Intelligence Artificielle: la fin du bullshit ? (AI News)
Summary
TLDRThe video critically explores the limitations of current AI models, particularly large language models (LLMs) like GPT-4. Despite the hype and massive investments, these models struggle with generalization, reasoning, and abstraction. Experts like Ilia Suskav and Yann LeCun argue that the pursuit of ever-larger models is misguided. Instead, they propose innovative approaches like Dino World Model and Test Time Training (TTT) to improve reasoning and adaptability. The video highlights the need for a paradigm shift in AI development to move beyond data scaling and towards models capable of true intelligence and planning.
Takeaways
- 😀 The scaling law, which posits that bigger models and more data automatically result in better performance, is increasingly being questioned by AI experts.
- 😀 Yan Lequin critiques the scaling approach and advocates for new AI methods that focus on reasoning, planning, and generalization, rather than just adding more data and computational power.
- 😀 OpenAI's new model, Orion, shows only modest improvements over GPT-4, reinforcing the idea that larger models do not always lead to significant advancements in performance.
- 😀 The myth of 'bigger is better' is exposed as models like GPT-4 and Orion struggle with generalization and reasoning tasks, leading to stagnation in AI development.
- 😀 LLMs (Large Language Models) excel at pattern matching but fail at generalizing to new or novel tasks, revealing their limitations in abstract reasoning and real-world problem solving.
- 😀 Predictions about the imminent arrival of superintelligence and AI breakthroughs often rely on hype and fearmongering rather than solid scientific evidence.
- 😀 Despite the huge investments and technological advancements, models like GPT-4 still struggle with tasks that require basic reasoning and logical thinking, such as simple word problems.
- 😀 The issue with LLMs is not just their size but their inability to truly understand or reason, which limits their usefulness for complex, novel tasks.
- 😀 Dino World Model, developed by Yan Lequin, presents a promising alternative by focusing on visual representations and predicting the outcomes of actions, which allows for better generalization in tasks like object manipulation and navigation.
- 😀 The future of AI likely lies in innovative approaches like Dino World Model, which emphasize adaptation and reasoning over brute-force scaling, offering new pathways for creating more autonomous and intelligent systems.
Q & A
What is the central critique of the scaling approach in AI models discussed in the transcript?
-The central critique is that the scaling approach, which involves increasing data and computational power, is reaching its limits. Despite adding more data and power, performance gains in tasks requiring generalization, reasoning, and complex problem-solving are minimal, highlighting the need for fundamentally new approaches in AI.
Who is Yan Lequin and what is his contribution to the AI field as mentioned in the transcript?
-Yan Lequin is a prominent AI researcher, known for his work on deep learning. In the transcript, he is highlighted for challenging the scaling approach to AI, proposing alternative methods like the Dino World model, which focuses on more intelligent, adaptable systems that can generalize better to new tasks.
What does the 'scaling law' in AI refer to and why is it considered a flawed approach?
-The 'scaling law' refers to the idea that simply increasing the size of AI models (through more data and computational power) will lead to improved intelligence. It is considered flawed because, despite its success in early AI breakthroughs, it has not resulted in substantial progress in tasks requiring reasoning, generalization, or understanding of new concepts.
How do current large language models (LLMs) like GPT-4 struggle with generalization?
-LLMs like GPT-4 excel at pattern matching and predicting text based on prior training data but struggle with generalizing beyond familiar patterns. They fail at tasks that require reasoning or applying knowledge to new situations, such as solving logic puzzles or understanding abstract concepts.
What example is given to demonstrate the limitations of LLMs in generalization?
-An example provided is the classic question: 'What is heavier, 10 kg of steel or 1 kg of feathers?' Early versions of LLMs answered incorrectly, indicating that these models fail to generalize or reason about simple, but conceptually important, relationships that humans can easily comprehend.
What is the ARC (Abstraction and Reasoning Corpus) and how does it test AI capabilities?
