Foundation Models Explained | Generative AI
Summary
TLDRFoundation models (FMs) are a new class of AI models, trained on vast, unstructured datasets to understand patterns and relationships across domains. They revolutionize AI by reducing reliance on curated data and making AI more accessible. Unlike specialized models, FMs can be fine-tuned for various tasks, including language processing and image generation. However, their massive scale comes with challenges, such as biases, computational costs, and ethical concerns. Despite this, the flexibility of FMs has led to their widespread use across industries, offering promising advancements and even more specialized models for future AI applications.
Takeaways
- 😀 Foundation Models (FMs) are AI models trained on extensive data, enabling them to adapt to various downstream tasks, but are not designed for AGI (Artificial General Intelligence).
- 😀 Unlike traditional AI, which requires specific data sets for each task, FMs can handle multiple tasks with minimal additional data, lowering the barrier to entry for AI applications.
- 😀 FMs are trained on large, unlabeled data sets (text, images, code, etc.), which helps them capture general knowledge, enabling fine-tuning for specific tasks without starting from scratch.
- 😀 While FMs reduce the need for large-scale data labeling, biases present in training data remain, requiring ongoing fairness considerations during fine-tuning.
- 😀 Foundation models, such as large language models (LLMs), excel at tasks involving text, including writing, translation, and summarizing, but specialized models may be more effective for niche tasks.
- 😀 FMs are highly versatile, similar to a Swiss Army knife, but specialized models like LLMs may offer more precision for tasks requiring deep understanding of language.
- 😀 Specialization in AI is valuable—smaller, domain-specific models often outperform large general-purpose models, such as in biomedical research, where specialized models can be more efficient.
- 😀 The growing availability of open-source FMs encourages collaboration, enabling smaller companies and individuals to leverage powerful AI tools without needing vast resources.
- 😀 Despite their strengths, FMs require careful consideration of ethical risks, including potential biases, misinformation, and their high computational cost, making them inaccessible to many smaller entities.
- 😀 Future directions in AI focus on intelligent model selection, where the right tool (specialized or general) is chosen based on the task, rather than relying on the largest model available.
- 😀 As FMs evolve, combining multiple data types (e.g., text, images, code) allows for new capabilities like generating images from text descriptions, but challenges such as bias and computational cost remain.
Q & A
What are foundation models (FMs) and why are they called so?
-Foundation models (FMs) are machine learning models trained on vast amounts of data, which can adapt to a wide range of tasks. They are called 'foundation' because they serve as the base for building AI applications across various domains.
What is the relationship between foundation models and artificial general intelligence (AGI)?
-Foundation models are not a pathway to AGI. While they are powerful, they focus on specific tasks and are not designed to think or learn like humans, which is the goal of AGI.
How do foundation models differ from traditional AI models?
-Traditional AI models require massive, carefully labeled, and structured data for each task, whereas foundation models are trained on unlabelled, large datasets and can be fine-tuned for specific tasks with smaller datasets.
What are the advantages of foundation models in terms of data usage?
-Foundation models reduce the need for extensive data labeling, as they are trained on massive unlabelled datasets. Fine-tuning these models for specific tasks requires less data and expertise, making AI more accessible.
What are the key challenges in using foundation models?
-Foundation models can inherit biases from their massive training data, and fine-tuning them still requires machine learning expertise. They are not always the best solution for every task, and specialized models may be more suitable for certain use cases.
How do foundation models enable more accessible AI development?
-By using pre-trained models and fine-tuning them for specific tasks, foundation models lower the barrier to entry for AI development, allowing developers with less machine learning expertise to create AI applications.
What is the potential downside of large-scale foundation models?
-Large foundation models can be computationally expensive to train, and their size and complexity might lead to issues such as bias, misinformation, and increased centralization of power in big tech companies.
How can smaller, specialized models outperform large, general-purpose models?
-Smaller models, trained on domain-specific data, can outperform larger general-purpose models by being more efficient and cost-effective for specific tasks, such as those in fields like medicine or finance.
What role do open-source foundation models play in the AI landscape?
-Open-source foundation models encourage collaboration and innovation by allowing smaller companies and individuals to leverage the power of large-scale AI without needing to train models from scratch.
How does the growing size of foundation models impact the AI field?
-As foundation models grow larger, their capabilities increase, but this also raises concerns about higher computational costs and the ethical implications of their use, particularly in areas such as bias, misinformation, and centralization of power.
