Brainpower - Best of replay: Prompt Fundamentals with ChatGPT
Summary
TLDRIn this episode of 'Brain Power', Josh explores the basics of prompting in large language models like GPT and Bard. He demonstrates how to build effective prompts by adding roles, tasks, and detailed instructions to improve the quality of responses from AI models, using the example of creating learning objectives for cooking a smoked potato salad.
Takeaways
- đ§ The episode focuses on the fundamentals of prompting in large language models like GPT, Bard, and Claude.
- đ» It emphasizes the importance of compute power and data in the functioning of large language models.
- đ The script explains how large language models are trained and the role of human intervention in setting guardrails for responses.
- đČ The concept of probability in generating responses from language models is discussed, highlighting the variability of outcomes based on input prompts.
- đ The video introduces the idea of 'zero-shot prompts' where the model is expected to generate a response without prior examples or additional information.
- đ The role of adding a professional role to prompts, such as 'instructional designer', is shown to influence the quality of responses.
- đ The script demonstrates the process of building up a prompt by adding specific tasks and detailed instructions to refine the model's output.
- đ„ A practical example is given using the task of creating learning objectives for cooking a smoked potato salad.
- đ The importance of specificity, measurability, achievability, result-orientation, and time-bound criteria in crafting effective learning objectives is discussed.
- đ The video mentions the availability of additional resources and prompts for learning and development, guiding viewers to access them.
- đ Lastly, the script encourages viewers to follow along with the examples and try using the prompts in their own large language model interactions.
Q & A
What is the main topic of the episode of 'Brain Power'?
-The main topic of the episode is exploring prompt fundamentals and how they work in large language models like chat GPT, Bard, and Claude.
What are the two important aspects of a large language model according to the script?
-The two important aspects are compute power, which is necessary to drive the model and provide results in a timely manner, and data, which includes the corpus of information used to train the model and the guardrails in place with that information.
Why does Josh mention the difference between GPT 3.5 and GPT 4 in terms of speed?
-Josh mentions the difference to highlight that there are variations in the speed of responses between different versions of the model, with GPT 4 being faster than GPT 3.5.
What is a 'zero-shot prompt' as mentioned in the script?
-A 'zero-shot prompt' is a simple request given to the model without any examples or additional information, relying on the model's training to provide a relevant response.
What is the role of 'control statement' in prompting?
-A 'control statement' is used when prompting to ensure that the model understands the context and limitations of the request, preventing inappropriate or irrelevant responses.
Why does Josh create a new chat for each prompt in the exercise?
-Josh creates a new chat for each prompt to avoid any influence from prior prompts, ensuring that each 'zero-shot prompt' is independent and not affected by previous interactions.
What is the purpose of adding a 'role' to the prompt?
-Adding a 'role' to the prompt, such as 'act like an instructional designer', influences the quality of the information returned by the model by aligning the response with the expertise associated with that role.
What are 'SMART' criteria used for in the context of learning objectives?
-SMART criteria are used to describe a learning objective in a way that is Specific, Measurable, Achievable, Result-oriented, and Time-bound, ensuring clarity and effectiveness in the objective.
How does adding detailed instructions to a prompt improve the results from the model?
-Adding detailed instructions to a prompt provides the model with more specific guidance on what is expected, leading to more accurate and relevant responses.
What is the significance of the 'Plinko game' analogy used in the script?
-The 'Plinko game' analogy is used to illustrate the concept of probability in how a large language model generates responses based on the input prompt.
Where can viewers find additional content and support for the episodes?
-Viewers can find additional content and support at Josh Cavalier's website or by accessing the prompts provided at JoshCavalier.com/brainpower.
Outlines
đ§ Introduction to Prompt Fundamentals
In this introductory segment, Josh welcomes viewers to an episode of 'Brain Power' focused on understanding the basics of prompting in large language models such as Chat GPT, Bard, and Claude. He emphasizes the importance of compute power and data in the functioning of these models, highlighting the role of human training to guide responses and ensure safety. Josh also mentions his plan to post additional content to support the episode, inviting viewers to follow along with their own language models and to check out his resources for further learning.
đČ Understanding Probability in Prompt Responses
Josh explains how prompts work in large language models by drawing an analogy to the Plinko game from 'The Price is Right'. He illustrates how the model's responses are based on probability, with certain words having a higher likelihood of being followed by specific others. This randomness can lead to varied outcomes even from the same prompt. He encourages viewers to experiment with free-form and structured prompts, demonstrating the difference between a simple prompt and one that is more refined with additional information.
