STAT115 Chapter 5.3 Multiple Hypotheses Testing and False Discovery Rate
Summary
TLDRThe transcript discusses the challenge of multiple hypothesis testing in high-throughput experiments, particularly when analyzing differential gene expression across 20,000 genes. It emphasizes the importance of controlling for false positives using techniques like the Bonferroni correction and False Discovery Rate (FDR). The speaker explains how FDR provides a less conservative approach compared to family-wise error rate, making it useful in identifying genuinely differentially expressed genes. The discussion also covers strategies for reducing noise in data and improving the accuracy of differential gene expression analysis.
Takeaways
- 🧬 High-throughput experiments can involve testing differential expression across thousands of genes, necessitating a method to determine which genes are significantly different.
- 📉 Relying solely on p-values for multiple hypothesis testing can lead to false positives due to the large number of tests, even if no genes are truly differentially expressed.
- 🎯 The Bonferroni correction is a conservative approach to control for the family-wise error rate, adjusting the p-value threshold to avoid any false positives, but it may be too strict.
- 🔍 False Discovery Rate (FDR) offers a less conservative approach than the family-wise error rate, allowing a small percentage of false positives while maintaining overall accuracy.
- 📊 FDR is calculated as the proportion of false positives among all the genes called significant, which helps in determining an acceptable balance between true and false positives.
- 🧪 The FDR value is always higher than the corresponding p-value because it accounts for the likelihood of false positives in multiple testing scenarios.
- 🚫 Noisy data can lead to a uniform p-value distribution, indicating that no genes are significantly differentially expressed; reducing the number of hypotheses can help mitigate this.
- 🔬 In RNA-seq experiments, genes with low expression levels in too few samples can be filtered out to reduce noise and improve the signal-to-noise ratio.
- 💡 The key to successful differential expression analysis is to correctly balance the stringency of statistical tests to avoid too many or too few significant results.
- 📚 The concept of multiple hypothesis testing has become increasingly important with the advent of high-throughput sequencing, emphasizing the need for statistical rigor in biological research.
Q & A
What is the primary concern when testing the expression of a large number of genes in a high-throughput experiment?
-The primary concern is controlling for multiple hypothesis testing, as testing a large number of genes (e.g., 20,000) can lead to a significant number of false positives due to random chance.
Why can't a simple p-value cutoff be used to identify differentially expressed genes in large-scale experiments?
-Using a simple p-value cutoff, such as 0.01, can lead to many false positives in large-scale experiments because, with a large number of tests, some genes will appear to be differentially expressed purely by chance.
What is the family-wise error rate (FWER) and how is it controlled?
-The family-wise error rate is the probability of making even one false positive call among all the hypotheses tested. It is controlled by using methods like the Bonferroni correction, which adjusts the p-value cutoff by dividing it by the number of tests performed.
Why might the Bonferroni correction be too conservative in some cases?
-The Bonferroni correction can be too conservative, especially when the data is noisy or there are few replicates, making it difficult to detect any differentially expressed genes because the p-value cutoff becomes very stringent.
What is the false discovery rate (FDR) and why is it preferred over the family-wise error rate in some experiments?
-The false discovery rate (FDR) is the expected proportion of false positives among all the genes called differentially expressed. It is preferred over the family-wise error rate in some experiments because it allows for some false positives, which is often acceptable in large-scale studies where controlling the FWER would be too stringent.
How is the FDR estimated in practice?
-FDR is estimated by looking at the distribution of p-values across all tests. The Benjamini-Hochberg method is a commonly used approach that estimates FDR by extrapolating the level of noise (false positives) from the right side of the p-value distribution and comparing it to the signal (true positives).
What is the significance of the p-value distribution in determining the quality of a statistical test in gene expression analysis?
-The p-value distribution helps determine the quality of a statistical test. A uniform distribution suggests no true signal, while a skewed distribution toward smaller p-values indicates the presence of differentially expressed genes. If the distribution is flat on the right side, it suggests the noise level is low, making the statistical test more reliable.
What does it mean if the p-value distribution is uniform in an RNA-seq experiment?
-If the p-value distribution is uniform, it suggests that the data is too noisy and that there are no significant differentially expressed genes in the experiment.
How can the number of hypotheses tested in an RNA-seq experiment be reduced to improve the signal-to-noise ratio?
-The number of hypotheses tested can be reduced by filtering out genes with low expression levels in too few samples, as these genes are unlikely to reach statistical significance. This reduces the noise and increases the likelihood of detecting true signals.
What role do full change and FDR play in reporting differentially expressed genes?
-Full change and FDR are often used together in reporting differentially expressed genes. FDR helps control for false positives, while filtering by full change ensures that only genes with a biologically meaningful change in expression are reported. This approach provides a more accurate and relevant list of differentially expressed genes.
