What’s changed in AlphaFold 3?
Summary
TLDRThe new AlphaFold3 algorithm surpasses AlphaFold2 in power and simplicity. It not only predicts single protein folding but also handles complexes of proteins, DNA, RNA, and potential drug candidates called ligands. The model simplifies protein structures by focusing on atomic positions and uses diffusion, a technique from AI art algorithms. AlphaFold3's general framework and multimodal approach enhance its performance. It shifts emphasis from evolutionary MSA data to pair representation, making it adaptable for molecules without evolutionary history. This generalization suggests future models will handle diverse inputs and outputs, advancing beyond constrained problems like structure prediction.
Takeaways
- 💪 AlphaFold3 is much more powerful than AlphaFold2 as it handles combinations of proteins, DNA, RNA, and ligands.
- 🧬 AlphaFold3's ability to analyze ligands is significant because they are potential drug candidates.
- 🎯 AlphaFold3 is simpler than AlphaFold2 due to a general approach that uses diffusion to determine the positions of atoms.
- 🔄 By generalizing beyond proteins, AlphaFold3 can use the same method to figure out the structure of any molecule.
- 🌍 Multi-modal algorithms, like AlphaFold3, which handle different types of data, are more powerful and versatile.
- 📊 AlphaFold3 embeds all types of molecules into the same space, making it more general and efficient.
- 🔄 The pair representation now encodes the majority of information, de-emphasizing the role of MSA in AlphaFold3.
- 🧩 The MSA module in AlphaFold3 mainly focuses on squeezing information into the pair representation.
- 📉 Evolutionary data is less emphasized in AlphaFold3, favoring direct learning of molecular interactions.
- 🔮 Future models like AlphaFold3 are expected to become more general, handling more input types and providing diverse outputs.
Q & A
What is the key improvement of AlphaFold3 over AlphaFold2?
-AlphaFold3 not only handles single protein folding but can also analyze complexes of different proteins, DNA, RNA, and ligands, making it a more powerful and versatile model.
Why are ligands significant in the context of AlphaFold3?
-Ligands are potential drug candidates, and AlphaFold3's ability to model their interactions with proteins can help in drug discovery by identifying how small molecules might bind to proteins to fix misbehaving proteins.
How does AlphaFold3 simplify the modeling process compared to AlphaFold2?
-AlphaFold3 simplifies the process by focusing on the positions of every atom rather than dealing with protein-specific structures like triangles, angles, and rotations. This generalization allows it to handle various types of molecules uniformly.
What role does diffusion play in AlphaFold3?
-Diffusion is used to add noise to the known positions of atoms during training, enabling the algorithm to learn to predict the correct positions of atoms in any molecule. This method is powerful and simplifies the modeling process.
What is meant by multimodal algorithms, and why are they important?
-Multimodal algorithms can handle different types of data, such as images and text, and are more powerful because they can transfer knowledge between these modalities. For example, a model trained on both images and text can perform better on text tasks than a model trained only on text.
How does AlphaFold3's approach to the MSA differ from AlphaFold2?
-In AlphaFold3, the MSA (multiple sequence alignment) is less emphasized. The information from the MSA is pushed into the pair representation, which now encodes the majority of the information and is used more extensively in the algorithm.
What is the pair representation in the context of AlphaFold algorithms?
-The pair representation is a matrix that encodes information about pairs of amino acids in a protein, such as their distances and angles relative to each other. It plays a crucial role in determining the protein's structure.
Why is the pair representation more important in AlphaFold3?
-AlphaFold3 relies more on the pair representation because it generalizes better across different types of molecules, including those without evolutionary data like ligands. This shift makes the model more versatile and less dependent on evolutionary history.
What is the potential future direction for models like AlphaFold3?
-Future models are expected to become more general, capable of taking more types of inputs and predicting various properties of molecules, not just their structure. This would make them more similar to large language models (LLMs) with open-ended capabilities.
Why might it be beneficial for models to de-emphasize evolutionary data in the future?
-De-emphasizing evolutionary data might be beneficial because it forces the model to learn the underlying chemistry and physics of molecular interactions, which could lead to better predictions for molecules without evolutionary histories, such as synthetic drugs.
