【潜在変数の関係を探る】構造方程式モデリング - 実応用の豊富な基礎分析なのです【いろんな分析 vol. 6 】 #063 #VRアカデミア
Summary
Please replace the link and try again.
Please replace the link and try again.
Q & A
What is Structural Equation Modeling (SEM)?
-Structural Equation Modeling (SEM) is an advanced statistical technique that is used to model complex relationships between variables. It incorporates both observed and latent variables and helps in understanding the underlying structure of data.
How did SEM evolve from factor analysis?
-SEM evolved from factor analysis by allowing researchers to model complex relationships between multiple factors. Unlike factor analysis, which assumes independence between factors, SEM can model correlations between these factors, providing a more comprehensive understanding.
What are latent variables in SEM?
-Latent variables are unobserved constructs that influence the observed data. They cannot be directly measured but are inferred from other observable variables. Examples include traits like intelligence or socioeconomic status.
What role do observed variables play in SEM?
-Observed variables are directly measured variables, such as test scores or survey responses. These variables are influenced by latent factors in SEM and serve as the data points that the model analyzes.
What is a path diagram in SEM?
-A path diagram is a visual representation of the relationships between variables in SEM. It shows how observed and latent variables are related and includes arrows indicating causal or correlation relationships.
How does SEM model correlations between variables?
-SEM models correlations by depicting how different observed variables are influenced by common latent factors. This allows SEM to not only identify correlations but also to uncover the underlying causes of these relationships.
What is the significance of error terms in SEM equations?
-Error terms in SEM equations represent the variability in observed variables that is not explained by the latent factors. They capture the discrepancies between the observed data and the model's predictions.
How does SEM differ from traditional regression analysis?
-Unlike traditional regression analysis, which focuses on direct relationships between observed variables, SEM allows for the inclusion of both observed and latent variables. It also models the relationships between multiple variables simultaneously, including their correlations.
In which fields is SEM commonly used?
-SEM is widely used in fields like psychology, education, social sciences, and marketing. It is particularly useful in research that aims to understand complex relationships between multiple variables and test theoretical models.
Why is SEM considered a powerful tool for researchers?
-SEM is considered powerful because it allows researchers to test complex theoretical models, understand the relationships between variables, and make predictions about future data. Its ability to incorporate both observed and latent variables makes it particularly valuable for understanding deep, underlying structures in data.
Outlines
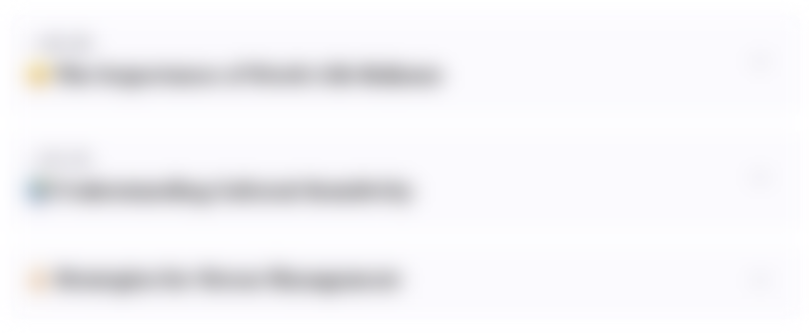
This section is available to paid users only. Please upgrade to access this part.
Upgrade NowMindmap
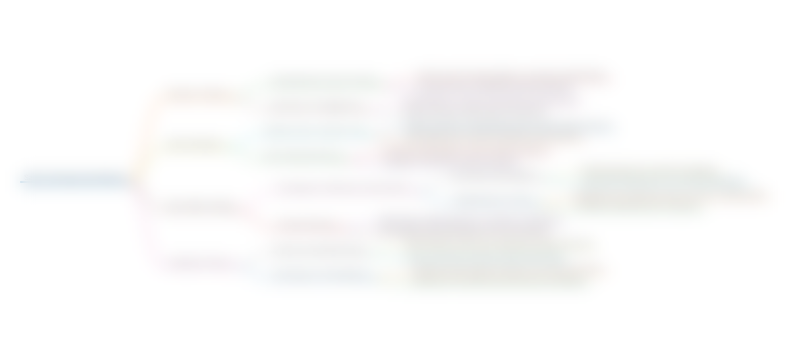
This section is available to paid users only. Please upgrade to access this part.
