Which Degree Should I get to be a Quant or Data Scientist?
Summary
TLDRThis video script offers a detailed insight into the importance of a strong foundation in mathematics, statistics, and computer science for fields like data science, financial engineering, and quantitative finance. The speaker emphasizes that while business knowledge is useful, it is the technical rigor in these disciplines that drives success. The video encourages viewers to pursue rigorous academic programs focusing on math and technical skills, explaining how these areas directly impact career paths. It also advises against relying on general or overly broad programs, urging prospective students to focus on technical excellence in their studies.
Takeaways
- 😀 Rigor is essential: A solid foundation in math, statistics, and computer science is crucial for success in fields like data science, financial engineering, and quantitative finance.
- 😀 Business knowledge is helpful but secondary: While understanding business is important, the focus should be on technical expertise in math, stats, and computer science.
- 😀 Specialization matters: The more specialized your knowledge in math, stats, or computer science, the better your chances of success in your chosen field.
- 😀 Data science is flexible: It spans across industries such as tech, finance, and manufacturing, but requires deep knowledge in both statistics and computer science.
- 😀 Avoid general programs: Degrees that are too general, mixing business with broad subjects, won’t provide the depth needed for roles in data science or financial engineering.
- 😀 Practical experience counts: It’s important to apply theoretical knowledge in real-world scenarios to fully understand and hone your skills.
- 😀 Know your strength: Whether it’s statistics, math, or computer science, identifying your strongest skill set will help you choose the right career path.
- 😀 Coursework should be rigorous: Programs with minimal courses in core subjects like stats or computer science are inadequate for entering highly technical fields.
- 😀 Career paths depend on your expertise: Your success in data science or financial engineering will depend on which area—stats, math, or computer science—you excel in.
- 😀 Program selection is critical: It's essential to do in-depth research when choosing a program, ensuring it offers a strong curriculum focused on rigorous technical training.
Q & A
Why is it important to have a strong foundation in math, stats, and computer science for careers in data science or financial engineering?
-A strong foundation in math, statistics, and computer science is crucial for these fields because they involve complex problem-solving, data analysis, and algorithm development. These skills are essential for effectively applying quantitative methods to real-world problems in data science, finance, and risk management.
What is the primary difference between business and technical skills in fields like financial engineering or data science?
-The primary difference is that business skills, while important, can be taught and trained relatively quickly on the job. In contrast, technical skills in math, statistics, and computer science require rigorous academic training and form the core of expertise in these fields.
Why is rigor in academic programs emphasized for fields like data science and financial engineering?
-Rigor in academic programs is emphasized because without a solid, deep understanding of mathematical, statistical, and computational concepts, professionals may struggle with applying these techniques effectively in their careers. A rigorous program ensures students gain the necessary depth and expertise.
How do personal interests and strengths play a role in determining which career path to take in data science?
-Personal interests and strengths play a critical role because data science encompasses both computer science and statistics. Those more inclined toward computer science may focus on roles like data engineering, while those with a stronger statistical background may lean toward data science or machine learning.
What is the significance of understanding both the technical and business sides of data science?
-Understanding both sides allows professionals to apply their technical skills effectively while aligning their work with business goals. While business knowledge helps contextualize problems, technical knowledge enables solutions through data-driven methods.
How do various backgrounds, such as engineering, physics, or mathematics, impact career outcomes in fields like risk management or derivatives pricing?
-Different backgrounds shape career outcomes because specific technical skills are required for certain roles. For example, those with engineering or physics backgrounds may excel in mathematical modeling for derivative pricing, while those with statistical expertise may thrive in risk management roles.
Why is it not recommended to focus too much on business-focused programs for data science careers?
-Business-focused programs are often too general and lack the depth required in areas like stats, math, and computer science. These fields need specialized skills that are difficult to acquire through a broad business education.
What can someone do if they want to go into financial engineering but don't have a finance degree?
-Someone without a finance degree can still pursue financial engineering by focusing on developing a strong background in math, statistics, and computer science. While business knowledge is useful, it's more important to have a solid technical foundation to succeed in this field.
What role does statistical expertise play in fields like financial engineering and data science?
-Statistical expertise is crucial for understanding and applying methods like predictive modeling, risk analysis, and machine learning. Statisticians are able to extract valuable insights from data, which is fundamental for making informed decisions in finance and data-driven industries.
What advice does the speaker give about choosing the right program for fields like data science or quantitative finance?
-The speaker advises that prospective students focus on programs that are highly rigorous in stats, math, and computer science. While the business aspect is important, it's essential to select a program that provides in-depth technical training to ensure success in these fields.
