The role of data in criminal justice
Summary
TLDRThe discussion centers around the growing role of data-driven systems in the criminal justice system and their limitations. While data is often seen as objective and a solution to human bias, the speakers argue that it frequently reflects existing racial biases and systemic inequalities. They raise concerns about the use of predictive tools and risk assessments, which may reinforce past injustices rather than address the underlying issues. The conversation emphasizes the need for a paradigm shift, focusing on social justice interventions instead of relying solely on technology to predict or prevent crime.
Takeaways
- 🧑⚖️ Data-driven systems in criminal justice are viewed with skepticism due to concerns that they replicate systemic biases, particularly racism and inequality inherent in historical data.
- 📊 Data used in criminal justice often reflects enforcement patterns rather than actual crime rates, leading to distorted outcomes when predicting crime or behavior.
- 🤔 Many believe that data is objective, but it often fails to capture unreported crimes or the nuanced reasons behind certain behaviors, leading to unreliable predictions.
- 🔍 There's a need for a paradigm shift in criminal justice—away from predictive models that attempt to foresee human behavior and towards addressing root causes of crime through social interventions.
- 🚫 Critics argue that current data-driven tools focus too much on individuals caught in the system rather than on those perpetuating it, such as police officers, judges, and prosecutors.
- 🪞 Algorithmic tools are often seen as mirrors reflecting existing biases rather than correcting them, leading to recommendations that preserve the status quo rather than drive meaningful change.
- ⚖️ The rapid development of technology often outpaces policy reform, making it difficult for legal systems to adapt to new tools and ensure that they are used in ways that are fair and effective.
- 📉 There’s growing advocacy for shifting focus from prediction to intervention, using data to test what measures actually help reduce crime and recidivism rather than just forecasting risk.
- 💡 Social justice approaches, such as investing in community resources and decriminalization, are seen as more effective than criminal justice responses in addressing the root causes of crime.
- 🔄 Concepts like pretrial risk and danger are often ill-defined, subjective, and legally recent, complicating the fair use of data in criminal justice decision-making.
Q & A
What is the main concern about data-driven systems in the criminal justice system?
-The main concern is that data used in criminal justice may be tainted by the country’s history of racism and inequality, potentially leading to biased outcomes and perpetuating systemic injustices.
Why do people trust data-driven systems despite these concerns?
-People tend to trust data-driven systems because they are perceived as more objective and less prone to human error, which can improve decision-making in some aspects of criminal justice.
What is a key misconception about data in the criminal justice system?
-A key misconception is that the data is purely objective. In reality, it often reflects enforcement patterns, not actual crime rates, and can be influenced by biases in policing.
How can domestic violence data be unreliable for criminal justice systems?
-Domestic violence is often underreported for various reasons, including fear of police or social stigma, leading to unreliable data that doesn't accurately represent the true extent of the issue.
What is the 'garbage in, garbage out' problem mentioned in the context of predictive tools?
-This refers to the idea that if biased or flawed data is used in a predictive model, the results will also be flawed and perpetuate existing inequalities.
Why is it difficult to ‘scrub’ data of racial bias?
-Racial bias and inequality are deeply woven into many aspects of American society, such as housing, education, and law enforcement, making it challenging, if not impossible, to completely remove racial influence from the data.
What alternative uses of data tools were suggested by the speakers?
-The speakers suggest using data tools to assess and modify the behavior of actors within the justice system, such as police officers, judges, and prosecutors, rather than predicting the behavior of individuals.
Why do the speakers doubt the ability of predictive tools to improve the criminal justice system?
-They believe that predictive tools often fail to understand the complexity of human behavior and risk, and may over-rely on flawed data that reinforces existing biases in the system.
What is the role of prediction in criminal justice, and why is it problematic?
-Prediction is used to foresee future actions, like the likelihood of reoffending, but it is problematic because it is based on biased historical data that may not accurately reflect individual or community realities.
What is a more forward-looking approach to criminal justice reform mentioned in the discussion?
-A forward-looking approach involves using causal inference methods to evaluate the effectiveness of interventions, rather than relying on predictive tools that reflect past enforcement patterns. This would focus on identifying what policies and changes actually work to improve outcomes.