Upgrade NowKeywords
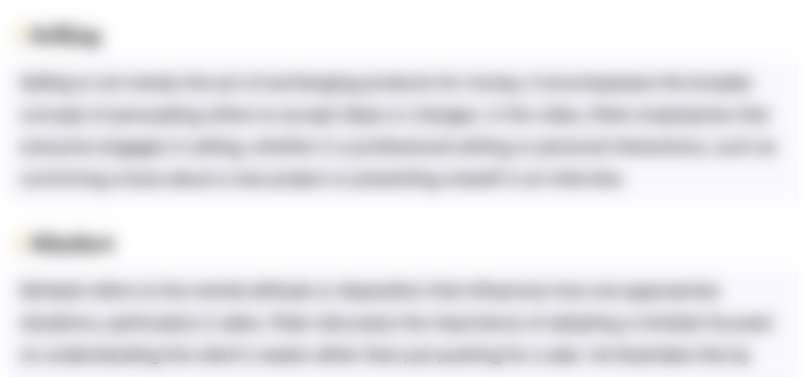
This section is available to paid users only. Please upgrade to access this part.
Upgrade NowHighlights
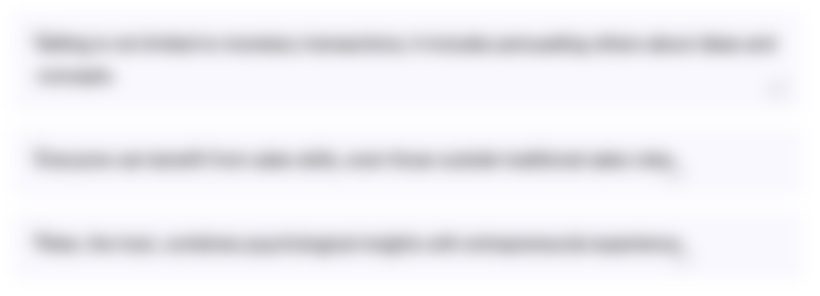
