Google's LUMIERE AI Video Generation Has Everyone Stunned | Better than RunWay ML?
Summary
TLDR谷歌最新推出的AI工具Lumiere,核心功能是将文本转换成视频。它不仅能够生成视频,还能对现有图像进行动画化,创造出具有特定风格的视频,例如“视频绘画”和在图像中创建特定动画部分。谷歌的研究论文揭示了其背后的科学原理,即时空扩散模型,该模型能够生成真实感强、多样化和连贯性强的视频。Lumiere在文本到视频、图像到视频、风格化生成等方面展示了其能力,与现有的视频模型相比,它在用户偏好和视频质量方面都表现出色。这项技术的发展预示着未来视频制作可能会变得更加容易和可访问,为电影制作和故事叙述开辟了新的可能性。
Takeaways
- 🚀 Google发布了最新的AI工具Lumiere,它是一个文本到视频的AI模型,可以将文本转换为视频。
- 🎨 Lumiere不仅能将文本转换为视频,还能对现有图像进行动画处理,创造出具有特定风格或绘画风格的视频。
- 📈 Google的研究论文提到了他们对SpaceTime扩散模型的改进,这种模型能够生成逼真的视频。
- 🤖 AI生成的视频在风格和动作上表现出了高度的一致性,这在以往的模型中是一个挑战。
- 🌌 Lumiere能够将静态图像转换成动画,例如将一幅熊的图片转换成在纽约行走的动画。
- 🎭 Lumiere通过使用目标图像来生成风格化的视频,例如让一只熊以某种风格旋转跳跃。
- 📹 Lumiere引入了Spacetime单元架构,这种架构能够在一开始就构建整个视频的概念,而不是逐帧生成。
- 🎨 Lumiere还包括视频风格化功能,可以改变视频的特定风格,例如只对视频中的某些部分进行动画处理。
- 🧩 Lumiere还能够进行视频和绘画,即使图像中有一部分缺失,AI也能猜测补充缺失的部分。
- 📈 通过与其他顶尖AI模型的比较,Lumiere在文本到视频和图像到视频生成方面被用户偏好。
- ⏱️ Lumiere在视频生成中实现了更好的全局时间一致性,与逐帧生成的视频模型相比,它在视频的整个时间跨度上保持了一致性。
Q & A
Google最新推出的AI工具Lumiere的核心功能是什么?
-Lumiere的核心功能是文本到视频的AI模型,用户输入文本后,AI神经网络将其翻译成视频。此外,它还能动画化现有图像,创建具有特定风格的视频,以及在图像中创建特定的动画部分。
Lumiere如何实现视频的一致性?
-Lumiere通过其研究中提到的SpaceTime扩散模型来实现视频的一致性,该模型能够在不同帧之间创建更一致的镜头,即所谓的时间一致性。
Lumiere的image to video功能是如何工作的?
-Lumiere的image to video功能可以将静态图像转换成动画,例如将一张熊在纽约行走的图片动画化,或者将大脚怪穿过森林的图片动画化。
Lumiere的styliz generation是如何实现的?
-Lumiere使用目标图像来创建彩色或动画效果,例如,可以创建一个以大象为参考图像的动画,并保持其风格一致性。
什么是Spacetime unit architecture,它在Lumiere中扮演什么角色?
-Spacetime unit architecture是Lumiere中的一种架构,它能够一次性创建整个视频的概念,而不是像其他模型那样逐帧生成,这有助于保持视频的整体一致性。
Lumiere的视频风格化功能是什么?
-视频风格化功能允许用户将源视频转换成不同的风格,例如,可以将跑步的女性视频转换成不同的风格,或者将狗、汽车和熊的视频风格化。
什么是cinemagraphs,Lumiere如何实现这一功能?
-Cinemagraphs是一种图像中只有特定部分动画化的技术。Lumiere通过AI猜测缺失图像部分的内容,例如,可以使图像中火车冒出的烟雾动画化。
Lumiere的视频和绘画功能是如何工作的?
-视频和绘画功能允许AI猜测图像中缺失部分的内容,例如,如果图像中缺少一只手,Lumiere可以使用AI来猜测并填补这部分内容。
Lumiere在视频生成方面与其他AI模型相比有何优势?