đ Crafting a Zero-Shot Prompt for Learning Objectives
Josh demonstrates how to create a zero-shot prompt by asking the language model to generate a list of learning objectives for cooking a smoked potato salad. He discusses the limitations of such prompts, which rely heavily on the model's training data. Josh critiques the initial response for its lack of specificity and measurable outcomes, emphasizing the need for clear, detailed objectives. He then shows how to refine the prompt by adding more context and detail to improve the quality of the model's response.
đšâđ« Role of an Instructional Designer in Prompting
Josh explores the impact of adding a role to a prompt, specifically that of an instructional designer, to influence the language model's response. He shows how this role can guide the model to provide more relevant and targeted learning objectives. Josh then further refines the prompt by adding specific details about the task, such as making a visually appealing and tasty smoked potato salad, to elicit more detailed and relevant objectives from the model.
đ Enhancing Prompts with Detailed Instructions
In the final segment, Josh emphasizes the importance of adding detailed instructions to prompts to achieve more precise and actionable learning objectives. He uses the SMART criteria (Specific, Measurable, Achievable, Result-oriented, Time-bound) to guide the language model in generating objectives that are clear and measurable. Josh demonstrates how these detailed prompts can lead to more effective outcomes, showcasing how the model's responses evolve to include time-specific goals and more precise actions.
Mindmap
Keywords
đĄPrompt Fundamentals
đĄLarge Language Models
đĄCompute Power
đĄData Inputs
đĄProbability
đĄFree Form Prompt
đĄStructured Prompt
đĄLearning Objectives
đĄZero-Shot Prompt
đĄSMART Criteria
đĄInstructional Designer
Highlights
Introduction to prompt fundamentals in large language models like chat GPT, Bard, and Claude.
Explanation of the importance of compute power and data in the functionality of large language models.
Discussion on the role of human training in models like GPT 3.5 and GPT 4 to establish response guardrails.
Illustration of how large language models operate based on probability using the Plinko game analogy.
Differentiation between free form and structured prompts for interacting with large language models.
The concept of zero-shot prompts and their reliance on the model's inherent training.
Demonstration of creating a learning objective for cooking a smoked potato salad using chat GPT.
The impact of adding a role (e.g., instructional designer) to a prompt to refine the model's response.
Enhancing prompts by detailing the task to improve the specificity and quality of the model's output.
The significance of using specific instructions within prompts to guide the model's response.
Example of refining a prompt by incorporating SMART criteria for learning objectives.
Observation of improved results when adding detailed instructions to prompts in chat GPT.
