AI Has a Fatal Flaw—And Nobody Can Fix It
Summary
TLDRThis video explores the limits of artificial intelligence (AI), focusing on the challenges and the diminishing returns faced by current machine learning models. The video delves into how AI models like GPT-3 and GPT-4 work, explaining concepts such as parameters, embeddings, and transformers. It highlights how AI excels at language prediction and certain tasks, but struggles with real-world decision-making, advanced mathematics, and creativity. The video discusses the bottleneck of data availability and the inefficiency of scaling models. The narrative concludes with a reflection on the future of AI, emphasizing the importance of innovation beyond traditional scaling.
Takeaways
- 😀 AI has a fundamental limitation in intelligence, which is constrained by mathematical and computational boundaries.
- 😀 GPT models work by predicting the next word in a sentence based on training data, but they are not truly intelligent as humans understand it.
- 😀 AI models, like GPT, excel in specific tasks like math solving and data storage, but struggle with creativity and decision-making in real-world scenarios.
- 😀 The number of parameters used to train AI models, such as GPT-3 with 175 billion parameters, is crucial in defining their capabilities.
- 😀 Transformers in GPT models break down words into tokens, which are then embedded in a multidimensional space to understand their meanings.
- 😀 GPT models use billions of parameters and millions of training steps to adjust their predictions through trial and error, mimicking a learning process.
- 😀 The scaling of AI models, such as GPT-4, hits a wall of diminishing returns, where adding more data and parameters does not result in significantly better performance.
- 😀 The biggest challenge for improving AI models lies in the limited availability of data for training, which hinders the ability to reduce error rates further.
- 😀 Despite improvements in efficiency, there's a point where the resources needed to train AI models surpass the available data and computational power.
- 😀 Although AI is making remarkable advancements in language understanding, the next frontier is connecting AI to systems like vision and reasoning for tasks like cooking or cleaning.
- 😀 AI models are beginning to tackle more complex tasks like creative problem-solving and advanced mathematics, but still struggle in tasks requiring deep reasoning and common sense.
Q & A
What is the main focus of the video script?
-The video focuses on explaining the limitations of current AI models, particularly language models like GPT, and the challenges faced in making them significantly smarter despite increasing their size and parameters.
What mathematical equation is referenced in the script?
-The script mentions a mathematical equation related to the limitations of AI models, specifically discussing a 'wall' of diminishing returns. The equation signifies the challenge in scaling AI models beyond a certain point, where further increases in size or parameters result in only marginal improvements in performance.
What is the key limitation of current AI models as described in the video?
-The key limitation of current AI models is that despite their massive size and training on vast amounts of data, they hit a wall where further improvements in performance become minimal, and there is a shortage of data and resources needed to continue improving them significantly.
How does GPT work at a basic level?
-GPT works by predicting the next word in a sequence. It is trained on large amounts of text data, using a process that breaks down words into tokens and embeds them into high-dimensional spaces. The model adjusts its parameters during training to minimize errors in prediction.
What are parameters in the context of AI models like GPT?
-Parameters are the adjustable values in the model that guide its predictions. In GPT-3, for example, there are 175 billion parameters, which are fine-tuned during training to reduce prediction errors and improve the model's ability to generate coherent text.
What is the 'wall' or 'limit' referred to in the video?
-The 'wall' refers to a point at which increasing the size of an AI model—whether by adding more parameters, training data, or computational resources—yields diminishing returns. This means that no matter how much larger the model gets, it will not achieve proportional improvements in performance.
What is the role of GPUs in training large AI models like GPT?
-GPUs are crucial in training large AI models because they are designed to handle massive parallel computations. These parallel processing capabilities make GPUs highly efficient for training models like GPT, which involve complex calculations across millions or billions of parameters.
What is 'embedding' in the context of AI language models?
-Embedding refers to the process of converting words or tokens into a numerical representation that the AI model can understand. This involves mapping words into high-dimensional spaces where similar meanings are grouped together, making it possible for the model to identify patterns and relationships between words.
Why does GPT struggle with math problems, as mentioned in the video?
-GPT struggles with math problems because it doesn't actually perform mathematical calculations. Instead, it predicts the most likely next word based on patterns it has seen in the data. While it can accurately predict common results like simple addition (e.g., 1+1=2), it doesn't 'understand' math in the traditional sense.
What is the significance of GPT-4's improvements over GPT-3?
-GPT-4 is an improvement over GPT-3 due to its increased number of parameters (1.8 trillion), enhanced training, and better performance in generating more accurate and coherent text. However, even with these advancements, the model still faces limitations due to the diminishing returns mentioned earlier.