Outlines
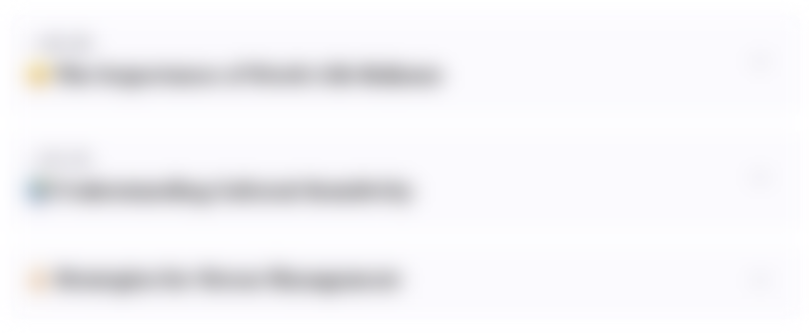
このセクションは有料ユーザー限定です。 アクセスするには、アップグレードをお願いします。
今すぐアップグレードMindmap
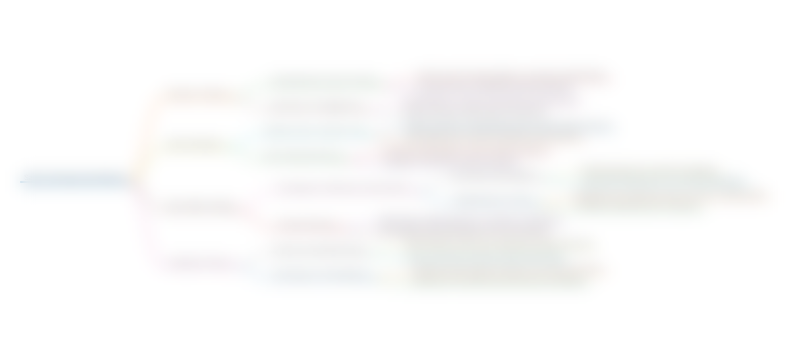
このセクションは有料ユーザー限定です。 アクセスするには、アップグレードをお願いします。
今すぐアップグレードKeywords
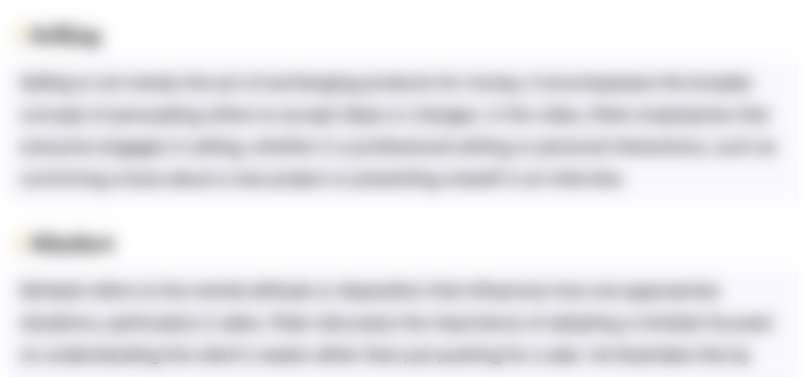