Outlines
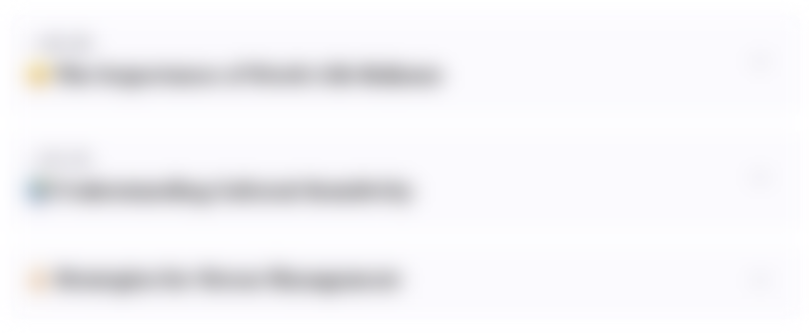
Cette section est réservée aux utilisateurs payants. Améliorez votre compte pour accéder à cette section.
Améliorer maintenantMindmap
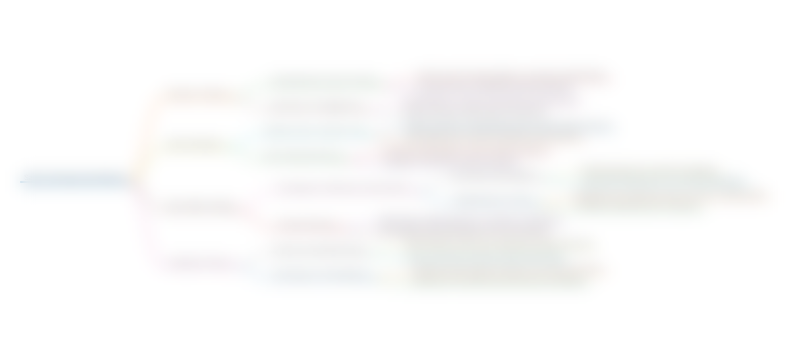
Cette section est réservée aux utilisateurs payants. Améliorez votre compte pour accéder à cette section.
Améliorer maintenantKeywords
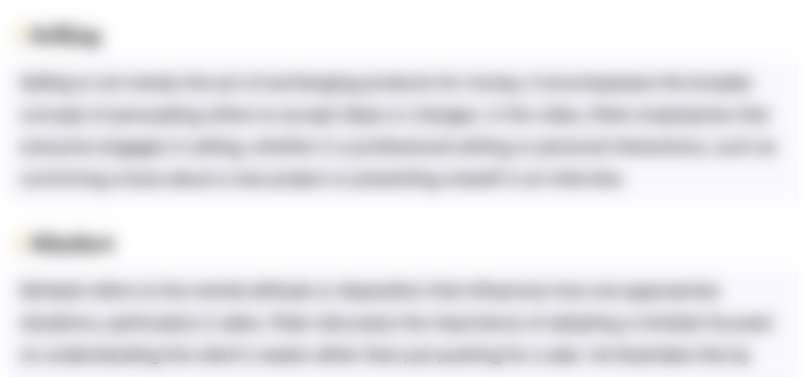
Cette section est réservée aux utilisateurs payants. Améliorez votre compte pour accéder à cette section.
Améliorer maintenantHighlights
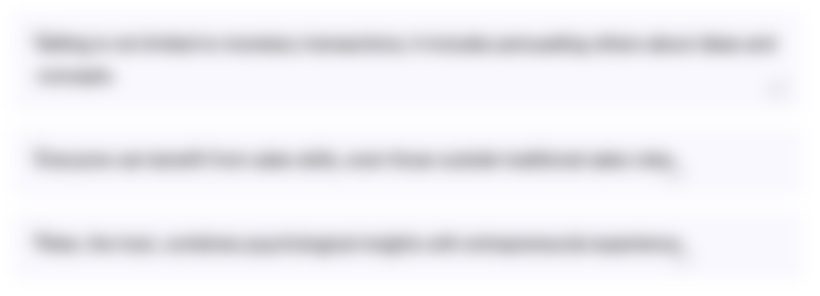
Cette section est réservée aux utilisateurs payants. Améliorez votre compte pour accéder à cette section.
