Praktikum Ekonometrika II - Analisis ARIMA di EViews
Summary
TLDRThis video tutorial demonstrates how to use EViews software for econometric analysis, specifically focusing on the Consumer Price Index (CPI) of Indonesia from January 2014 to June 2019. It covers steps such as data importation from Excel, performing stationarity tests, selecting ARFIMA model orders, and conducting automatic forecasting. The tutorial also explores seasonal patterns in the data and offers guidance on generating accurate forecasts, including the creation of plots and saving outputs. It's a comprehensive guide for those interested in econometric modeling and time series forecasting.
Takeaways
- đ The video demonstrates the use of EViews software for econometric analysis, focusing on time series data.
- đ The example dataset used is the Consumer Price Index (CPI) for Indonesia, from January 2014 to June 2019 (monthly data).
- đ Data from Excel is imported into EViews using a quick copy-paste method for easy analysis.
- đ The first analysis step is checking the stationarity of the CPI data using the Augmented Dickey-Fuller test.
- đ If the data is not stationary, differencing is applied to achieve stationarity (e.g., first differencing).
- đ The correlogram is used to visually inspect autocorrelation patterns and determine potential orders for ARFIMA models.
- đ Seasonal patterns in the data are assessed based on monthly frequencies, with emphasis on a 12-month cycle.
- đ EViews includes an automatic model selection feature, which helps determine the best ARFIMA model without manual trial and error.
- đ After selecting the ARFIMA model, diagnostic checks are performed to ensure the model is valid (e.g., checking for randomness in residuals).
- đ The ARFIMA model is then used to forecast future CPI values for six months (July to December 2019).
- đ Forecast results are visualized with plots, including confidence intervals, and saved as separate objects within EViews for further use.
Q & A
What software is used for the econometrics analysis in this video?
-The software used for the econometrics analysis in this video is EViews.
What type of data is being used for the analysis?
-The data used for the analysis is the Consumer Price Index (CPI) of Indonesia, with monthly data from January 2014 to June 2019.
How is the data imported into EViews from Excel?
-The data is copied from Excel and imported into EViews by selecting 'Quick' and then choosing 'Empty Group' before pasting the data.
What is the first step to visualize the data in EViews?
-The first step to visualize the data in EViews is to click on 'Quick' and then 'View', followed by 'Graph' to plot the data.
What does the Augmented Dickey-Fuller test check for in time series data?
-The Augmented Dickey-Fuller (ADF) test checks for stationarity in time series data, specifically whether a unit root is present, which would indicate non-stationarity.
What does it mean when the ADF test p-value is 0.5775?
-A p-value of 0.5775 means that the null hypothesis (the presence of a unit root) cannot be rejected, indicating that the data is non-stationary at the original level.
What adjustment is made to the data after the first ADF test shows non-stationarity?
-After the first ADF test shows non-stationarity, the data is differenced (first difference) to make it stationary, which is confirmed by a p-value of 0.0000.
What does the correlogram analysis reveal about the data?
-The correlogram analysis helps identify patterns in autocorrelation. In this case, it shows a slow decline, indicating that the data may still have some non-stationarity or other structure, like seasonality.
What does the 'automatic archival casting' feature in EViews do?
-The 'automatic archival casting' feature in EViews automatically selects the best ARFIMA (AutoRegressive Fractionally Integrated Moving Average) model based on the data characteristics, eliminating the need for manual trial and error.
How is forecasting performed in EViews for the CPI data?
-Forecasting is performed by creating a new workfile, setting the sample period for the forecast, and then using the 'forecast' function to predict future values, which can be plotted alongside actual data.
Outlines
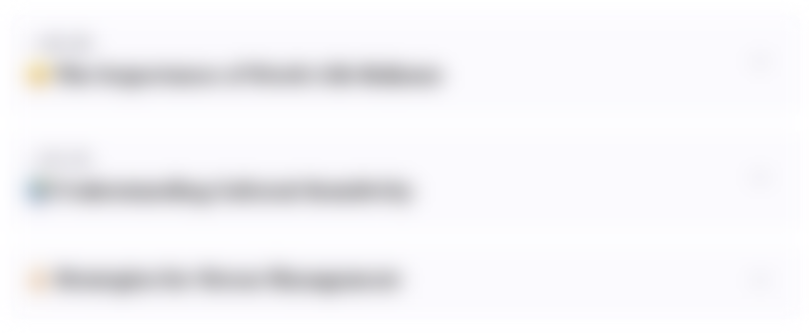
Cette section est réservée aux utilisateurs payants. Améliorez votre compte pour accéder à cette section.
