Tutorial Klasifikasi Algoritma Naive Bayes Classifier dengan Python - Google Colab
Summary
TLDRIn this tutorial, we demonstrate how to implement a Naive Bayes classification algorithm in Python using Google Colab. The dataset, sourced from Kaggle, contains features of oranges and grapefruits, including diameter, weight, and color. We walk through preprocessing steps like label encoding and data splitting, followed by scaling and training the Naive Bayes model. After making predictions, we evaluate the model's performance with a confusion matrix, classification report, and accuracy score, ultimately achieving 92% accuracy. The tutorial also includes instructions for comparing actual vs. predicted values and exporting the results as an Excel file.
Takeaways
- 😀 Assalamualaikum, this tutorial covers a step-by-step guide on using Naive Bayes for data mining with Python in Google Colab.
- 📊 The dataset used for classification consists of fruits (oranges and grapefruits) with attributes like diameter, weight, and color.
- 📥 Google Colab is used to write and run Python code, which is ideal for beginners as it is free and accessible.
- 📚 The script uses three primary libraries: NumPy (for matrices and vectors), Pandas (for structured data processing), and Scikit-learn (for machine learning tasks).
- 🔄 Label Encoding is applied to convert categorical data (e.g., fruit names) into numerical labels, which are easier for the model to process.
- 🧑🏫 The dataset is split into training and testing data using `train_test_split` with a test size of 20% and random state for reproducibility.
- ⚖️ Feature scaling is performed using StandardScaler to normalize the features, ensuring that data with different ranges (like diameter and color) don't skew the model.
- 🧠 Naive Bayes, specifically Gaussian Naive Bayes, is the classification algorithm used for training the model with the preprocessed data.
- 📈 The model’s accuracy is evaluated using metrics such as Confusion Matrix, Classification Report (Precision, Recall, F1-Score), and Accuracy Score.
- 📂 The final results are saved in an Excel file for further analysis, comparing actual and predicted labels in a table format.
Q & A
What is the main goal of this tutorial?
-The main goal of this tutorial is to demonstrate how to perform data mining using the Naive Bayes classification algorithm in Python. The script covers the steps of importing a dataset, preprocessing the data, training a classifier, making predictions, and evaluating the model.
What libraries are required for this tutorial?
-The tutorial requires three main Python libraries: pandas for data manipulation, numpy for numerical operations, and scikit-learn for machine learning algorithms and evaluation metrics.
Why is label encoding used in this tutorial?
-Label encoding is used to convert categorical variables (such as the fruit names 'orange' and 'grapefruit') into numeric labels. This is necessary because machine learning models, including Naive Bayes, require numeric data to perform calculations.
What is the purpose of splitting the dataset into training and testing sets?
-Splitting the dataset into training and testing sets is crucial for evaluating the model's performance. The model is trained on the training set and then tested on the testing set to ensure it generalizes well to unseen data.
What does data scaling do in this context, and why is it important?
-Data scaling normalizes the feature values so that they are within a similar range. This is important because without scaling, features with larger numerical ranges (like weight) might dominate over others, causing the model to be biased towards those features.
How does Naive Bayes classify data in this tutorial?
-In this tutorial, Naive Bayes is used to classify the fruits (orange vs. grapefruit) based on features such as diameter, weight, and color. The algorithm assumes independence between the features and calculates the probability of each class given the feature values.
What metrics are used to evaluate the performance of the Naive Bayes model?
-The performance of the Naive Bayes model is evaluated using the confusion matrix, classification report, and accuracy score. The confusion matrix shows the number of correct and incorrect predictions, while the classification report provides additional metrics like precision, recall, and F1-score.
What does a confusion matrix tell us about the model's performance?
-A confusion matrix shows the number of true positive, false positive, true negative, and false negative predictions. This helps evaluate the accuracy and reliability of the model's predictions by comparing predicted labels with actual labels.
What is the role of the classification report in evaluating a model?
-The classification report provides detailed metrics for each class, such as precision, recall, and F1-score. These metrics help assess how well the model is performing for each class (e.g., orange and grapefruit) and give a more comprehensive view of its accuracy.
How is the accuracy of the model calculated, and what was the result in this tutorial?
-The accuracy of the model is calculated by comparing the number of correct predictions to the total number of predictions. In this tutorial, the accuracy of the Naive Bayes model was 92%, meaning 92% of the test data was correctly classified.
