Stable Cascade released Within 24 Hours! A New Better And Faster Diffusion Model!
Summary
TLDRThe video discusses the latest AI diffusion model, Stable Cascade, released by Stability AI. The model is built on the Versatile architecture, which allows for faster training with smaller pixel images and produces high-quality images. It supports Latent Control Net IP and LCM, and has been compared favorably to other models in terms of prompt alignment and aesthetic quality. The video demonstrates the model's ability to handle complex text prompts and generate detailed images, showcasing its potential for future AI animations. However, it is currently for research purposes only and not yet available for commercial use.
Takeaways
- 🚀 Stable Cascade is a new AI diffusion model released by Stability AI, showcasing rapid advancements in AI development.
- 🌟 The model is built upon the Verchin architecture, which allows for faster training with smaller pixel images, leading to more efficient image generation.
- 📈 Stable Cascade uses a 24x24 pixel encoding, which is 42 times smaller in training data compared to traditional stable diffusions, enhancing processing speed.
- 🔍 The model supports Latent Control Net (LCN) and LCM, offering more control over image generation and potentially enabling advanced features like face swapping.
- 🔗 A demo page is available for testing the Stable Cascade model, allowing users to experiment with the new diffusion model's capabilities.
- 📝 The model separates the image generation process into three stages: latent generator, latent decoder, and refinement, improving the quality and detail of the final image.
- 🎨 Evaluations show that Stable Cascade outperforms other models in prompt alignment and aesthetic quality, offering better image recognition and handling of multiple elements in text prompts.
- 📊 The model introduces advanced options such as prior guidance scale, prior inference steps, and decoder guidance scale, providing users with more control over the image generation process.
- 📸 Users can input text prompts in a more natural language manner, which the model handles effectively, generating images that closely align with the input prompts.
- 🚫 It's important to note that Stable Cascade is not yet available for commercial purposes and is intended for research and testing at this stage.
- 🔄 The model's capabilities suggest potential future applications in AI animations, offering higher quality and more detailed images compared to current models.
Q & A
What is the name of the new AI diffusion model discussed in the transcript?
-The new AI diffusion model discussed is called 'Stable Cascade'.
Which company developed the Stable Cascade AI model?
-The Stable Cascade AI model was developed by Stability AI.
What is the basis for the Stable Cascade model's architecture?
-The Stable Cascade model is built upon the Verchin architecture.
What is the advantage of using a smaller pixel size for the encoder training in the Stable Cascade model?
-Using a smaller pixel size for the encoder training allows for faster processing and a reduction in training data size, which is 42 times smaller compared to traditional stable diffusions.
How does Stable Cascade support image generation with text input?
-Stable Cascade separates the image generation process into three stages: latent generator, latent decoder, and refinement. It uses text input to generate brief ideas of the image in the latent generator stage, decodes it into pixel representations in the latent decoder stage, and refines the objects in the final stage to produce the full image.
What is the significance of the ControlNet and LCM support in Stable Cascade?
-Support for ControlNet and LCM allows for more precise control over facial identity and other elements during the image generation process, including the ability to handle face swap features within the model.
How does Stable Cascade compare to previous models in terms of prompt alignment and aesthetic quality?
-Stable Cascade outperforms older models in prompt alignment and has a better aesthetic quality score than most, except for Playground version 2, which has a slightly higher score.
What is the current status of Stable Cascade's compatibility with web UI systems like Automatic1111 or Comy UI?
-As of the time of the transcript, Stable Cascade has not been officially released for support in Automatic1111 or Comy UI. However, updates may come in the future to support these systems.
What are the advanced options available for users in the Stable Cascade demo page?
-The advanced options include negative prompts, seed numbers for image generation, width and height settings, prior guidance scale, prior inference steps, and decoder guidance scale.
How does Stable Cascade handle multiple elements in a text prompt for image generation?
-Stable Cascade handles multiple elements of a text prompt effectively, generating images that incorporate all the principles of the prompt, unlike some previous models that struggled with multiple element handling.
What is the current intended purpose of the Stable Cascade AI model?
-As of the time of the transcript, Stable Cascade is intended for research purposes and not yet for commercial use.
What is the significance of the demo page for Stable Cascade on Hugging Face?
