Trinity of Artificial Intelligence | Anima Anandkumar | TEDxIndianaUniversity
Summary
TLDRIn this talk, the presenter explores the concept of artificial intelligence, focusing on the Trinity of AI: data, learning algorithms, and computer infrastructure. They discuss the importance of each component in creating task-oriented intelligence and highlight the rapid advancements in image classification due to the ImageNet dataset and deep learning models. The presenter also touches on the future of AI, including multi-dimensional data processing with tensors and the integration of AI with robotics to create instinctive, deliberative, and multi-agent robots.
Takeaways
- 🤖 The speaker discusses the Trinity of AI, which includes data, learning algorithms, and computer infrastructure as the three main ingredients for AI success.
- 🧠 Intelligence is defined as the ability to acquire and apply knowledge and skills, contrasting the pre-programmed actions of robots with the improvisational skills of animals like dogs.
- 📈 The importance of large datasets, such as ImageNet, is highlighted for training AI systems to recognize and categorize images effectively.
- 💾 The shift from CPU to GPU computing is identified as a key factor in the advancement of AI, with GPUs providing the necessary parallel processing power.
- 📊 Deep learning models with many layers are crucial for processing complex data like images, allowing AI to learn from examples and improve its performance.
- 📉 The speaker points out the rapid progress in image classification, noting how AI systems have reached and even surpassed human-level performance on certain datasets.
- 🔮 Future research directions include multi-dimensional data processing using tensors, which can handle data with more than two dimensions, like videos or text documents.
- 🤝 The integration of AI (the mind) with robotics (the body) is presented as a challenge and opportunity, aiming to create more instinctive, deliberative, and interactive robots.
- 🛸 An example of AI in robotics is given, where drones learn to land more effectively through data-driven learning rather than pre-programmed algorithms.
- 🌪️ The potential for AI to enhance drone performance in adverse conditions, such as different wind patterns, is explored through simulation and data collection.
Q & A
What is the Trinity of AI as discussed in the transcript?
-The Trinity of AI consists of three main ingredients: data, learning algorithms, and computer infrastructure. Data provides the examples or information needed for learning, learning algorithms process the data to extract knowledge, and computer infrastructure provides the necessary computational power to perform these tasks.
Why is data considered the most important ingredient in the Trinity of AI?
-Data is considered the most important ingredient because it provides the examples or observations that an AI system needs to learn from. Without sufficient data, an AI system cannot acquire the knowledge and skills required to perform tasks effectively.
How does the image classification task exemplify the success of AI?
-The image classification task exemplifies the success of AI by demonstrating how AI systems can learn from a large dataset of images, such as ImageNet, to recognize and categorize new images with high accuracy. This progress was made possible by combining data, deep learning models, and powerful computer infrastructure like GPUs.
What is the significance of the ImageNet dataset in AI development?
-The ImageNet dataset is significant because it provided a large and diverse collection of categorized images, which allowed AI systems to learn and improve their ability to recognize and classify images. This dataset was crucial in advancing computer vision and deep learning algorithms.
How do deep learning models contribute to AI's ability to perform tasks?
-Deep learning models contribute to AI's ability to perform tasks by processing input data through multiple layers, allowing the system to learn complex patterns and features. These models can extract relevant information from vast amounts of data, enabling AI systems to perform specific tasks, such as image recognition, with high accuracy.
What is the role of GPU computing in AI?
-GPU computing plays a crucial role in AI by providing the parallel processing capabilities needed to handle the massive computational demands of deep learning algorithms. GPUs enable AI systems to perform billions of operations per image in a highly efficient manner, which is essential for training and deploying large-scale AI models.
Why did the speaker mention the Boston Dynamics Atlas robot and a dog attempting a backflip?
-The speaker mentioned the Boston Dynamics Atlas robot and a dog attempting a backflip to illustrate the difference between pre-programmed actions and true intelligence. While the robot executed a perfect backflip, it did so based on a pre-programmed sequence, whereas the dog, despite failing, demonstrated the ability to learn and adapt, which is a sign of intelligence.
What is the challenge with AI systems when it comes to generalizing beyond their training data?
-The challenge with AI systems when it comes to generalizing beyond their training data is that they may perform well on specific datasets or tasks but struggle to adapt to new or unseen data. This limitation highlights the need for AI systems to develop more robust learning capabilities that allow them to generalize and apply their knowledge to a wider range of situations.
