Quantitative Forecasting Methods in Business Operations
Summary
TLDRThe script delves into quantitative forecasting methods, emphasizing the importance of historical data quality for predicting future trends. It distinguishes between extrinsic and intrinsic quantitative methods, with examples like correlating weather data with ice cream sales and how cold winters in New York might influence travel bookings to Mexico. The script cautions against mistaking correlation for causation and suggests leveraging existing market research. It also touches on time series analysis for intrinsic forecasting, highlighting the limitations of relying solely on historical data for future predictions.
Takeaways
- 📊 Quantitative forecasting methods rely on historical numerical data to predict future trends, emphasizing the importance of data quality.
- 🔍 Extrinsic quantitative methods use external data, such as market or weather data, to forecast sales, like correlating ice cream sales with temperature.
- 📈 Regression analysis is a statistical tool used to identify relationships between variables, helping to predict demand based on influencing factors.
- 🌡️ Understanding causation is crucial for forecasting; correlation alone is not enough, as seen with the unrelated correlation between mozzarella cheese consumption and engineering doctorates.
- 🌟 Econometrics applies regression analysis in economic modeling, such as studying the relationship between disposable income and spending.
- 📚 Leveraging existing forecasts and market research can provide valuable insights and save resources, by building on data already analyzed by others.
- 📉 Intrinsic quantitative methods focus on a company's internal data, often using time series analysis to predict future sales based on past performance.
- 📉 Time series analysis includes techniques like moving averages, exponential smoothing, and more complex methods to forecast future sales trends.
- ⚠️ Historical data alone has limitations for forecasting, as it may not account for unique future events or changes in market conditions.
- 👀 The script concludes with a cautionary note on the limitations of relying solely on historical data for making predictions.
Q & A
What are quantitative forecasting methods?
-Quantitative forecasting methods involve using numerical data, typically from the past, to make predictions about future events. They require sufficient historical data to be considered valid for forecasting.
Why is historical data important in quantitative forecasting?
-Historical data is crucial as it provides the basis for identifying patterns and trends that can be used to predict future outcomes. Without high-quality historical data, the accuracy of the forecasting method may be compromised.
What is the difference between extrinsic and intrinsic quantitative methods?
-Extrinsic quantitative methods use data from external sources, such as market data or weather patterns, while intrinsic methods rely on data from within the company, such as past sales figures.
Can you provide an example of an extrinsic quantitative method?
-An example of an extrinsic method is using historical weather data to forecast ice cream sales, assuming that warmer weather is correlated with higher sales.
What is regression analysis and how is it used in forecasting?
-Regression analysis is a statistical method that tries to determine if one variable influences another. In forecasting, it can help establish causational relationships, such as how weather affects ice cream sales, which can then be used to predict future sales based on future weather forecasts.
Why is it important to differentiate between correlation and causation in forecasting?
-Differentiating between correlation and causation is important because correlation only indicates a relationship between variables, while causation implies that one variable causes changes in another. For accurate forecasting, understanding causation is essential to predict how changes in one variable will affect another.
What is econometrics and how does it relate to regression analysis?
-Econometrics is the application of statistical methods, including regression analysis, to economic data. It is used by economists to analyze relationships between economic variables and to make predictions or test economic theories.
How can existing forecasts by others be utilized in one's own forecasting efforts?
-Existing forecasts by others can be used as a reference or starting point for one's own forecasts. Understanding the data sources and assumptions used by these forecasts can provide valuable insights and help improve the accuracy of one's own predictions.
What is time series analysis and how does it apply to intrinsic quantitative forecasting?
-Time series analysis is the statistical analysis of data points collected over time. In intrinsic quantitative forecasting, it is used to analyze historical sales data to predict future sales trends.
What are some common methods used in time series analysis?
-Common methods in time series analysis include moving averages, exponential smoothing, time series decomposition, and pattern analysis. These methods help in identifying patterns and trends in historical data to forecast future values.
What is a potential limitation of using only historical data for forecasting?
-A limitation of using only historical data is that it may not account for unique or unusual events that could occur in the future, which were not present in the historical data. This can lead to inaccurate forecasts if such events significantly impact the variable being predicted.