The influence of prior prompts on subsequent model responses and the strategy of creating new chats for each prompt.
Josh Cavalier's offer of additional content and resources to support the episode's topics.
Promotion of Josh Cavalier's prompts for Learning and Development and the associated worksheet.
Conclusion summarizing the process of building effective prompts using role, task, and detailed instructions.
Transcripts
in this episode of brain power we are
going to explore prompt fundamentals
let's go ahead and jump in
[Music]
hello everyone it's Josh thanks for
showing up for another edition of brain
power
today we're gonna get really basic and
talk about prompting and how it works in
large language models like chat GPT Bard
and Claude so today's episode we are
going to start with some very simple
prompts and then build them up and talk
about the reasons why you would want to
go in and modify your prompts with
additional information to get back
better results now if you're following
along at home this is actually a
recording typically I will do this show
live live but because of prior
commitments you're now watching a
recording which is happening on January
19th
2024 okay but you could still follow
along with me if you have a large
language model open up like chat GPT
today I'm going to be using GPT 3.5
model so even the free model you can use
here today or if you want to use
Microsoft co-pilot Bard Claude whatever
your flavor of large language model is
you can go ahead and follow along with
the prompts also I will be posting
additional content to support these
episodes they are not up yet but if you
go to Josh
cavaliers. brainpower or Josh
cavalier.pdf
not up yet but coming very soon so you
may want to check it out over the
weekend right okay without further Ado
let's go ahead and let's jump into
prompt fundamentals the first thing I
want to talk
about is how a large language model
works there's really two things that are
incredibly important when it comes to a
large language model the first one is
compute power
right so you need to have the
computational power to drive the large
language model to give you results in an
appropriate period of time if you've
worked with chat GPT you understand that
there are differences between the speed
of 3.5 and four okay so that's the first
one the second
one the data right so the inputs are
incredibly important what information
or Corpus of data was used to train the
model once you have that information
what guard rails are in place with that
information and in regards to chat GPT
and the 35 and four models humans were
used to help train the model and the
responses that were coming back again to
put Protections in so that it isn't the
wild west and you can really ask it
anything that you want uh I know that
when I first started working with chat
GPT you're going to see this in a moment
but I have a control statement that I
use when I
prompt how do I cook a smoked potato
salad well when I first started
prompting I was asking it how do I smoke