Améliorer maintenantTranscripts
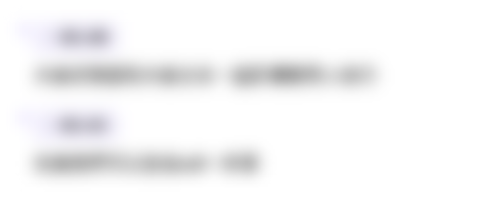
Cette section est réservée aux utilisateurs payants. Améliorez votre compte pour accéder à cette section.
Améliorer maintenantVoir Plus de Vidéos Connexes
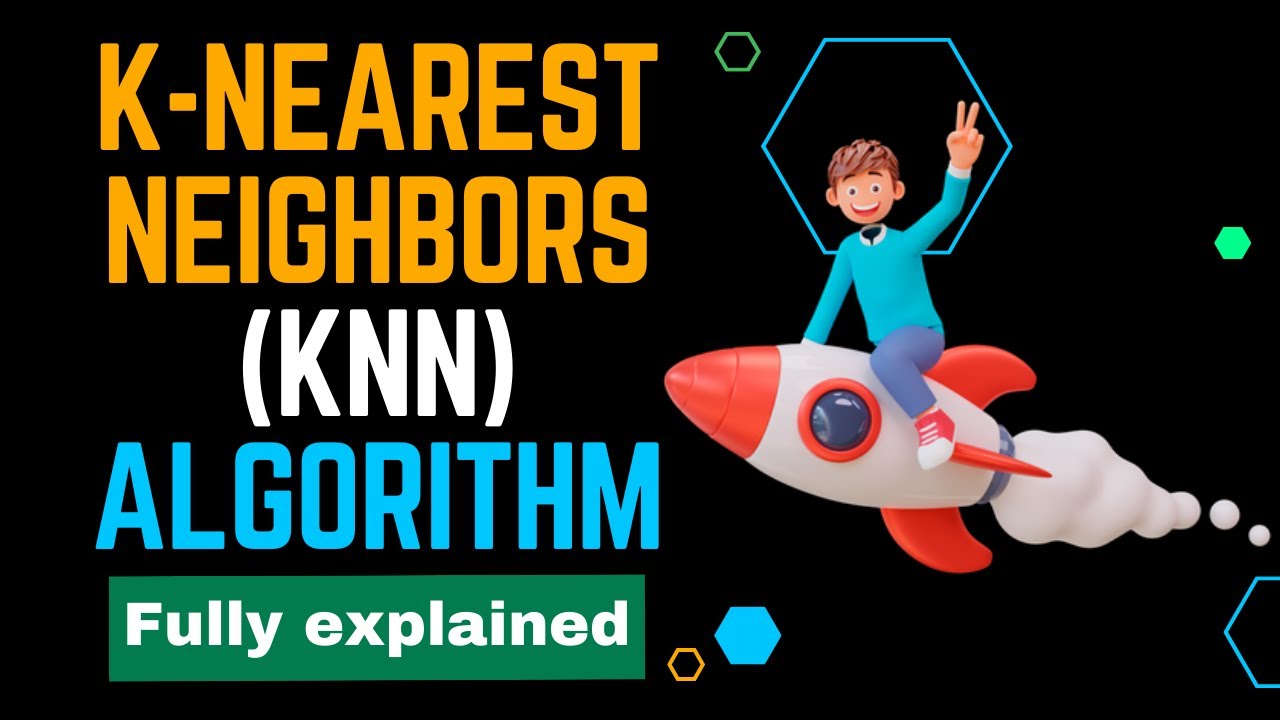
