Why Does YouTube Keep Recommending You Videos You’ve Already watched?
Summary
TLDRIn this video, the creator delves into the mysteries of YouTube's recommendation algorithm, exploring how it can sometimes recommend videos the user has already watched. The script traces the creator's journey of trying to fix their YouTube algorithm, discussing various approaches that don’t seem to work. The narrative then delves into the inner workings of recommendation systems, from collaborative filtering to matrix factorization, explaining how user preferences shape recommendations. The video concludes with a thought-provoking question about whether it's worth it for users to lose control over their recommendations in exchange for tailored content, despite the complexities and occasional issues with the system.
Takeaways
- 😀 YouTube's recommendation system can get stuck in a loop, showing you videos you've already watched, which can be frustrating.
- 😀 Attempts to fix the issue by clearing or resetting your YouTube history often fail to resolve the problem permanently.
- 😀 The recommendation system relies heavily on your *recent* watch history rather than your entire history, which can lead to repeated recommendations.
- 😀 One of the main issues with fixing this problem is that YouTube uses complex algorithms like collaborative filtering and matrix factorization, which make it difficult to identify the source of the issue.
- 😀 Collaborative filtering matches you with content based on the preferences of other users who share similar tastes, rather than just matching content to your personal watch history.
- 😀 While collaborative filtering improves recommendations by expanding your content variety, it can also lead to irrelevant suggestions.
- 😀 The problem with algorithmic recommendations is that it often becomes harder to understand why certain videos are being suggested, especially when the system no longer provides clear explanations.
- 😀 Privacy concerns arise from the fact that YouTube collects massive amounts of data on your watch behavior, which it uses to tailor content recommendations.
- 😀 Resetting your YouTube history or account doesn’t always help, as the system continues to pull from your past interactions and user-based recommendations.
- 😀 YouTube's algorithm uses hundreds of different data points to make its recommendations, from your watch history to video characteristics and user preferences.
- 😀 The big question raised is whether the benefits of personalized recommendations, despite their flaws, are worth the trade-off of privacy and data collection.
Q & A
What is the main issue the user is facing with YouTube's algorithm?
-The user is frustrated with YouTube recommending videos they have already watched, which clutters their homepage with repetitive content.
What did the user do to try and fix the problem with their YouTube recommendations?
-The user tried removing offending videos from their watch history and resetting their entire account, but neither solution worked to fix the problem.
Why is it difficult for the user to fix the problem by clearing their watch history?
-Clearing the watch history is time-consuming because the user would have to go through months or years of videos to find and remove the ones they’ve watched multiple times, and even then, it's not guaranteed to resolve the issue.
What is the significance of Hulu's breakthrough related to recommendation systems?
-Hulu's breakthrough was finding that people are more likely to click and watch videos based on their most recent watch history rather than their overall viewing history, which influences recommendation algorithms.
What is the key difference between item-based filtering and collaborative filtering in recommendation systems?
-Item-based filtering matches videos with users based on characteristics of the items, while collaborative filtering matches users with videos based on shared preferences from other users.
Why is collaborative filtering dominant in recommendation systems?
-Collaborative filtering is dominant because it allows for more effective recommendations by matching users with content based on the preferences of similar users, making it easier to predict what someone might like.
What is a potential downside of YouTube's recommendation system, according to the user?
-A downside is that the system relies heavily on implicit feedback (what users watch and how often), making it difficult for users to explicitly control or correct their recommendations.
How do hybrid systems work in the context of recommendation algorithms?
-Hybrid systems combine both item-based filtering and collaborative filtering to provide more personalized and accurate recommendations, which is likely what platforms like YouTube use given their vast resources.
What is matrix factorization, and how is it used in recommendation systems?
-Matrix factorization is a technique used to break down data into two matrices—one representing the items (like videos) and one representing user preferences. It helps match users with content by analyzing patterns in both sets of data.
What is the impact of the lack of explanations for recommendations on YouTube?
-The lack of explanations makes it harder for users to understand why certain videos are being recommended to them, which can be frustrating and complicates efforts to correct issues with the algorithm.