-Lumiere在文本到视频和图像到视频生成方面,用户偏好度高于其他最先进模型,如Pika和Gen-2,它在视频质量和与文本提示的一致性方面表现更好。
Lumiere的AI模型是否能够学习到比表面统计更深层次的内容?
-根据Google的研究,尽管Lumiere的AI模型仅训练于二维图像,但它们似乎能够发展出一种内部线性表示,与场景几何学相关,这表明AI模型可能在学习到比表面统计更深层次的内容。
Runway ml提出的General World models是什么?
-General World models是Runway ml提出的一个概念,它认为下一代AI的重大进步将来自于能够理解视觉世界及其动态的系统,即通过构建世界模型来理解它们生成的图像,并利用这些模型来创建更真实的视频。
Lumiere的Spacetime diffusion model在视频生成中扮演什么角色?
-Lumiere的Spacetime diffusion model旨在创建能够展现现实、多样化和连贯运动的视频,它通过SpaceTime unet架构一次性生成整个视频的时间持续,以解决现有视频模型在全局时间一致性方面的挑战。
Lumiere的AI模型在视频生成方面有哪些创新之处?
-Lumiere的AI模型创新之处在于它能够一次性生成整个视频的时间持续,而不是逐帧生成,这有助于保持视频的全局时间一致性,避免了对象在视频中出现不一致的情况。
Lumiere的AI模型在视频生成方面的表现如何?
-根据Google的研究,Lumiere的AI模型在视频生成方面的表现优于其他最先进的模型,它能够创建更连贯、更一致的视频内容。
Lumiere的发布对视频制作领域意味着什么?
-Lumiere的发布意味着视频制作领域将迎来重大变革,它使得普通用户也能够在家中创建具有好莱坞风格的电影,这将大大降低视频制作的门槛,推动个性化和创新性内容的产生。
Outlines
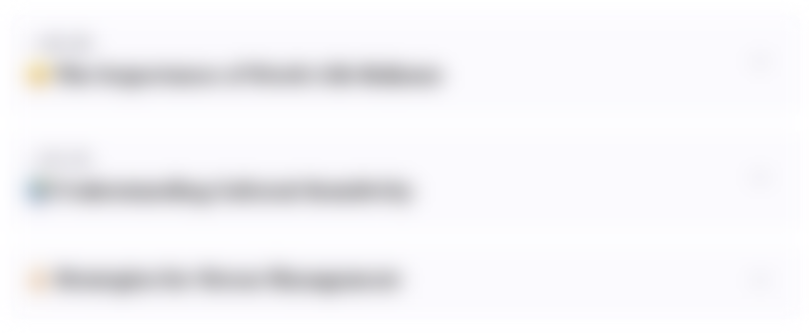
Этот раздел доступен только подписчикам платных тарифов. Пожалуйста, перейдите на платный тариф для доступа.
Перейти на платный тарифMindmap
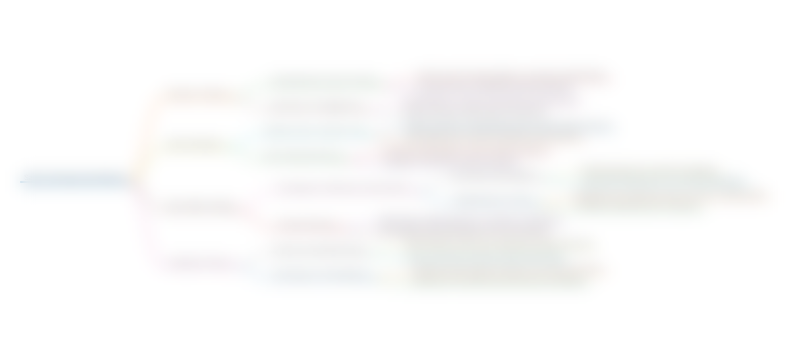
Этот раздел доступен только подписчикам платных тарифов. Пожалуйста, перейдите на платный тариф для доступа.