Outlines
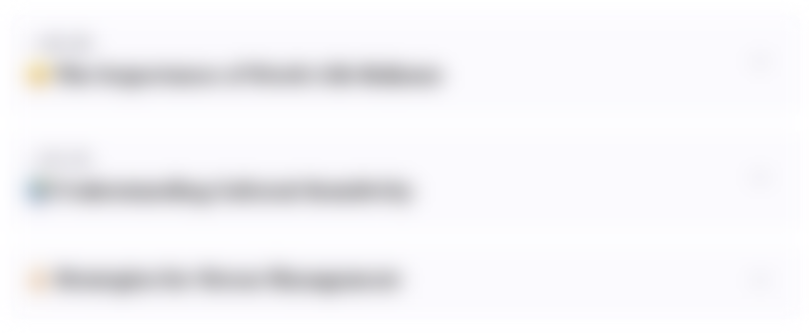
Cette section est réservée aux utilisateurs payants. Améliorez votre compte pour accéder à cette section.
Améliorer maintenantMindmap
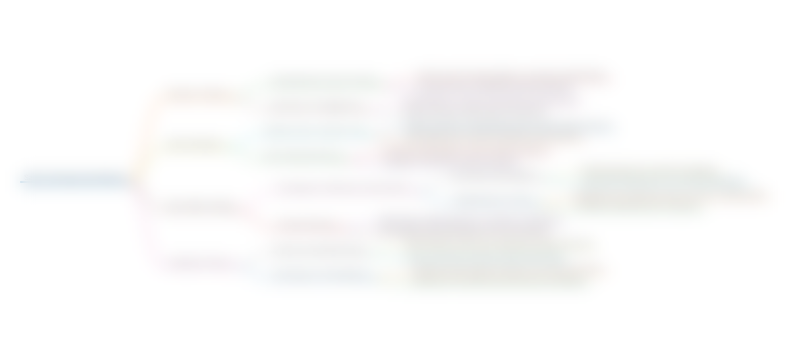
Cette section est réservée aux utilisateurs payants. Améliorez votre compte pour accéder à cette section.
Améliorer maintenantKeywords
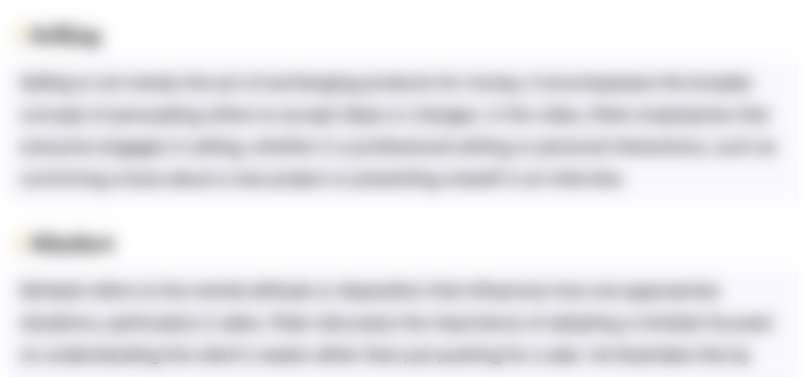
Cette section est réservée aux utilisateurs payants. Améliorez votre compte pour accéder à cette section.
Améliorer maintenantHighlights
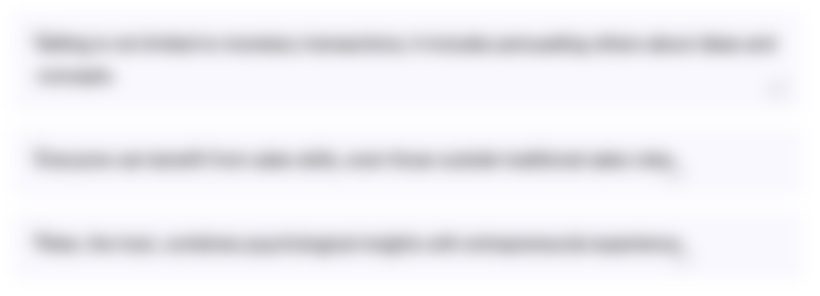
Cette section est réservée aux utilisateurs payants. Améliorez votre compte pour accéder à cette section.
Améliorer maintenantTranscripts
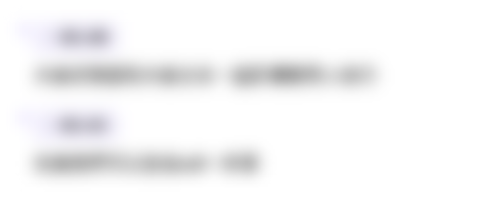
Cette section est réservée aux utilisateurs payants. Améliorez votre compte pour accéder à cette section.
Améliorer maintenantVoir Plus de Vidéos Connexes
5.0 / 5 (0 votes)