Accelerate the value of generative AI with three secret ingredients
Summary
TLDREl video ofrece una visión detallada sobre cómo las organizaciones pueden aprovechar el poder de los datos y la inteligencia artificial (IA) para transformar sus negocios. Se discuten tres componentes clave para el éxito en la implementación de soluciones generativas de IA: patrones, perfiles de usuario y controles. Los patrones se refieren a componentes comunes que promueven la reutilización y la escala, los perfiles de usuario se centran en los resultados de negocio y cómo los individuos obtienen valor de la tecnología, y los controles son esenciales para garantizar la seguridad y la confianza en la solución. Además, se exploran técnicas para la selección de modelos de IA, la ingeniería de prompts y la gestión de sesiones para mejorar la calidad de los resultados. La presentación incluye una demostración de cómo PWC ha desarrollado una plataforma para facilitar la experimentación rápida y la personalización basada en perfiles de usuario, con un enfoque en el control y la gobernanza para garantizar la seguridad y el rendimiento de las soluciones de IA a gran escala.
Takeaways
- 📈 **Estrategias de patrones**: Los patrones son componentes comunes que impulsan la reutilización y la escala, disminuyendo costos y evitando la duplicación de esfuerzos en la organización.
- 🧑 **Personas clave**: El enfoque en los resultados de negocio y en los individuos que utilizan la tecnología es fundamental para integrar y obtener el máximo valor de las soluciones generativas de IA.
- 🛡 **Controles de seguridad**: La seguridad y la conformidad son cruciales para garantizar la confianza en la implementación de soluciones de IA, requiriendo un control sistemático para auditorías y garantizar la calidad de los resultados.
- 🔍 **Búsqueda de información**: La capacidad de generar respuestas a partir de datos estructurados y no estructurados es una de las aplicaciones destacadas de la IA generativa.
- 🤖 **Evolución de bots**: Los bots de servicio al cliente y la capacidad de responder rápidamente a consultas son ejemplos de cómo la IA generativa puede mejorar la productividad y la eficiencia.
- 🌐 **Transformación de idiomas**: La traducción y el procesamiento de lenguaje natural son áreas en las que la IA generativa está teniendo un impacto significativo, mejorando la productividad del desarrollador y la comunicación.
- 📚 **Creación de contenido**: La generación de contenido de marketing, documentos, código e imágenes es una aplicación en crecimiento donde la IA demuestra su versatilidad.
- 🔢 **Modelos y tamaños**: La elección del modelo de IA correcto depende del caso de uso y del tamaño de los datos, lo que afecta el rendimiento y la precisión de la solución.
- 📈 **Escala y producción**: El desafío principal para las empresas es la capacidad para escalar y poner en producción casos de uso exitosos de IA generativa a lo largo de múltiples funciones empresariales.
- 👥 **Diversidad de roles**: La colaboración entre múltiples roles dentro de una organización es esencial para la implementación exitosa y escalable de soluciones de IA generativa.
- 📝 **Gestión de cambios**: La educación y el acompañamiento durante la implementación de nuevas tecnologías son clave para su adopción y éxito a gran escala.
Q & A
¿Quién es Sora Mohanti y qué papel desempeña en PWC?
-Sora Mohanti es socio en PWC y se enfoca en la práctica de datos y AI de la empresa, pasando la mayor parte de su tiempo ayudando a organizaciones a desbloquear el poder de los datos y la inteligencia artificial para transformar sus negocios.
¿Qué áreas de la empresa están experimentando una transformación con la ayuda de la IA?
-La transformación está ocurriendo en múltiples funciones empresariales, donde las soluciones de IA están siendo implementadas con éxito y luego desafiantes a escalar y producirse para aprovechar su valor y medir el retorno de inversión (ROI).
¿Cuáles son los tres ingredientes clave mencionados para soluciones generativas de IA?
-Los tres ingredientes clave son patrones, personas y controles. Los patrones son componentes comunes que impulsan la reutilización y la escala; las personas se enfocan en los resultados de negocio y en qué obtienen de la solución; y los controles aseguran la seguridad y la confianza en la implementación de la tecnología.
¿Cómo son diferentes los patrones de manejo de datos no estructurados y estructurados?
-Los patrones de manejo de datos no estructurados y estructurados difieren en cómo se interactúa con ellos. Mientras que los datos estructurados se convierten en comandos SQL para interactuar con bases de datos, los datos no estructurados requieren un enfoque diferente para funcionar eficazmente.
