Unraveling AI Bias: Principles & Practices
Summary
TLDRThis video explores the impact of generative AI across various industries and highlights the emerging risks associated with its use, particularly bias. It defines AI bias, explains the different types such as algorithmic, cognitive, and exclusion bias, and discusses how these biases affect AI outcomes. The video provides actionable strategies for mitigating bias, including AI governance, diverse teams, careful data processing, and continuous monitoring. With a focus on fairness, transparency, and ethical development, the video emphasizes how enterprises can build more inclusive and responsible AI systems.
Takeaways
- 😀 Generative AI has a broad impact, driving economic transformation, industry advancements, and improved productivity across various sectors, such as legal, customer engagement, and cost savings.
- 😀 The three main benefits of generative AI are the ability to perform complex tasks, increased productivity, and shorter time to market for products and services.
- 😀 Like all new technologies, generative AI brings risks, such as downstream model retraining, copyright issues, and privacy concerns with proprietary or personal data.
- 😀 Bias is one of the most amplified risks in generative AI, with the potential for harm in AI systems if not properly addressed.
- 😀 AI bias refers to systems that produce biased results, often reflecting historical and societal inequalities, and these results can be harmful.
- 😀 Types of biases in AI include algorithmic bias, cognitive bias, outgroup homogeneity bias, prejudice bias, and exclusion bias, each with its own impact on AI systems.
- 😀 Algorithmic bias occurs when AI systems systematically produce unfair or discriminatory outcomes, like the example of a loan application system rejecting applicants born before 1945.
- 😀 Cognitive biases, such as recency bias and confirmation bias, can be embedded into AI systems due to human decision-making during system design.
- 😀 Exclusion bias arises when important data is inadvertently left out during sampling, leading to skewed or inaccurate results.
- 😀 Effective AI governance is crucial for managing and mitigating bias, ensuring responsible AI development, and promoting fairness, equity, and inclusion in AI systems.
- 😀 Proven methods for avoiding bias include selecting diverse stakeholders to choose training data, leveraging fairness tools in unsupervised learning models, creating balanced AI teams, mindful data processing, and continuous monitoring of AI systems to adapt to evolving data.
Q & A
What are the three main benefits of generative AI mentioned in the video?
-The three main benefits of generative AI highlighted in the video are: the ability to perform complex tasks, increased productivity, and shorter time to value for enterprises to launch products and services.
What are the key risks associated with generative AI?
-The key risks associated with generative AI include model retraining risks, copyright infringement, exposure of private or personal data, lack of transparency in model outputs, and, most notably, bias in AI systems.
How is AI bias defined in the video?
-AI bias, or machine learning bias, refers to AI systems producing biased results that reflect and perpetuate human biases in society, often linked to historical and current social inequalities.
What is algorithmic bias, and can you provide an example?
-Algorithmic bias is a systematic and erroneous behavior of AI systems that consistently produce unfair outcomes. An example would be a loan application system that automatically rejects applicants born before 1945, creating age bias.
What is cognitive bias, and how does it affect AI systems?
-Cognitive bias refers to biases introduced by human designers when creating AI systems. For instance, recency bias, where recent events disproportionately influence decision-making, can be embedded in AI systems during development.
What is outgroup homogeneity bias?
-Outgroup homogeneity bias occurs when a diverse dataset is assumed to represent a broad spectrum of people, but there is an unconscious bias that those outside the 'diverse' group are all similar to each other.
What are prejudice and exclusion bias, and how do they affect AI systems?
-Prejudice bias is based on faulty societal assumptions, such as the belief that all nurses are female or all doctors are male. Exclusion bias happens when important data is unintentionally left out during sampling, such as excluding lower-performing employees from a survey.
How can organizations address bias in AI development?
-Organizations can address AI bias by adopting AI governance practices, selecting diverse teams for data selection and algorithm creation, ensuring proper data processing, and regularly monitoring the AI system to detect and correct bias over time.
Why is AI governance important in reducing bias?
-AI governance is crucial because it provides frameworks, policies, and practices that help direct, manage, and monitor AI activities, ensuring that the development of AI is responsible and that fairness, equity, and inclusion are maintained.
What methods can be used to ensure bias-free AI development?
-To ensure bias-free AI development, organizations can select diverse and representative learning models, create balanced AI teams, carefully process data to avoid bias at each stage, and monitor systems regularly to adapt to evolving societal trends and data.
What role do third-party assessments play in addressing AI bias?
-Third-party assessments provide an external evaluation of AI systems to detect bias, ensuring that the systems are fair, transparent, and meet industry standards for equity, which can also serve as a validation of the enterprise's efforts to avoid bias.
