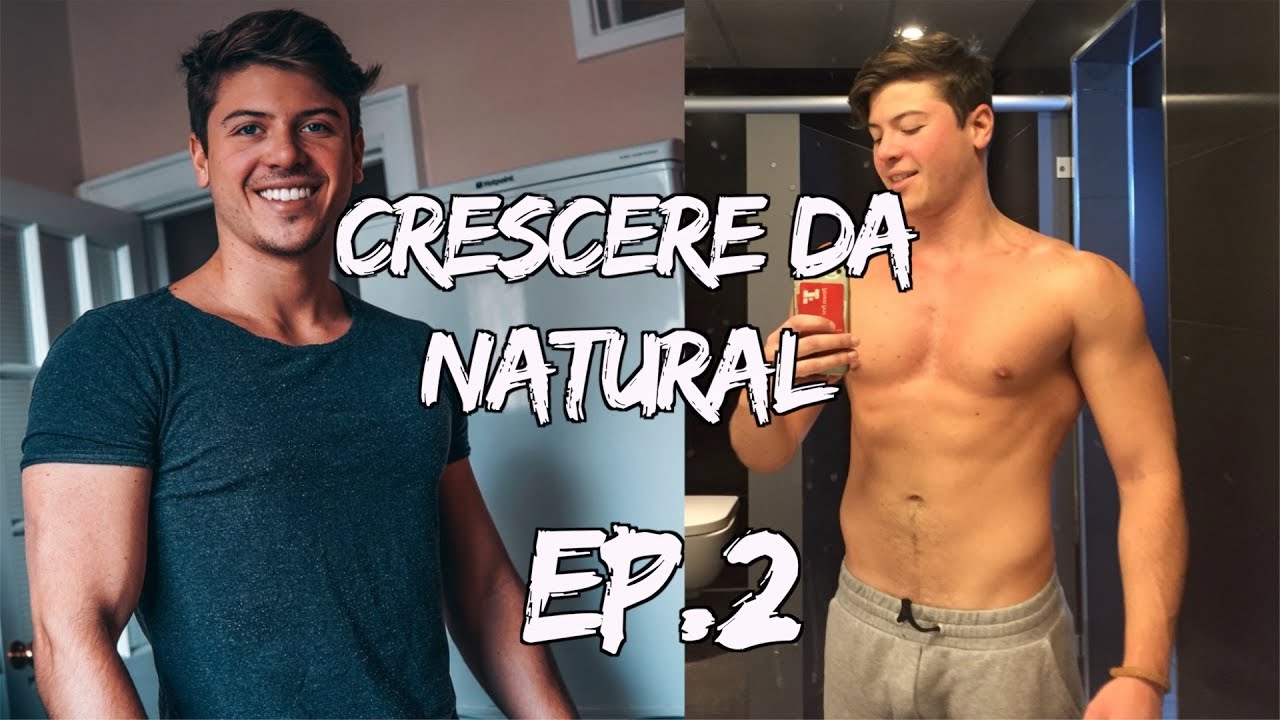
Volume Ottimale Per CRESCERE (Braccia, Petto, Spalle, Schiena) *RICERCA SCIENTIFICA* | CDN EP.2
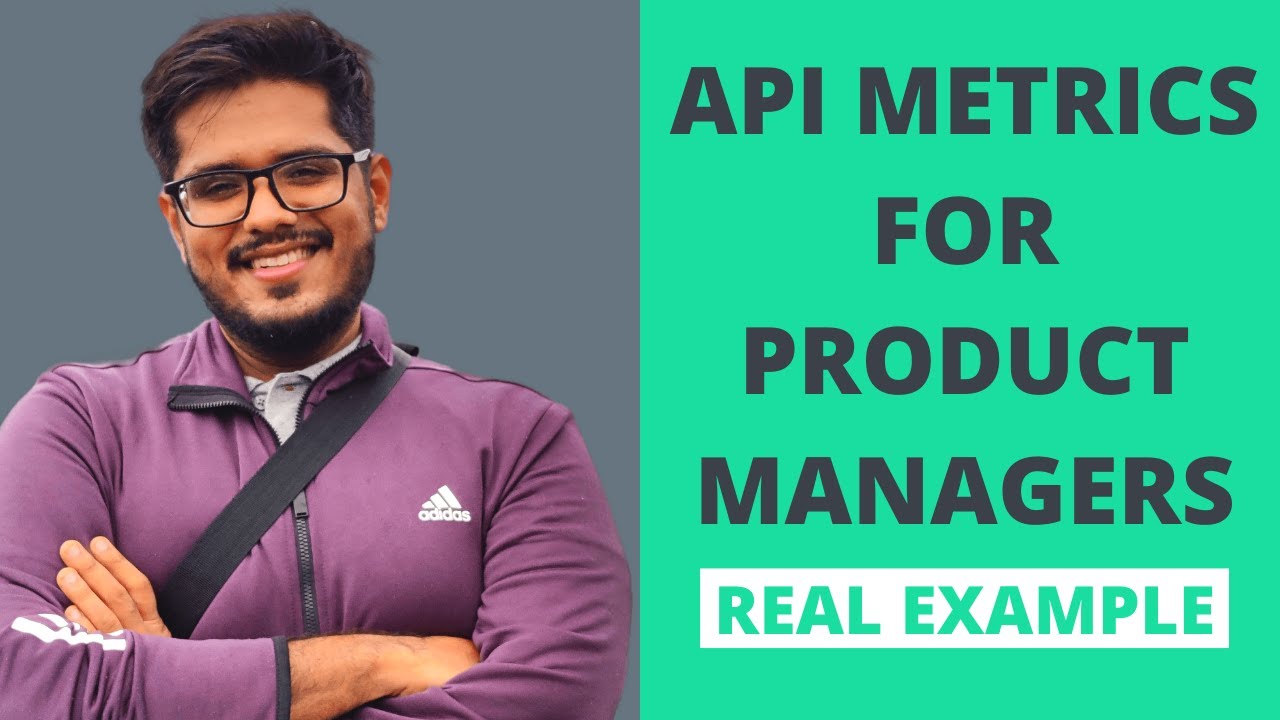
API Metrics for Product Managers | API Product Management
5.0 / 5 (0 votes)