-The demo page on Hugging Face allows users to test the Stable Cascade model, explore its capabilities, and see the results of image generation based on various text prompts.
Outlines
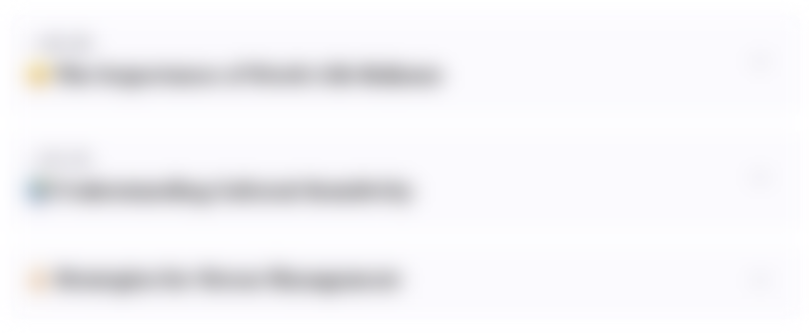
Cette section est réservée aux utilisateurs payants. Améliorez votre compte pour accéder à cette section.
Améliorer maintenantMindmap
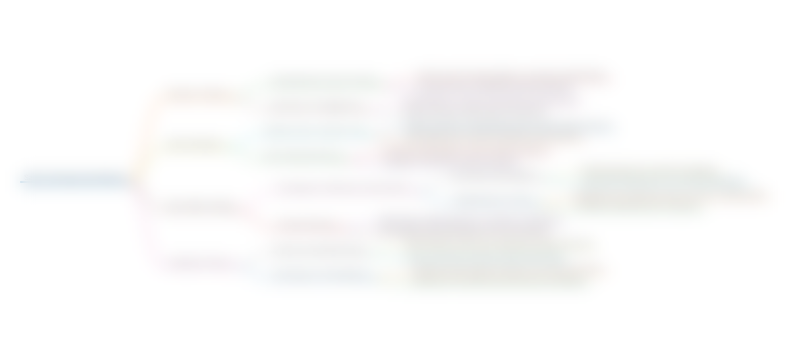
Cette section est réservée aux utilisateurs payants. Améliorez votre compte pour accéder à cette section.
Améliorer maintenantKeywords
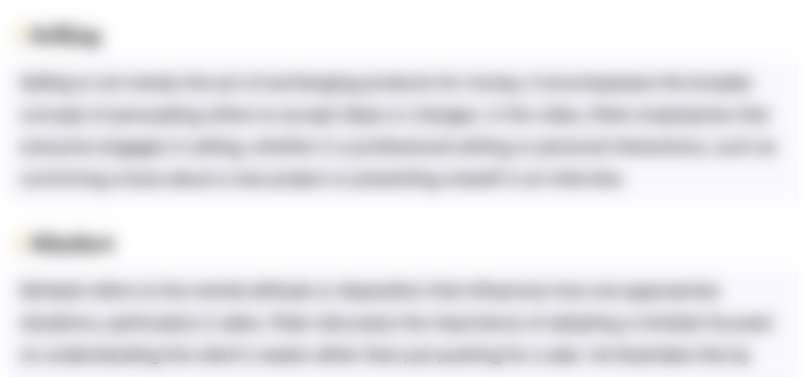
Cette section est réservée aux utilisateurs payants. Améliorez votre compte pour accéder à cette section.
Améliorer maintenantHighlights
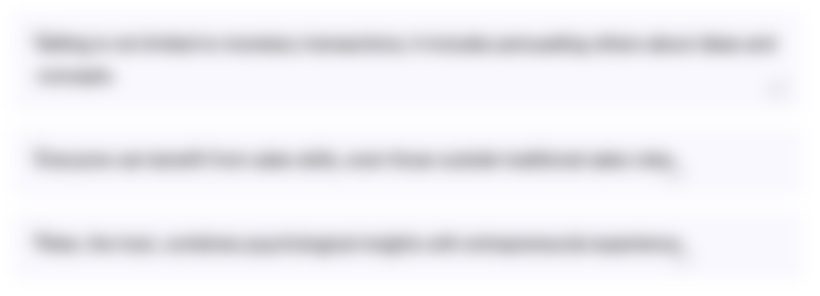
Cette section est réservée aux utilisateurs payants. Améliorez votre compte pour accéder à cette section.