Outlines
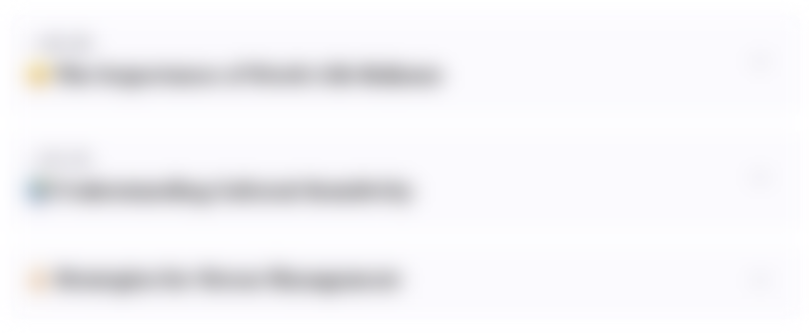
Этот раздел доступен только подписчикам платных тарифов. Пожалуйста, перейдите на платный тариф для доступа.
Перейти на платный тарифMindmap
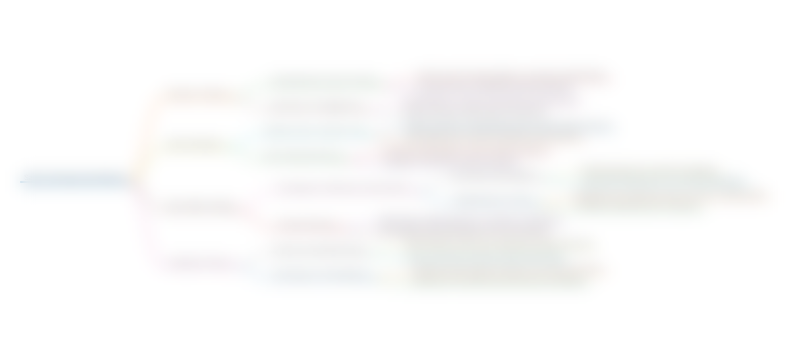
Этот раздел доступен только подписчикам платных тарифов. Пожалуйста, перейдите на платный тариф для доступа.
Перейти на платный тарифKeywords
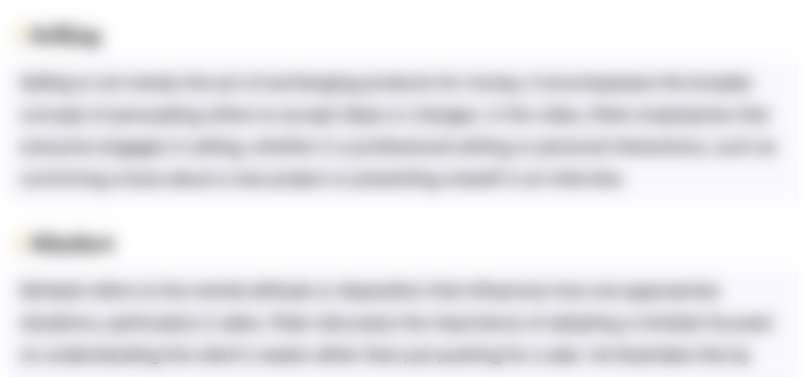
Этот раздел доступен только подписчикам платных тарифов. Пожалуйста, перейдите на платный тариф для доступа.
Перейти на платный тарифHighlights
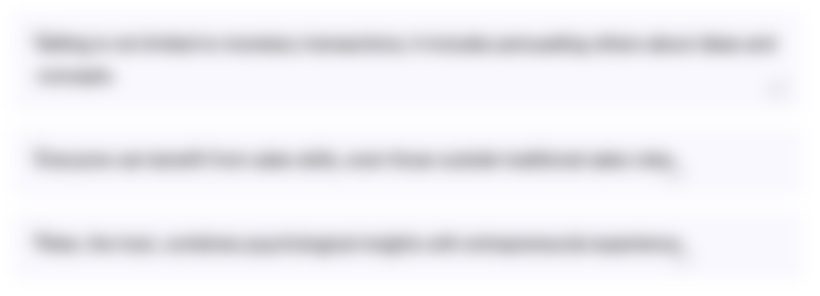
Этот раздел доступен только подписчикам платных тарифов. Пожалуйста, перейдите на платный тариф для доступа.