How does the concept of tensors relate to multi-dimensional data processing in AI?
-Tensors are mathematical objects that enable processing in any number of dimensions, generalizing the concepts of vectors and matrices. In AI, tensors are used to handle and analyze multi-dimensional data, such as images, videos, and text, by capturing and processing information across different dimensions, which can lead to more effective learning and understanding of complex data structures.
What is the potential future of integrating AI 'mind' with robotics 'body'?
-The potential future of integrating AI 'mind' with robotics 'body' involves creating robots that are instinctive, deliberative, multi-agent, and behavioral. This integration aims to develop robots that can react and control their reactions in a fine-grained manner, make plans and consider consequences, coordinate and work with others, and interact with humans by understanding emotions and acting as partners.
Outlines
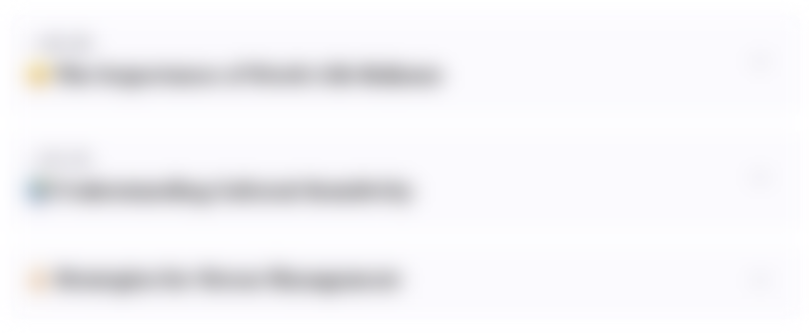
Этот раздел доступен только подписчикам платных тарифов. Пожалуйста, перейдите на платный тариф для доступа.
Перейти на платный тарифMindmap
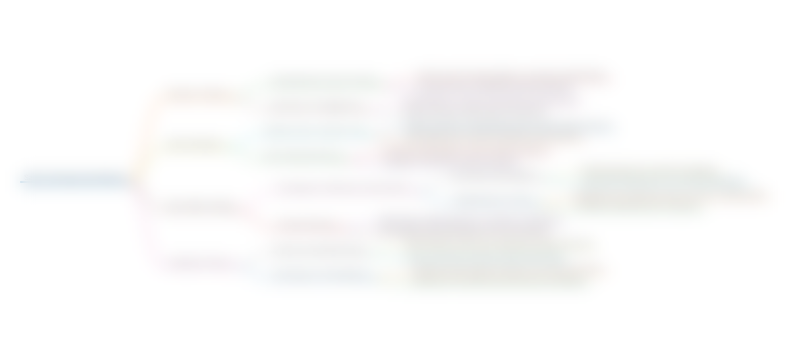
Этот раздел доступен только подписчикам платных тарифов. Пожалуйста, перейдите на платный тариф для доступа.
Перейти на платный тарифKeywords
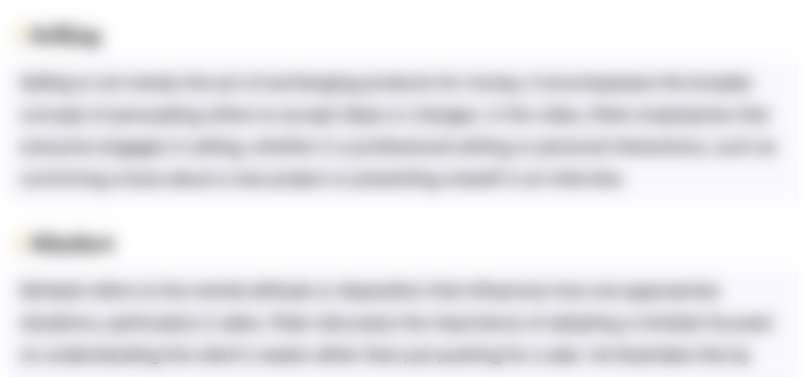
Этот раздел доступен только подписчикам платных тарифов. Пожалуйста, перейдите на платный тариф для доступа.
