Decompostion1
Summary
TLDRThis video discusses how to forecast a more complex time series using the data provided by a piano store. It covers the process of decomposing the time series into its components: trend, seasonality, and random deviations. The speaker explains that neither a purely trend-based nor seasonal approach would yield accurate forecasts, so a multiplicative model is used. The steps involve isolating seasonality, removing the trend, projecting the trend into the future, and incorporating seasonality back into the forecast. Finally, the video demonstrates how to assess the accuracy and reliability of the forecast.
Takeaways
- 😀 Time series forecasting involves predicting future values based on historical data.
- 😀 The script demonstrates how to forecast demand for piano sales using historical data from a piano house.
- 😀 The primary objective is to estimate demand for 2016 based on existing data.
- 😀 A graphical representation of the data reveals seasonality and a trend in the time series.
- 😀 A simple moving average or seasonal analysis alone would not provide accurate forecasts for this data.
- 😀 Decomposition is used to break down the time series into trend, seasonality, and random fluctuations.
- 😀 Two models are discussed for forecasting: multiplicative and additive models.
- 😀 The multiplicative model assumes that the forecast is a product of trend, seasonality, and random factors.
- 😀 The additive model assumes that the forecast is a sum of these components.
- 😀 The forecasting process involves isolating seasonality, removing it to focus on the trend, and then forecasting future values.
- 😀 Once the trend is forecasted, seasonality is reintroduced into the forecast, followed by validation of the forecast's accuracy.
Q & A
What is the main goal of the script?
-The main goal of the script is to demonstrate how to forecast demand for pianos in 2016 using time series analysis, breaking down the time series into components such as trend, seasonality, and randomness.
What type of data is being analyzed in the script?
-The data being analyzed is the sales or demand data for pianos over a number of years, which is used to forecast future sales.
Why does the script mention both trend and seasonality in the time series data?
-The script highlights that both trend and seasonality are present in the data, which means that the time series is influenced by long-term growth (trend) and recurring patterns (seasonality), making it more complex to forecast.
Why would a moving average method or purely seasonal analysis not provide good results for the forecast?
-Both methods would fail to capture the full complexity of the data. A moving average would not address the seasonality or trend, while a purely seasonal approach would ignore the underlying trend in the data, leading to inaccurate forecasts.
What is the decomposition process mentioned in the script?
-The decomposition process refers to breaking down the time series into its three main components: trend, seasonality, and random deviations. This process helps to isolate and analyze each factor separately for more accurate forecasting.
What are the two models for decomposing time series data mentioned in the script?
-The script mentions two models for decomposition: a multiplicative model, where the forecast is calculated by multiplying the trend, seasonality, and randomness, and an additive model, where the components are simply added together.
Why does the script choose the multiplicative model over the additive model?
-The multiplicative model is chosen because it is more commonly used in practice, particularly when the components of the time series (trend, seasonality, randomness) interact multiplicatively rather than additively.
What are the four main steps in the decomposition process?
-The four main steps are: 1) Determine the seasonality and remove it, 2) Isolate the trend from the remaining data, 3) Forecast the trend for the future, and 4) Reintroduce the seasonality by multiplying it with the forecasted trend.
How is the quality of the forecast assessed according to the script?
-The quality of the forecast is assessed by evaluating its accuracy, ensuring that the predictions made from the time series decomposition are reliable and valid for decision-making.
What practical purpose does the video aim to serve?
-The video aims to demonstrate how to quickly and easily implement the five steps of time series decomposition and forecasting, helping viewers learn to apply these methods to their own data.