-The ARC is a benchmark designed to evaluate the ability of AI models to generalize and reason. Unlike traditional benchmarks that focus on memorization or pattern matching, ARC tests a model’s ability to solve novel problems that require real-time adaptation to unseen tasks. The results show that current LLMs significantly underperform compared to humans in these tasks.
What is the difference between 'test time training' (TTT) and 'test time compute' (TTC)?
-The key difference is that TTT adjusts the model's parameters during inference to adapt to a specific task, essentially 'retraining' the model in real-time, whereas TTC involves performing additional computations during testing without modifying the model itself. TTT has shown significant improvements in task performance over TTC.
What are the results of using test time training (TTT) on tasks like ARC, and how does it compare to traditional models?
-TTT has greatly improved performance on tasks like ARC, achieving up to 53% accuracy with an 8-billion-parameter model, significantly outperforming traditional models like GPT-3.5 and GPT-4, which score around 21% and 5-9%, respectively. This shows the effectiveness of TTT in enhancing model adaptation and generalization.
What is the Dino World model and how does it address some of the limitations of current AI models?
-The Dino World model, based on Dino V2, is an innovative approach that focuses on using visual representations and action anticipation to handle complex tasks like robot manipulation and navigation. Unlike traditional models, which rely on feedback from large amounts of data, Dino World model excels in generalizing to new tasks with higher accuracy.
How does Dino World model outperform traditional reinforcement learning approaches?
-Dino World model outperforms traditional reinforcement learning approaches by focusing on evolving visual representations rather than relying on large feedback loops. This allows it to generalize across various tasks, like manipulating different objects or navigating through unfamiliar environments, with greater accuracy and adaptability.
Outlines
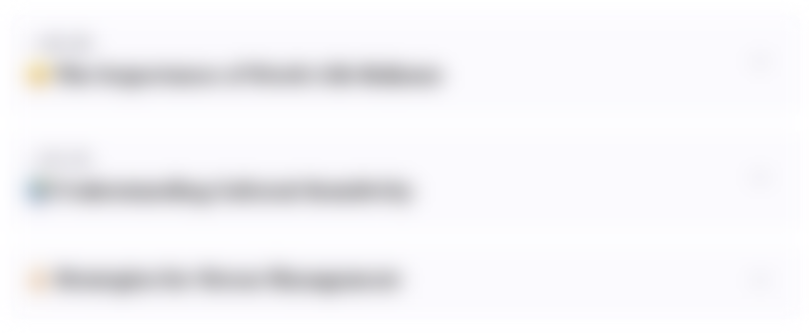
Этот раздел доступен только подписчикам платных тарифов. Пожалуйста, перейдите на платный тариф для доступа.
Перейти на платный тарифMindmap
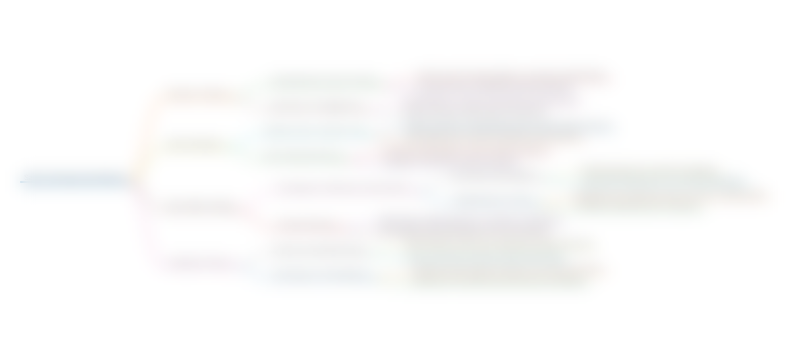
Этот раздел доступен только подписчикам платных тарифов. Пожалуйста, перейдите на платный тариф для доступа.