Outlines
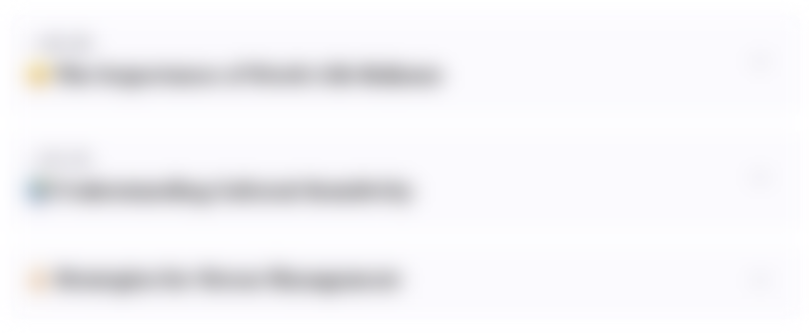
Этот раздел доступен только подписчикам платных тарифов. Пожалуйста, перейдите на платный тариф для доступа.
Перейти на платный тарифMindmap
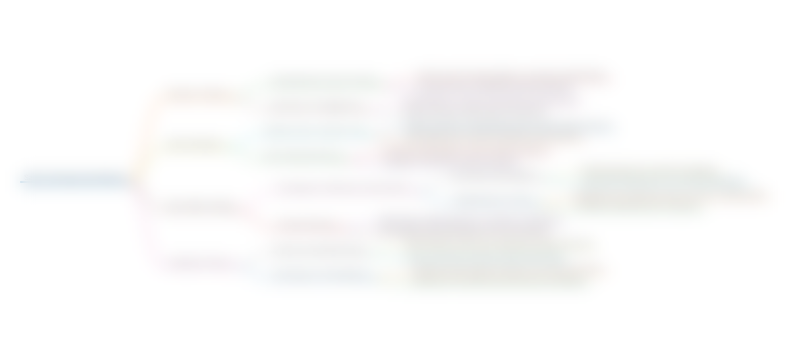
Этот раздел доступен только подписчикам платных тарифов. Пожалуйста, перейдите на платный тариф для доступа.
Перейти на платный тарифKeywords
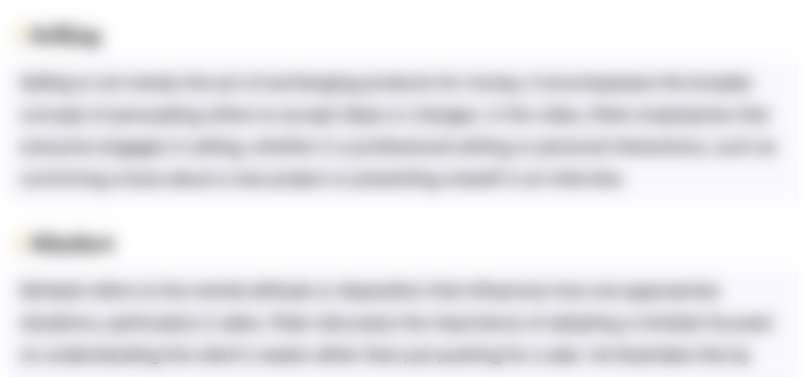
Этот раздел доступен только подписчикам платных тарифов. Пожалуйста, перейдите на платный тариф для доступа.
Перейти на платный тарифHighlights
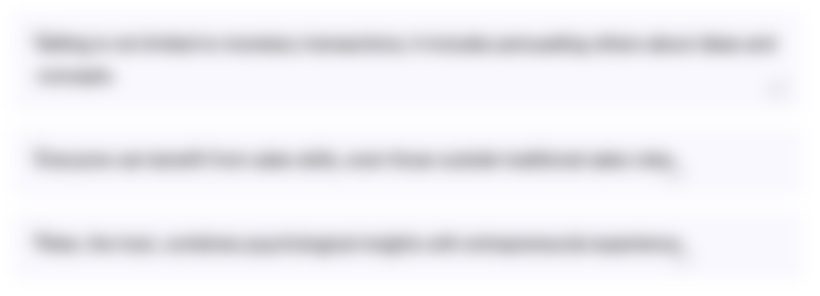
Этот раздел доступен только подписчикам платных тарифов. Пожалуйста, перейдите на платный тариф для доступа.
Перейти на платный тарифTranscripts
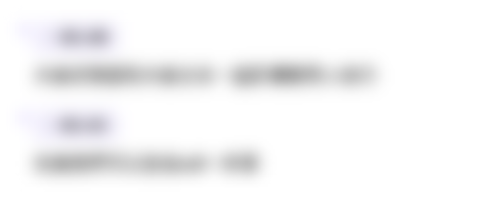
Этот раздел доступен только подписчикам платных тарифов. Пожалуйста, перейдите на платный тариф для доступа.
Перейти на платный тарифПосмотреть больше похожих видео
5.0 / 5 (0 votes)