Améliorer maintenantTranscripts
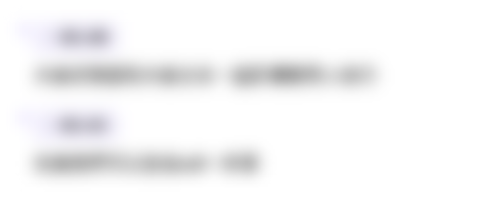
Cette section est réservée aux utilisateurs payants. Améliorez votre compte pour accéder à cette section.
Améliorer maintenantVoir Plus de Vidéos Connexes
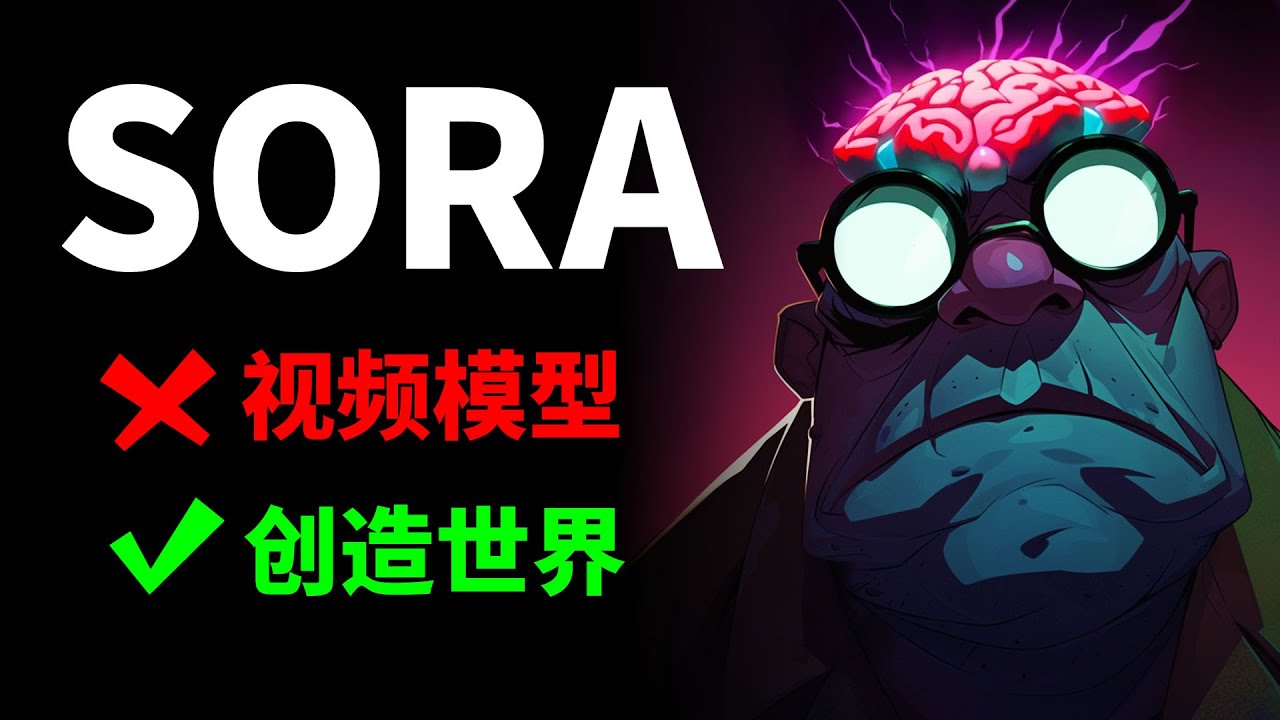
Sora来了!AI生成视频的里程碑时刻!OpenAI发布最强视频生成模型SORA,终极目标是世界模型!Sora模型原理详解、案例应用解读以及影响 | SORA是什么 | SORA怎么用
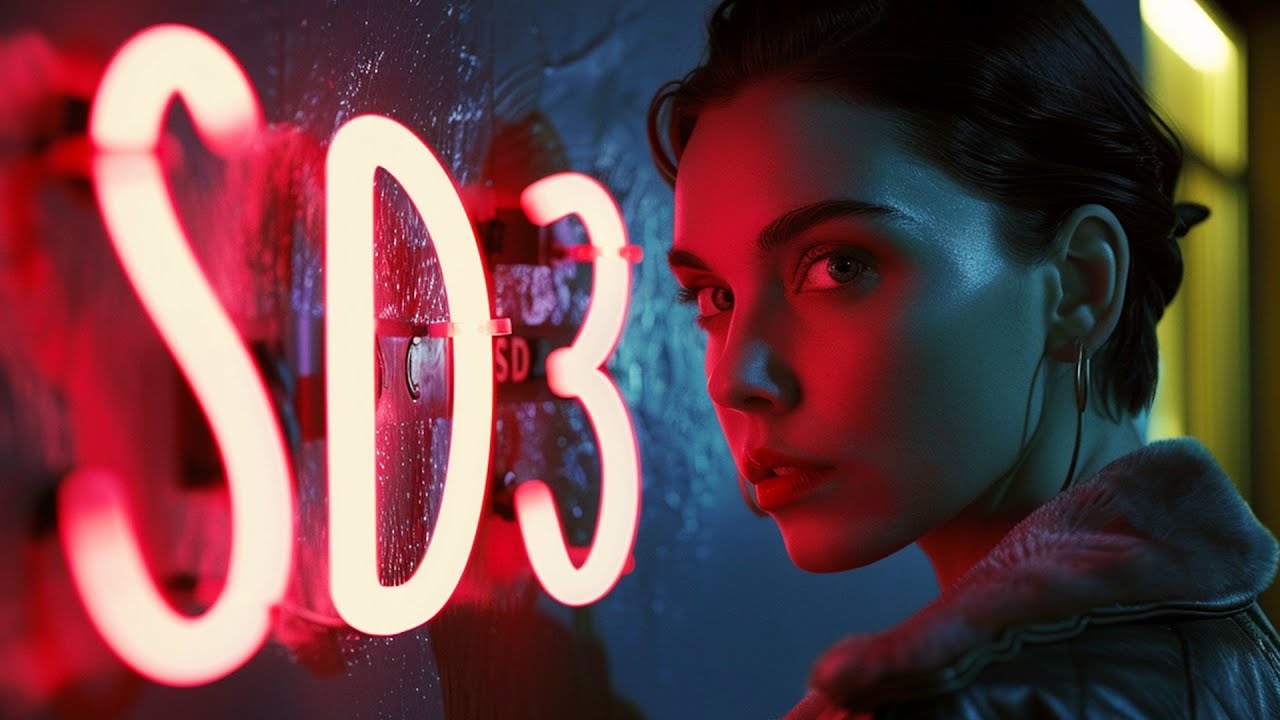
Stable Diffusion 3 Announced! How can you get it?