a potato and it thought I was really
trying to smoke the potato like a
cigarette and wouldn't allow me to do it
so yes these models are trained and they
are
based on probability I know that for
some of you when you're working with
chat GPT it feels like a Google search
or it's grabbing the information from
the internet and that's not the case at
all um you have to keep in mind that we
are working with a vector database and
everything that the model was trained on
are just points of information and so
when you type in a prompt there are
words that are coming back and it's all
based on probability now what I want to
do is go ahead and show you an example
here and if you have ever watched the
prices right my gosh I hope that you at
least caught one episode The Price is
Right youve probably seen the Plinko
game and the Plinko game is when they
have a chip and the chip drops down and
it's going to go ahead and you know hit
the bottom of the board with an
amount so in this addition of the Plinko
game this is the probability example of
a prompt in chat GPT or really any large
language model and you'll notice that I
have a prompt over here on the Le hand
side that says as I cross the street I
noticed from out of nowhere a
huge what
like what's going to come up next in
regards to the response from chat GPT
well based upon probability if I use the
word
black or if I use the word huge the next
word could be dog it could be black it
could be rain it could be bird all these
various different options are possible
and again it's all based on probability
now some words have higher probability
than others and in this instance dog so
if I get a response back from chat GPT
from out of nowhere a huge
dog was running towards
me again that could be completely
different based upon various factors and
what the model comes back with and so if
you have worked with chat gbt and you're
wondering well why
am I getting a different response from
the same
prompt well this is the reason why okay
again probability kicks in and all it
takes is a single word or a different
word to appear to give you a completely
different
result all right so now would be the
time that you would want to open up chat
GPT or whatever large language model
you're working with and I'm going to put
some prompts in here again you can type
these prompts in if you want or once the
documents are uploaded you could
download the sheet and copy and paste it
from the
worksheet so this concept I first want
to talk about is a free form prompt
versus a structured prompt if you take a
look at the prompt over on the left hand
side you could see that it's just simply
sentences it's just like plain language
to the model that you're giving it
within the prompt over on the right hand
side is a structured prompt I'm not
going to get into that today but there
are some Advanced Techniques that you
can use to get a more refined response
with additional information within the
prompt for today because we are talking
about prompt fundamentals we just want
to keep it simple and begin with a free
