Regresi dan Korelasi Sederhana
Summary
TLDRThis video script covers key concepts in statistics, focusing on simple linear regression and correlation. It explains how regression analysis helps in predicting the relationship between dependent and independent variables, using formulas and examples like fertilizer use and crop yield. The script also introduces correlation, detailing how it measures the strength of relationships between two variables. Various methods, such as Pearson's correlation and rank-based measures, are explained. Additionally, the script discusses how the coefficient of determination quantifies the impact of one variable on another, emphasizing practical applications in business and research.
Takeaways
- 😀 Regression analysis is used for forecasting or estimating the value of a dependent variable (Y) based on an independent variable (X).
- 😀 Simple linear regression involves a formula where Y = a + b * X, with 'a' as the intercept and 'b' as the slope (regression coefficient).
- 😀 The purpose of simple linear regression is to predict or estimate the value of the dependent variable using the known independent variable.
- 😀 In the given example, data on fertilizer (X) and harvest (Y) is used to build a regression equation to predict harvest based on the amount of fertilizer.
- 😀 The formula for calculating the slope 'b' and intercept 'a' involves using summation formulas for variables X and Y, as well as their squares and product.
- 😀 The regression equation obtained from the example is Y = 10.3 + 1.5 * X, indicating that for every additional kg of fertilizer, the harvest increases by 1.5 quintals.
- 😀 Correlation analysis measures the relationship between two variables, with strength and direction being key outcomes. Values range from 0 (no correlation) to 1 (perfect correlation).
- 😀 Correlation is categorized as strong (>0.8), moderate (0.6 - 0.79), weak (0.2 - 0.39), or very weak (0.0 - 0.19).
- 😀 Different types of correlation coefficients are used depending on data type: Pearson's for interval/ratio data, Spearman's for ordinal data, and Kendall’s for measuring irregularities in ranked data.
- 😀 The coefficient of determination (R²) explains how much variation in the dependent variable can be attributed to the independent variable. For example, if R² = 0.78, 78% of the variation in sales can be explained by promotional costs.
- 😀 The relationship between promotional costs and sales is strong, with an R-value of 0.885, indicating a strong positive correlation, meaning increasing promotion will likely lead to higher sales.
Q & A
What is the concept of linear regression as explained in the script?
-Linear regression refers to the statistical method used to predict the value of a dependent variable (Y) based on the value of an independent variable (X). It assumes a linear relationship, meaning that as the independent variable changes, the dependent variable will change in a consistent manner.
What does the script mention about the formula for simple linear regression?
-The formula for simple linear regression is given as Y = a + bX. Here, Y represents the dependent variable, X is the independent variable, a is the intercept (the value of Y when X equals 0), and b is the regression coefficient (representing the slope or rate of change).
How does the script describe the purpose of regression analysis?
-The purpose of regression analysis is to make accurate predictions or estimations about the value of a dependent variable (Y) based on the value of an independent variable (X). It helps in forecasting the outcome of one variable given the behavior of another.
What are the steps involved in calculating the regression coefficients (a and b)?
-To calculate the regression coefficients, you need to find the values of b (slope) and a (intercept). The slope b is calculated using a formula involving the sum of products of X and Y, the sum of X, Y, and the sum of squares of X. The intercept a is then calculated using the formula involving the average of Y and X values.
What does the regression equation Y = 10.3 + 1.5X represent in the example from the script?
-In the example, the regression equation Y = 10.3 + 1.5X indicates that for every 1 kg increase in fertilizer (X), the yield (Y) will increase by 1.5 quintals. This relationship helps in predicting the yield based on the amount of fertilizer used.
What is the difference between simple linear regression and correlation as explained in the script?
-Linear regression focuses on predicting the dependent variable (Y) based on the independent variable (X), while correlation measures the strength and direction of the relationship between two variables. Regression provides a predictive model, whereas correlation quantifies the degree of relationship between variables.
How is correlation measured and interpreted according to the script?
-Correlation is measured using a coefficient, which can range from -1 to 1. A correlation coefficient above 0.8 indicates a strong positive relationship, between 0.6 and 0.79 indicates a moderate relationship, and below 0.2 suggests a very weak or no relationship.
What are the types of correlation mentioned in the script, and what data are they used for?
-The script mentions four types of correlation: Pearson correlation (for interval or ratio data), Spearman rank correlation (for ordinal data), Kendall's rank correlation (for measuring inconsistencies in rank orders), and Contingency coefficient (for nominal data). Each is used for different types of data depending on their measurement scales.
What does the coefficient of determination (R²) represent in regression analysis?
-The coefficient of determination (R²) measures the proportion of the variance in the dependent variable that is explained by the independent variable. It reflects how well the regression model predicts the outcome and is expressed as a percentage.
In the example of sales and promotion expenses, what did the correlation coefficient of 0.885 indicate?