¿Qué es un modelo LLM y cómo se relaciona con la experiencia del usuario?
-Un modelo LLM (Large Language Model) es un modelo de lenguaje grande que se utiliza en la generación de texto y comprensión. La experiencia del usuario está enfocada en cómo se les proporciona contexto y cómo se les permite interactuar con el modelo para lograr los resultados deseados.
¿Por qué es importante la selección del modelo LLM adecuado para una tarea específica?
-La selección adecuada del modelo LLM es crucial porque determina la eficacia de la solución generativa de IA en un caso de uso específico. Un modelo no adecuado puede generar respuestas vagas o no precisas, lo que afecta negativamente la calidad y confianza en los resultados.
¿Qué es un 'prompting template' y cómo ayuda en la formación de usuarios?
-Un 'prompting template' es una herramienta que guía a los usuarios a entender para quién están formulando un prompt, qué información buscan, quién será el receptor de la información y qué restricciones deben considerar en su prompt. Esto ayuda a los usuarios a ser más efectivos en la obtención de la información requerida y a ser más precisos en sus solicitudes a los modelos de IA.
¿Cómo se aborda la gobernanza y el control de riesgos al implementar soluciones de IA generativa a gran escala?
-La gobernanza y el control de riesgos se abordan estableciendo un mecanismo de gobernanza que incluya roles y responsabilidades definidos, políticas de uso estrictas, capacitación y un equipo responsable de regular todas las implementaciones dentro de la organización. Esto ayuda a equilibrar los riesgos y el ROI al mismo tiempo que se promueve la innovación.
¿Qué es la 'chunking' y por qué es importante en la calidad de los resultados de los modelos de IA?
-La 'chunking' se refiere a cómo se divide la información para su procesamiento por los modelos de IA. Es importante porque afecta directamente la calidad de los resultados, ya que la forma en que se 'chunkea' los datos puede influir en la precisión y la coherencia de las respuestas generadas por el modelo.
¿Cómo se implementan los controles de seguridad y calidad en la plataforma demostrada por Brad Foster?
-Los controles de seguridad y calidad se implementan a través de opciones configurables por el usuario, como el tamaño de chunking, la región de ejecución, los límites de tokens, el umbral de seguridad y la selección del modelo de IA. Esto permite a los usuarios experimentar y configurar iterativamente sin la necesidad de programación constante y asegura que se mantengan estándares de seguridad y calidad en los procesos.
¿Cómo se puede mejorar la calidad de los resultados en las soluciones de IA generativa?
-La calidad de los resultados se puede mejorar a través del modelado de versiones, pruebas de regresión, el control de la aleatoriedad en la selección de respuestas, la gestión de sesiones de chat y la actualización y control de versiones de datos. Estos aspectos son cruciales para mantener la precisión y confianza en las soluciones de IA a gran escala.
Outlines
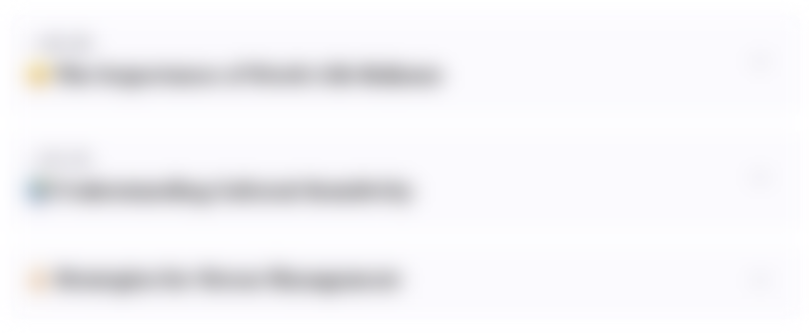
Этот раздел доступен только подписчикам платных тарифов. Пожалуйста, перейдите на платный тариф для доступа.
