OpenAI's 'AGI Robot' Develops SHOCKING NEW ABILITIES | Sam Altman Gives Figure 01 Get a Brain
Summary
TLDRFigure AI, in partnership with OpenAI, presents a groundbreaking amalgamation of robotics and neural networks. The robot, Figure 01, demonstrates advanced capabilities such as full conversations, understanding and responding to visual cues, and executing complex tasks like picking up trash and handling objects. The robot operates through a combination of a large language model for reasoning and neural network policies for physical actions, showcasing a significant step towards general AI and practical applications in embodied AI.
Takeaways
- 🤖 Figure AI is a robotics company that has partnered with OpenAI to integrate robotics and AI expertise.
- 🔄 The collaboration aims to showcase the synergy of metal and neural networks, marking a significant advancement in the field.
- 🍎 Figure One, the robot, can engage in full conversations with people, demonstrating high-level visual and language intelligence.
- 🧠 The robot's actions are powered by neural networks, not pre-programmed scripts, allowing for adaptive and dexterous movements.
- 🎥 The video demonstrates the robot's ability to understand and execute tasks based on visual input and verbal commands.
- 🔄 The robot can multitask, such as picking up trash while responding to questions, showcasing its ability to handle complex tasks.
- 🤔 Figure AI's technology suggests the potential for general AI robots (AGI), with capabilities beyond scripted movements.
- 💻 The robot's neural networks are likely running on a model provided by OpenAI, possibly GPT-4 or a similar advanced model.
- 📈 The partnership between Figure AI and OpenAI highlights the potential for scaling up embodied AI technology.
- 🚀 The demonstration of the robot's capabilities, such as handling objects and understanding context, indicates significant progress in AI and robotics.
- 📌 The video serves as a clear example of the integration of AI and robotics, moving towards more autonomous and interactive machines.
Q & A
What is the significance of the partnership between Figure AI and OpenAI?
-The partnership between Figure AI and OpenAI is significant because it combines Figure AI's expertise in building robotics with OpenAI's advanced AI and neural network capabilities. This collaboration aims to create robots that can understand and interact with their environment in a more sophisticated and autonomous manner.
How does Figure AI's robot utilize neural networks?
-Figure AI's robot uses neural networks to perform a variety of tasks. These include low-level dexterous actions, such as picking up objects, as well as high-level visual and language intelligence to understand and respond to human commands and questions.
What does the term 'end-to-end neural networks' refer to in the context of the video?
-In the context of the video, 'end-to-end neural networks' refers to the complete system of neural networks that handle all aspects of a task, from perception (seeing or understanding the environment) to action (physically manipulating objects), without the need for human intervention or pre-programming.
How does the robot determine which objects are useful for a given task?
-The robot determines which objects are useful for a task by processing visual input from its cameras and understanding the context through a large multimodal model trained by OpenAI. This model uses common sense reasoning to decide which objects are appropriate for the task at hand.
What is the role of the 'speech to speech' component in the robot's operation?
-The 'speech to speech' component allows the robot to engage in full conversations with humans. It processes spoken commands, understands the context, and generates appropriate verbal responses through text-to-speech technology.
How does the robot handle ambiguous or high-level requests?
-The robot handles ambiguous or high-level requests by using its AI model to interpret the request in the context of its surroundings and the objects available. It then selects and executes the most appropriate behavior to fulfill the command, such as handing a person an apple when they express hunger.
What is the significance of the robot's ability to reflect on its memory?
-The robot's ability to reflect on its memory allows it to understand and respond to questions about its past actions. This capability provides a richer interaction experience, as the robot can explain why it performed certain actions based on its memory of the situation.
How does the robot's whole body controller contribute to its stability and safety?
-The whole body controller ensures that the robot maintains balance and stability while performing actions, such as picking up objects or moving around. It manages the robot's dynamics across all its 'degrees of freedom', which include leg movements, arm positions, and other body adjustments necessary for safe and effective task execution.
What is the role of the 'Vis motor Transformer policies' in the robot's learned behaviors?
-The 'Vis motor Transformer policies' are neural network policies that map visual input directly to physical actions. They take onboard images at a high frequency and generate detailed instructions for the robot's movements, such as wrist poses and finger joint angles, enabling the robot to perform complex manipulations and react quickly to its environment.
What are some of the challenges that Figure AI and OpenAI aim to overcome with their partnership?
-Figure AI and OpenAI aim to overcome challenges related to creating robots that can operate autonomously in complex, real-world environments. This includes developing AI that can understand and reason about its surroundings, learn from new experiences, and execute a wide range of physical tasks without human intervention.