このセクションは有料ユーザー限定です。 アクセスするには、アップグレードをお願いします。
今すぐアップグレードHighlights
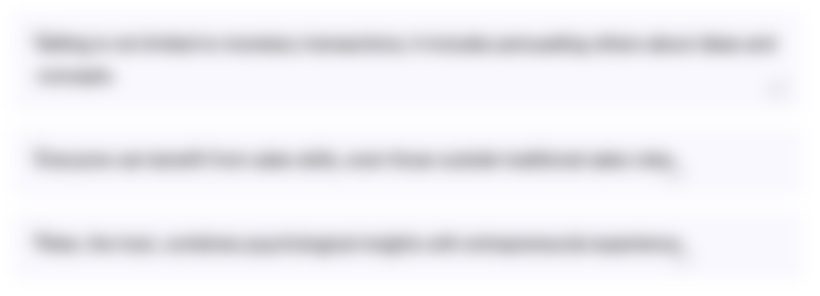
このセクションは有料ユーザー限定です。 アクセスするには、アップグレードをお願いします。
今すぐアップグレードTranscripts
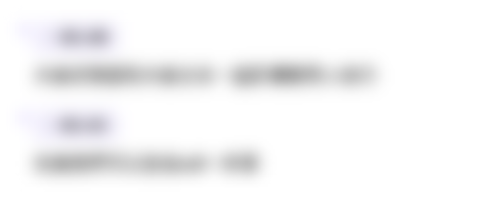
このセクションは有料ユーザー限定です。 アクセスするには、アップグレードをお願いします。