Перейти на платный тарифKeywords
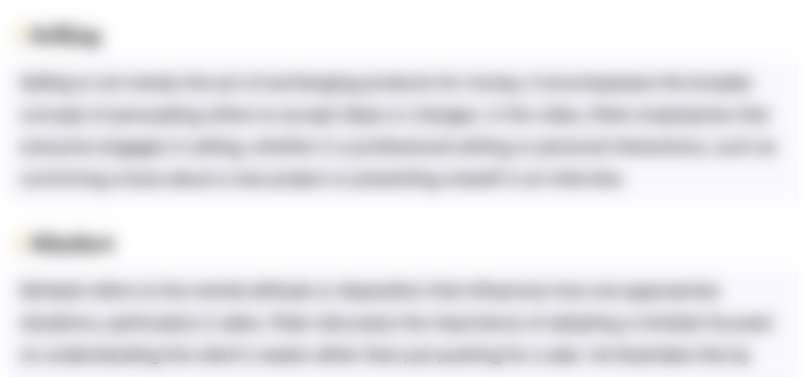
Этот раздел доступен только подписчикам платных тарифов. Пожалуйста, перейдите на платный тариф для доступа.
Перейти на платный тарифHighlights
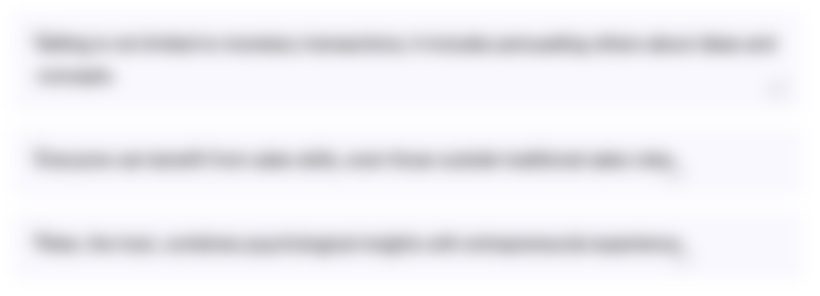
Этот раздел доступен только подписчикам платных тарифов. Пожалуйста, перейдите на платный тариф для доступа.
