Metode Statistika | Sebaran Peluang Diskrit | Bernoulli | Binomial | Poisson
Summary
TLDRThis video discusses the concepts of success and failure through the lens of probability distributions, focusing on Bernoulli, binomial, and Poisson distributions. It explains how each distribution works, using practical examples like job applications and accident rates, and demonstrates how to calculate probabilities using these models. Bernoulli is used for binary outcomes (success or failure), binomial for multiple independent trials with the same success probability, and Poisson for events occurring within a fixed time or space interval. The video encourages practice to master these probability distributions.
Takeaways
- 🤔 Success and failure are common occurrences in human life, and they can be analyzed statistically.
- 📊 Bernoulli distribution is a discrete probability distribution with only two possible outcomes: success (1) or failure (0).
- 🎯 In Bernoulli trials, the probability of success is denoted as 'P,' and the probability of failure is '1 - P.'
- 💼 Applying for jobs can be modeled using Bernoulli and binomial distributions, where the outcomes are independent and follow specific probabilities.
- 👩💼 If you apply to five companies, the number of acceptances follows a binomial distribution with parameters N (number of trials) and P (probability of success).
- 📐 The binomial probability formula involves a combination of successes and failures, calculated using the binomial coefficient.
- 📈 The probability of being accepted by exactly two companies (out of five) can be computed using binomial probability formulas.
- ⏲️ Poisson distribution models the probability of events occurring within a specific time or space interval, such as the number of accidents in a month.
- 🛣️ An example of a Poisson distribution is calculating the probability of six accidents happening in a month, given an average rate of four accidents.
- 🔍 The key difference between binomial and Poisson distributions is that binomial deals with independent trials, while Poisson focuses on the frequency of events in a continuous interval.
Q & A
What is the main topic discussed in the video?
-The main topic discussed is the probability of success and failure, using Bernoulli, Binomial, and Poisson distributions as examples.
What is a Bernoulli distribution?
-A Bernoulli distribution describes a random variable that has only two possible outcomes: success (coded as 1) and failure (coded as 0), with a given probability of success (P).
How does the video describe a binomial distribution?
-A binomial distribution involves multiple independent Bernoulli trials, each with the same probability of success (P). The random variable in a binomial distribution represents the number of successes in a fixed number of trials.
What is the example given for a binomial distribution?
-The example given is applying to five companies for a job. The number of companies that accept the application is a binomial random variable, with each acceptance having a 0.6 probability.
What is the key difference between Bernoulli and binomial distributions?
-A Bernoulli distribution represents a single trial with two outcomes (success or failure), while a binomial distribution represents multiple independent trials, where each trial follows a Bernoulli distribution.
How does the video explain calculating probabilities in a binomial distribution?
-Probabilities in a binomial distribution are calculated using the binomial probability formula: P(X=x) = C(n, x) * P^x * (1-P)^(n-x), where C(n, x) is the binomial coefficient, and n is the number of trials.
What is a Poisson distribution, as explained in the video?
-A Poisson distribution describes the probability of a given number of events occurring in a fixed interval of time or space, with the average rate of occurrence known. The random variable can take non-negative integer values.
What is an example of a Poisson distribution from the video?
-The video gives an example of the number of traffic accidents occurring on a toll road in a month. The average number of accidents is 4, and the probability of having 6 accidents in a month is calculated using the Poisson distribution.
What is the probability formula for a Poisson distribution?
-The probability of observing x events in a Poisson distribution is given by P(X=x) = (e^(-λ) * λ^x) / x!, where λ is the average rate of occurrence, and x is the number of events.
What conditions must be met for a binomial distribution to apply?
-For a binomial distribution to apply, the trials must be independent, and the probability of success (P) must remain constant across all trials.