Outlines
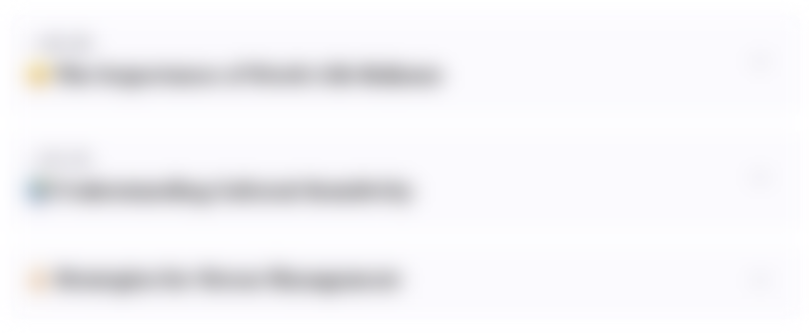
このセクションは有料ユーザー限定です。 アクセスするには、アップグレードをお願いします。
今すぐアップグレードMindmap
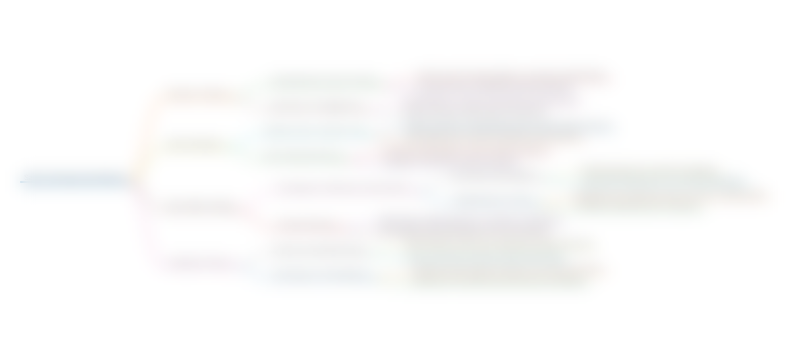
このセクションは有料ユーザー限定です。 アクセスするには、アップグレードをお願いします。
今すぐアップグレードKeywords
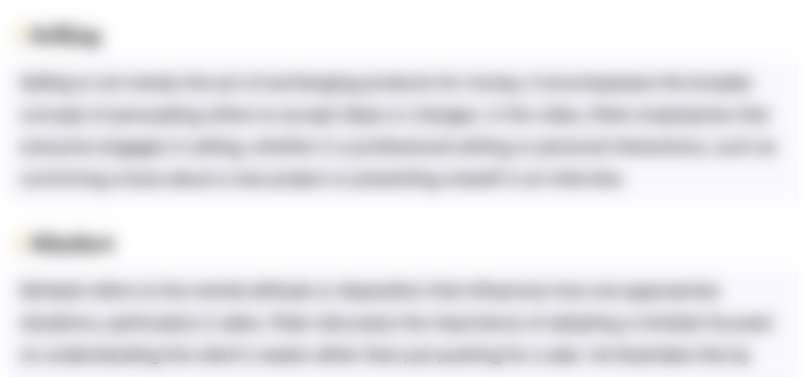
このセクションは有料ユーザー限定です。 アクセスするには、アップグレードをお願いします。
今すぐアップグレードHighlights
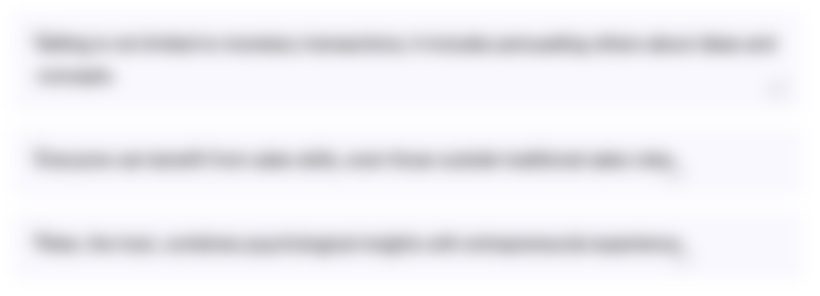
このセクションは有料ユーザー限定です。 アクセスするには、アップグレードをお願いします。
今すぐアップグレードTranscripts
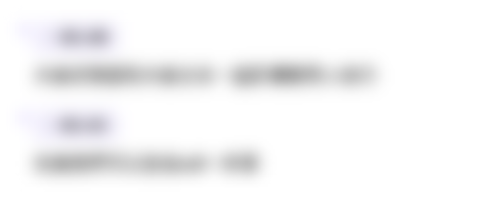
このセクションは有料ユーザー限定です。 アクセスするには、アップグレードをお願いします。
今すぐアップグレード5.0 / 5 (0 votes)