Outlines
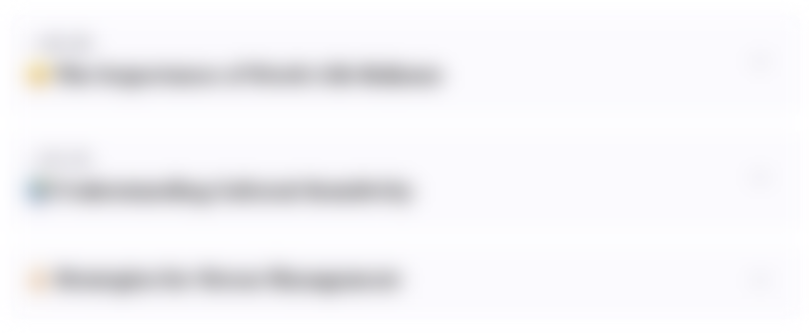
Этот раздел доступен только подписчикам платных тарифов. Пожалуйста, перейдите на платный тариф для доступа.
Перейти на платный тарифMindmap
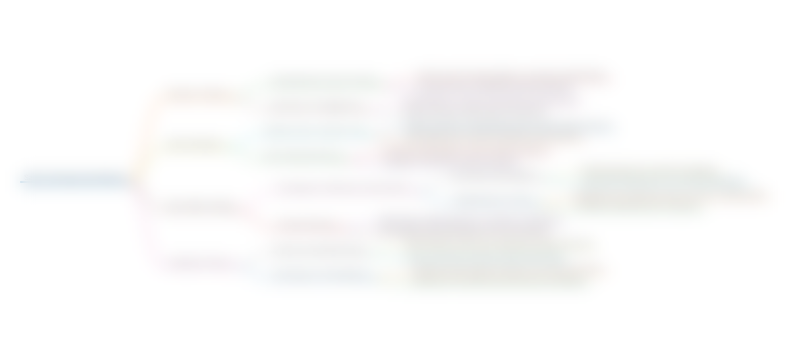
Этот раздел доступен только подписчикам платных тарифов. Пожалуйста, перейдите на платный тариф для доступа.
Перейти на платный тарифKeywords
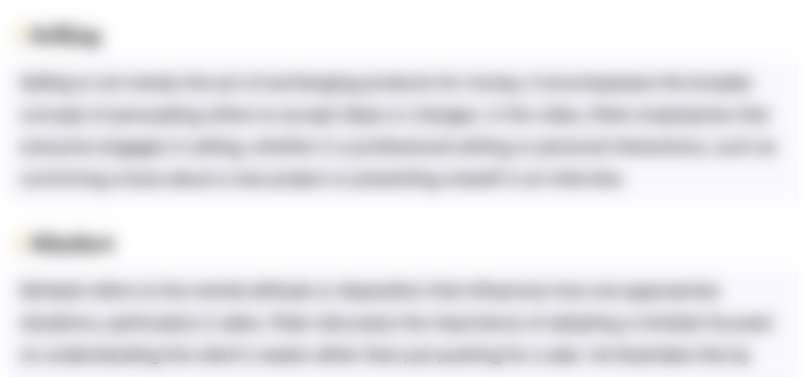
Этот раздел доступен только подписчикам платных тарифов. Пожалуйста, перейдите на платный тариф для доступа.
Перейти на платный тарифHighlights
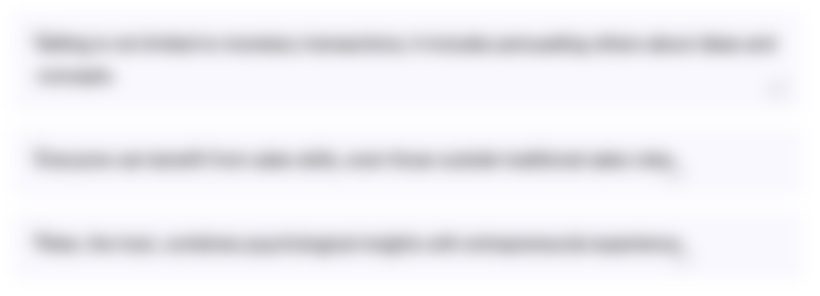
Этот раздел доступен только подписчикам платных тарифов. Пожалуйста, перейдите на платный тариф для доступа.
Перейти на платный тарифTranscripts
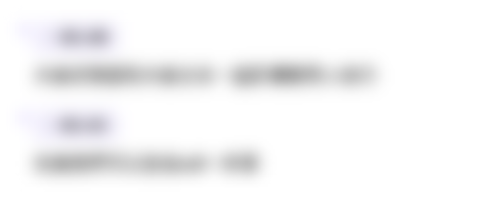
Этот раздел доступен только подписчикам платных тарифов. Пожалуйста, перейдите на платный тариф для доступа.