Outlines
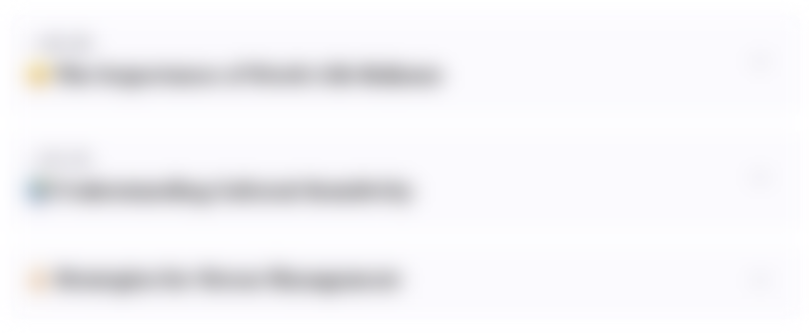
このセクションは有料ユーザー限定です。 アクセスするには、アップグレードをお願いします。
今すぐアップグレードMindmap
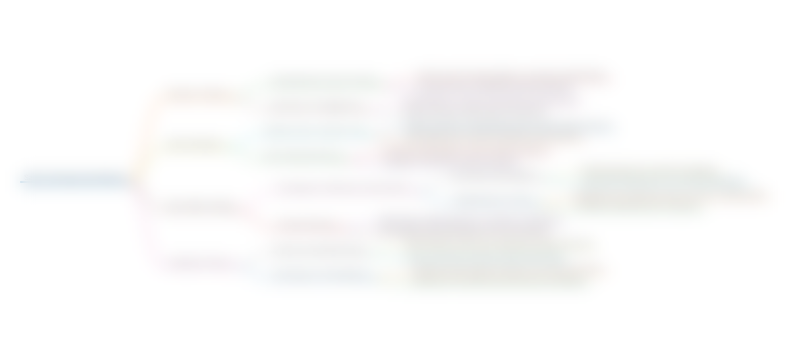
このセクションは有料ユーザー限定です。 アクセスするには、アップグレードをお願いします。
今すぐアップグレードKeywords
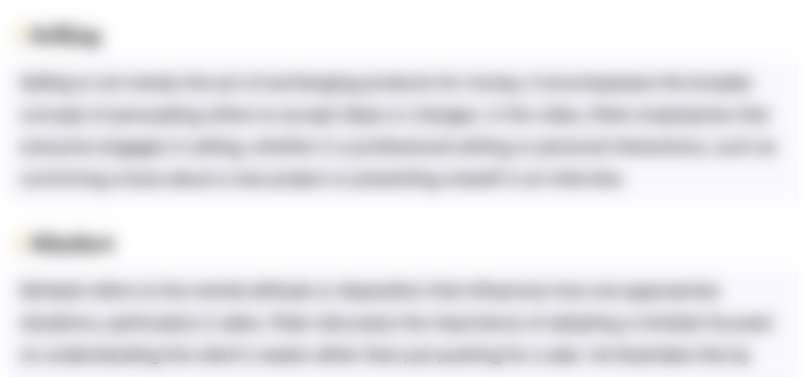
このセクションは有料ユーザー限定です。 アクセスするには、アップグレードをお願いします。
今すぐアップグレードHighlights
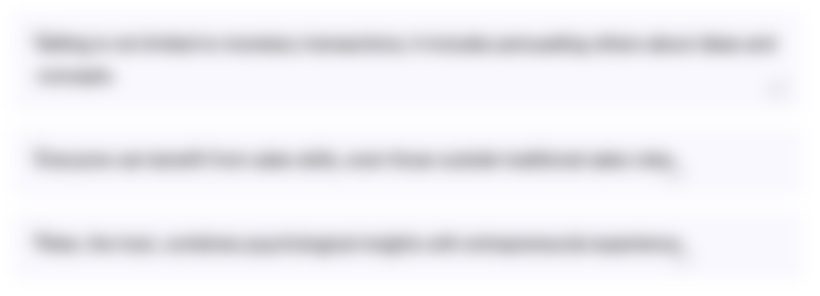
このセクションは有料ユーザー限定です。 アクセスするには、アップグレードをお願いします。
今すぐアップグレードTranscripts
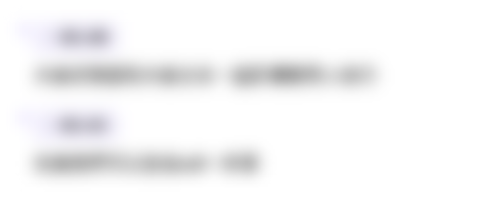
このセクションは有料ユーザー限定です。 アクセスするには、アップグレードをお願いします。
今すぐアップグレード5.0 / 5 (0 votes)