Outlines
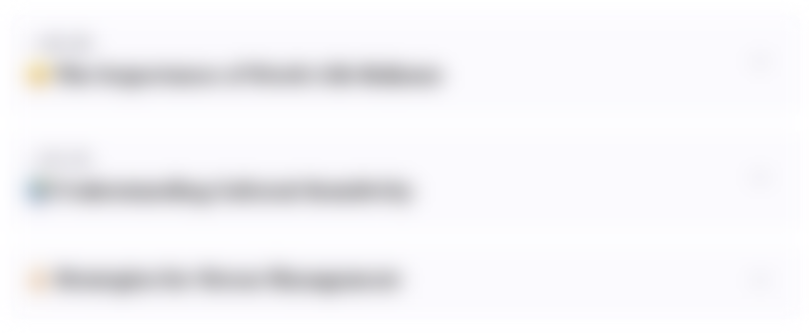
Этот раздел доступен только подписчикам платных тарифов. Пожалуйста, перейдите на платный тариф для доступа.
Перейти на платный тарифMindmap
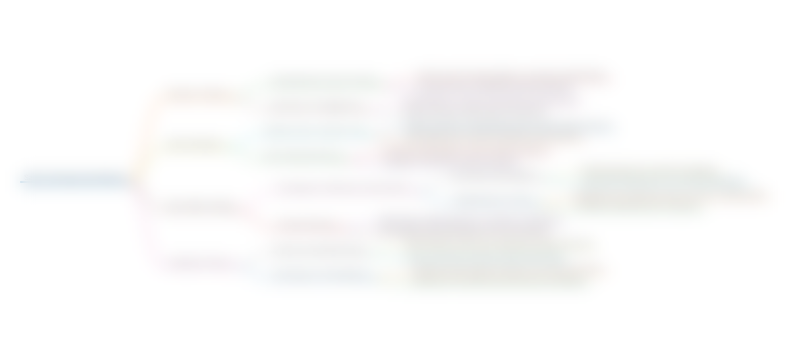
Этот раздел доступен только подписчикам платных тарифов. Пожалуйста, перейдите на платный тариф для доступа.
Перейти на платный тарифKeywords
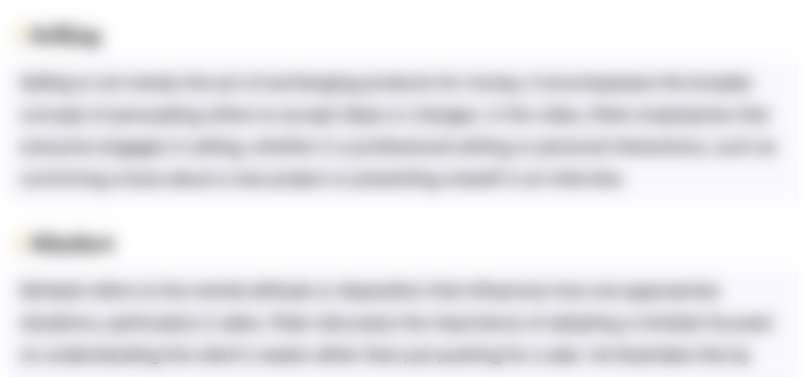
Этот раздел доступен только подписчикам платных тарифов. Пожалуйста, перейдите на платный тариф для доступа.
Перейти на платный тарифHighlights
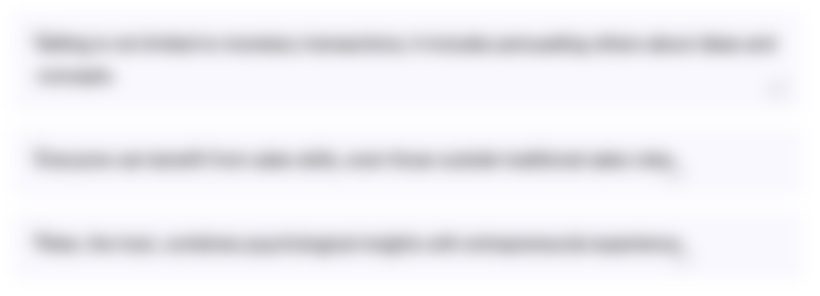
Этот раздел доступен только подписчикам платных тарифов. Пожалуйста, перейдите на платный тариф для доступа.
Перейти на платный тарифTranscripts
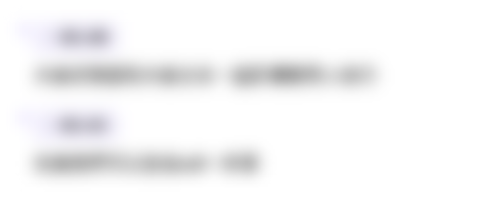
Этот раздел доступен только подписчикам платных тарифов. Пожалуйста, перейдите на платный тариф для доступа.
Перейти на платный тарифПосмотреть больше похожих видео
5.0 / 5 (0 votes)