Outlines
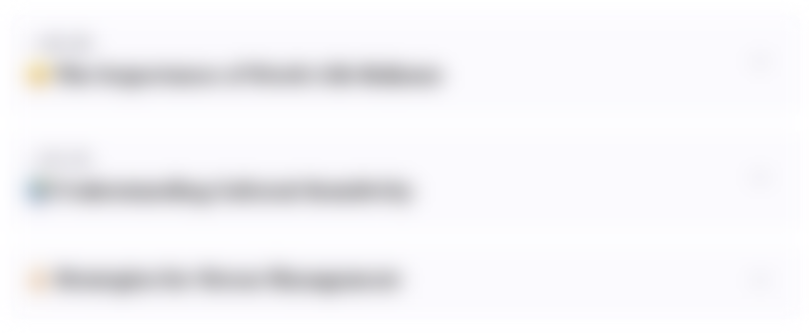
Cette section est réservée aux utilisateurs payants. Améliorez votre compte pour accéder à cette section.
Améliorer maintenantMindmap
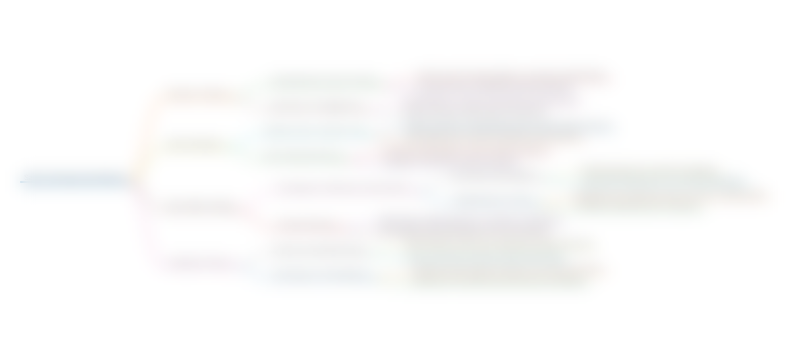
Cette section est réservée aux utilisateurs payants. Améliorez votre compte pour accéder à cette section.
Améliorer maintenantKeywords
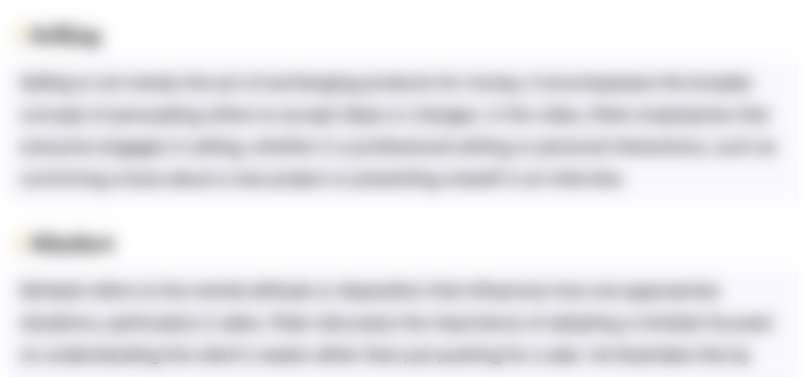
Cette section est réservée aux utilisateurs payants. Améliorez votre compte pour accéder à cette section.
Améliorer maintenantHighlights
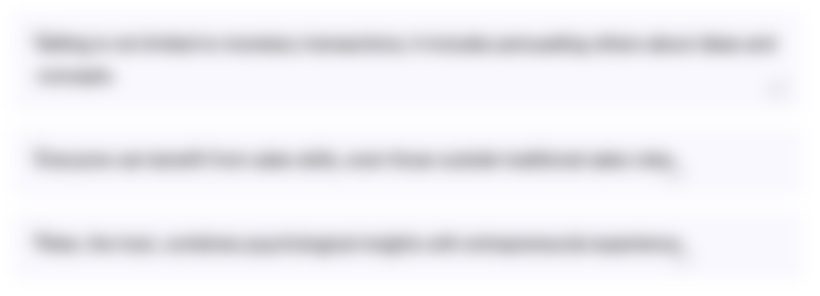
Cette section est réservée aux utilisateurs payants. Améliorez votre compte pour accéder à cette section.
Améliorer maintenantTranscripts
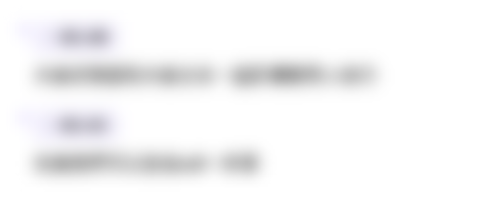
Cette section est réservée aux utilisateurs payants. Améliorez votre compte pour accéder à cette section.
Améliorer maintenant5.0 / 5 (0 votes)