Перейти на платный тарифTranscripts
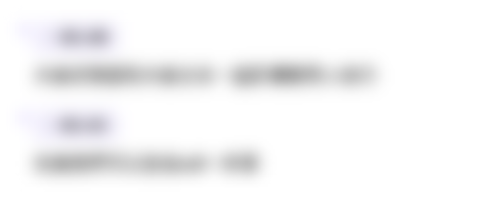
Этот раздел доступен только подписчикам платных тарифов. Пожалуйста, перейдите на платный тариф для доступа.
Перейти на платный тарифПосмотреть больше похожих видео

So Google's Research Just Exposed OpenAI's Secrets (OpenAI o1-Exposed)
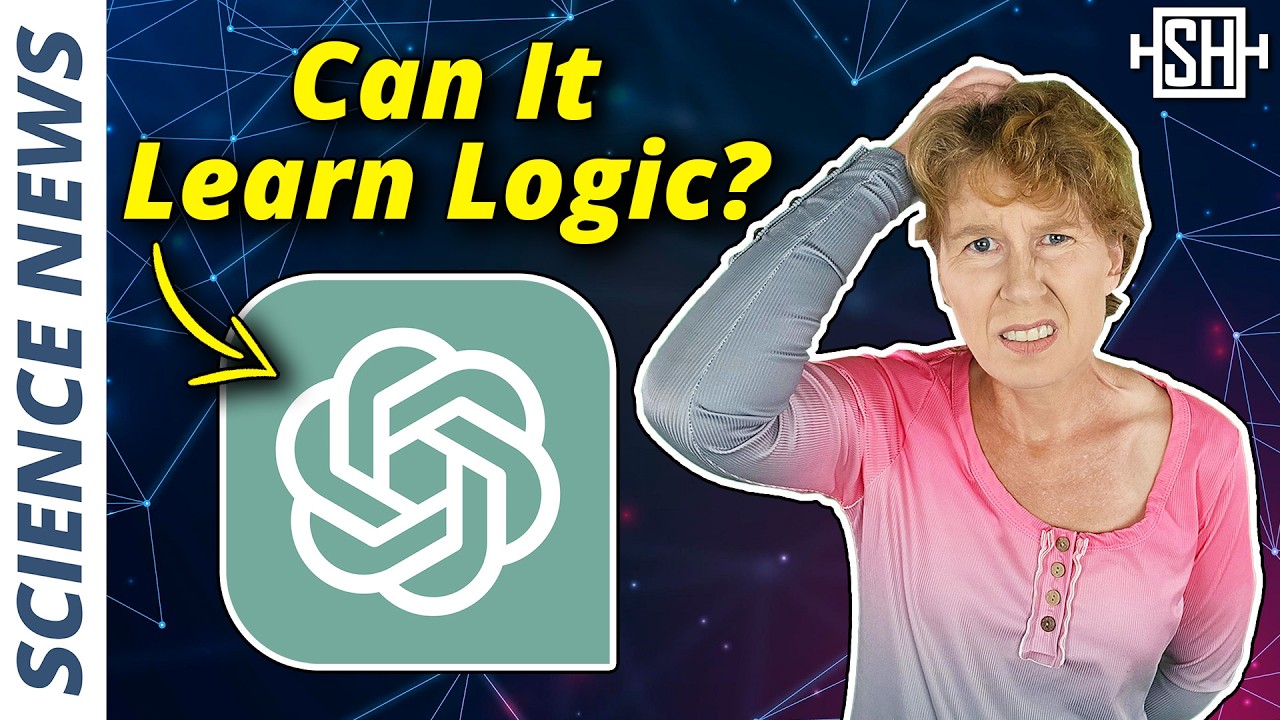