Lecture 3.4 | KNN Algorithm In Machine Learning | K Nearest Neighbor | Classification | #mlt #knn

CSC645 - Chapter 1 (Continued) - Fundamentals of Algorithm Analysis
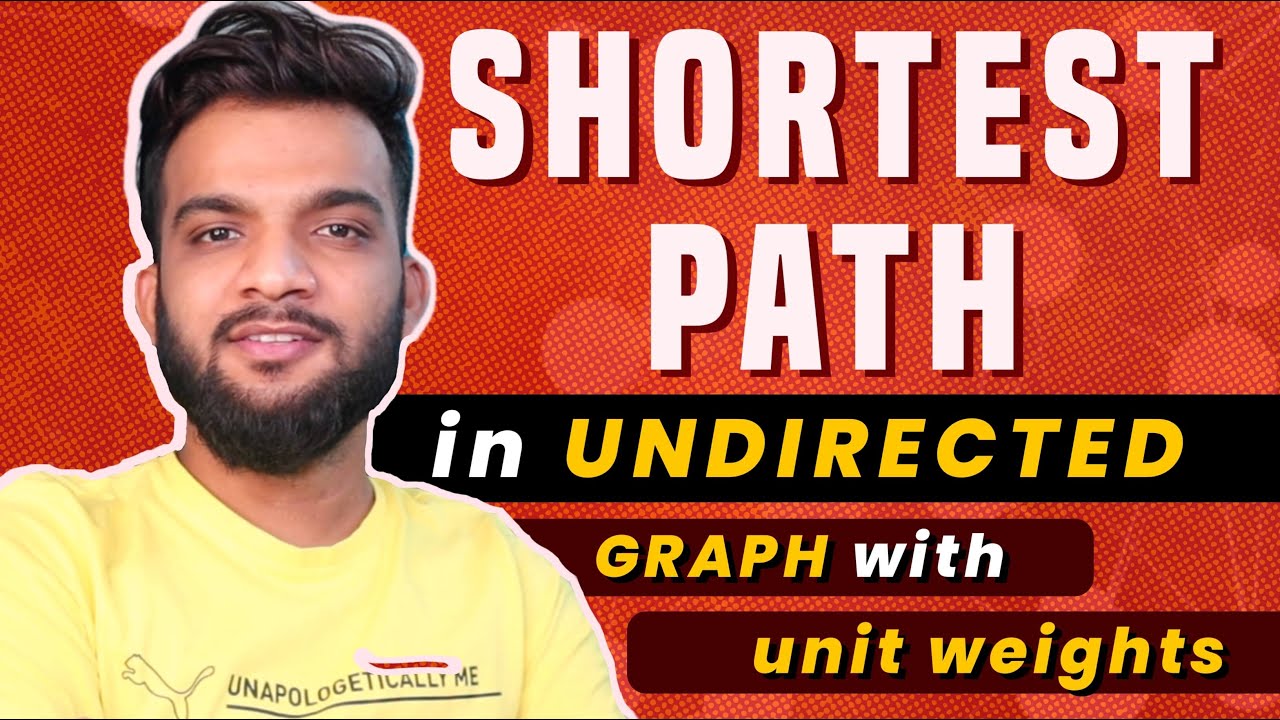
G-28. Shortest Path in Undirected Graph with Unit Weights