Перейти на платный тарифHighlights
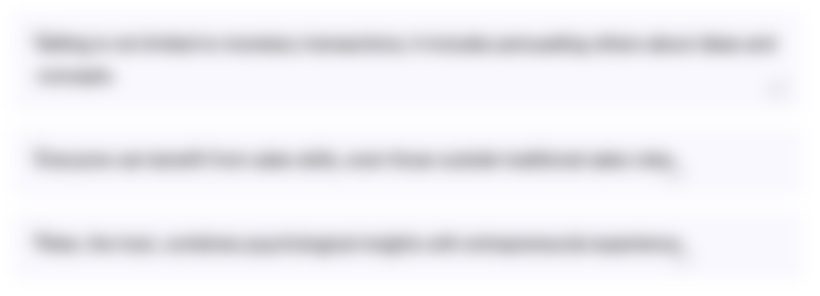
Этот раздел доступен только подписчикам платных тарифов. Пожалуйста, перейдите на платный тариф для доступа.
Перейти на платный тарифTranscripts
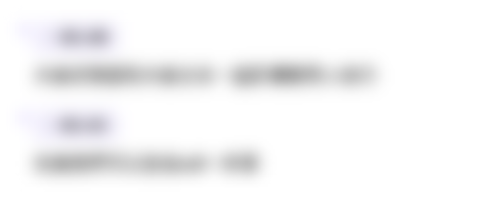