Перейти на платный тарифMindmap
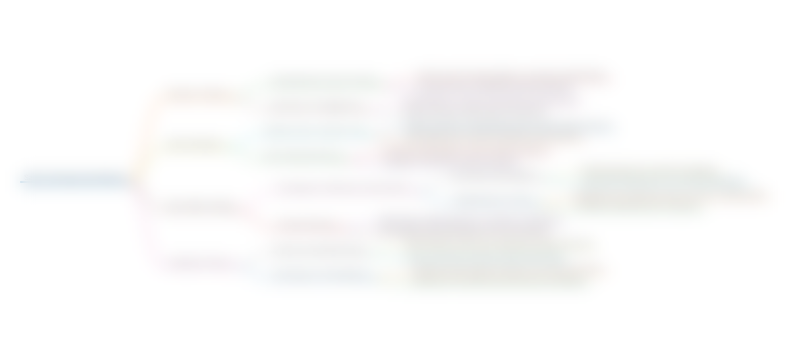
Этот раздел доступен только подписчикам платных тарифов. Пожалуйста, перейдите на платный тариф для доступа.
Перейти на платный тарифKeywords
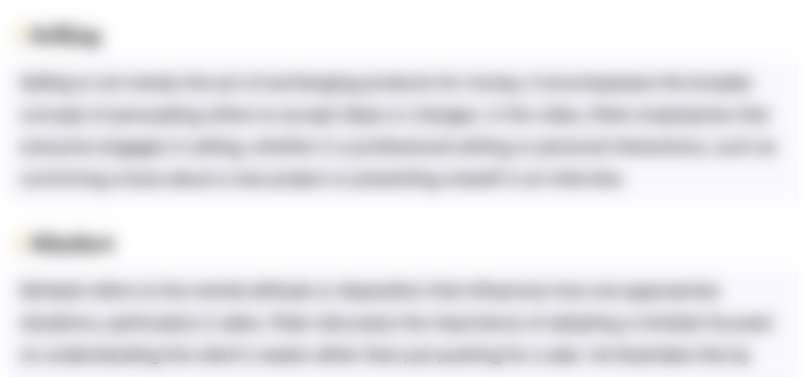
Этот раздел доступен только подписчикам платных тарифов. Пожалуйста, перейдите на платный тариф для доступа.
Перейти на платный тарифHighlights
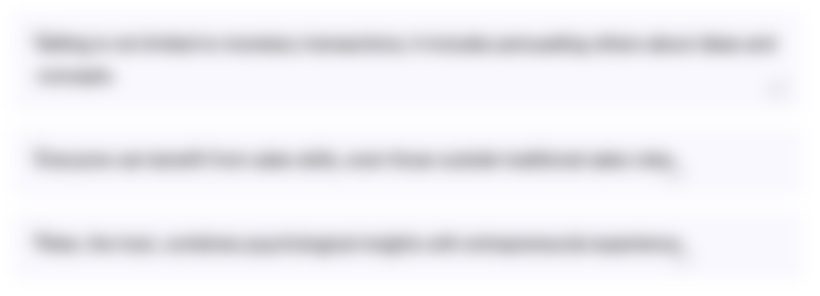
Этот раздел доступен только подписчикам платных тарифов. Пожалуйста, перейдите на платный тариф для доступа.
Перейти на платный тарифTranscripts
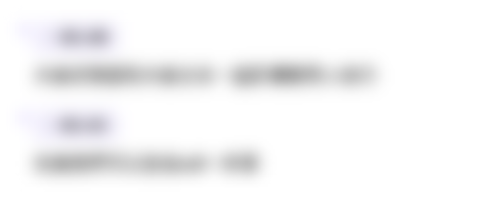
Этот раздел доступен только подписчикам платных тарифов. Пожалуйста, перейдите на платный тариф для доступа.