This section is available to paid users only. Please upgrade to access this part.
Upgrade NowTranscripts
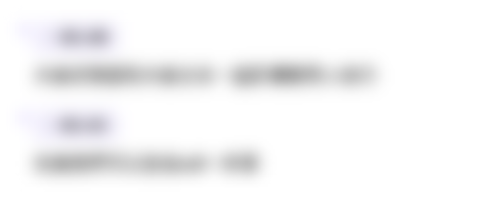
This section is available to paid users only. Please upgrade to access this part.
Upgrade NowBrowse More Related Video
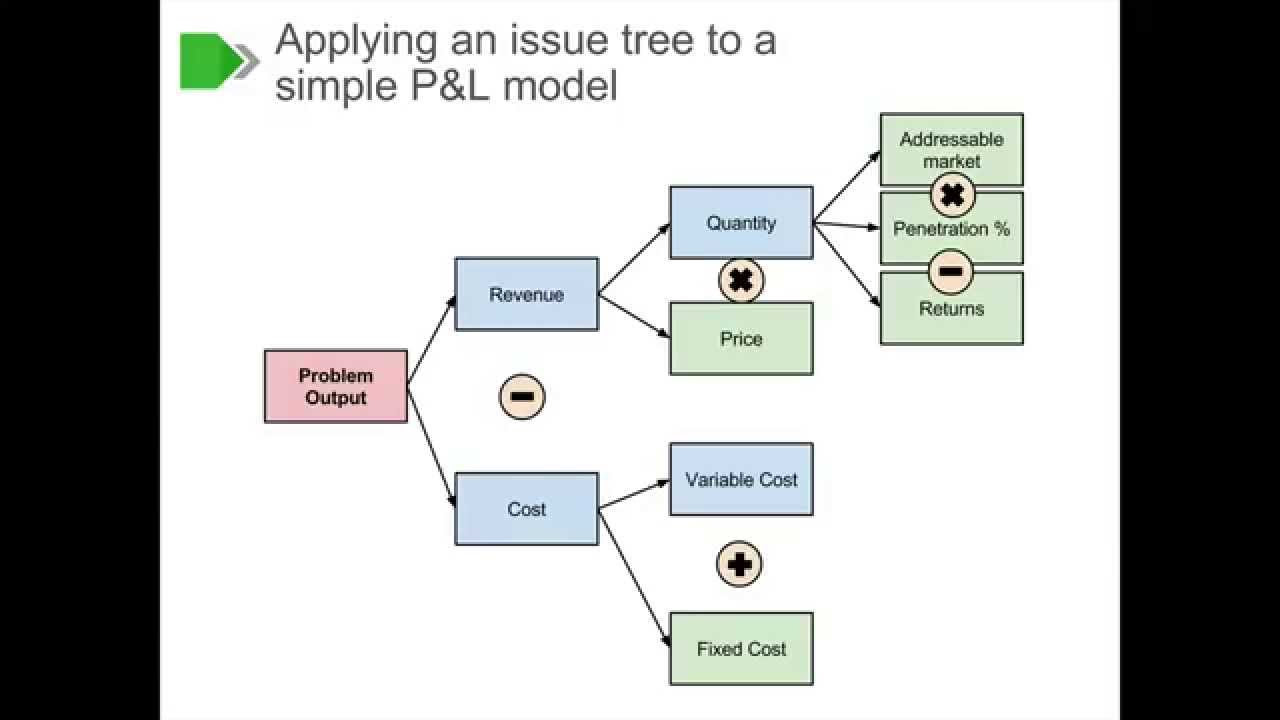
BeyondFormulas Modeling - 3. PROBLEM SOLVING - 5. Issue Trees
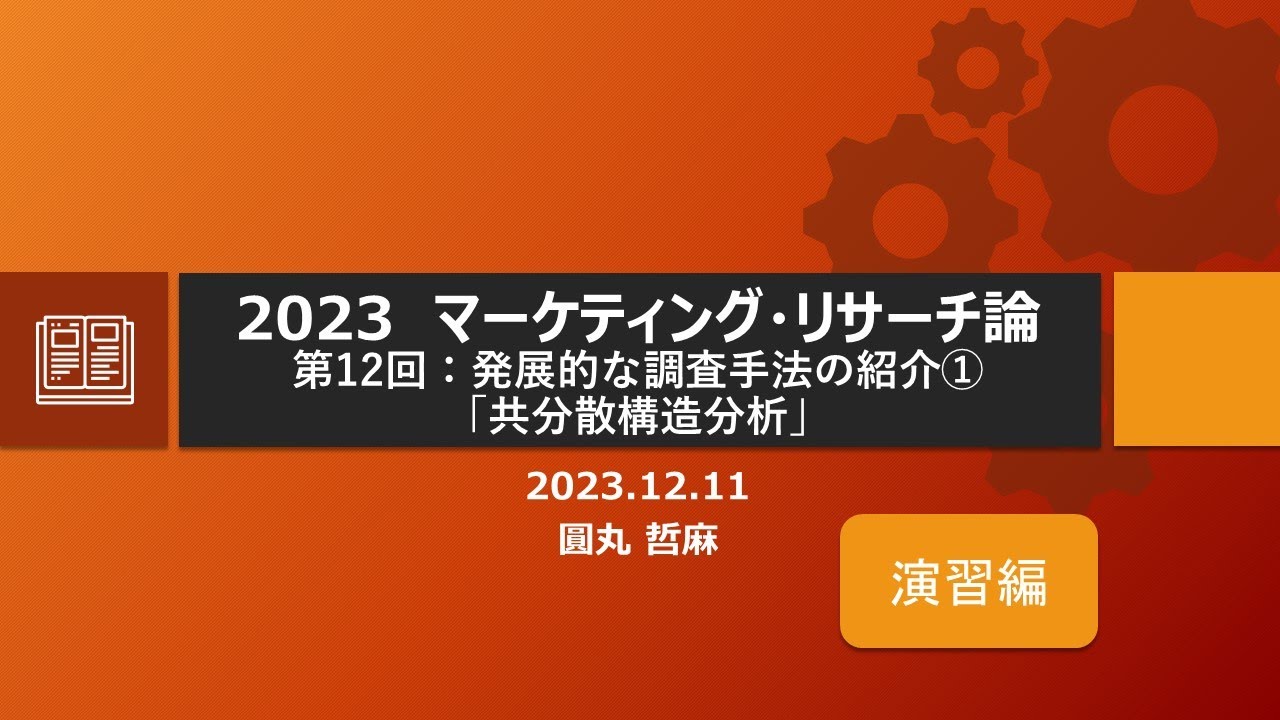
2023年後期 マーケティング・リサーチ論 第12回目の講義動画(発展的な調査手法の紹介①「共分散構造分析」演習編)
