System Design: Apache Kafka In 3 Minutes
Summary
TLDRThis video provides an overview of Apache Kafka, a distributed streaming platform used for real-time data pipelines and streaming applications. Originally developed at LinkedIn, Kafka is now a critical component of modern architectures, enabling scalable, real-time data streaming. The script highlights Kafka's key features, such as its ability to handle massive data volumes, flexibility, and fault tolerance. It also covers common use cases, including activity tracking, microservices communication, and big data stream processing, while noting Kafka's complexities and resource requirements. Subscribe to the ByteByteGo newsletter for more insights on system design.
Takeaways
- 🌟 Apache Kafka is a distributed streaming platform designed for building real-time data pipelines and streaming applications at scale.
- 🚀 Originally developed by LinkedIn, Kafka was created to handle high volumes of event data with low latency and was open-sourced in 2011.
- 📚 Kafka organizes event streams into topics distributed across multiple brokers, enhancing data accessibility and resilience.
- 📦 Producers feed data into Kafka, while consumers retrieve it, highlighting Kafka's role in decoupling data flow for independent operation.
- 🔥 Kafka's strength is its ability to manage massive data volumes, offering flexibility and fault tolerance compared to simpler messaging systems.
- 🔑 Kafka is a critical component in modern system architectures due to its real-time, scalable data streaming capabilities.
- 📈 Kafka serves as a reliable, scalable message queue, decoupling data producers from consumers for efficient operation at scale.
- 👣 Ideal for activity tracking, Kafka is used by companies like Uber and Netflix for real-time analytics of user activities.
- 🔌 Kafka consolidates disparate data streams into unified pipelines, useful for aggregating IoT and sensor data for analytics and storage.
- 🌐 In microservices architecture, Kafka acts as a real-time data bus, facilitating communication between different services.
- 👀 Kafka enhances monitoring and observability when integrated with the ELK stack, collecting real-time metrics and logs for system health analysis.
- 🔧 Kafka enables scalable stream processing of big data, handling massive real-time data streams for various applications like product recommendations and anomaly detection.
- 🚧 Despite its strengths, Kafka has limitations, including a steep learning curve, requiring expertise for setup, scaling, and maintenance.
- 💡 Kafka can be resource-intensive, necessitating substantial hardware and operational investment, which may not be suitable for smaller startups.
- ⏱️ Kafka is not ideal for ultra-low-latency applications, such as high-frequency trading, where microseconds are crucial.
Q & A
What is Apache Kafka, and what is its primary purpose?
-Apache Kafka is a distributed streaming platform designed to build real-time data pipelines and streaming applications at a massive scale. It was originally developed at LinkedIn to solve the problem of ingesting high volumes of event data with low latency.
How are event streams organized in Kafka, and why is this important?
-Event streams in Kafka are organized into topics that are distributed across multiple servers called brokers. This organization ensures that data is easily accessible and resilient to system crashes, making Kafka highly reliable.
What roles do producers and consumers play in the Kafka ecosystem?
-In the Kafka ecosystem, producers are applications that feed data into Kafka, while consumers are applications that consume data from Kafka. This decouples the data producers from consumers, allowing them to operate independently and efficiently at scale.
What are some common use cases for Kafka?
-Common use cases for Kafka include activity tracking (e.g., ingesting real-time events like clicks, views, and purchases), consolidating data from multiple sources into unified real-time pipelines, serving as a data bus in microservices architecture, and enabling scalable stream processing of big data.
Why is Kafka particularly suited for activity tracking?
-Kafka is ideal for activity tracking because it can ingest and store real-time events like clicks, views, and purchases from high-traffic websites and applications. Its ability to handle massive amounts of data in real-time makes it perfect for analytics in scenarios like those used by Uber and Netflix.
How does Kafka support monitoring and observability?
-Kafka supports monitoring and observability by collecting metrics, application logs, and network data in real-time. When integrated with tools like the ELK stack, this data can be aggregated and analyzed to monitor overall system health and performance.
What limitations does Kafka have?
-Kafka has several limitations, including its complexity and steep learning curve, the need for expertise in setup, scaling, and maintenance, and its resource-intensive nature, requiring substantial hardware and operational investment. It is also not suitable for ultra-low-latency applications like high-frequency trading.
In what scenarios might Kafka not be the ideal solution?
-Kafka might not be ideal for smaller startups due to its resource-intensive nature and complexity. It is also not suitable for ultra-low-latency applications, such as high-frequency trading, where microseconds matter.
How does Kafka enable scalable stream processing of big data?
-Kafka's distributed architecture allows it to handle massive volumes of real-time data streams, making it ideal for scalable stream processing. Examples include processing user clickstreams for product recommendations, detecting anomalies in IoT sensor data, and analyzing financial market data.
What sets Kafka apart from simpler messaging systems?
-Kafka's ability to handle massive amounts of data, its flexibility to work with diverse applications, and its fault tolerance set it apart from simpler messaging systems. These features make Kafka a critical component of modern system architectures, especially for scalable, real-time data streaming.