Перейти на платный тарифПосмотреть больше похожих видео

Naive Bayes dengan Python & Google Colabs | Machine Learning untuk Pemula
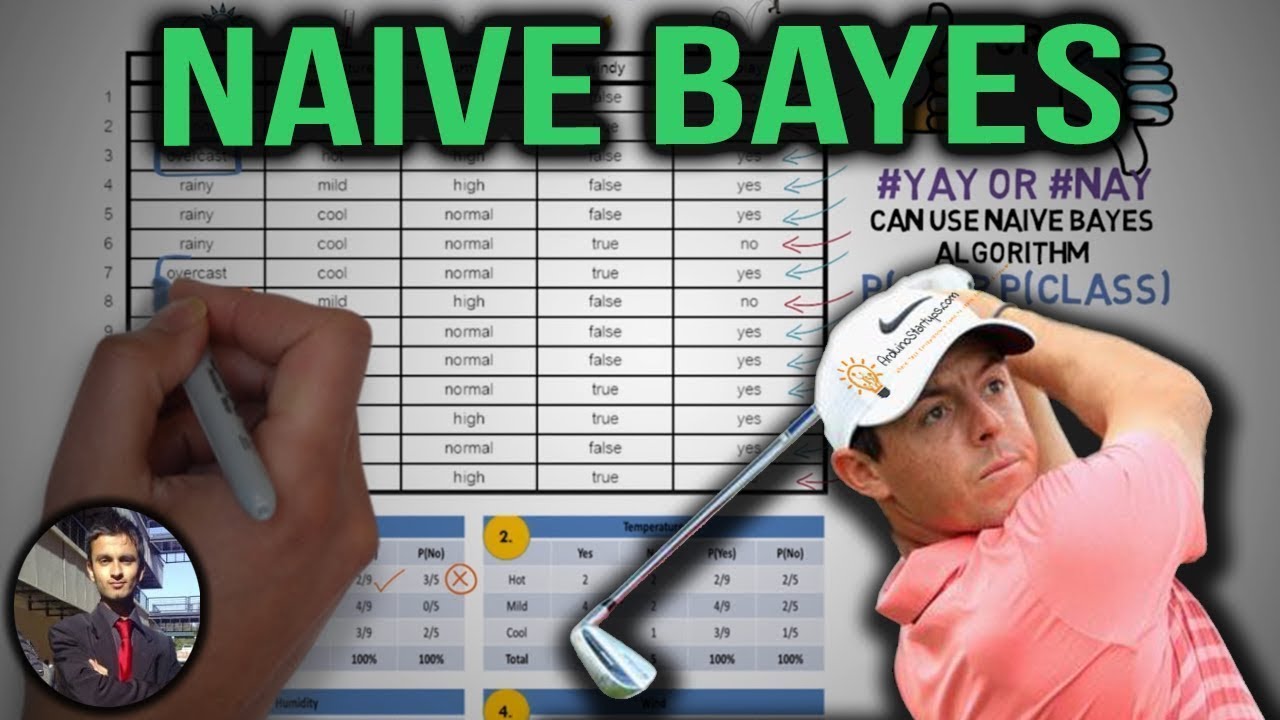
Naïve Bayes Classifier - Fun and Easy Machine Learning
