Debunking AI: Tech Industry Secrets Exposed!
Summary
TLDRIn this discussion, the guests delve into the current state of AI and its impact on various professions, particularly software development and law. They address the limitations of AI in analyzing complex data like medical records and the 'garbage in, garbage out' issue. The conversation also explores AI's role in content creation, from writing code to generating art and its potential to disrupt traditional jobs. The guests highlight the importance of critical thinking when adopting AI tools and the need for human oversight to ensure accuracy and ethical considerations.
Takeaways
- 🤖 Current AI and Large Language Models (LLMs) are not recommended for critical tasks like medical record analysis due to the risk of inaccurate results.
- 🧐 AI-generated content can be creative but is also susceptible to 'hallucinations,' where it invents information that doesn't exist.
- 👨💻 For experienced developers, traditional search methods like Google are often faster and more reliable than using LLMs to write code.
- 📈 The distribution of software development talent shows that top developers are significantly more productive, and tools like LLMs may be more applicable to novices.
- 🎨 AI is being integrated into various creative fields like music and art, raising questions about authenticity and originality.
- 🔍 AI tools can quickly process large amounts of data, which can be useful for tasks like transcription, but their accuracy in critical applications is still questionable.
- 📚 AI is not a replacement for human creativity and expertise; it is a tool that can be used to augment human efforts.
- 🤔 There is a cultural and political bias in AI training data, which can lead to problematic outputs if not properly managed.
- 💬 AI can produce varied and entertaining content, but its ability to understand context and produce meaningful output is still limited.
- 👂 The human desire for answers drives the appeal of AI, even when the answers provided are not always accurate or reliable.
Q & A
What is the main theme discussed regarding current AI and LLMs in the transcript?
-The main theme discussed is the skepticism towards relying on current AI and Large Language Models (LLMs) for critical tasks such as medical record analysis, due to the potential for inaccuracies and the 'garbage in, garbage out' (GIGO) issue.
What is Brad Hutchings' background, as mentioned in the transcript?
-Brad Hutchings has a Bachelor of Science degree in Computer Science from UC Irvine, with a concentration in algorithms and data structures, which he obtained in 1994 when the program was ranked in the top five.
Why does the speaker express caution about using AI for analyzing medical records?
-The speaker cautions against using AI for analyzing medical records because AI might provide answers, but its reliability is questionable, as it could potentially invent citations or make errors that could have serious consequences.
What is Brad's perspective on AI's impact on software development?
-Brad believes that AI and LLMs are not going to change the way coding is done significantly. He finds himself faster at finding coding solutions through traditional search methods like Google than relying on AI to write code for him.
What example does Brad give to illustrate the distribution of talent in software development?
-Brad illustrates the distribution of talent in software development by comparing it to a bell curve, where the top 10 to 20 percent of coders are significantly more productive than the median coder.
What does Brad think about the usefulness of AI in generating code for developers?
-Brad thinks that while AI can generate code snippets, he can typically find those same snippets faster through Google search or by searching his own code, making AI less useful for him.
What is the 'GIGO' issue mentioned in the transcript?
-The 'GIGO' issue stands for 'garbage in, garbage out,' which means that the output of a system is only as good as the data it is fed. It implies that if AI is trained on poor quality data, it will produce poor quality results.
What is the 'ironic razor' concept mentioned by the speaker?
-The 'ironic razor' is a concept where whatever result AI provides, it tends to be ironic. It plays on the idea that AI can give answers that are satisfying or entertaining because of their irony, rather than their accuracy.
How does the speaker feel about AI's role in the arts and entertainment?
-The speaker expresses concern that AI's role in the arts and entertainment could lead to a loss of authenticity and originality, as AI can generate content that mimics human creativity but lacks the genuine human touch.
What is the potential impact of AI on jobs according to the discussion in the transcript?
-The potential impact of AI on jobs discussed includes both the threat of AI replacing certain job functions, particularly in areas like data analysis and repetitive tasks, and the opportunity for AI to augment human work by handling mundane tasks more efficiently.