Outlines
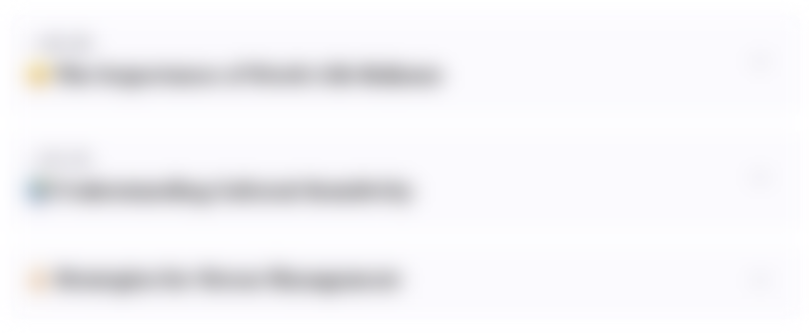
Cette section est réservée aux utilisateurs payants. Améliorez votre compte pour accéder à cette section.
Améliorer maintenantMindmap
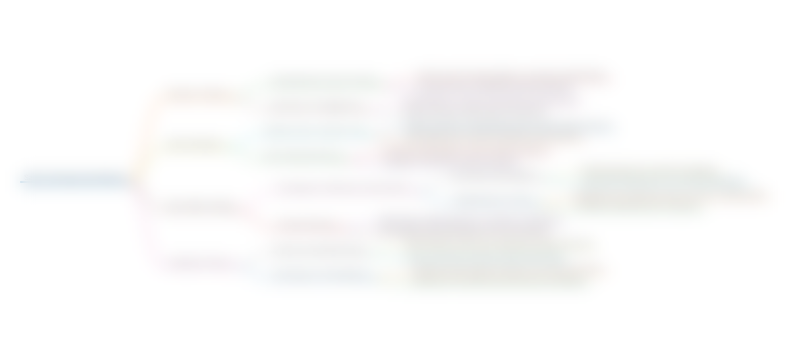
Cette section est réservée aux utilisateurs payants. Améliorez votre compte pour accéder à cette section.
Améliorer maintenantKeywords
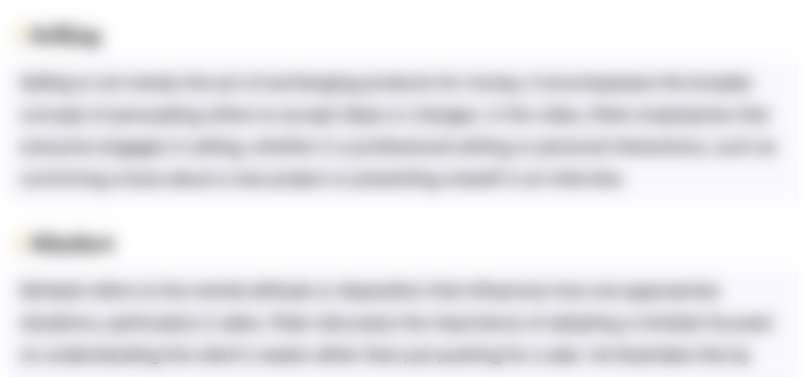
Cette section est réservée aux utilisateurs payants. Améliorez votre compte pour accéder à cette section.
Améliorer maintenantHighlights
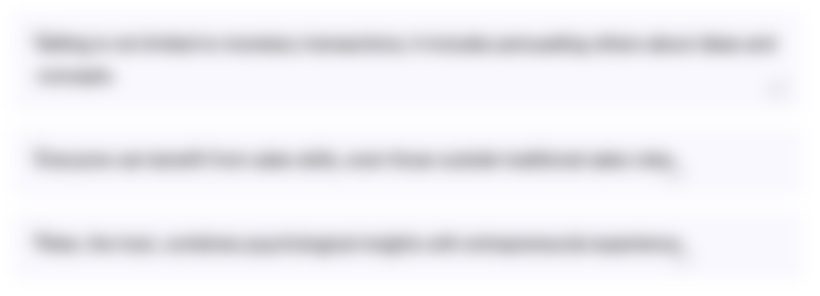
Cette section est réservée aux utilisateurs payants. Améliorez votre compte pour accéder à cette section.
Améliorer maintenantTranscripts
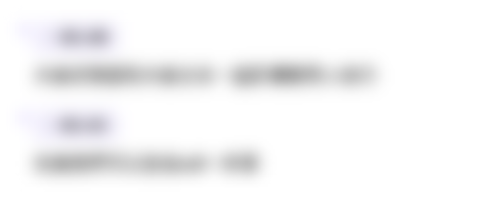
Cette section est réservée aux utilisateurs payants. Améliorez votre compte pour accéder à cette section.
Améliorer maintenantVoir Plus de Vidéos Connexes
5.0 / 5 (0 votes)