Этот раздел доступен только подписчикам платных тарифов. Пожалуйста, перейдите на платный тариф для доступа.
Перейти на платный тарифПосмотреть больше похожих видео
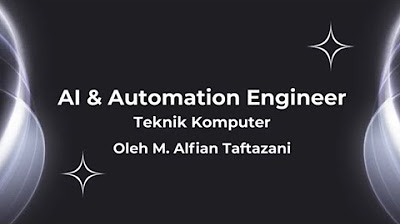
AI & Automation Engineer Teknik Komputer
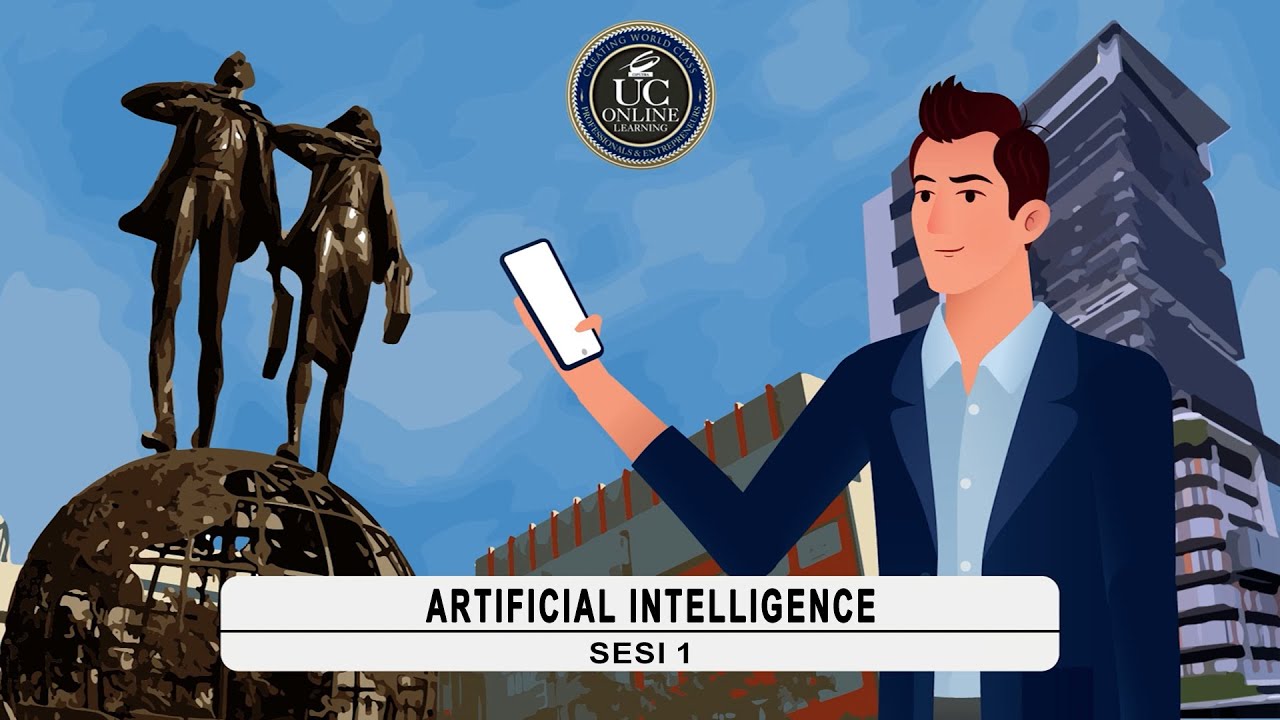