Перейти на платный тарифKeywords
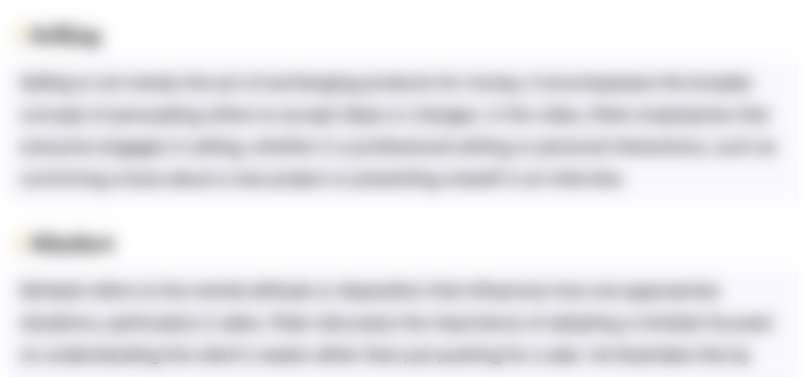
Этот раздел доступен только подписчикам платных тарифов. Пожалуйста, перейдите на платный тариф для доступа.
Перейти на платный тарифHighlights
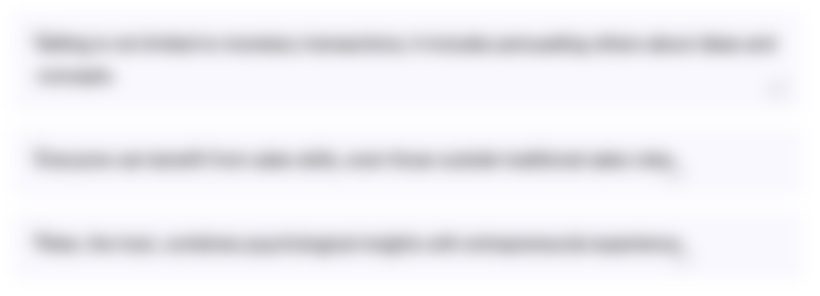
Этот раздел доступен только подписчикам платных тарифов. Пожалуйста, перейдите на платный тариф для доступа.
Перейти на платный тарифTranscripts
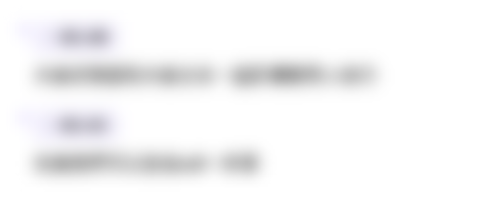
Этот раздел доступен только подписчикам платных тарифов. Пожалуйста, перейдите на платный тариф для доступа.