form prompt just by writing
sentences all right so today we are
going to be working with a learning
objective and learning objectives are
extremely important if you're an
instructional designer uh again just
giving Focus to the uh instructional
content uh that you are creating and
making sure that it's very specific and
measurable and that you have guidance uh
towards those objectives again whether
it's knowledge skill attitude Behavior
whatever the case may be so that is the
topic for today is creating a learning
objective but we're going to do it in
chat
GPT all right so now out of the gate
let's just go ahead and open up chat
GPT and
just start with a very simple request
all right so I'm going to go ahead and
switch
over to chat
GPT and again it's the it's the 35 model
here right
so uh it's not the four it's the again
the free model so that's
fine and now we're going to give it a
very simple
prompt now remember I I do have a
control statement that I use so I'm
going to add that in here and so for
this
prompt I'm going to go ahead and
say create a list of learning objectives
to cook a smoked potato
salad seems like it's going to work I
mean you know there's nothing um crazy
about this prompt it's just a simple
request
when you uh when you create a
prompt and you give no examples or
additional
information about that
prompt uh this is what we call a zero
shot prompt okay what we're doing is
we're leaning really hard on the model
to again we hope that learning
objectives was trained in the model
somewhere
right uh if it wasn't you're going to
get back really horrible results but uh
you know with 175 billion parameters in
the 35 model and over a trillion
parameters in the gp4 model odds are
learning objectives and its definition
are in there but now you're going to see
the results that are going to come back
and how we are going to coax the model
to give us better results as we prompt
so out of the gate let's go ahead and
try this simple prompt create a list of
learning objectives to cook a smoked
potato salad and let's let it rip and
see what
happens all right so you know one of the
first things that I see here uh with
these learning objectives is one it does
give me many different bits of criteria
uh about this smoked potato salad if I
move back up to top you can see that the
topics include safety cautions selecting
ingredients potato preparation smoking
techniques and so on but the language in
here it could be way better um things
like or words like learn and
understand uh that doesn't really give
me anything specific about the task at
hand uh as far as it's measurement right
and so I'm looking for some learning
objectives again that are very specific
that are measurable achievable result
oriented time bound uh if it's specific
task and I want more detailed learning
objectives so I mean this is a good
start actually I was it's pretty
impressive how um it has all these
different uh criteria in here listed
okay so not too bad now for this
particular exercise I am going to create