Перейти на платный тарифПосмотреть больше похожих видео
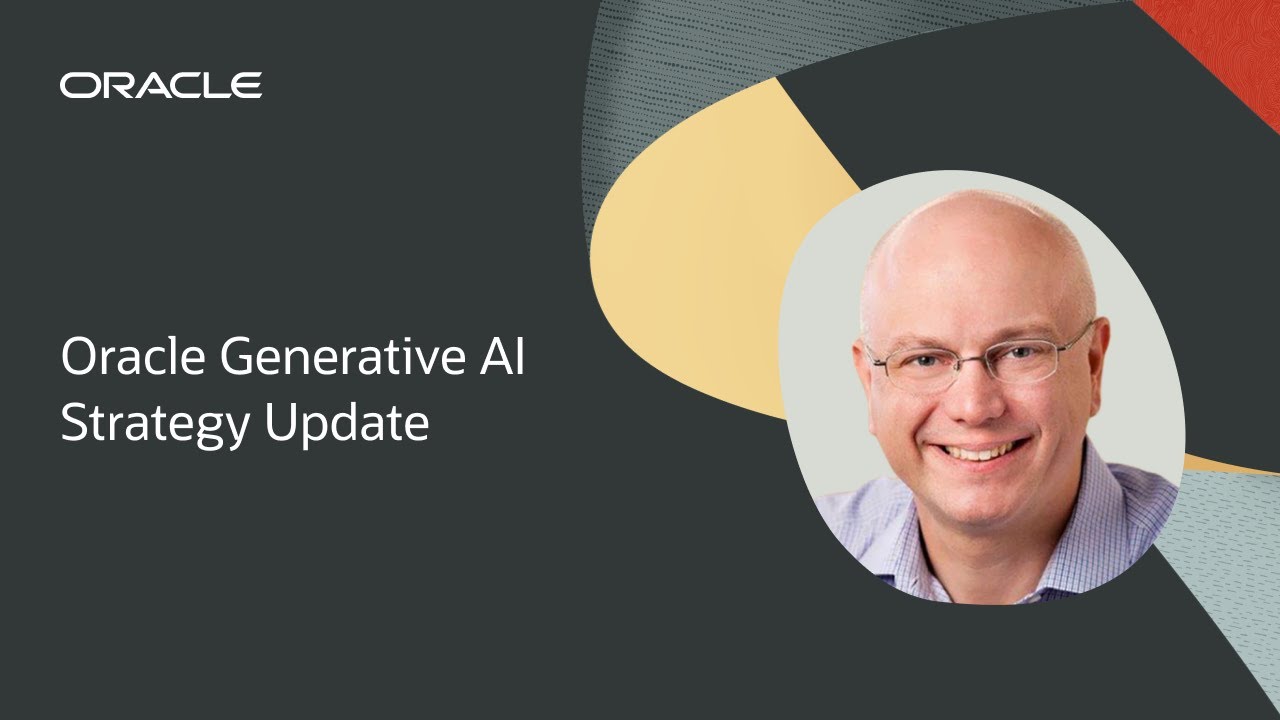
Oracle Generative AI Strategy Update
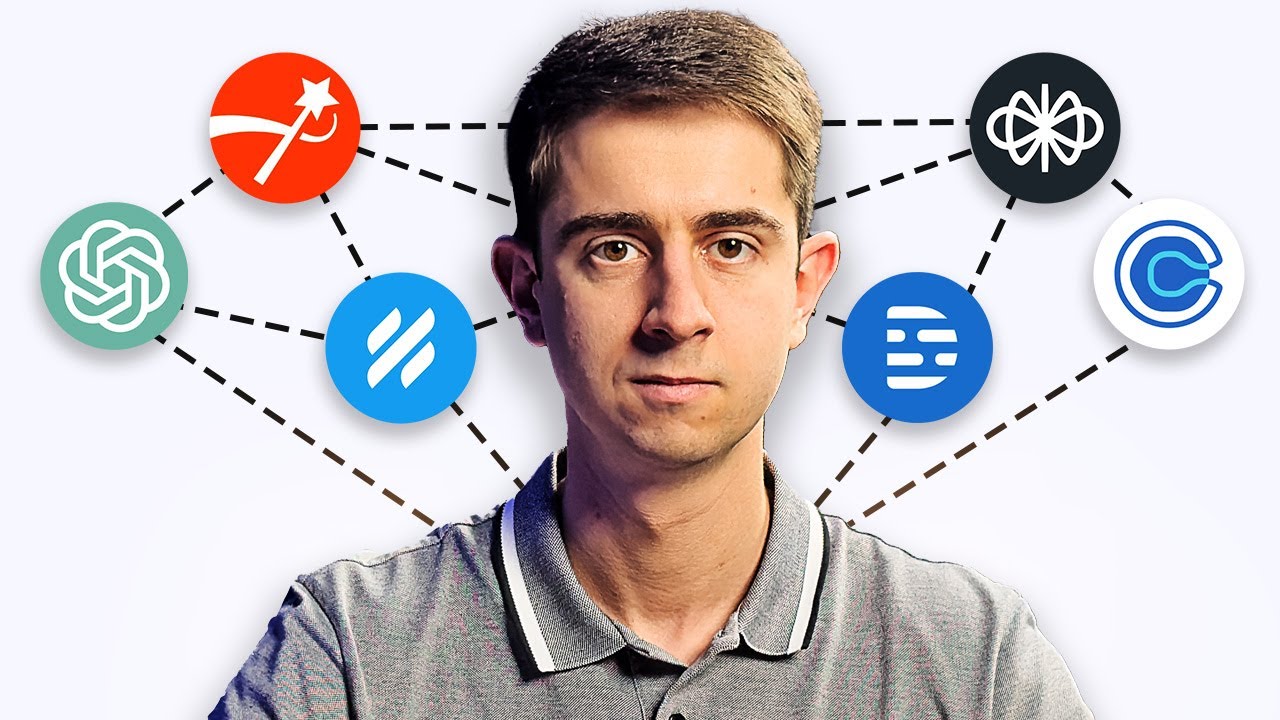