Перейти на платный тарифПосмотреть больше похожих видео

脚踢runway,平替sora,AI视频开源来袭,Stable video震撼公测 完全免费开放 无限使用

Ai工具大全 鬼手 #GhostCut 视频语音+文字翻译,去水印,一键换风格,换画面和声音查不出搬运,100K大礼 #Ai工具 #视频解说
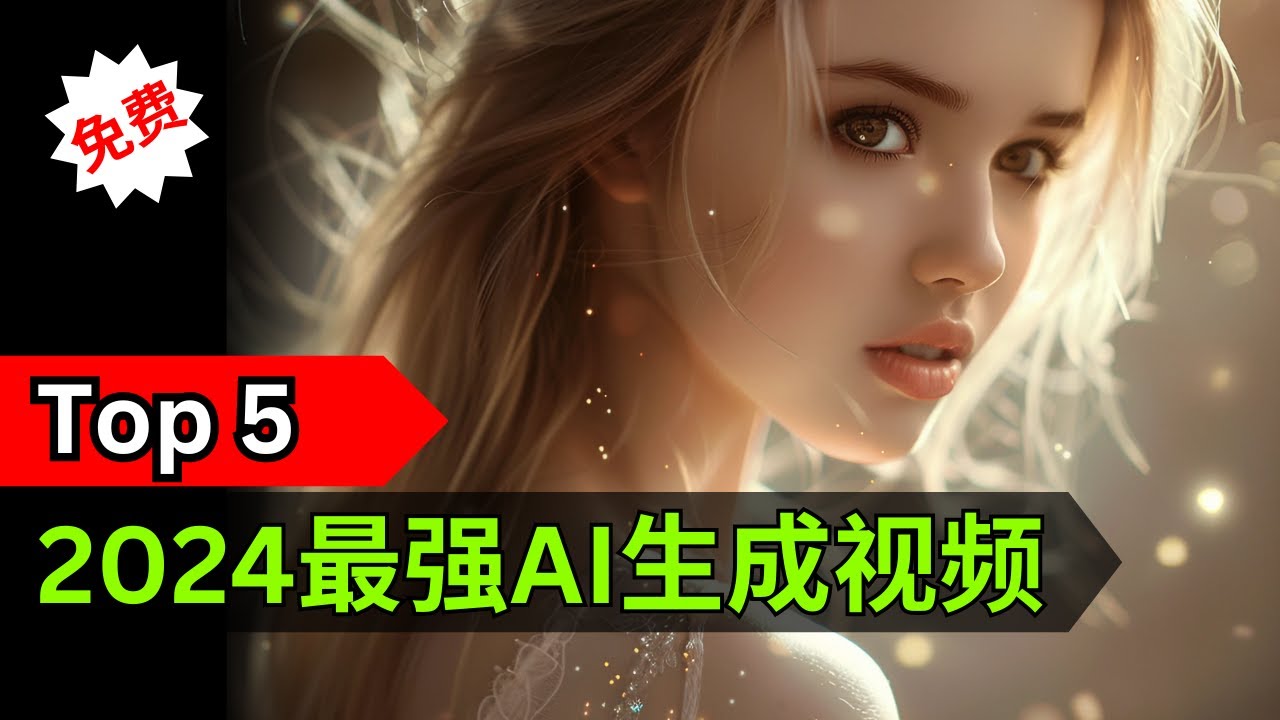
【AI】2024年最强AI视频生成工具TOP 5,其中三款完全免费
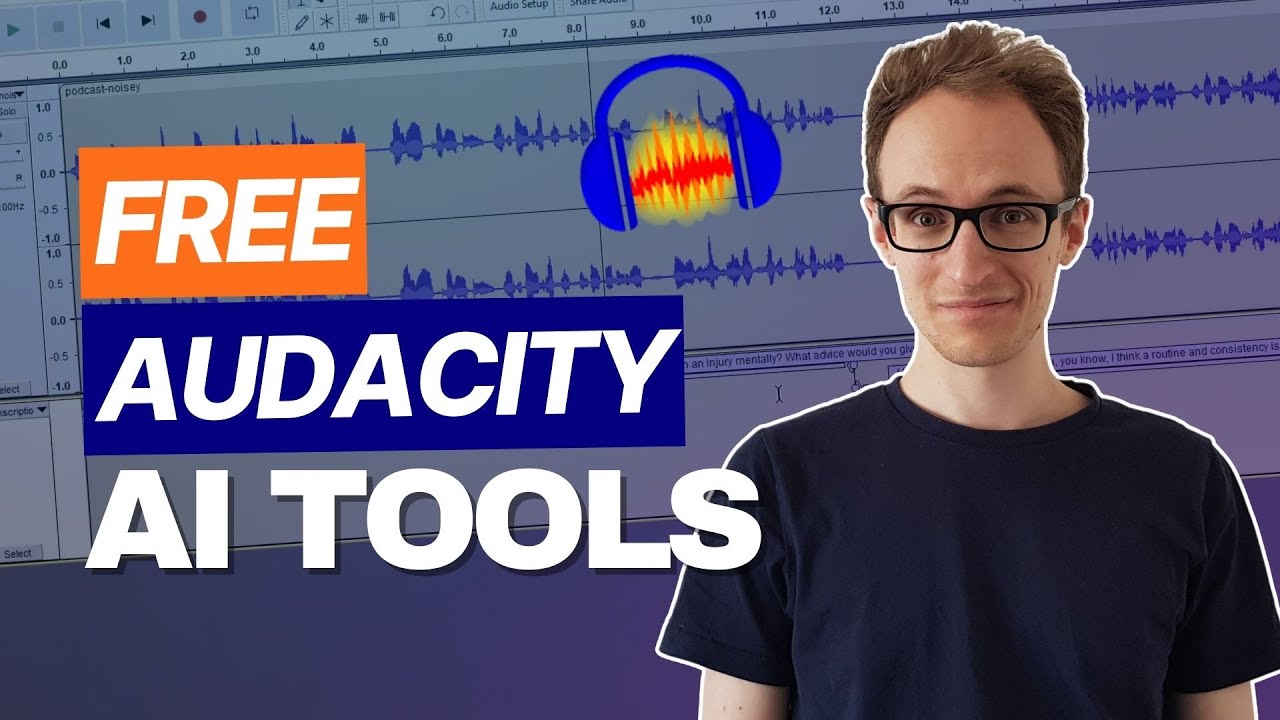
Audacity AI Tools (Free AI Plugins - OpenVINO)

什么是谷歌分析Google Analytics?谷歌分析从0到懂全教程 (网络赚钱必学免费工具)

I TRIED Earning $1400 a Day With Google News! (FREE) Way to Make Money Online?!