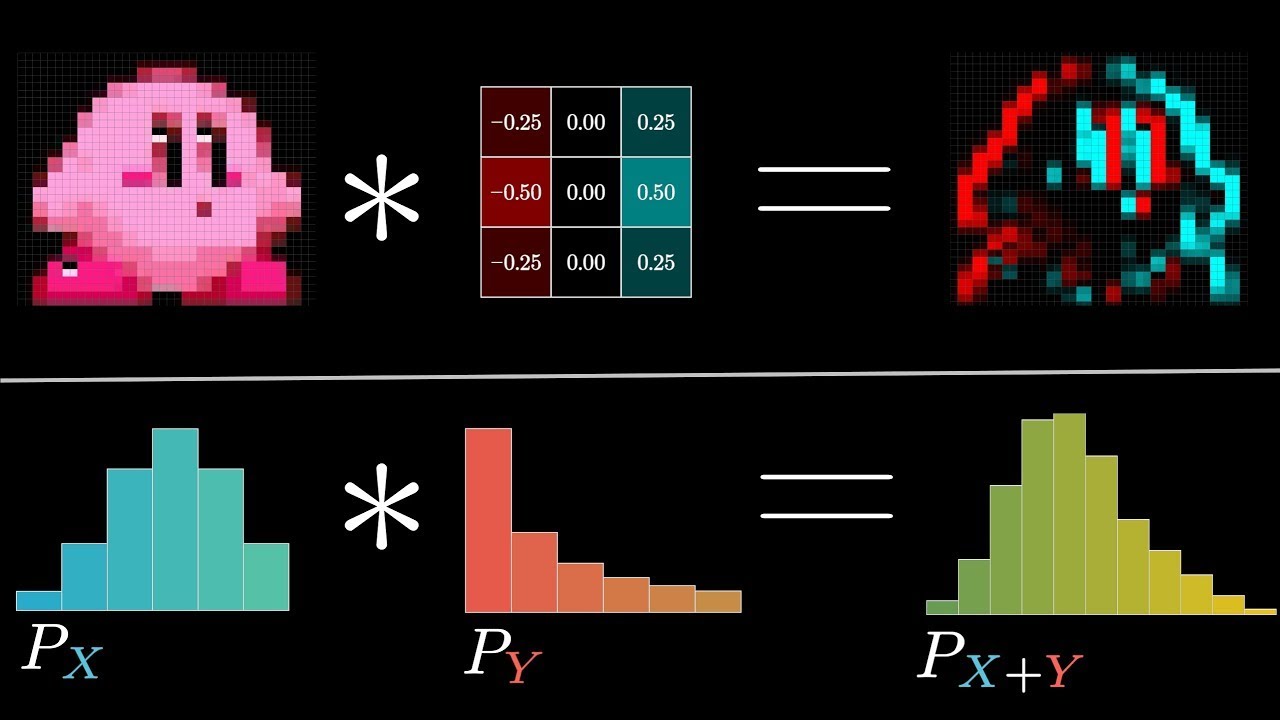
畳み込みの仕組み | Convolution
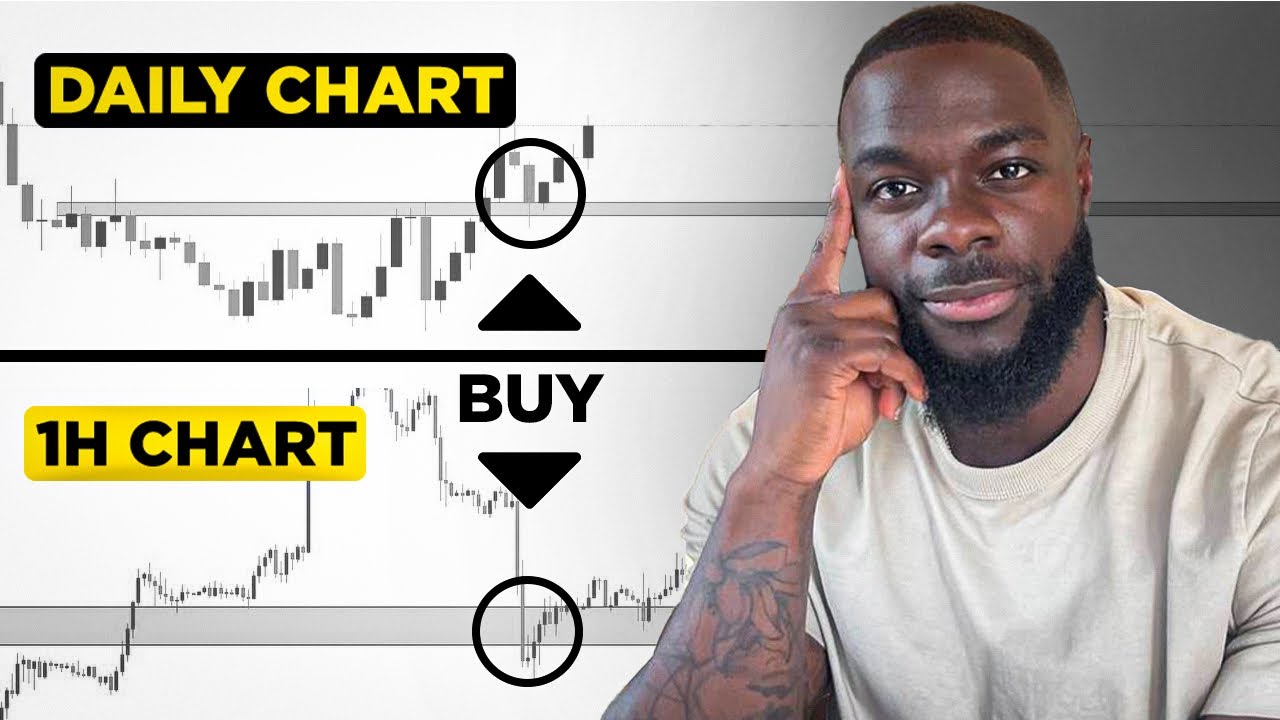
LEARN THIS SIMPLE FOREX DAY TRADING STRATEGY 2023 | How to MASTER Multiple Time Frame Analysis 💰