7 herramientas de Inteligencia Artificial para crecer tu negocio
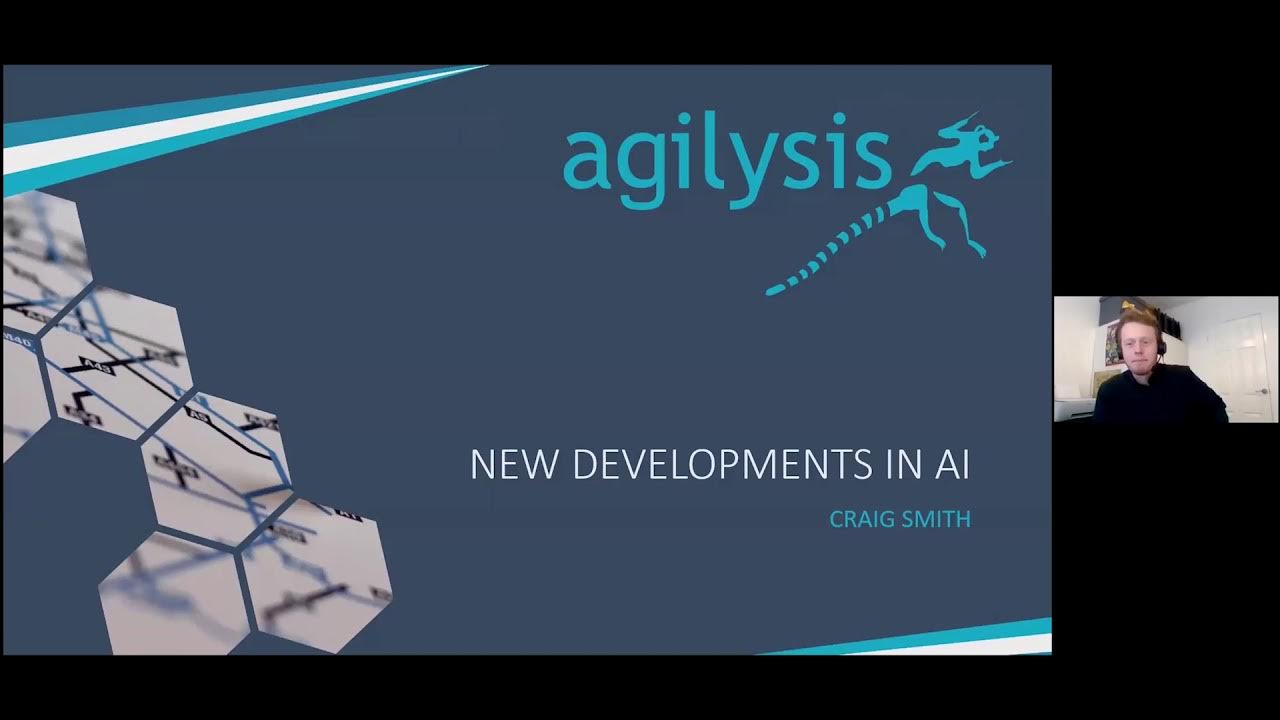
Road Danger Prediction: Classic Models, AI Models and Data Challenges