Outlines
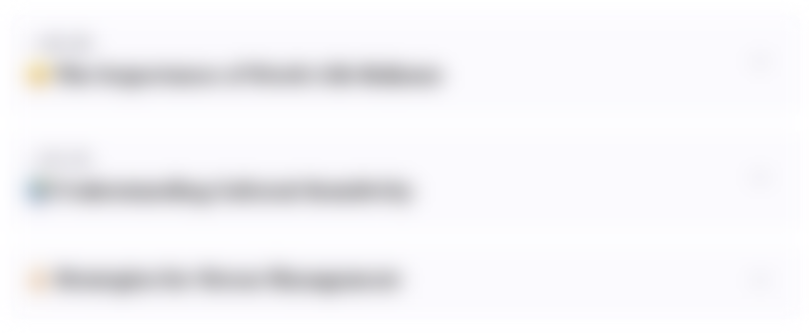
Этот раздел доступен только подписчикам платных тарифов. Пожалуйста, перейдите на платный тариф для доступа.
Перейти на платный тарифMindmap
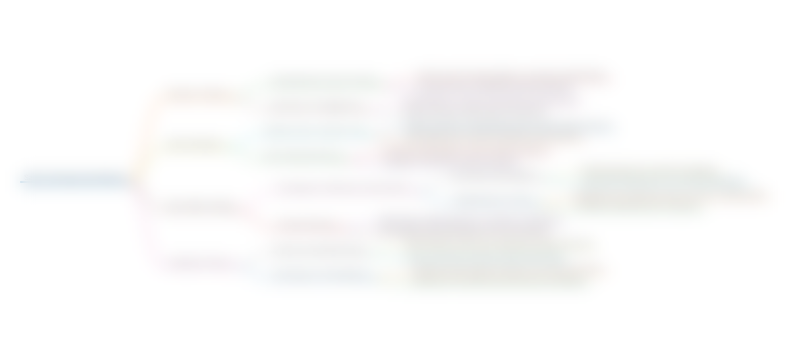
Этот раздел доступен только подписчикам платных тарифов. Пожалуйста, перейдите на платный тариф для доступа.
Перейти на платный тарифKeywords
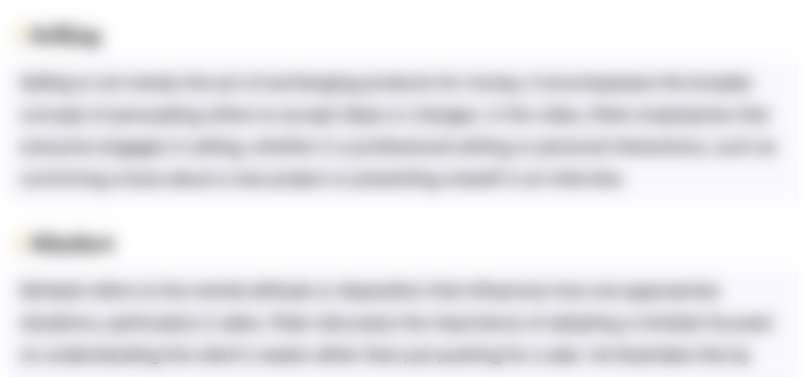
Этот раздел доступен только подписчикам платных тарифов. Пожалуйста, перейдите на платный тариф для доступа.
Перейти на платный тарифHighlights
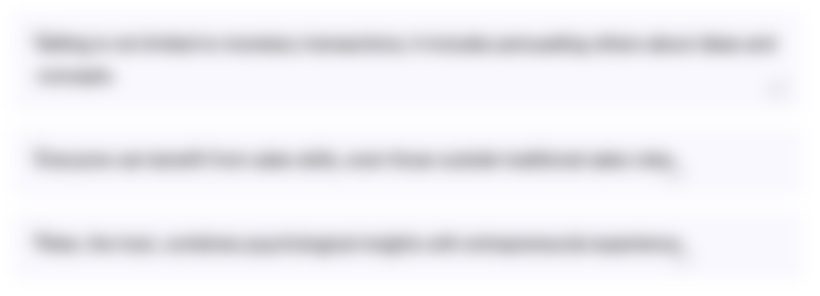
Этот раздел доступен только подписчикам платных тарифов. Пожалуйста, перейдите на платный тариф для доступа.
Перейти на платный тарифTranscripts
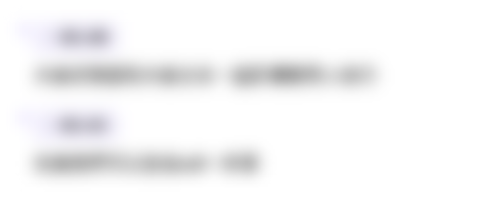
Этот раздел доступен только подписчикам платных тарифов. Пожалуйста, перейдите на платный тариф для доступа.