IMT - AI (1) - Apa itu AI?, Sejarah, dan Penerapan AI
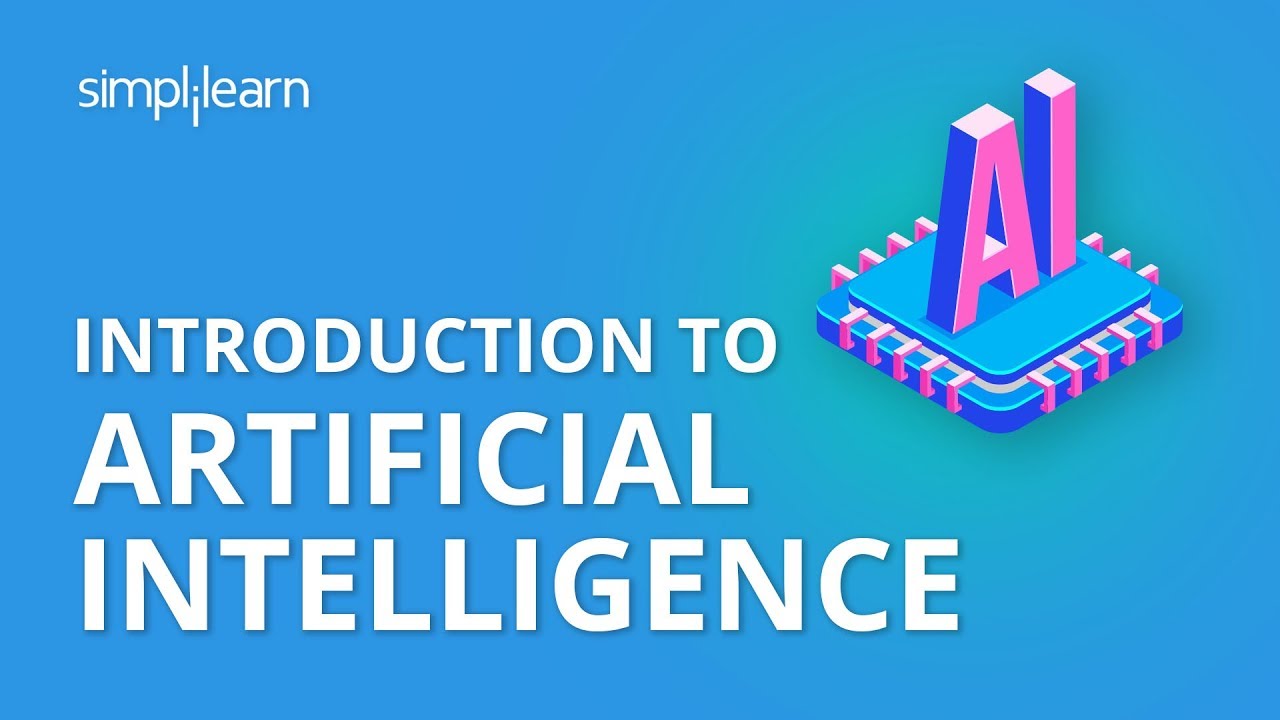
Introduction To Artificial Intelligence | What Is AI?| Artificial Intelligence Tutorial |Simplilearn
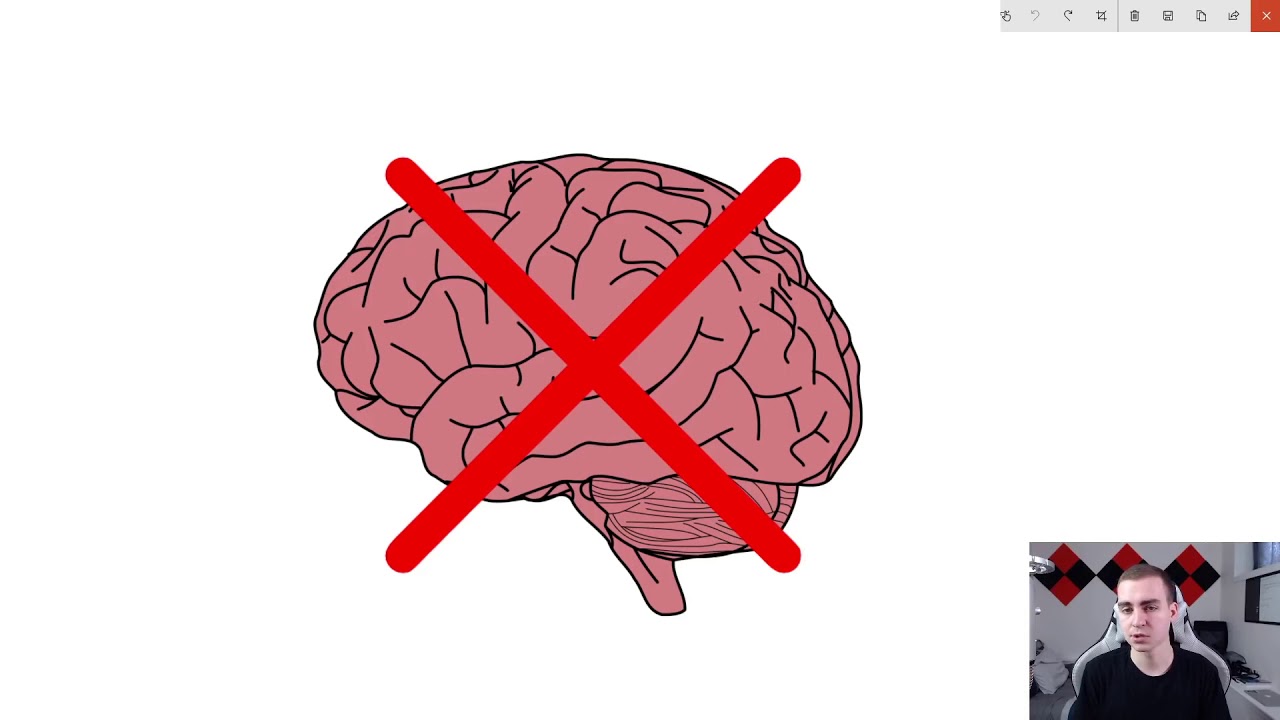
