A Day in the Life of a Data Analyst (2023)
Summary
TLDRThis video script offers an insightful look into the daily life of a data analyst, highlighting their role as storytellers, mathematicians, coders, and business consultants. It covers their morning routines, team meetings, data analysis tasks, report production, code maintenance, stakeholder meetings, and the importance of continuous learning and documentation.
Takeaways
- 🧑💼 Data Analysts are considered some of the 'sexiest job titles in the 21st Century', highlighting their importance in the modern workforce.
- 📊 A data analyst's role combines skills of storytelling, mathematics, coding, and business consulting to interpret and present data.
- 🔍 Data analysts use code to extract and analyze data from various sources, aiming to find meaningful insights for the business.
- 🏠 The flexibility of remote work is common for data analysts, with the option for a hybrid model combining remote work with in-person collaboration.
- ☕ Starting the day with a coffee and a morning ritual, such as meditation or exercise, helps set the tone for a productive day.
- 📅 Prep work in the morning involves meditation, checking emails, and staying updated with the latest in data analytics and science.
- 👥 Team meetings are crucial for aligning objectives and prioritizing tasks within sprint cycles for data analysts.
- 🔬 The core of a data analyst's work involves breaking down tasks, processing data, and ensuring data quality through testing and documentation.
- 📈 Data visualization and report creation are essential for communicating findings to stakeholders in interactive dashboards or static presentations.
- 💻 Code maintenance, including writing new code, peer reviews, and tests, is a significant part of a data analyst's daily routine.
- 📚 Documentation is vital for a data analyst to record insights, lessons learned, and findings for future reference and business continuity.
- 🤝 Stakeholder meetings are an opportunity for data analysts to communicate their work and insights to various parts of the business.
- 🔍 Continuous learning and research are essential for data analysts to stay updated with new techniques and advancements in the field.
- 🌐 Networking and engaging with the wider data analytics community can provide valuable insights and collaboration opportunities.
Q & A
What is the significance of being a data analyst in a company according to Tom?
-Tom suggests that a data analyst is like the most important person in the company, highlighting the crucial role they play in interpreting and presenting data to drive business decisions.
Why have data-related roles been called some of the 'sexiest job titles in the 21st Century'?
-The term 'sexiest job titles' refers to the allure and demand for roles like data analysts and data scientists, which are in high demand due to the increasing importance of data in decision-making.
What are the core skills a data analyst needs to possess according to the script?
-A data analyst needs to be a storyteller, a mathematician, a coder, and a business consultant. These roles require the ability to analyze data, extract meaning, and communicate findings effectively.
How does a data analyst typically start their day?
-A data analyst might start their day with a morning ritual, which can include meditation, exercise, and preparing for the day by meditating, checking emails, and reading news articles related to data analytics.
What is the importance of team meetings for a data analyst?
-Team meetings are crucial for aligning objectives and prioritizing tasks. They help data analysts work collaboratively within sprint cycles towards achieving specific goals.
Can you explain the concept of 'sprint cycles' mentioned in the script?
-Sprint cycles refer to a time-oriented structure where data analysts and teams focus on achieving a set of goals within a short, defined period, typically one or two weeks.
What does exploratory data analysis involve for a data analyst?
-Exploratory data analysis involves checking for data quality issues such as missing data or incorrect data types. It's about understanding the data to ensure it is suitable for answering the business questions at hand.
How does a data analyst ensure the data quality and code quality are maintained over time?
-A data analyst ensures data and code quality by writing tests, documenting processes, and using version control platforms like Git or GitHub to maintain and roll back to previous versions if needed.
What are the different ways a data analyst can communicate actionable insights from data?
-Data analysts can communicate insights through interactive dashboards using tools like Tableau, Power BI, or Looker, or by creating static presentations for one-off project findings.
Why is code maintenance an important part of a data analyst's job?
-Code maintenance is important for a data analyst to ensure that new code blocks are efficient, existing code is peer-reviewed for quality, and tests are written to maintain data and code quality over time.
How does a data analyst balance working remotely with face-to-face interaction with colleagues?
-A data analyst might prefer a hybrid model, where they spend most of their time working remotely but also have the opportunity to come into a central place for face-to-face interaction with peers and colleagues.
What is the role of documentation in a data analyst's workflow?
-Documentation is crucial for a data analyst to record their work, insights, and lessons learned, ensuring that the wider business understands the process and findings for future reference.
Why is continuous research important for a data analyst?
-Continuous research is vital for data analysts to stay updated with the rapidly changing field of data analytics and data science, learn new techniques, and adapt to new tools and methodologies.