Algoritma Greedy - Informatika Kelas XI
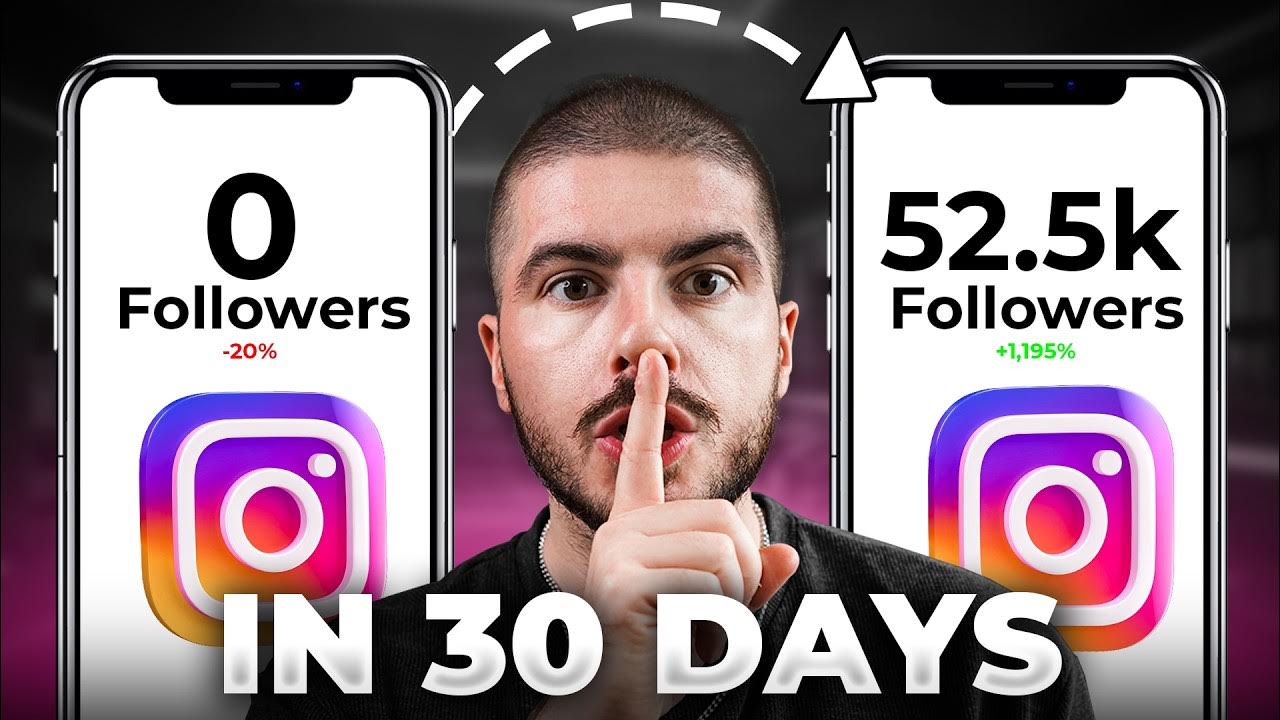
How I Gained 50,000 Followers In 1 Month (9 Easy Steps)
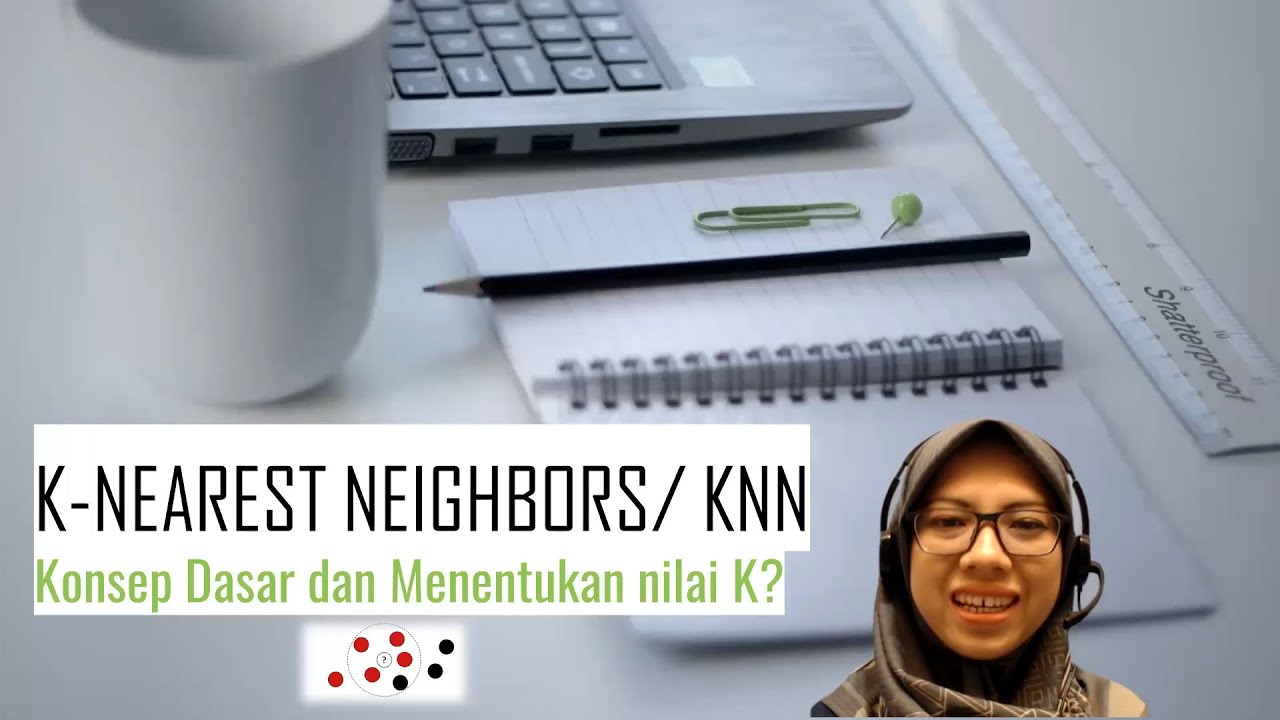
Konsep Algoritma KNN (K-Nearest Neigbors) dan Tips Menentukan Nilai K
5.0 / 5 (0 votes)