Machine Learning Fundamentals A - TensorFlow 2.0 Course
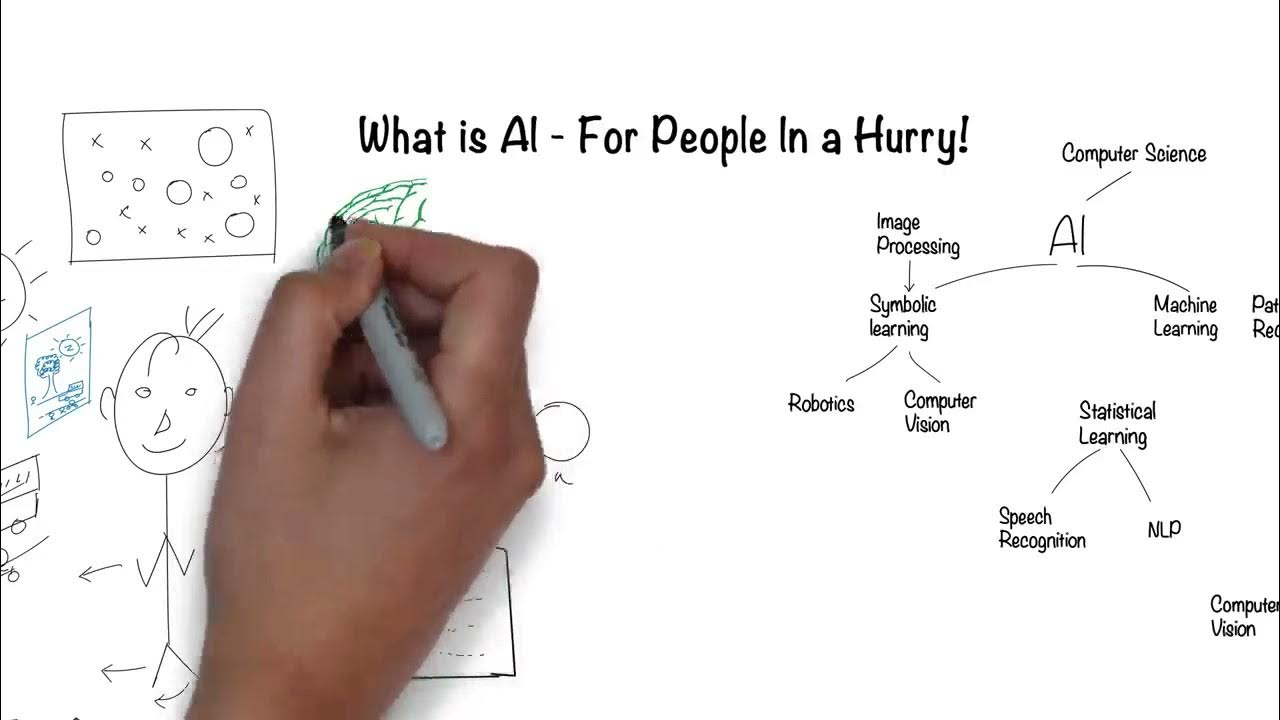
Artificial Intelligence (AI) for People in a Hurry
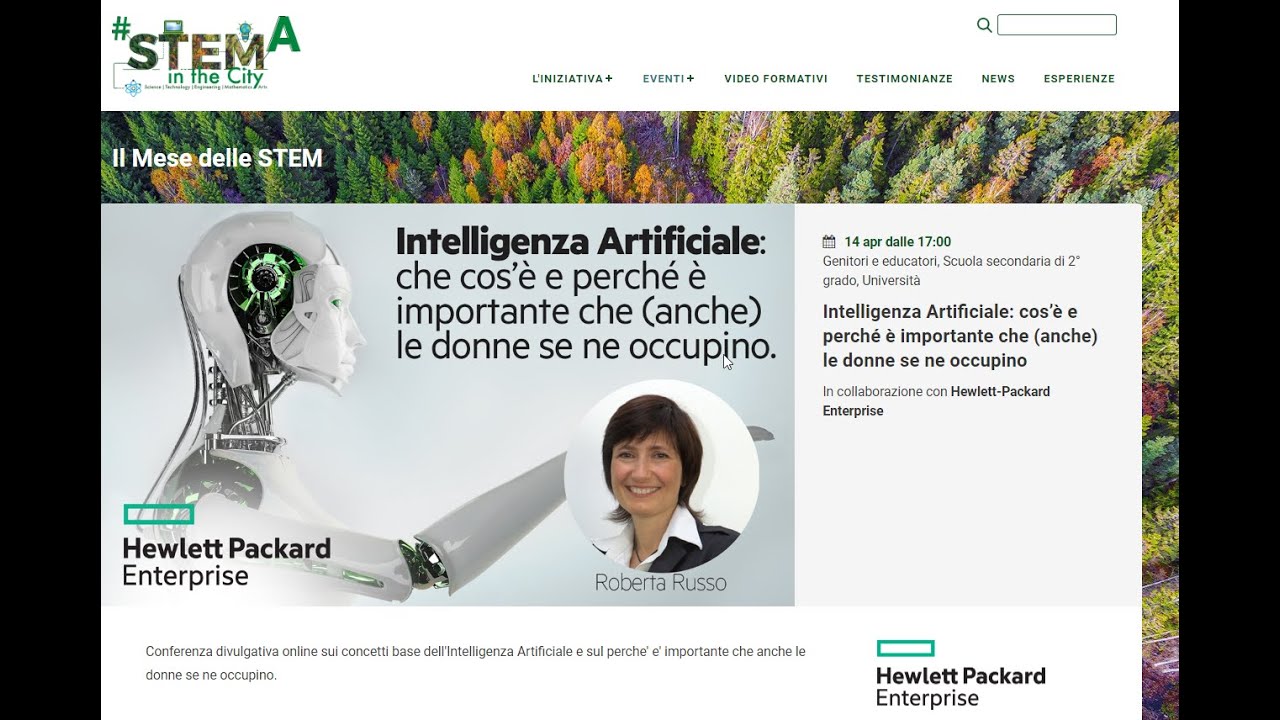
Intelligenza Artificiale: cos'e' e perche' e' importante che (anche) le donne se ne occupino
5.0 / 5 (0 votes)