Outlines
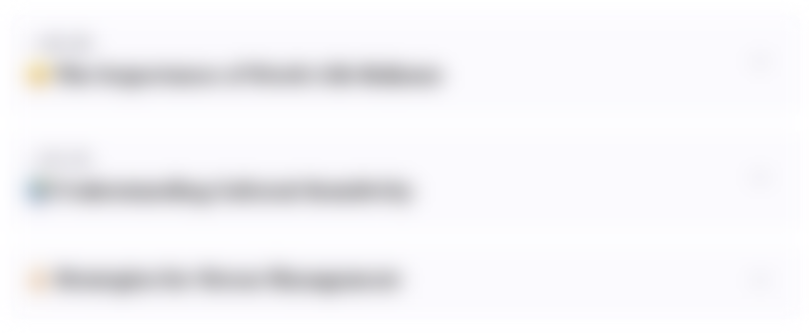
Этот раздел доступен только подписчикам платных тарифов. Пожалуйста, перейдите на платный тариф для доступа.
Перейти на платный тарифMindmap
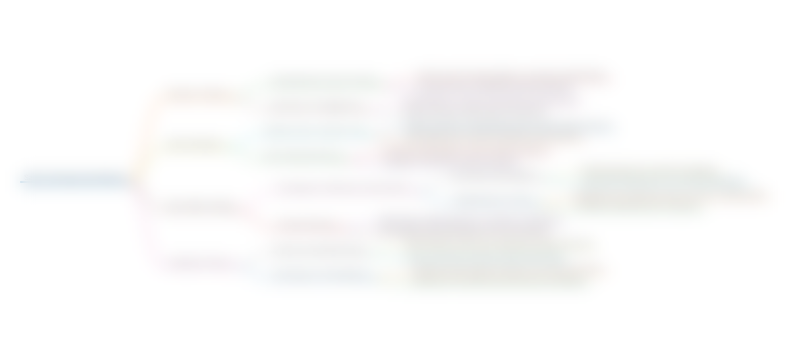
Этот раздел доступен только подписчикам платных тарифов. Пожалуйста, перейдите на платный тариф для доступа.
Перейти на платный тарифKeywords
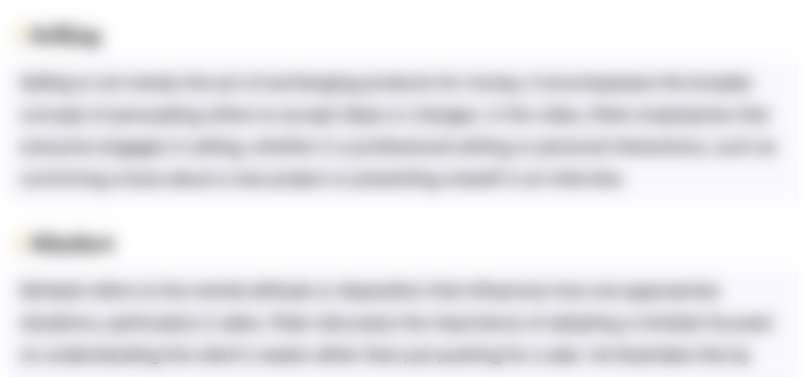
Этот раздел доступен только подписчикам платных тарифов. Пожалуйста, перейдите на платный тариф для доступа.
Перейти на платный тарифHighlights
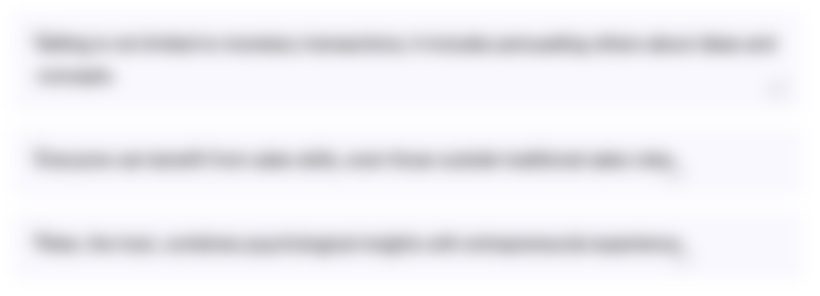
Этот раздел доступен только подписчикам платных тарифов. Пожалуйста, перейдите на платный тариф для доступа.
Перейти на платный тарифTranscripts
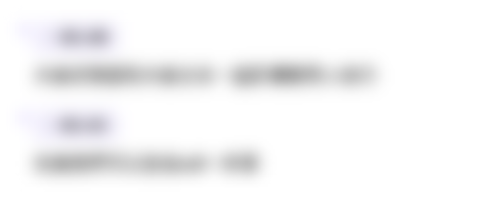
Этот раздел доступен только подписчикам платных тарифов. Пожалуйста, перейдите на платный тариф для доступа.