-A correlation coefficient of 0.885 indicates a strong positive relationship between promotional expenses and sales. This means that as promotional expenses increase, sales tend to increase as well.
Outlines
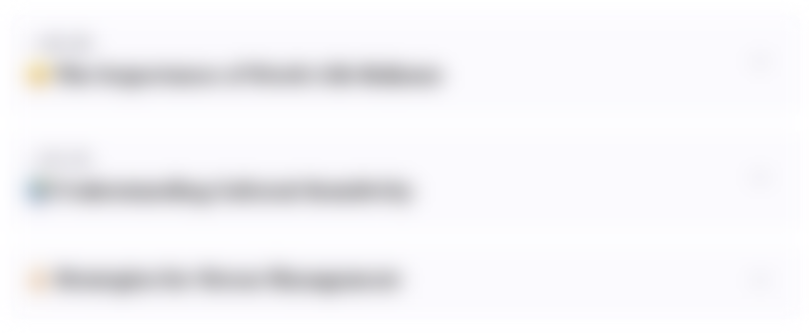
このセクションは有料ユーザー限定です。 アクセスするには、アップグレードをお願いします。
今すぐアップグレードMindmap
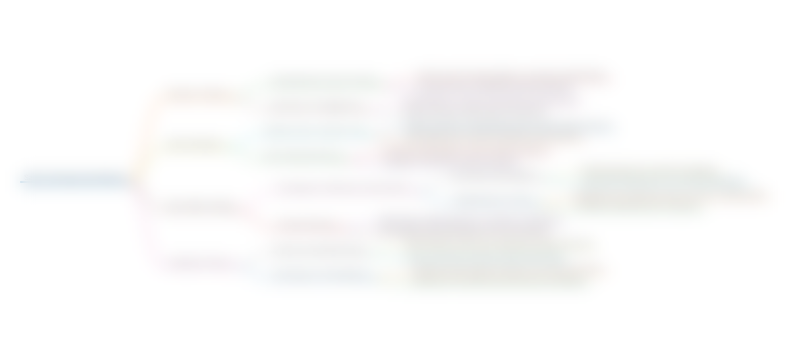
このセクションは有料ユーザー限定です。 アクセスするには、アップグレードをお願いします。
今すぐアップグレードKeywords
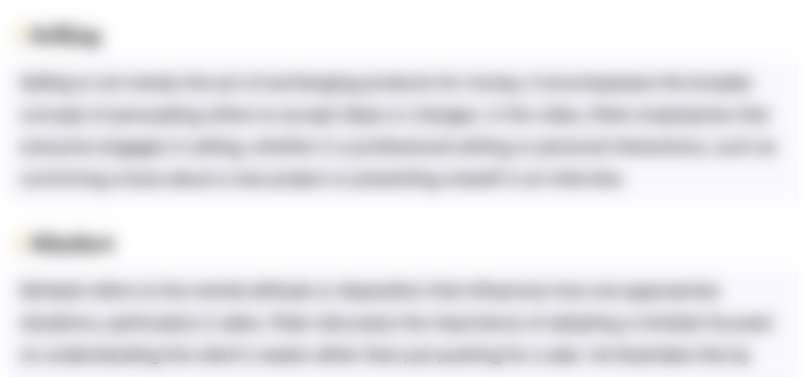
このセクションは有料ユーザー限定です。 アクセスするには、アップグレードをお願いします。
今すぐアップグレードHighlights
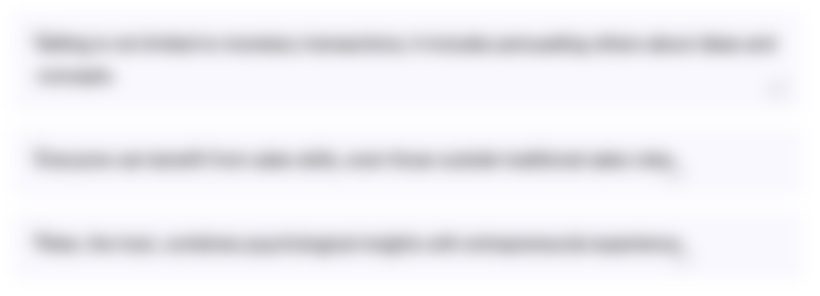
このセクションは有料ユーザー限定です。 アクセスするには、アップグレードをお願いします。
今すぐアップグレードTranscripts
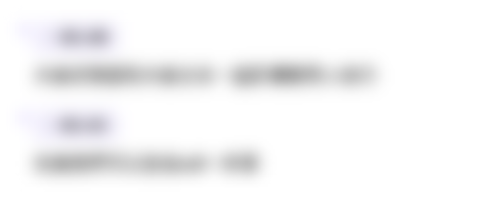
このセクションは有料ユーザー限定です。 アクセスするには、アップグレードをお願いします。
今すぐアップグレード関連動画をさらに表示
5.0 / 5 (0 votes)