Перейти на платный тарифTranscripts
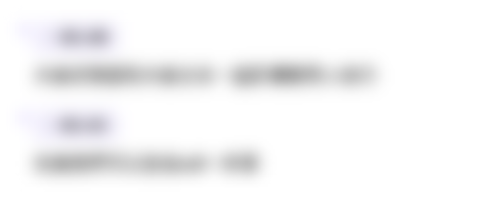
Этот раздел доступен только подписчикам платных тарифов. Пожалуйста, перейдите на платный тариф для доступа.
Перейти на платный тарифПосмотреть больше похожих видео
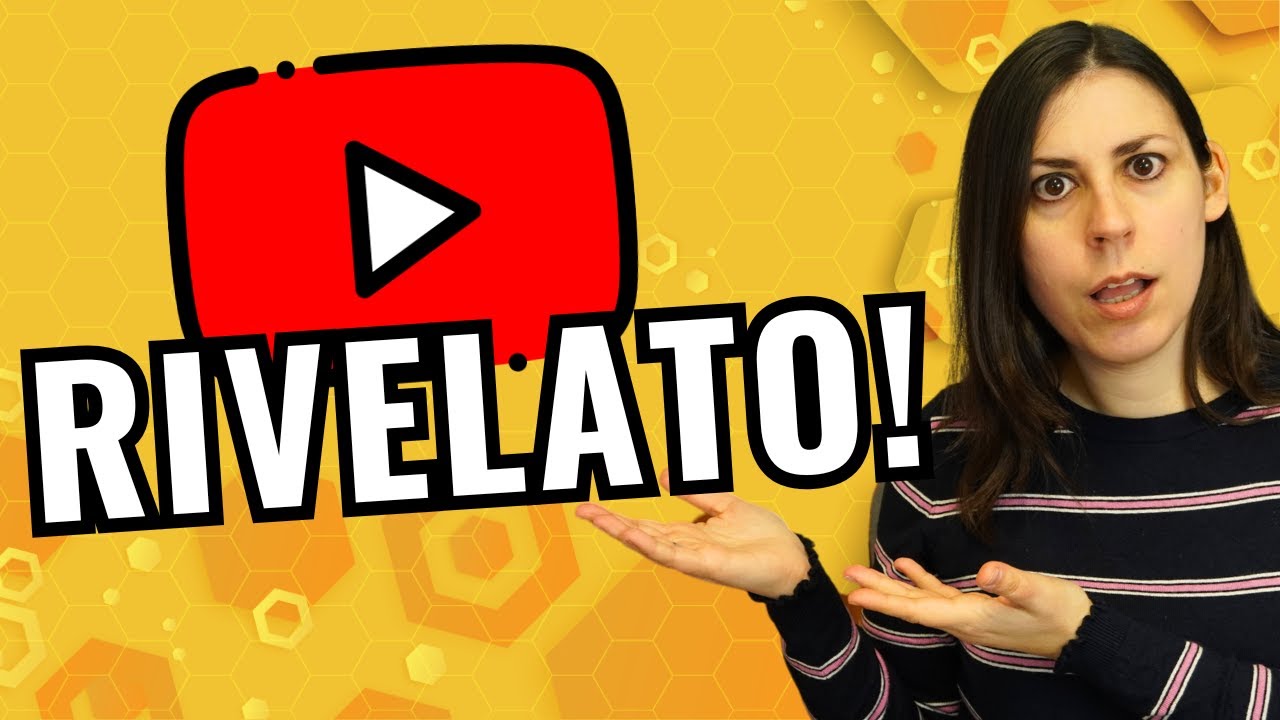
Ecco il SEGRETO dell'ALGORITMO di Youtube!