Перейти на платный тарифПосмотреть больше похожих видео
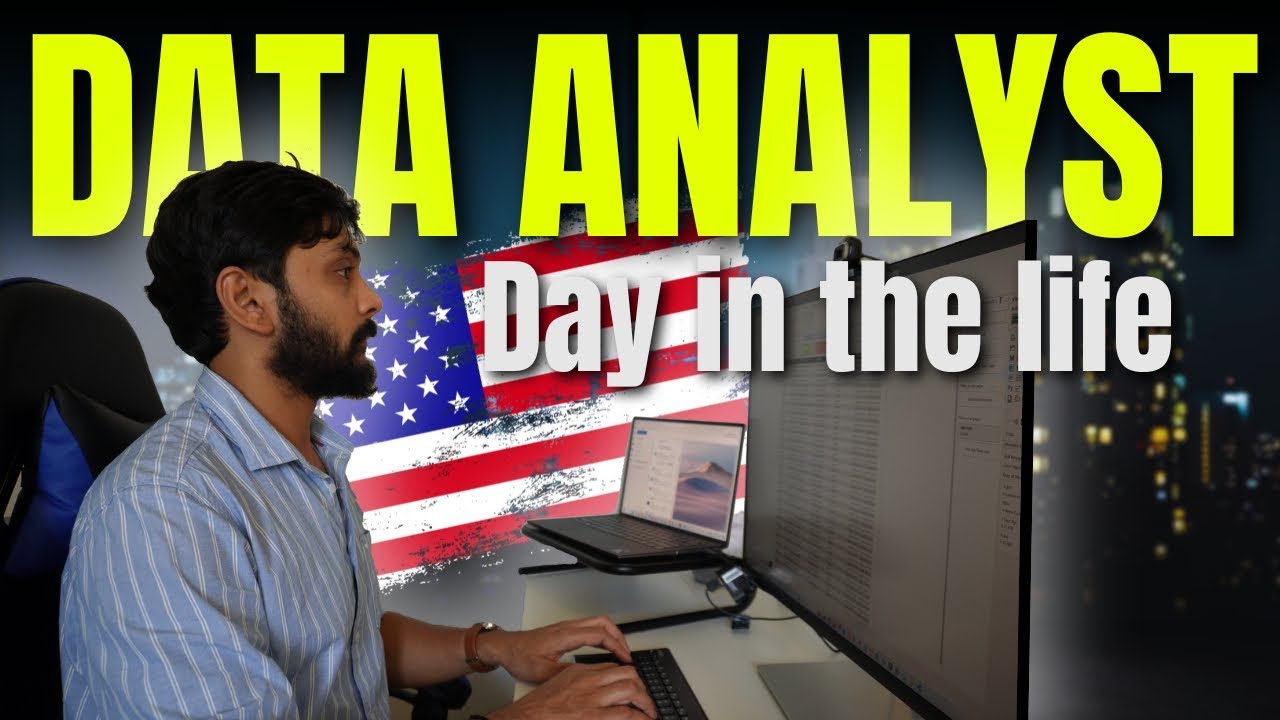
Day in the Life of a Data Analyst in USA
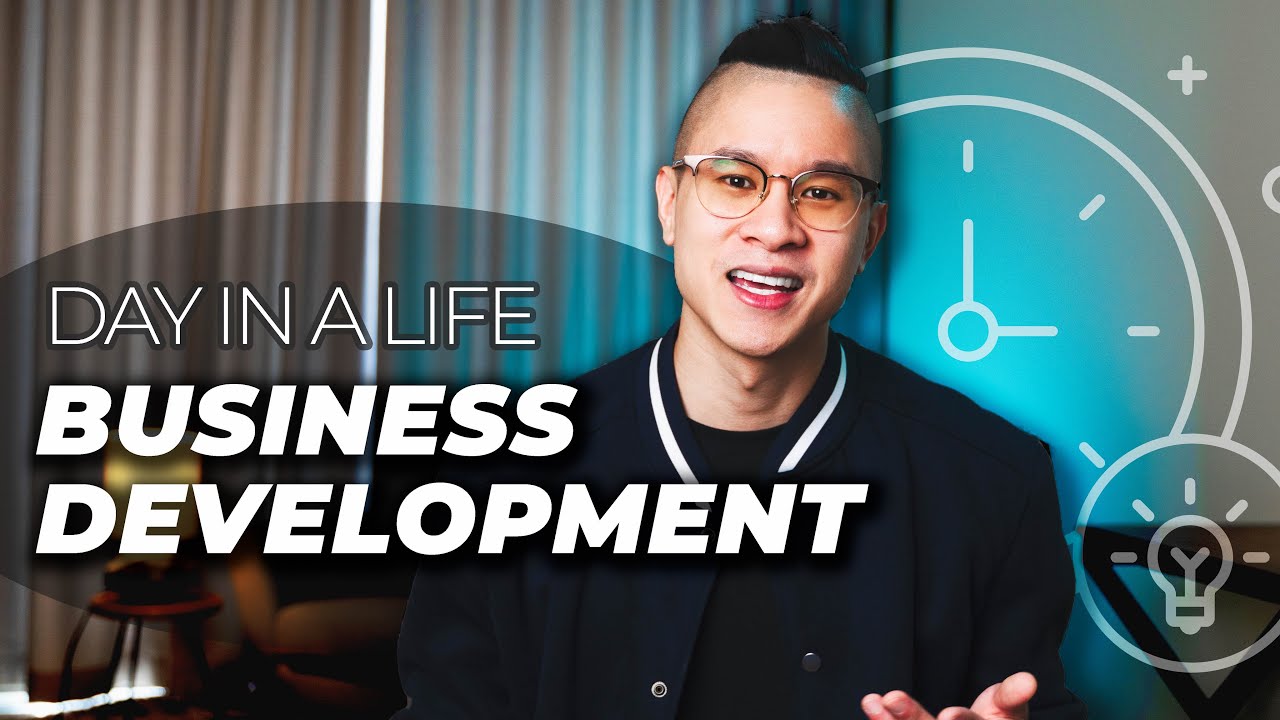
Business Development - Day In the Life in Business Development

CEO บริษัทระดับโลก มีคติในการทำงานยังไง I EP.307