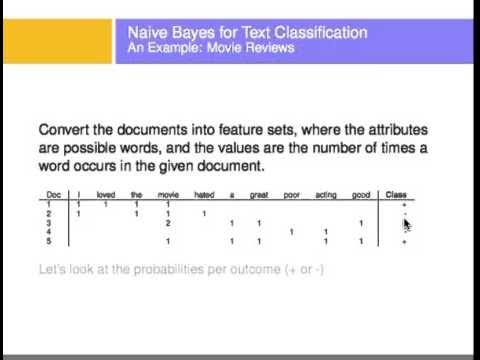
Text Classification Using Naive Bayes
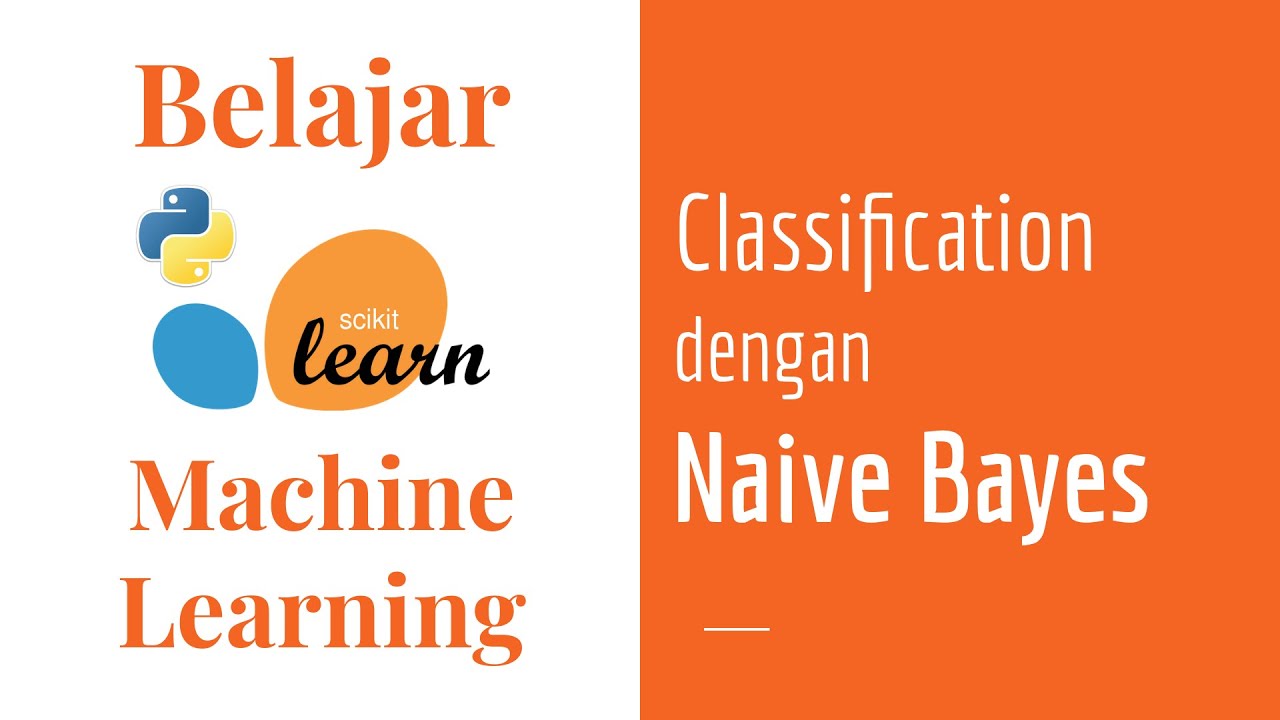
SKLearn 13 | Naive Bayes Classification | Belajar Machine Learning Dasar