a brand new chat for each prompt and the
reason for that is I don't want any kind
of influence from a prior prompt when
you're prompting in chat
GPT you have to keep in mind that when
you use words and a prompt and then you
get a result back all of those words
your prompt and the return result are
going to influence the
conversation the words that are in there
and related words have a higher
probability of showing up so I want to
again for this exercise create zero shot
prompts every single time so you could
see the difference between the results
now if I were to go ahead and attempt to
ask it and coax it towards better
results in here I could do that in the
same conversation this is what we call a
prompt chain but again we want to remove
that and just see
with the zero shot prompt what we get by
modifying our prompts each time all
right so let's go
back now I want to go ahead and create a
brand new
conversation and this time we are going
to add in
here a
roll we're going to add a role to the
prompt and in this case it's going to be
act like an instructional designer
now you know when we're talking about
you know adding or uh you know modifying
a
prompt in this case by adding a role the
words instructional designer beside
themselves is going to influence the
quality of the information coming back
just because there are words related to
instructional designer that deal with
learning objectives hopefully it's
trained that way in the model but
because you know these models are
trained on all kinds of information on
the internet odds are that relationship
is there right so again we are now going
to add a role in here and take a look at
the results all right so we'll go back
over to chat
GPT and we'll modify the
prompt in here and say act like an
instructional designer create a list of
learning objectives to cook a smoked
potato salad and let's take a look at
the
results all right
well very interesting it looks
like we have objectives in here and it's
going to go in and give us certain
outcomes
so we do have
some modifications of the learning
objective by the end of the lesson okay
that's that's good um Learners will be
able to identify and gather all
necessary ingredients for making a
smoked potato salad all right not too
bad I mean we could be better here with
the learning objective uh but again we
can still see language in here Learners
will
understand all right these are a little
bit
better but we can continue continue to
modify our prompt and uh to get better
results in there right and we want to
continue again with our experimentation
and building up until we have extremely
good quality learning objectives so the
next item
here that we want to talk
about is the task
itself now I mean you could keep it
simple in regards to the task but the
more
information the more details that you
give about the task is going to give you
better results so let's go ahead and go
back and we're going to add some
additional details to the task and take
a look at the results from chat GPT so
back over we go we're going to create a