Day in the Life of a Product Manager | Stanford Online Product Management

KAIZEN: Change Your Business and Create Success (with Paul Akers) Pt. 1
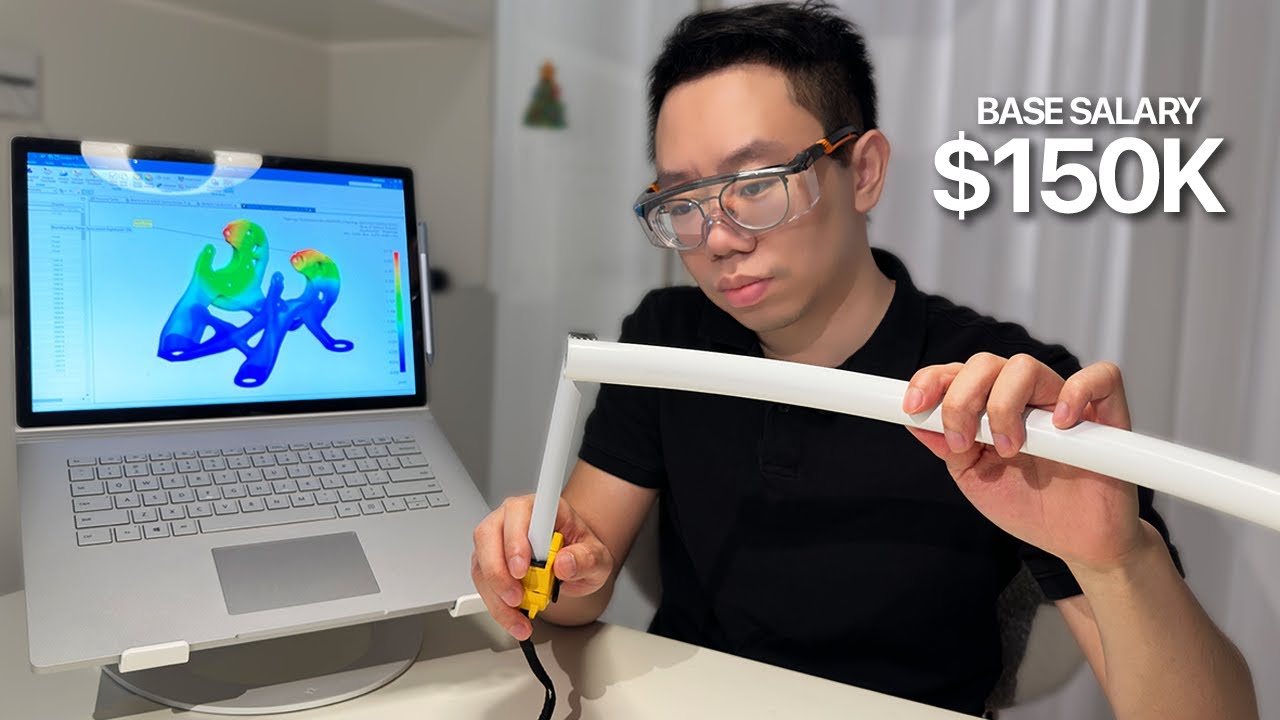
What do I do as a Mechanical Engineer?
5.0 / 5 (0 votes)