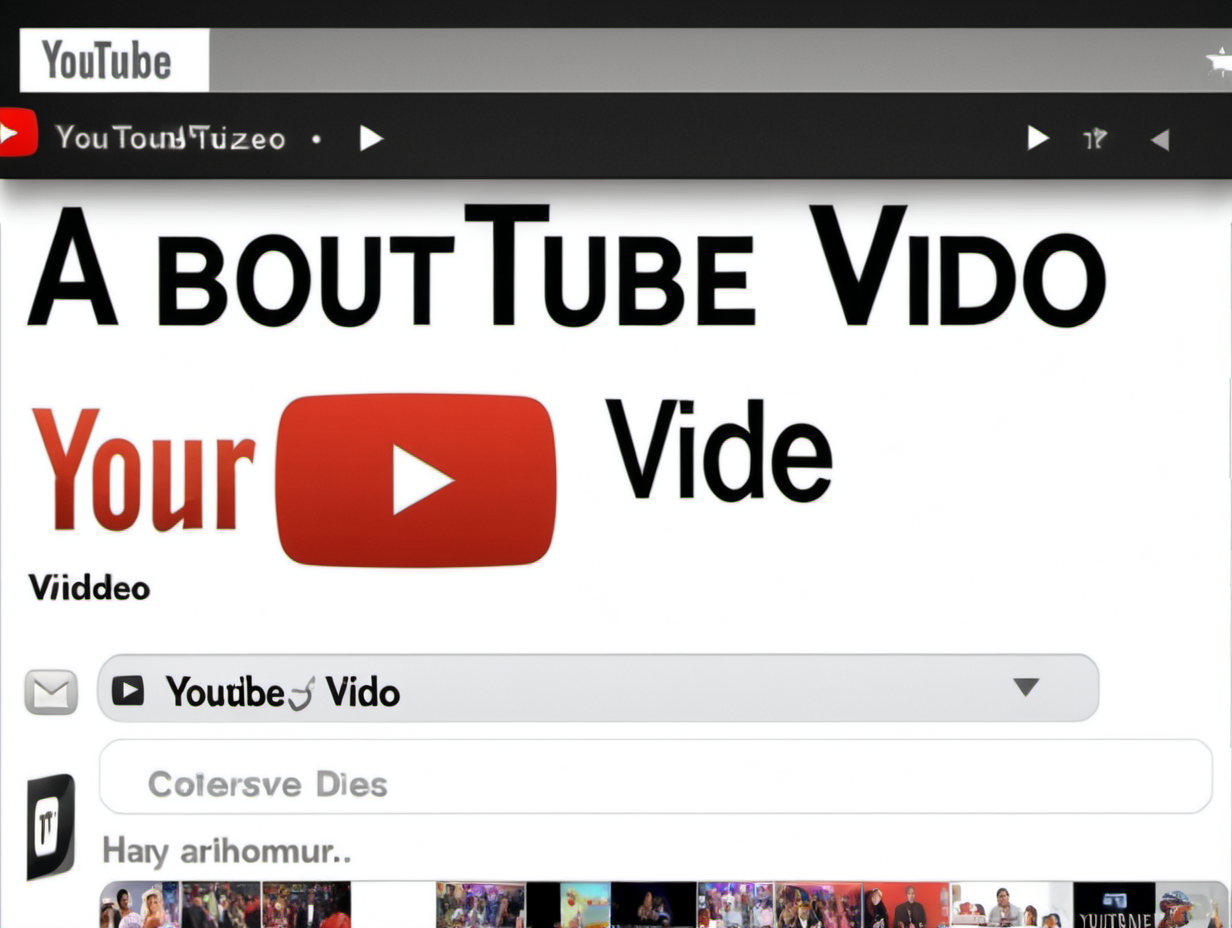
HOW much 💵💰💵 did Stable Diffusion COST to Train?
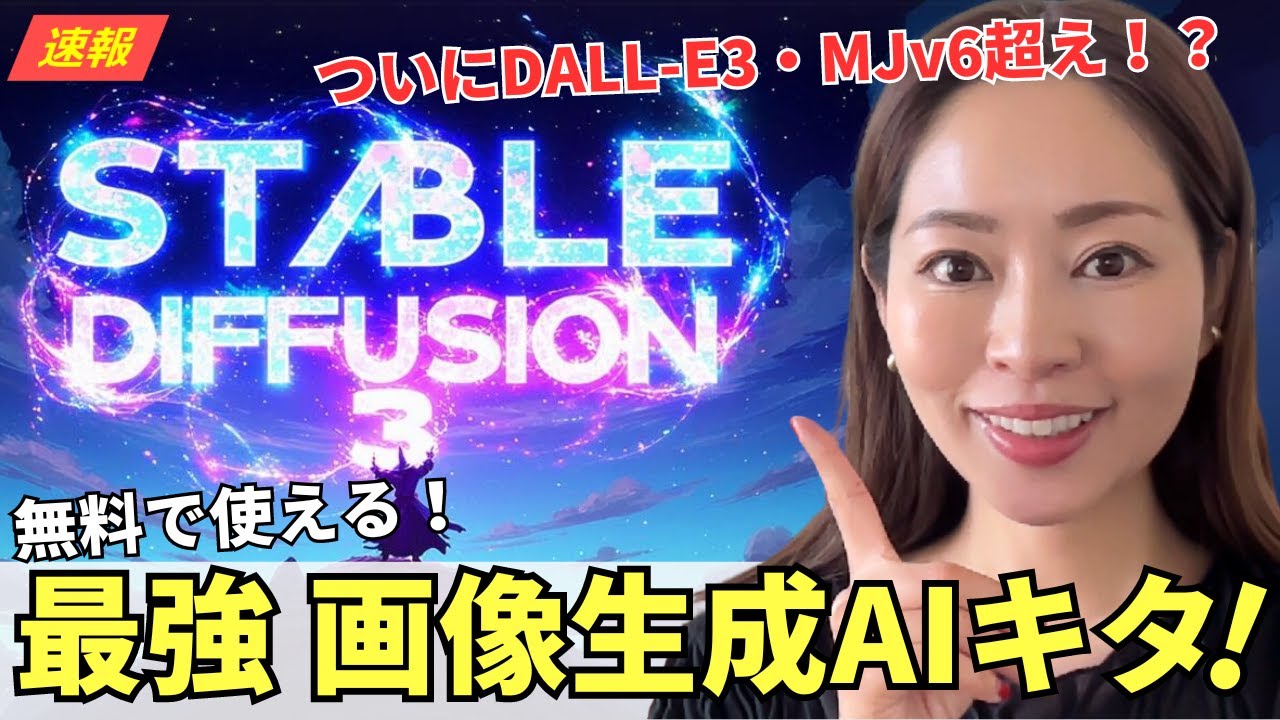
【速報】無料・最強画像生成AI「Stable Diffusion3.0」を徹底レビューしていきます!DALL-E3・Midjourney V6越えは確定!?