今すぐアップグレード関連動画をさらに表示
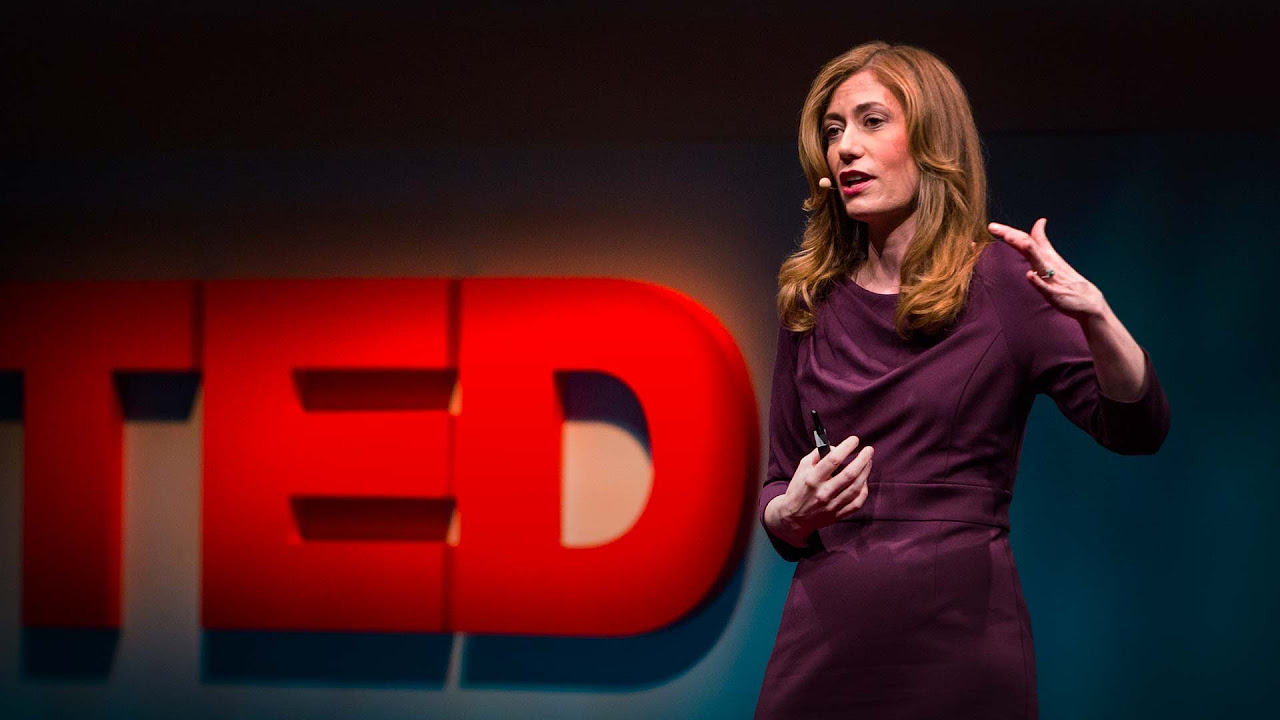
Anne Milgram: Why smart statistics are the key to fighting crime
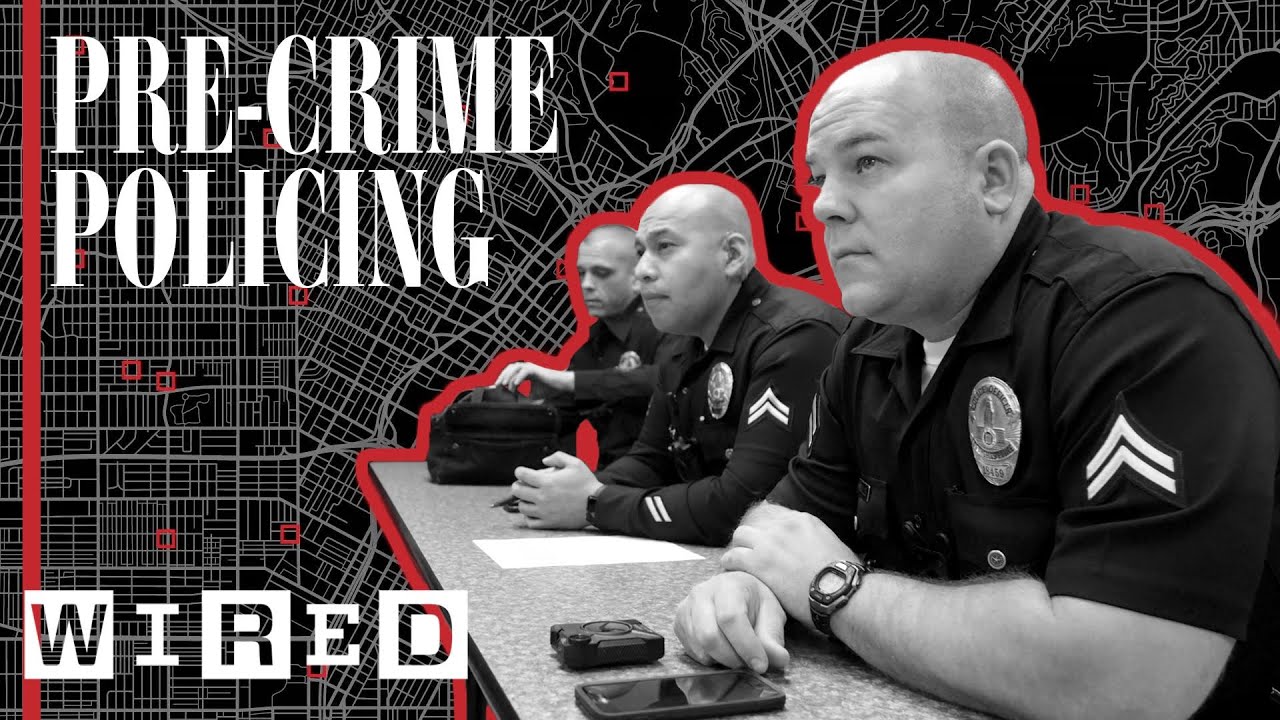
How Cops Are Using Algorithms to Predict Crimes | WIRED
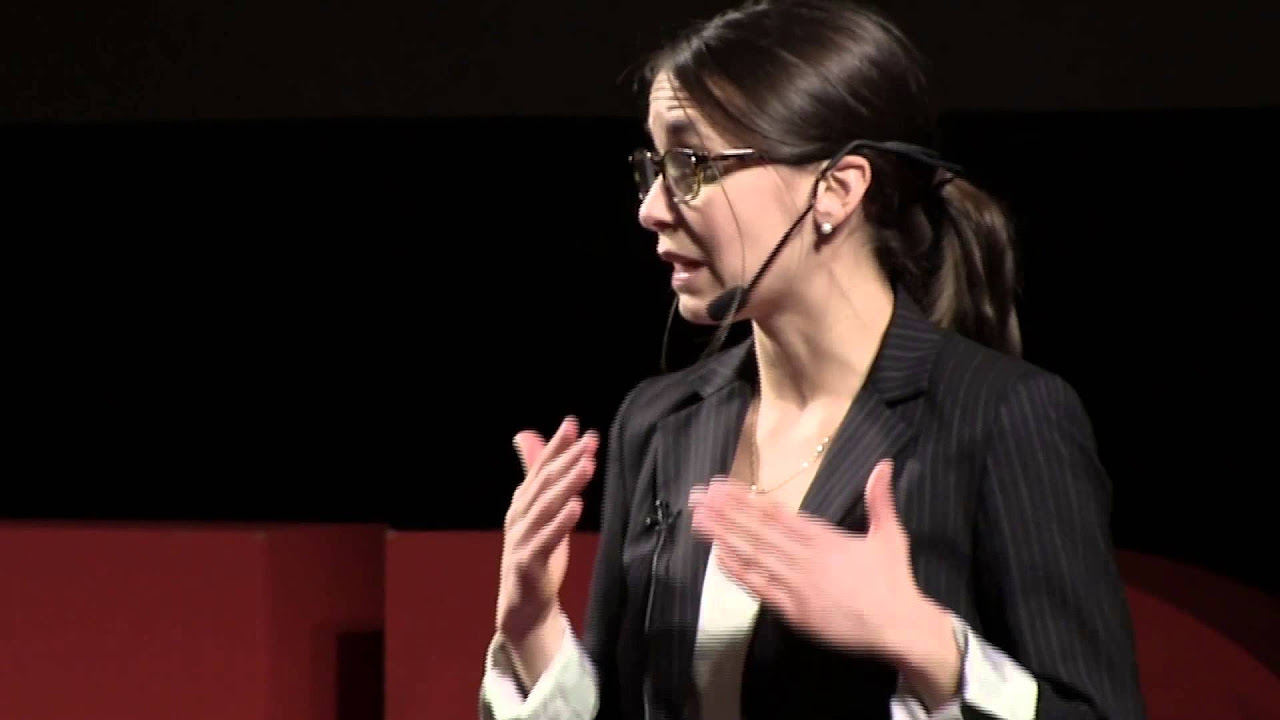