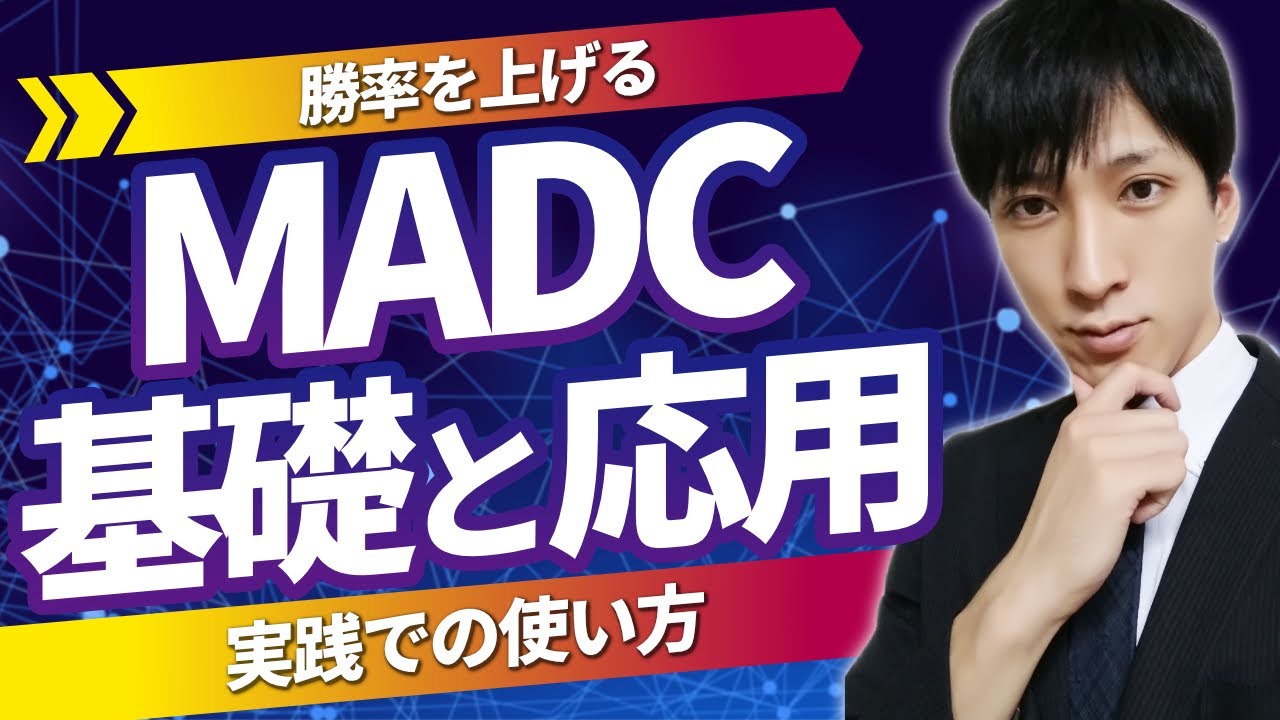
【テクニカル分析】MACDの基礎と応用。株式投資の勝率を上げる方法。
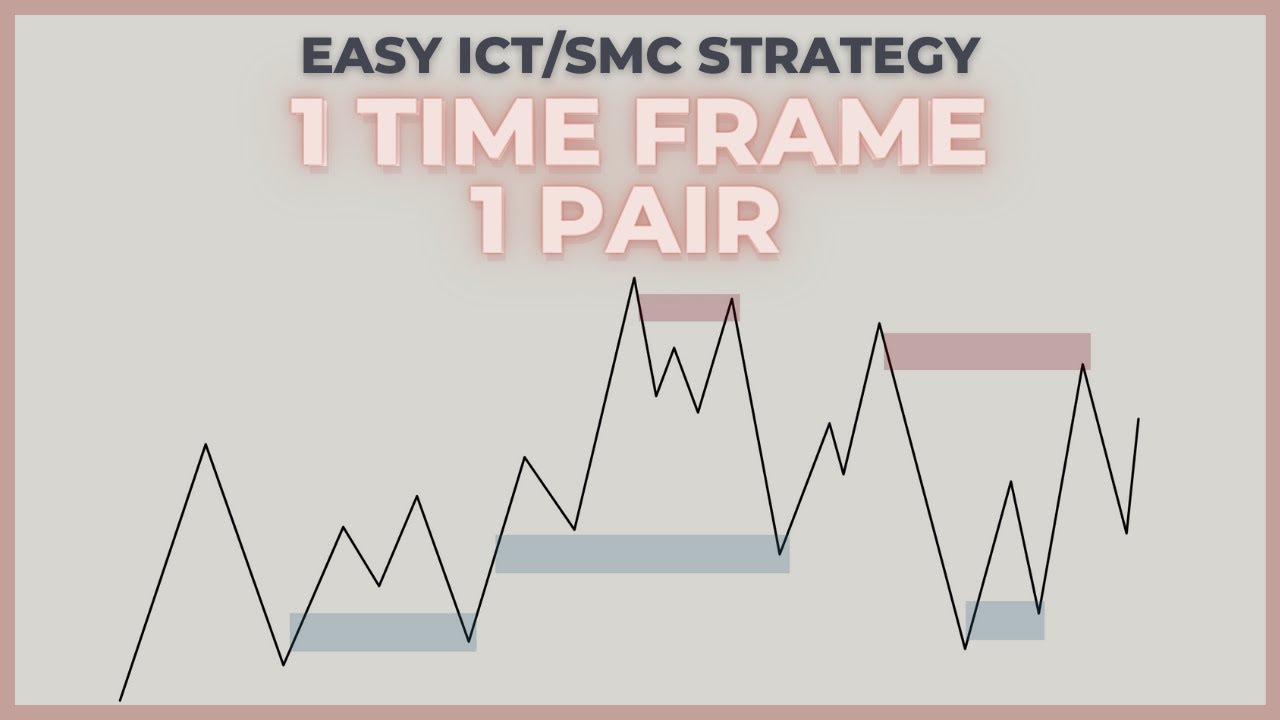
The Easiest SMC/ICT Strategy Ever: One Time Frame, One FVG, One Pair!
5.0 / 5 (0 votes)