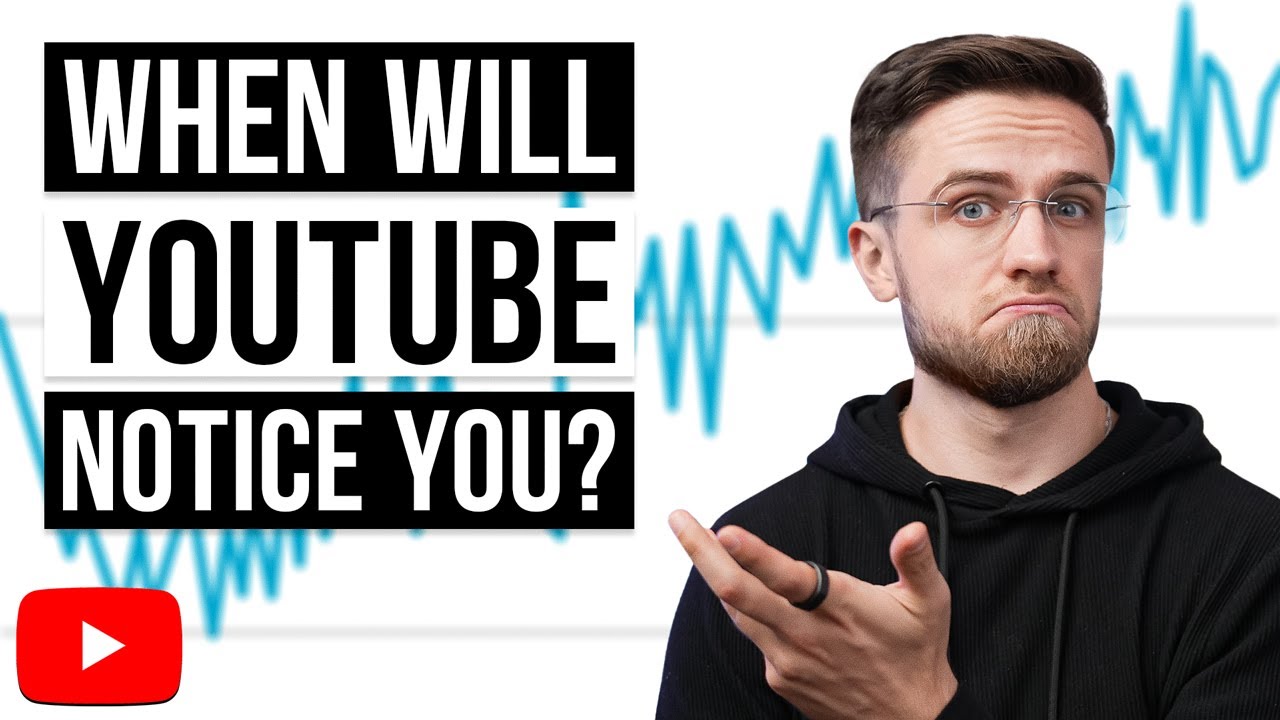
When Does YouTube Start Promoting Small Channels? EXPLAINED!
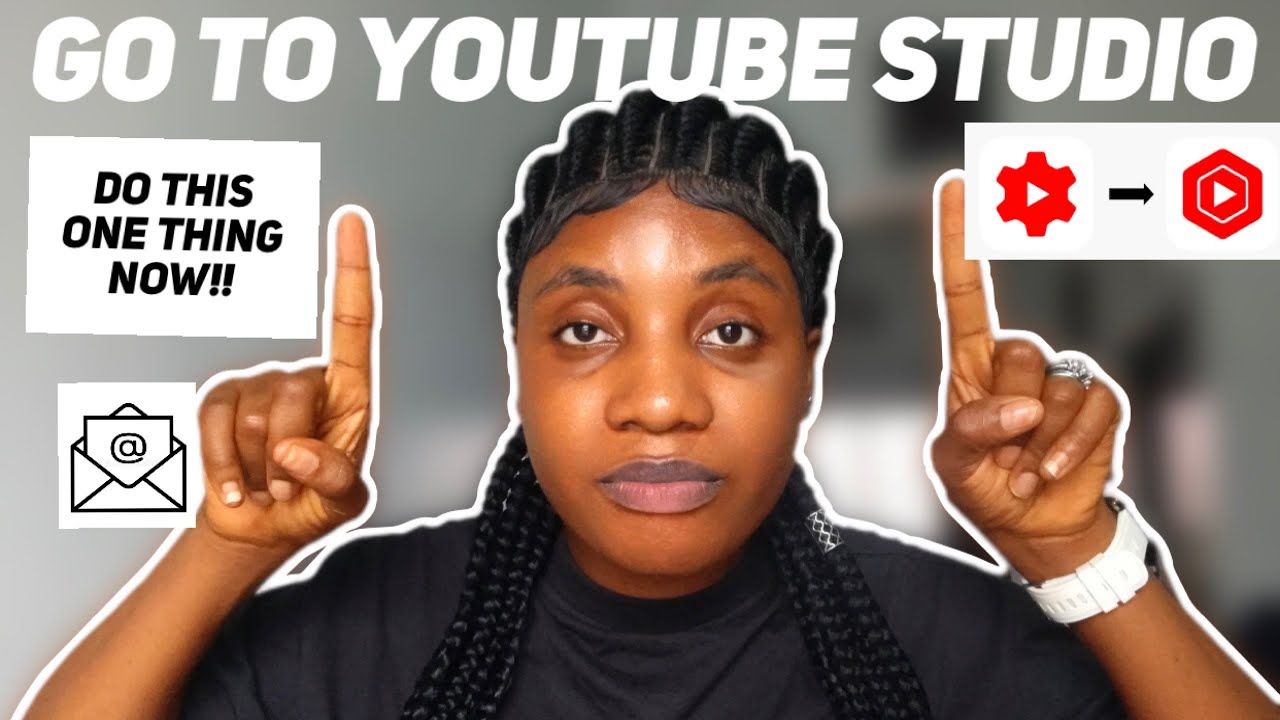
Updates: If YouTube is not recommending your videos, Turn on this feature now!!