Перейти на платный тарифПосмотреть больше похожих видео
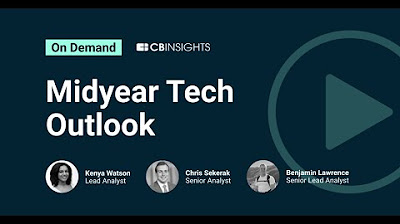
Midyear Tech Outlook: Where Industry Activity is Heating Up
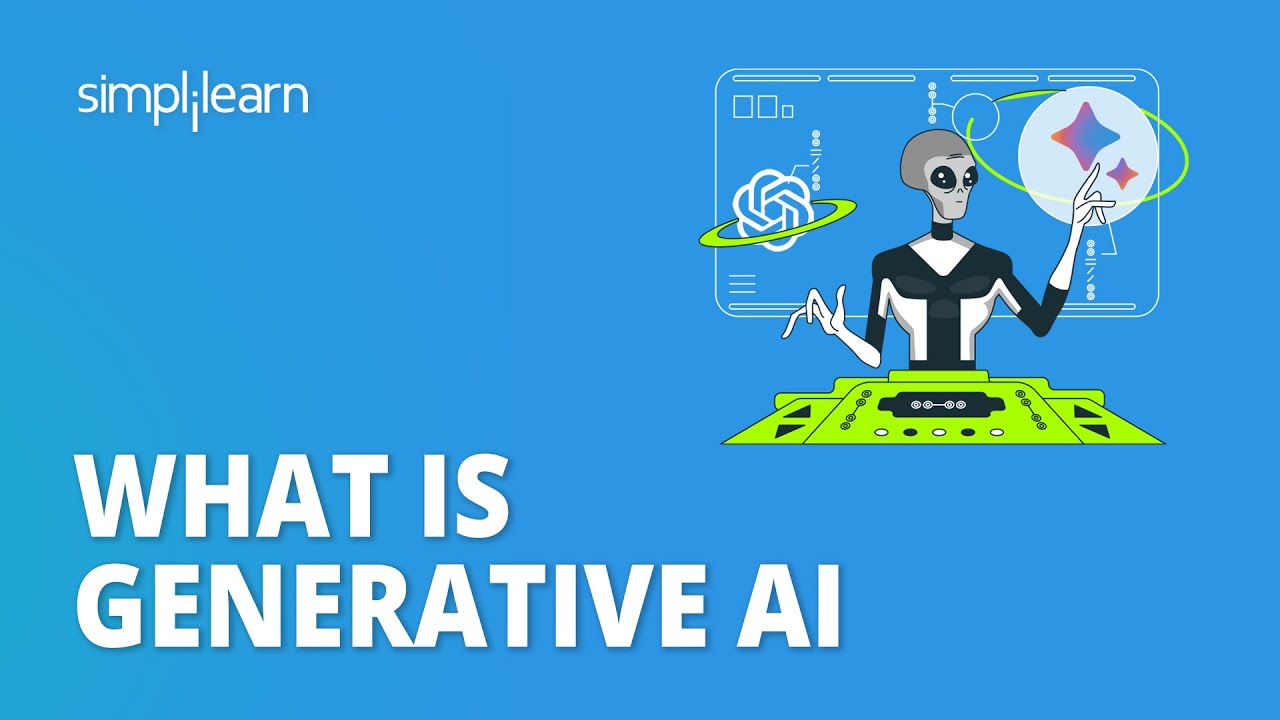
What is Generative AI | Introduction to Generative AI | Generative AI Explained | Simplilearn