brand new chat and for this particular
prompt we'll keep in there act as an
instructional
designer create list of learning
objectives to cook a smoked potato salad
that is visually appealing and
tastes fantastic to your
guests all right so there is
the
prompt and I apologize for not switching
the screen but there it is now that you
have
it let's run it and see what we get
okay so now we have each you know bit of
criteria in here and we have identify
and select key ingredients explain the
role of each ingredient demonstrate safe
and efficient knife skills describe
select but you can still see we still
have like understand in
here I'm looking for better verbs than
that but these are getting better all
right you can see that you know if we
actually could combine uh some of these
bullet points together into one sentence
uh we probably would
have uh some decent learning objectives
again depending upon the criteria in
here all right so there's that but again
the additional words of visually
appealing and tastes fantastic is going
to
influence the criteria and the
information within those learning
objectives all right now let's take a
look at this last bit of information
here
so one additional thing that you can add
to a prompt in addition to the task are
very specific
instructions and so in this example it
says learning objectives are only one
sentence learning objectives contain a
goal Behavior Criterion and conditions
now depending upon where you learned how
to write a learning objective um it
could vary depending upon again what you
are looking for in that learning
objective um now in our prompt today we
are going to use the smart which is
specific measurable achievable result
oriented in time bound uh criteria or
the description of a learning objective
and so that's what we're going to be
using here
today and those are going to be our
detailed instructions for the learning
objectives so back over to chat GPT we
go we are going to create a brand new
chat and for this last prom
prompt let me go in and grab that
prompt and paste it in
here act like an instructional designer
create a list of learning objectives to
cook a smoke potato salad that is
visually appealing and tastes fantastic
to your guest learning objectives are
specific measurable achievable result
oriented and time
bound learning objectives are only one
sent
so those are our detailed information
and you know it
really doesn't matter what task you are
trying to perform within chat
GPT the
role the task and the detailed
instructions alone if you add those
items in there are going to give you
fantastic
results okay and you'll notice that
again as we've built this prompt up and
we've added that additional information
in there we have gotten back better
results now it should be interesting
here when we run this prompt especially
the time specific I think you're going
to see in these learning objectives that
there's going to be time values
associated with some of these tasks or
some of these learning objectives well
let's check it out and see if it happens