What if justice was something we felt | Ardath Whynacht | TEDxConcordia
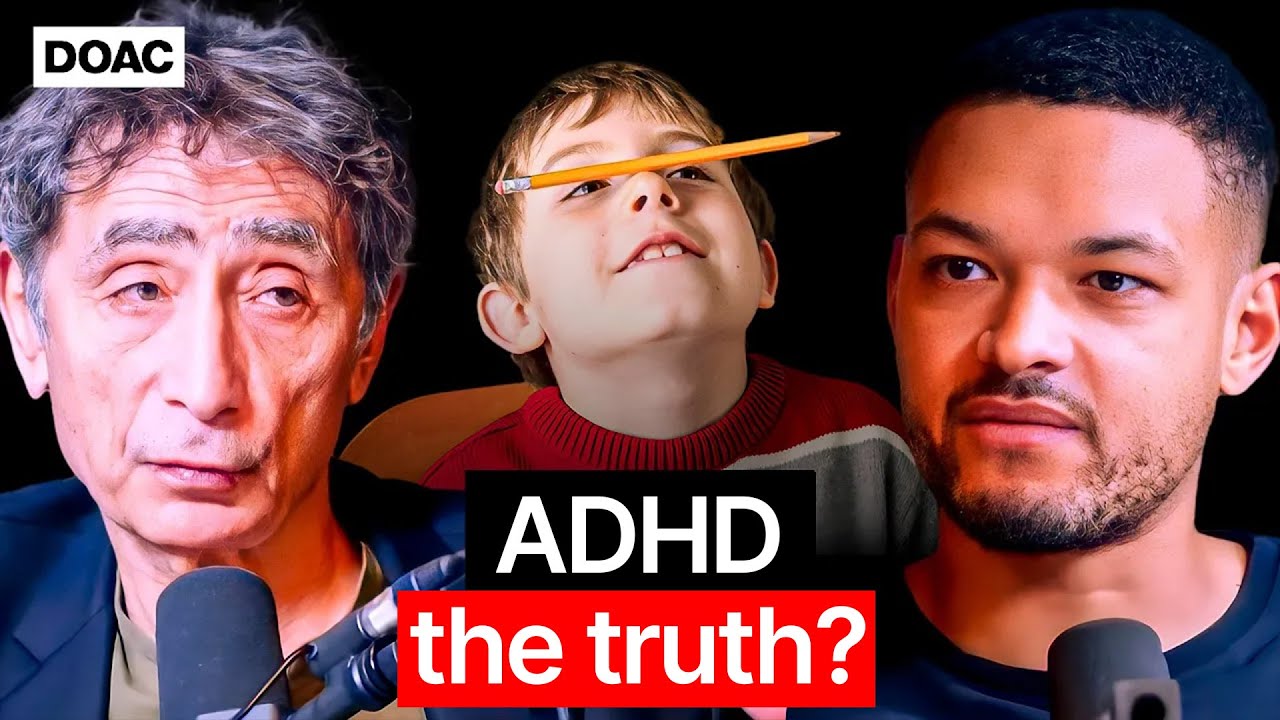
World Leading Physician View On ADHD: Gabor Mate

Algorithmic Bias Explained

Pengamat Politik: Omnibus Law Memperparah Ketimpangan di RI
5.0 / 5 (0 votes)