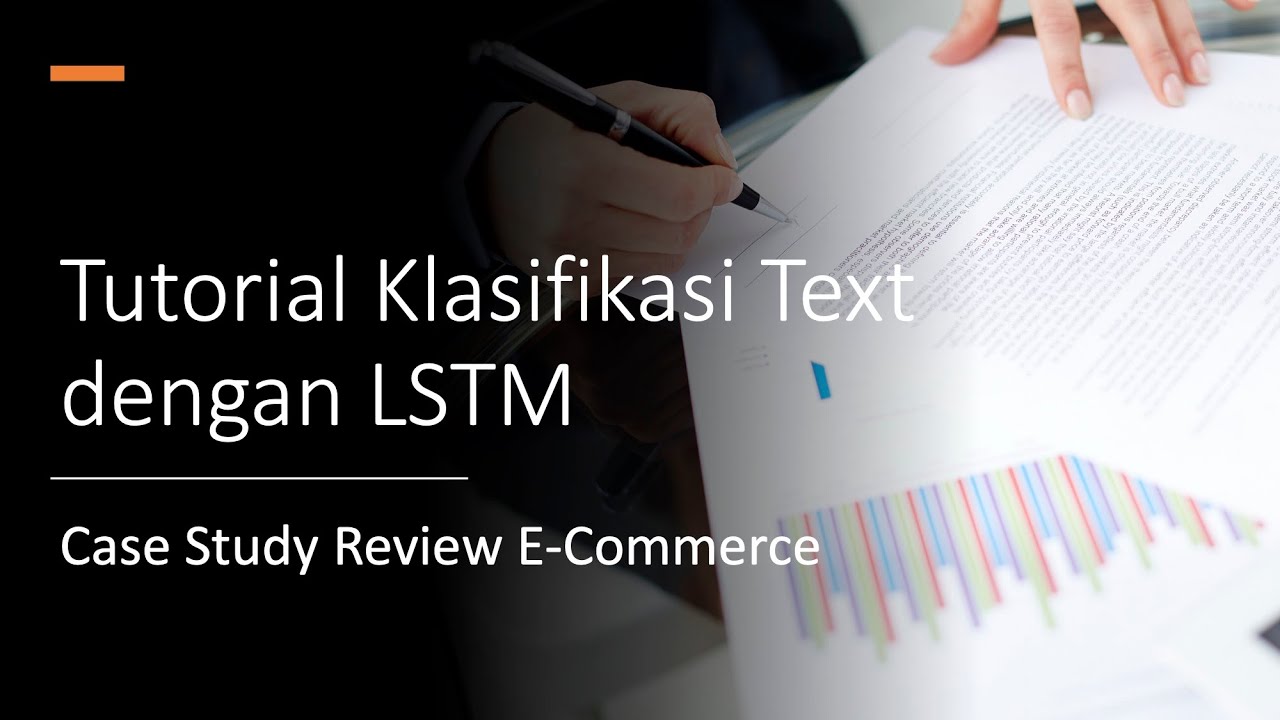
Tutorial Klasifikasi Teks dengan Long Short-term Memory (LSTM): Studi Kasus Teks Review E-Commerce
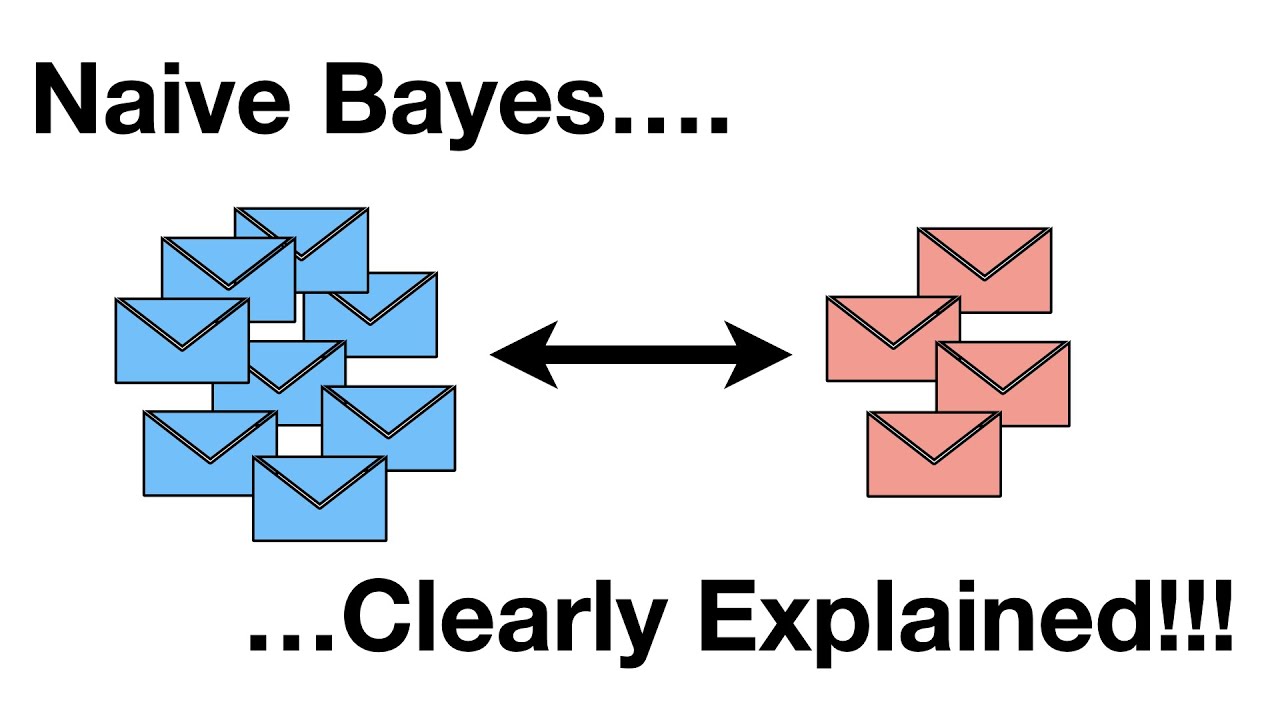
Naive Bayes, Clearly Explained!!!
5.0 / 5 (0 votes)