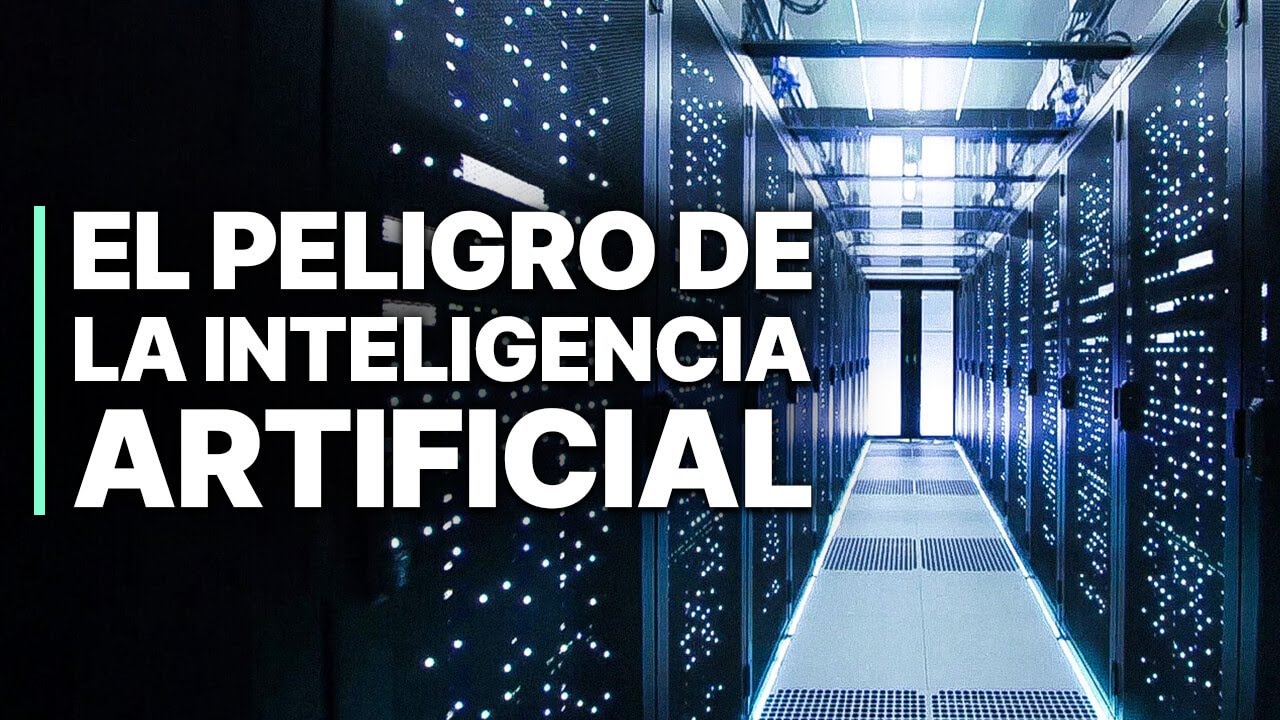
El Peligro de la Inteligencia Artificial | Tecnología de miedo | Documental
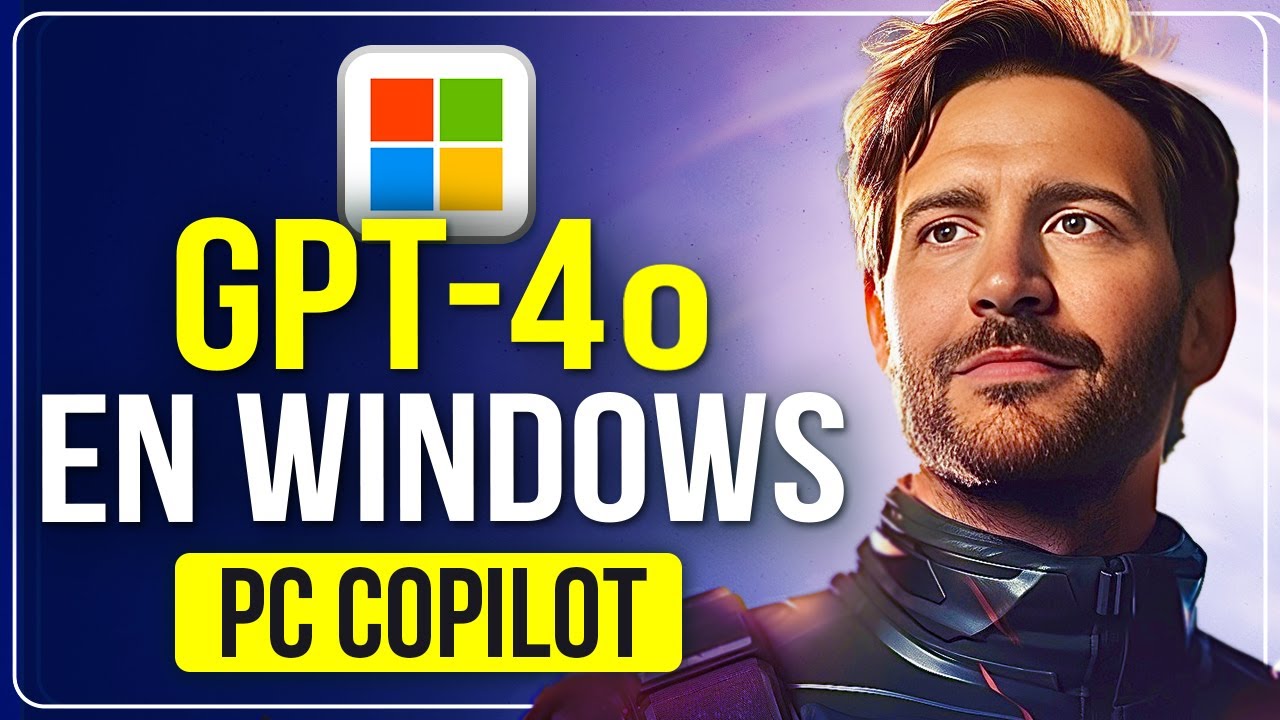