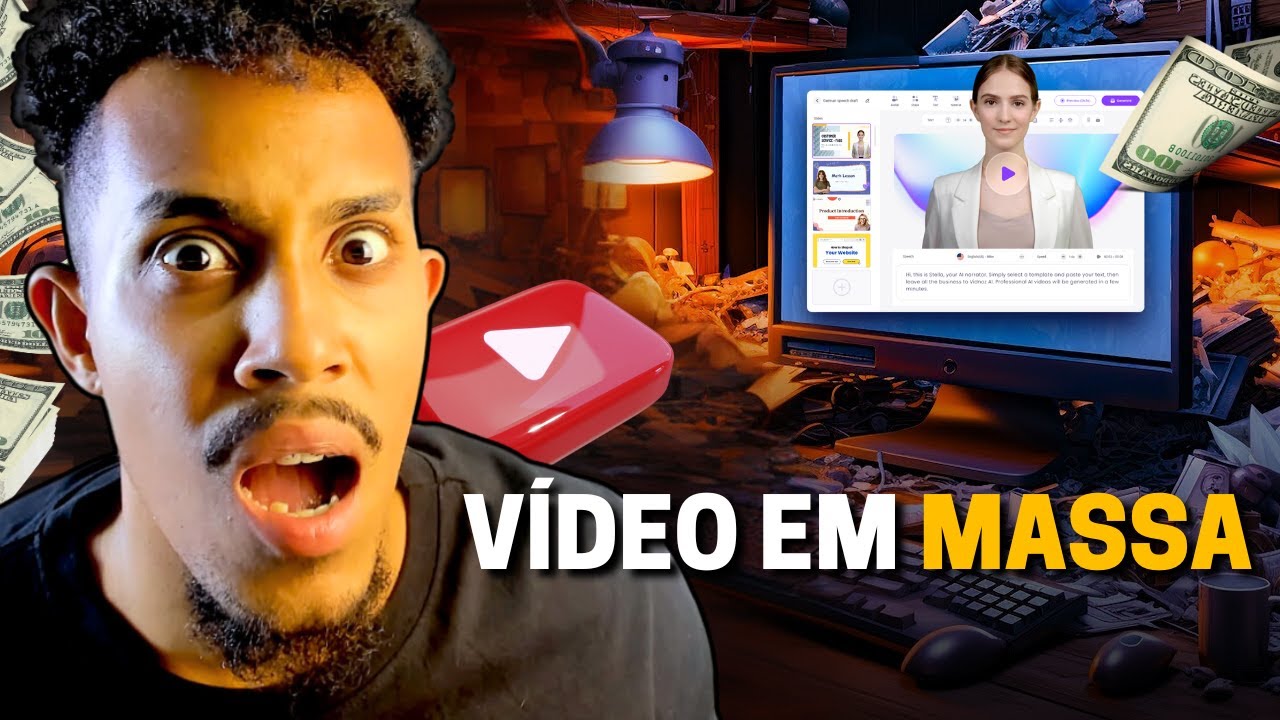
IA que faz voce ganha 100 mil por mes com YOUTUBE sem fazer nada

How the YouTube Algorithm Works in 2024-25: EXPLAINED (Get More Views)

YouTube Shorts Algorithm 2024 EXPLAINED (Get More Views)
5.0 / 5 (0 votes)