Outlines
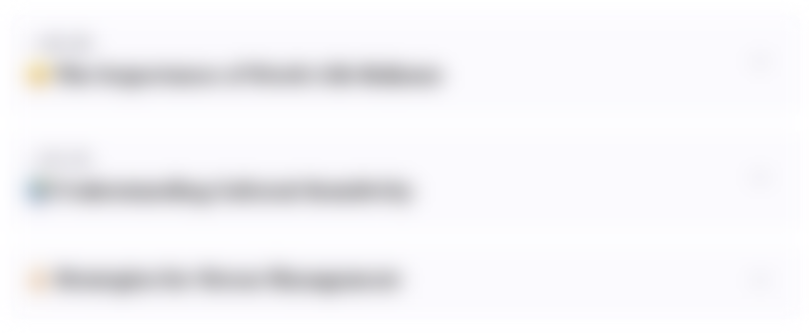
Cette section est réservée aux utilisateurs payants. Améliorez votre compte pour accéder à cette section.
Améliorer maintenantMindmap
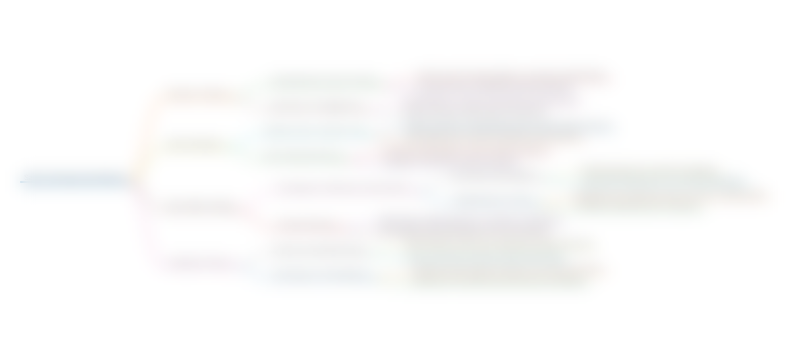
Cette section est réservée aux utilisateurs payants. Améliorez votre compte pour accéder à cette section.
Améliorer maintenantKeywords
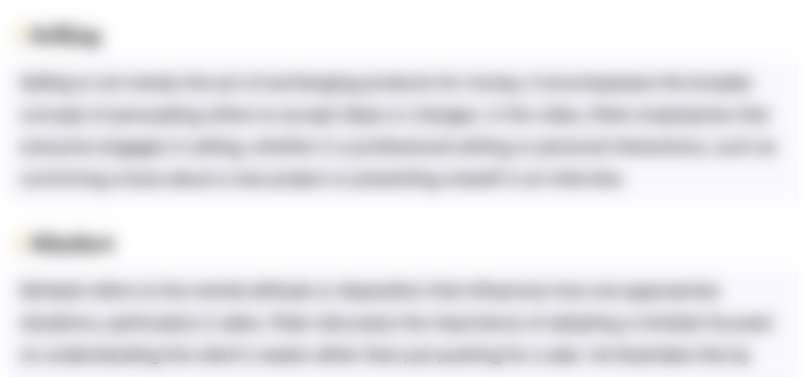
Cette section est réservée aux utilisateurs payants. Améliorez votre compte pour accéder à cette section.
Améliorer maintenantHighlights
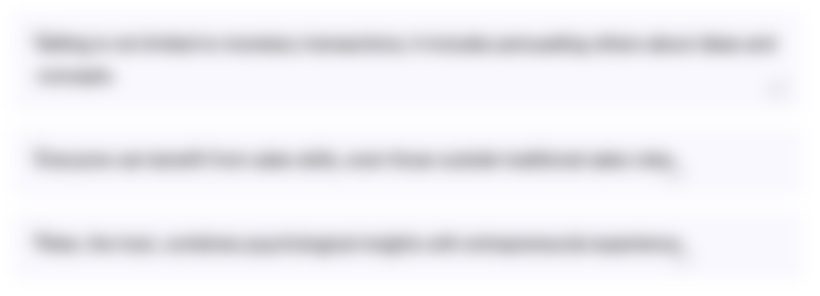
Cette section est réservée aux utilisateurs payants. Améliorez votre compte pour accéder à cette section.
Améliorer maintenantTranscripts
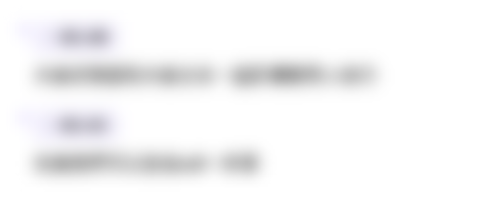
Cette section est réservée aux utilisateurs payants. Améliorez votre compte pour accéder à cette section.
Améliorer maintenantVoir Plus de Vidéos Connexes
5.0 / 5 (0 votes)