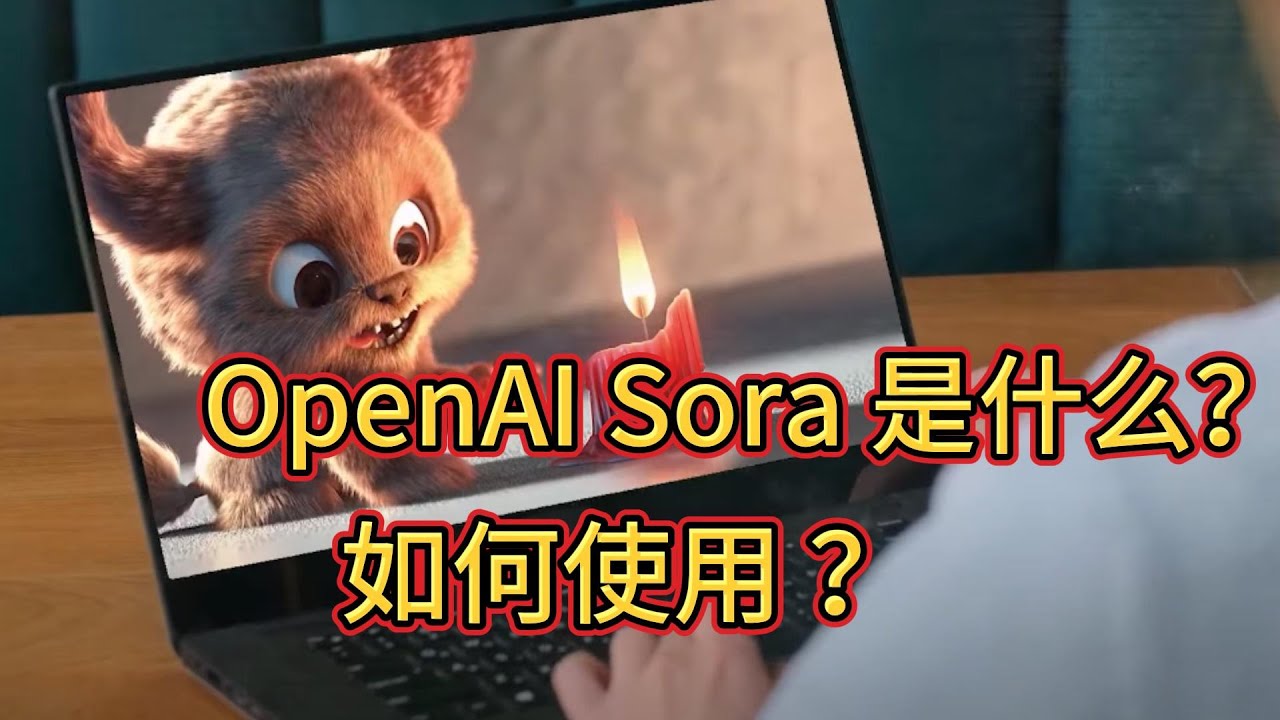
OpenAI Sora 创建视频:它是什么、如何使用它、是否可用以及其他问题的解答
5.0 / 5 (0 votes)