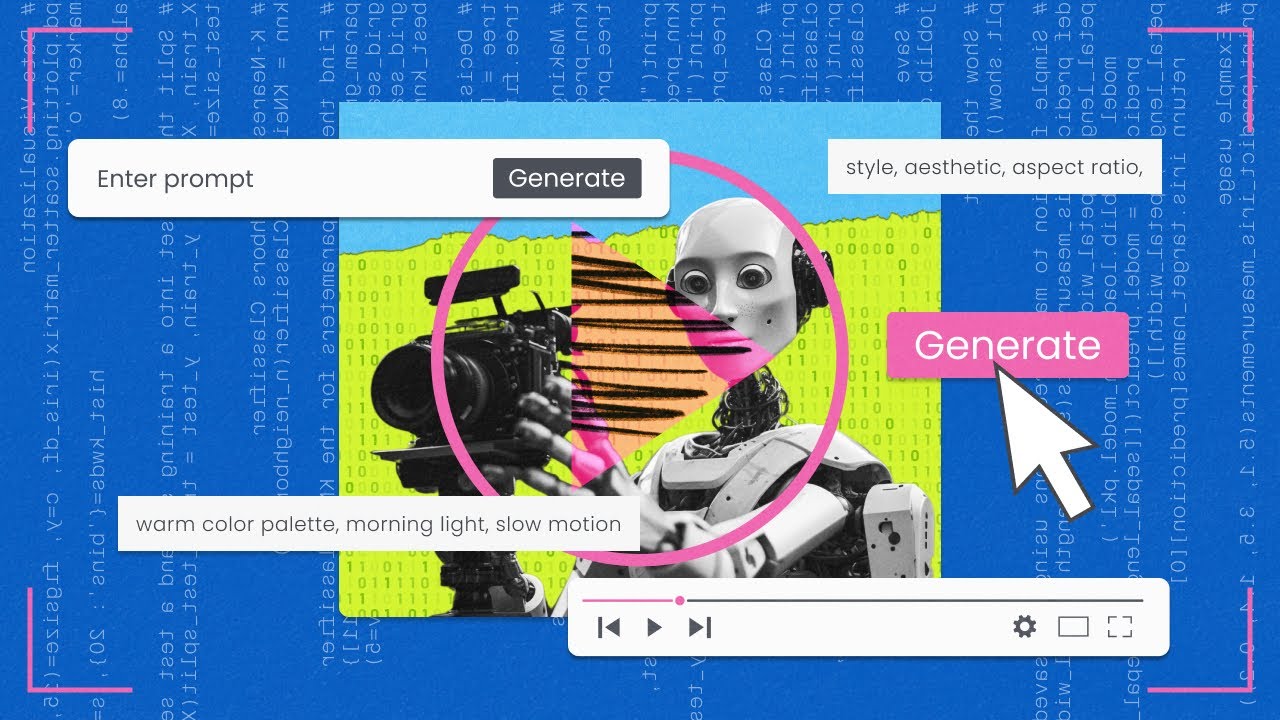
Text to Video: The Next Leap in AI Generation

Create Unlimited Logos with AI (For FREE)
5.0 / 5 (0 votes)