AI Can’t Reason. Should It Drive Cars?
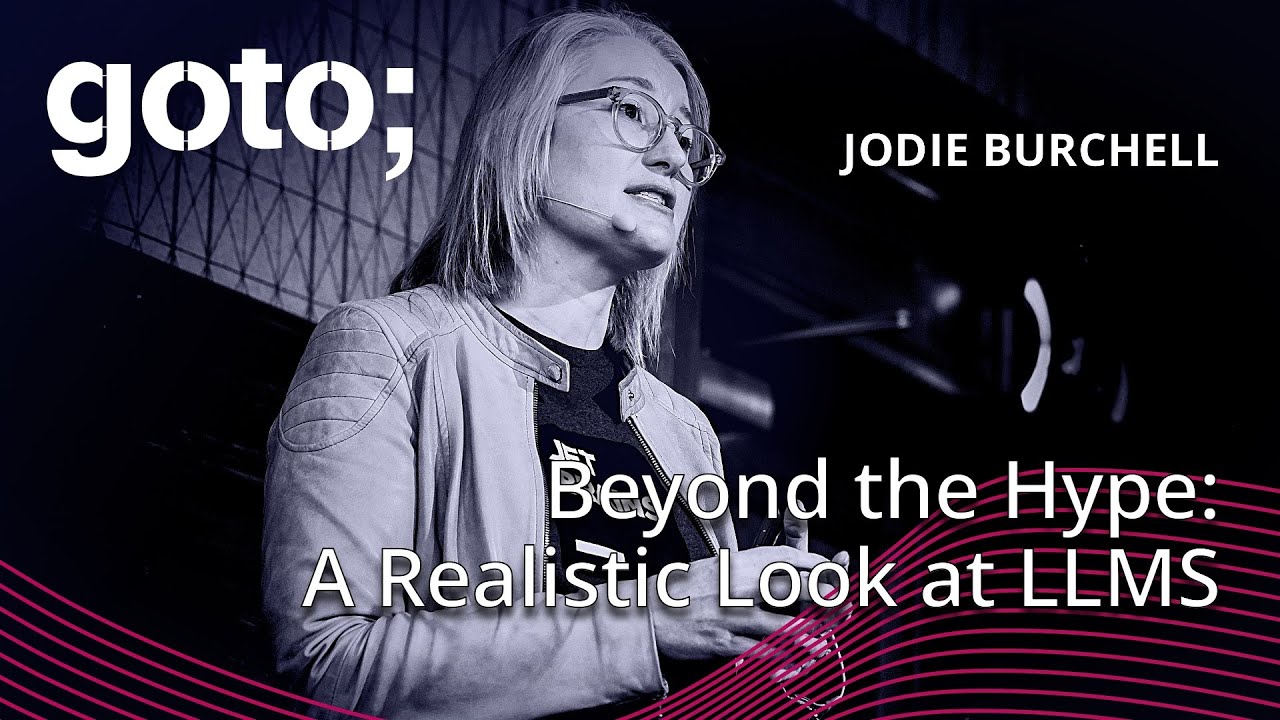
Beyond the Hype: A Realistic Look at Large Language Models • Jodie Burchell • GOTO 2024
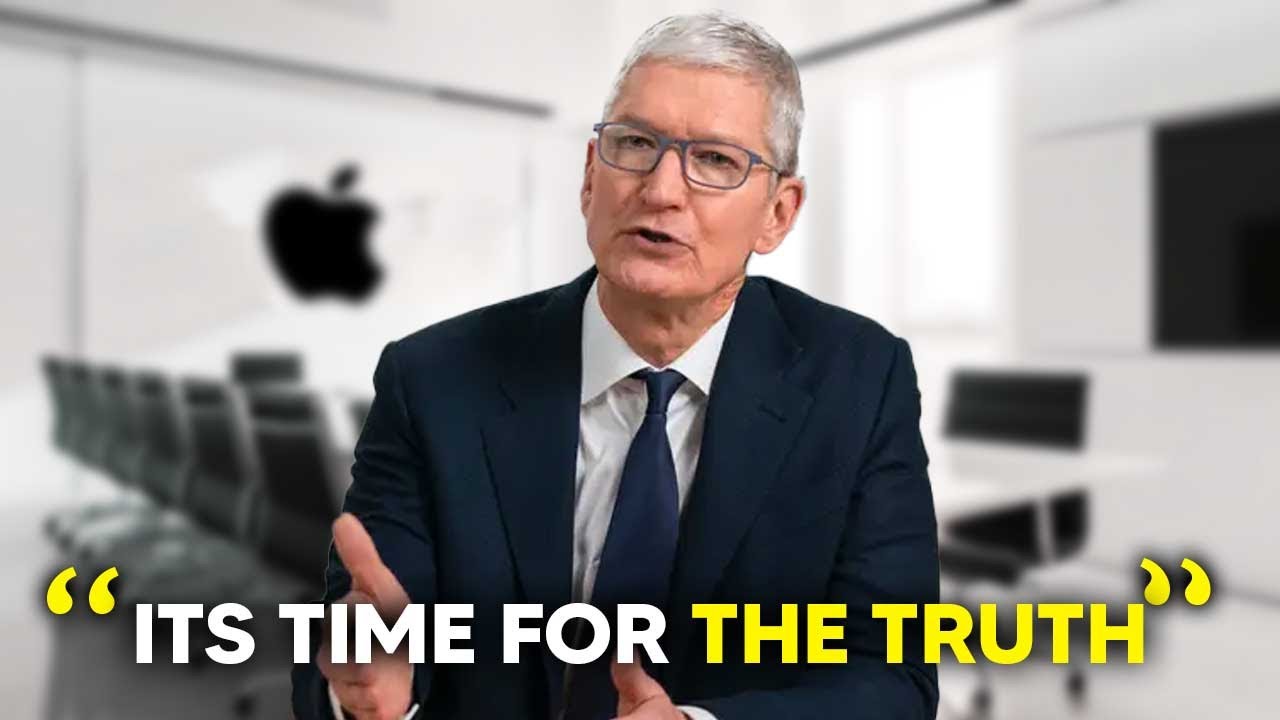
Apple DROPS AI BOMBSHELL: LLMS CANNOT Reason

Are Hallucinations Popping the AI Bubble?

A basic introduction to LLM | Ideas behind ChatGPT
5.0 / 5 (0 votes)