Outlines
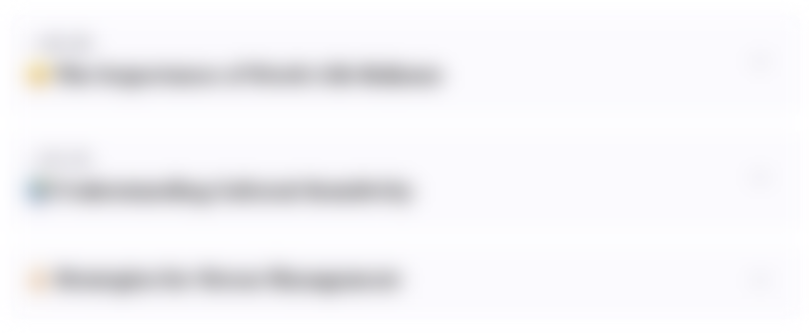
Этот раздел доступен только подписчикам платных тарифов. Пожалуйста, перейдите на платный тариф для доступа.
Перейти на платный тарифMindmap
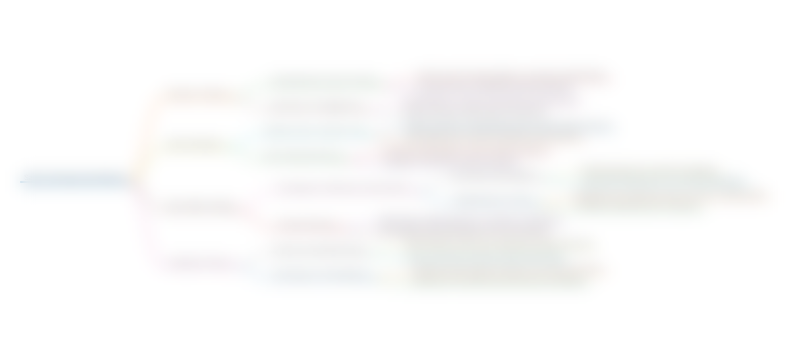
Этот раздел доступен только подписчикам платных тарифов. Пожалуйста, перейдите на платный тариф для доступа.
Перейти на платный тарифKeywords
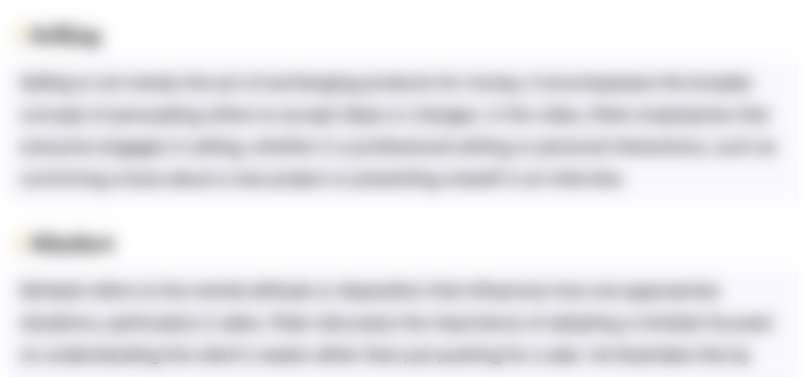
Этот раздел доступен только подписчикам платных тарифов. Пожалуйста, перейдите на платный тариф для доступа.
Перейти на платный тарифHighlights
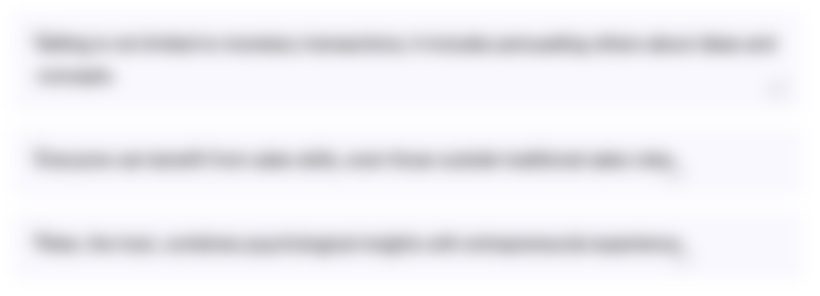
Этот раздел доступен только подписчикам платных тарифов. Пожалуйста, перейдите на платный тариф для доступа.
Перейти на платный тарифTranscripts
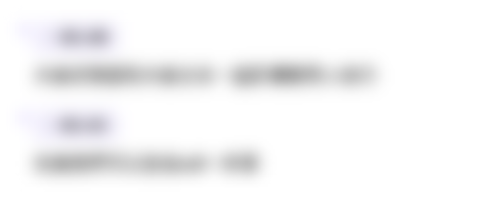
Этот раздел доступен только подписчикам платных тарифов. Пожалуйста, перейдите на платный тариф для доступа.
Перейти на платный тариф5.0 / 5 (0 votes)