Copilot llega al PC: ¡GPT-4o + IA en local + NUEVO HARDWARE! 🤯 Microsoft BUILD
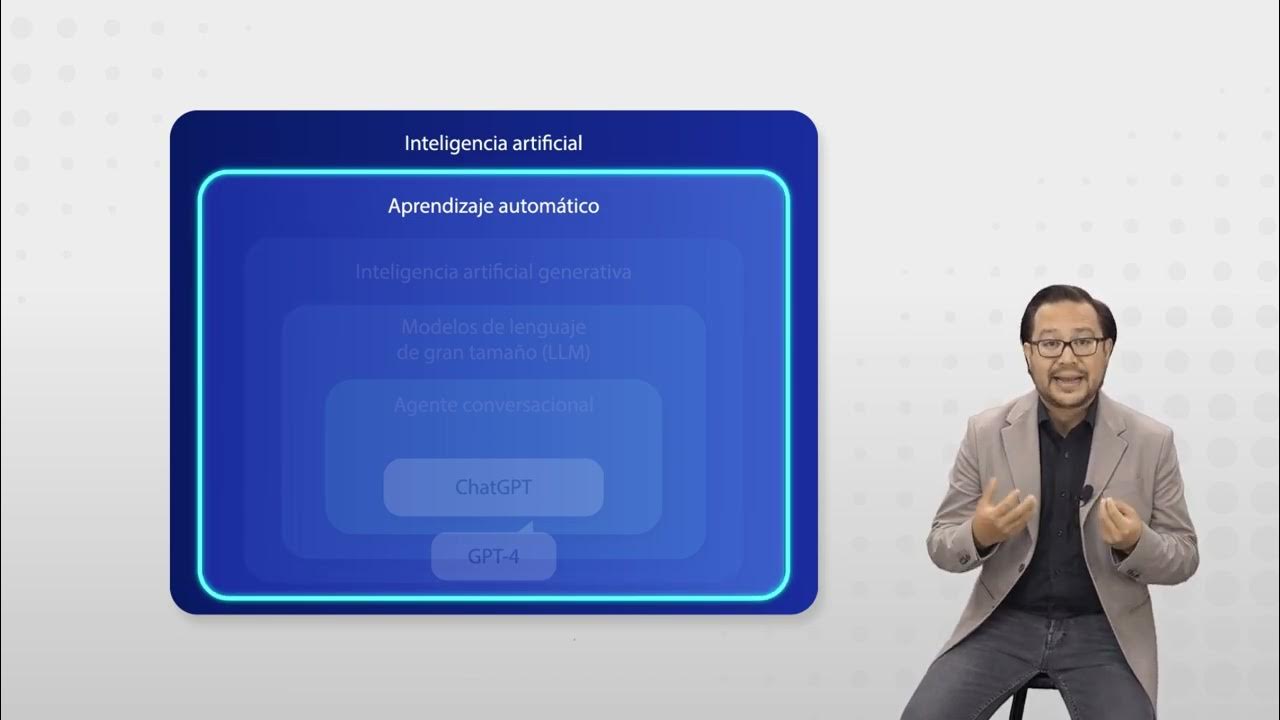
Qué es Inteligencia Artificial Generativa?
5.0 / 5 (0 votes)