Telus SmartHome | AWS Events
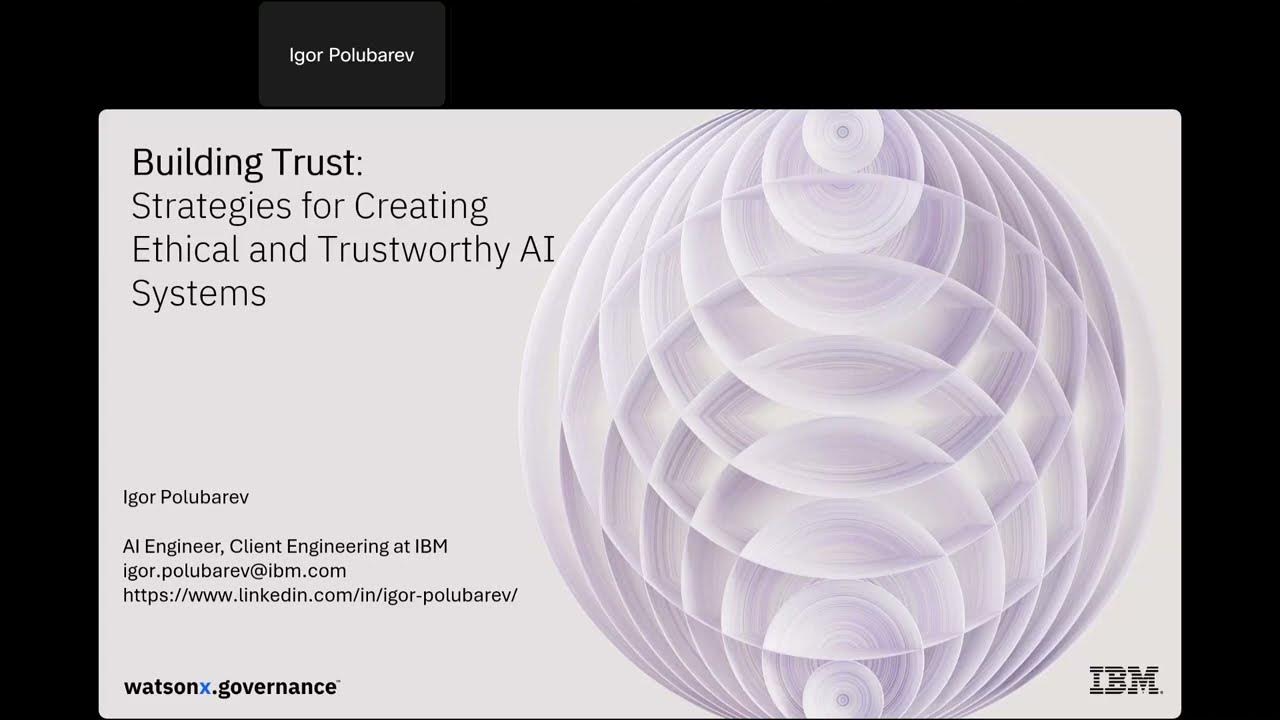
Building trust: Strategies for creating ethical and trustworthy AI systems

Understanding Generative AI, Its Impacts and Limitations
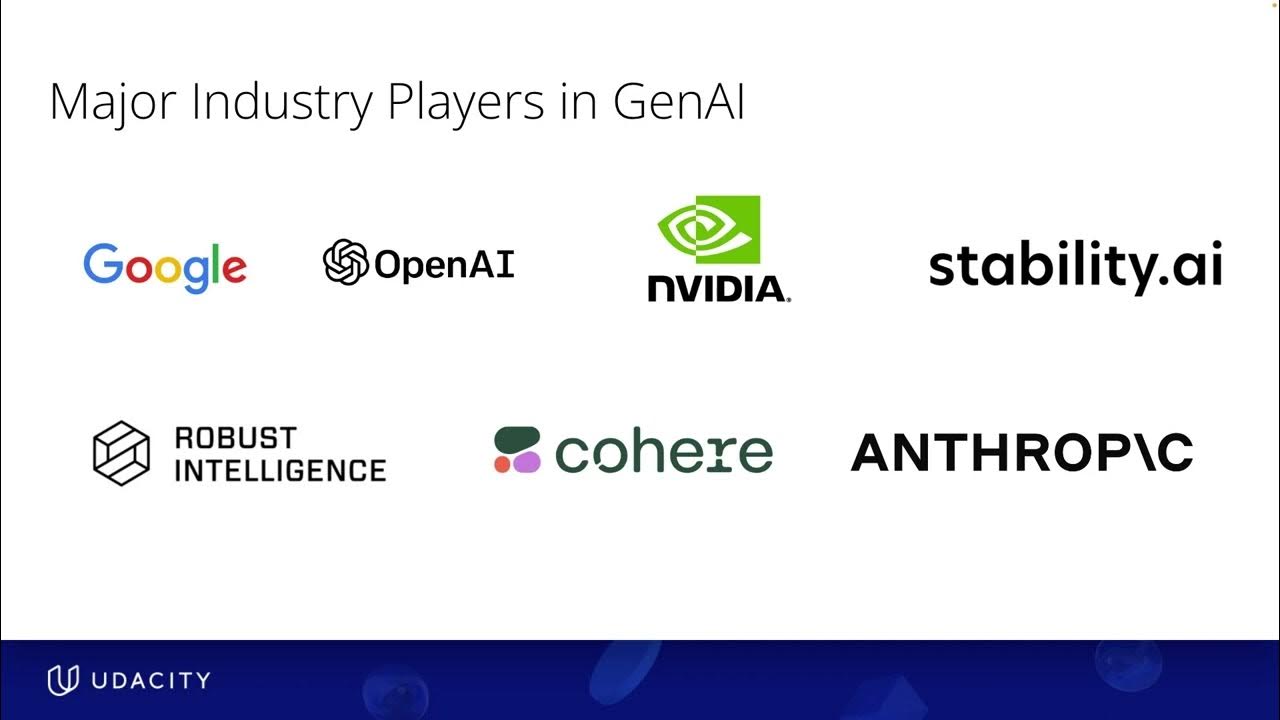
U8-04 V2 Wichtige Akteure der Branche V3
5.0 / 5 (0 votes)