so back we go to chat GPT let's go ahead
and let's run this
yeah so we definitely have instances
where time is now a factor by the end of
this cooking lesson Learners will be
able to identify and gather the
necessary ingredients for smoked potato
salad within 15 minutes Learners will be
able to properly wash peel and diced
potatoes ensuring uniformity and size
after following the provided
instructions Learners will be able to
prepare a flavorful marinade for the
potatoes consisting of measurable
quantities of herbs and spices you get
the idea
H well well well so now we have some
decent learning objectives in here uh
you could see that verbs like understand
and learn uh and even describe are not
even in there anymore U and we have some
decent learning objectives that we can
then use in our project so hopefully
today this gives you a really good idea
of
how to use
prompts first by just going in and
requesting or having a conversation with
chat GPT but then building
up building up the prompt by adding a
role of any professional doesn't have to
be instructional designer right it could
be an accountant it could be a marketing
professional whoever out in the world is
very proficient at that task you can put
in for the role
then we go in and we have a very
specific task in there right and you and
you want to be detailed with that and
any criteria or any specifics about that
task and in the case of the learning
objective we had those smart uh criteria
in there for the learning objective you
want to add that in
there and that's going to go ahead and
give you a better result so hopefully uh
today you were able to go in and and see
how to create a prompt and build it up
using roll task and detailed
instructions I want to thank you for
joining me today uh just a
couple items here uh before we roll out
uh again you can find me up at Josh
cavaliers. or Josh
cavaliers. uh also if you want to go
ahead and download or get access to some
prompts I do have 150 prompts right now
eventually within a few weeks that's
going to change to 250 prompts uh but
this link is going to work right now uh
you can just put your name in email in
there and you get access to a notion
site with 150 prompts for Learning and
Development and finally you'll be able
to go ahead and go to josh.com
brainpower and download the associated
worksheet with these episodes so with
that again thank you so much for joining
me and I hope to see you here in the
next episode take care
Voir Plus de Vidéos Connexes
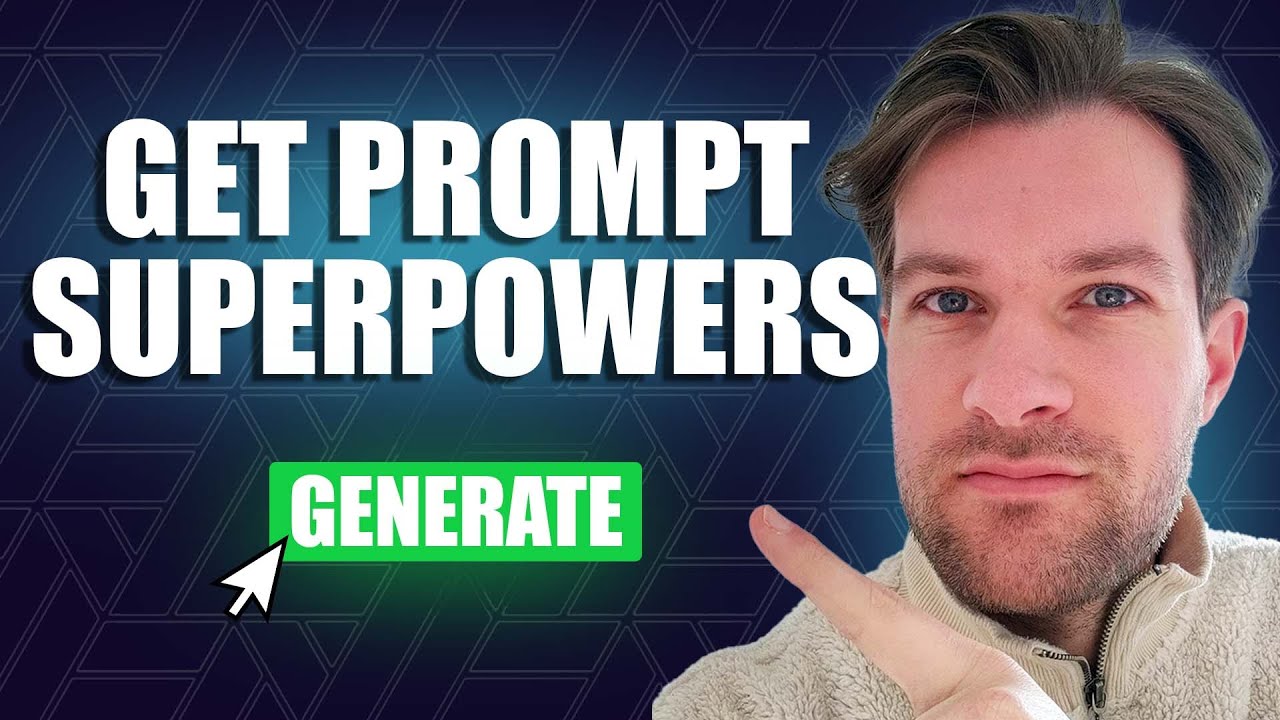
The Perfect Prompt Generator No One Knows About
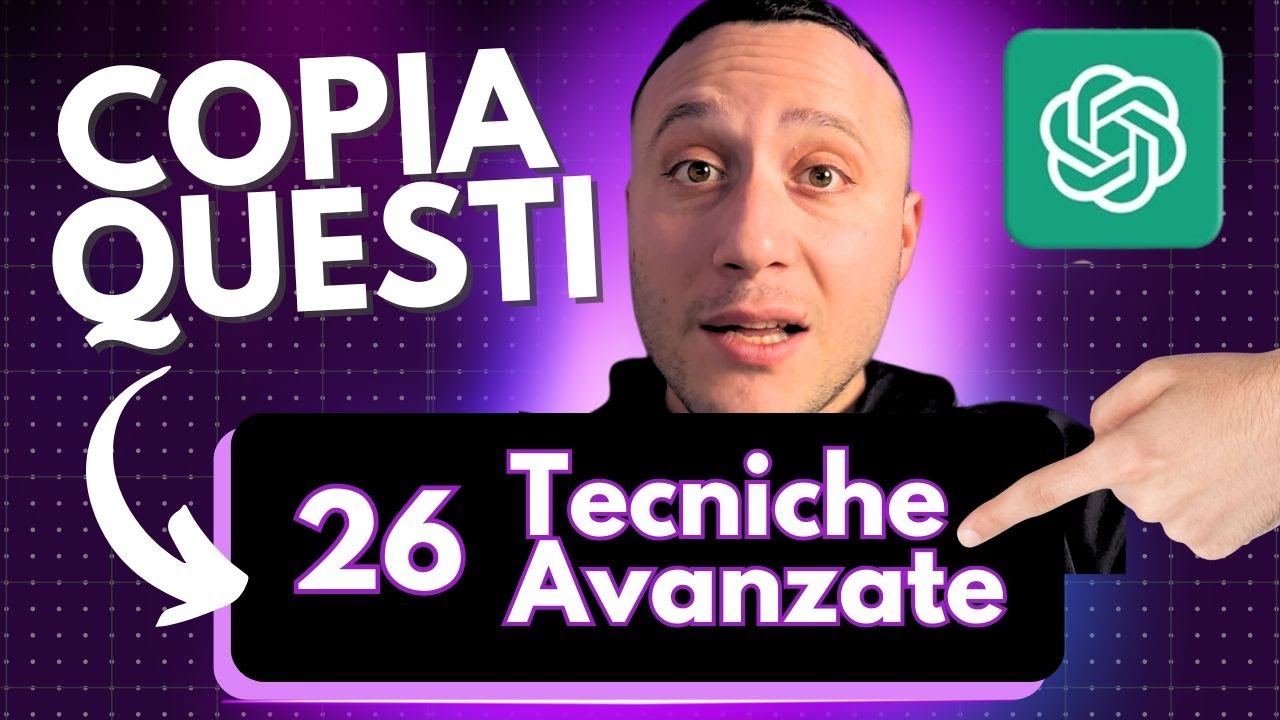
26 prompt ChatGPT +50% di qualitĂ â

Mastering Summarization Techniques: A Practical Exploration with LLM - Martin Neznal
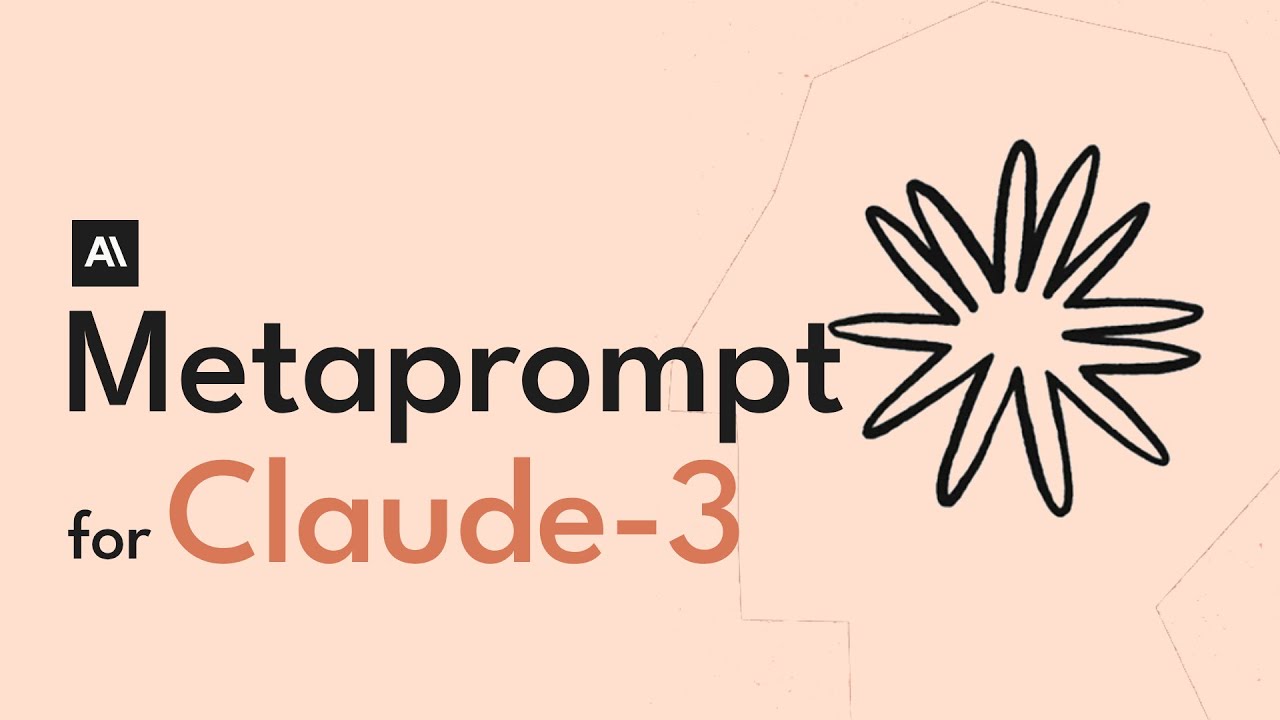
Anthropic's Meta Prompt: A Must-try!
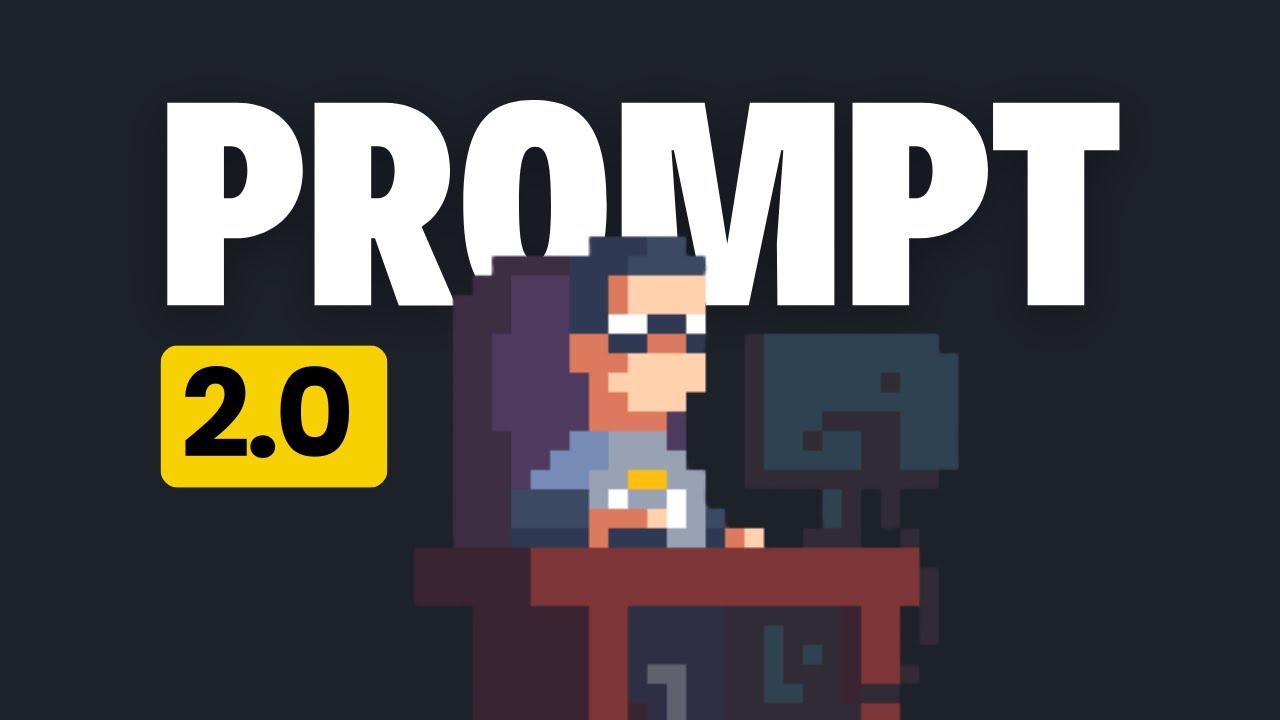
"Next Level Prompts?" - 10 mins into advanced prompting
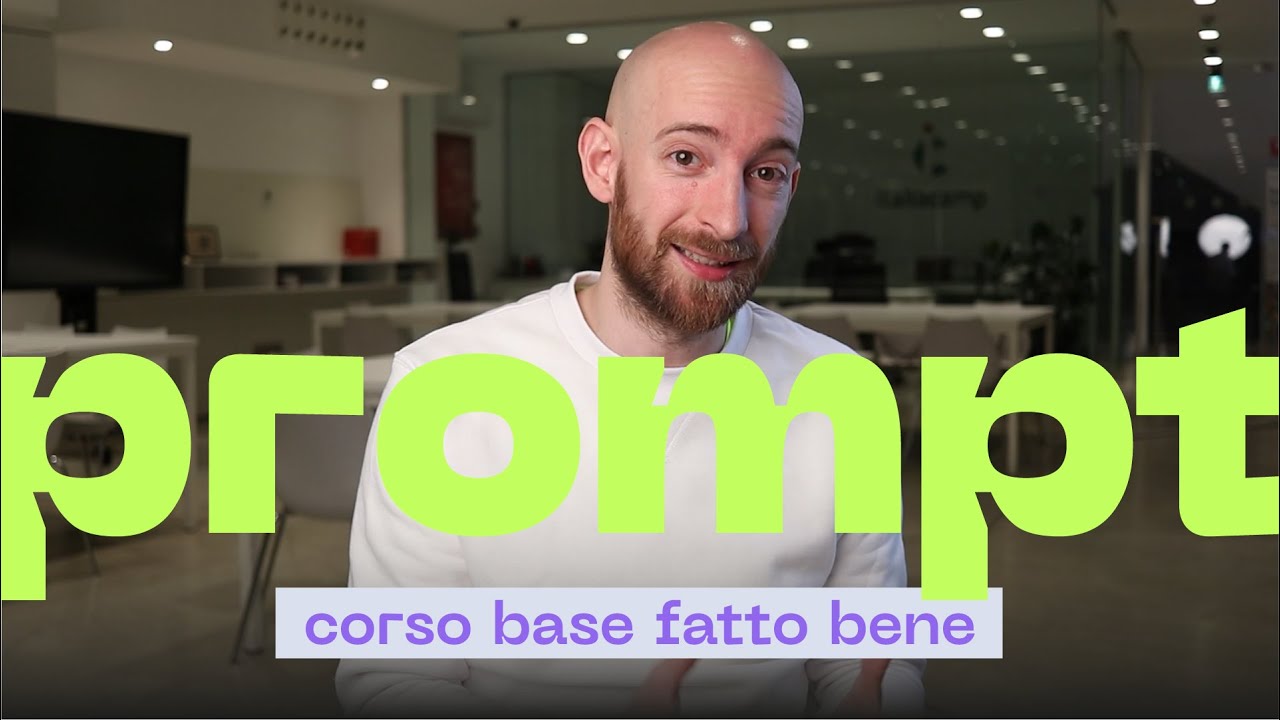
Prompt Engineering - Corso professionale Parte 1/2
5.0 / 5 (0 votes)