Outlines
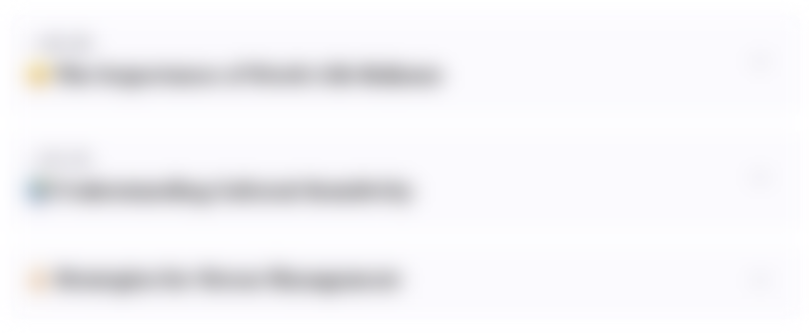
Этот раздел доступен только подписчикам платных тарифов. Пожалуйста, перейдите на платный тариф для доступа.
Перейти на платный тарифMindmap
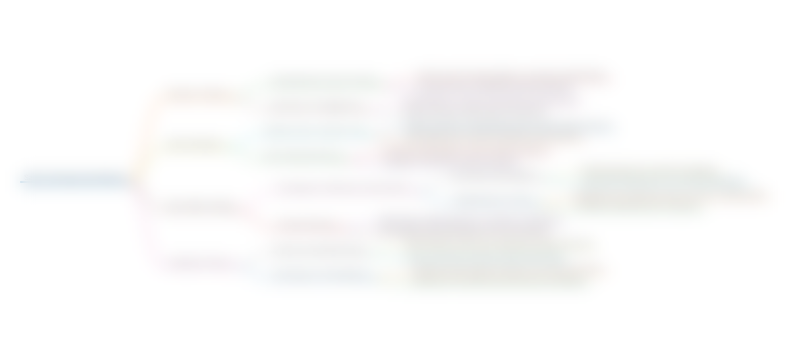
Этот раздел доступен только подписчикам платных тарифов. Пожалуйста, перейдите на платный тариф для доступа.
Перейти на платный тарифKeywords
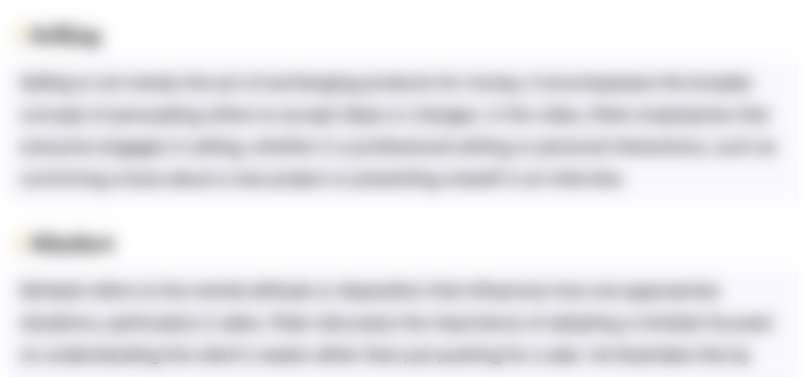
Этот раздел доступен только подписчикам платных тарифов. Пожалуйста, перейдите на платный тариф для доступа.
Перейти на платный тарифHighlights
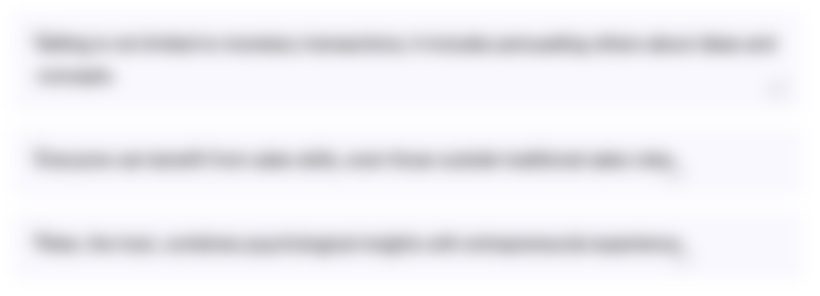
Этот раздел доступен только подписчикам платных тарифов. Пожалуйста, перейдите на платный тариф для доступа.
Перейти на платный тарифTranscripts
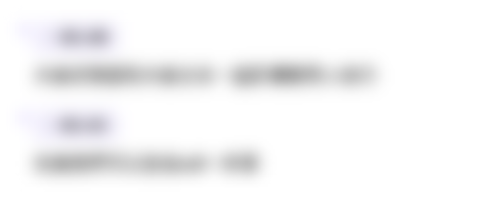
Этот раздел доступен только подписчикам платных тарифов. Пожалуйста, перейдите на платный тариф для доступа.
Перейти на платный тариф5.0 / 5 (0 votes)