Améliorer maintenantMindmap
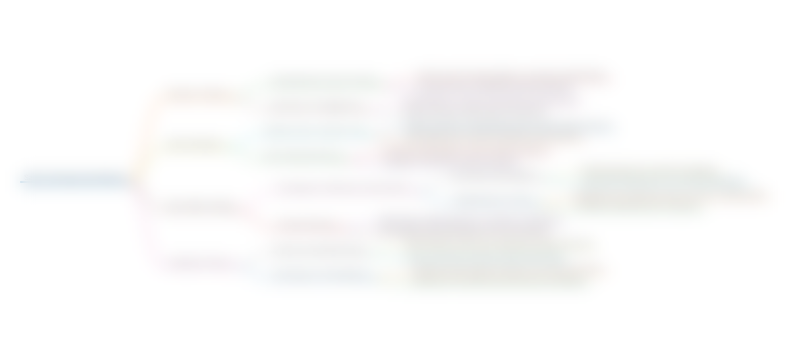
Cette section est réservée aux utilisateurs payants. Améliorez votre compte pour accéder à cette section.
Améliorer maintenantKeywords
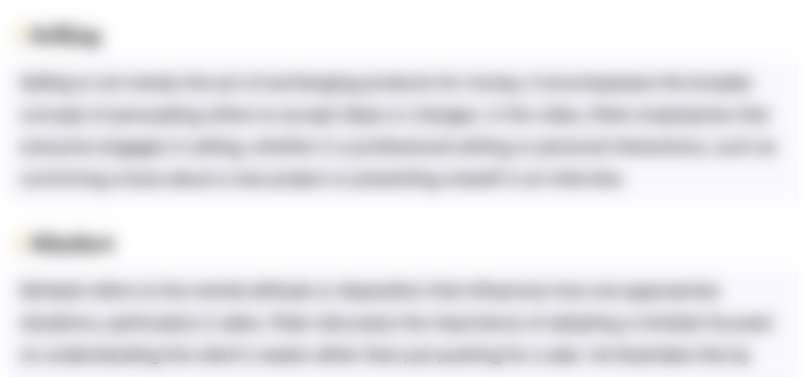
Cette section est réservée aux utilisateurs payants. Améliorez votre compte pour accéder à cette section.
Améliorer maintenantHighlights
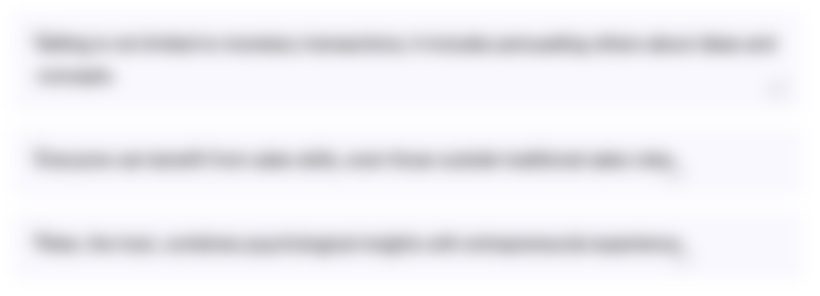
Cette section est réservée aux utilisateurs payants. Améliorez votre compte pour accéder à cette section.
Améliorer maintenantTranscripts
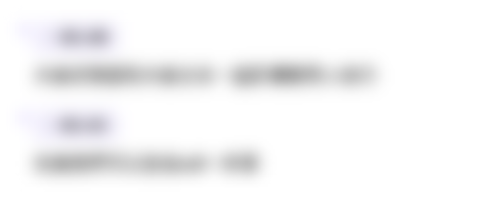
Cette section est réservée aux utilisateurs payants. Améliorez votre compte pour accéder à cette section.
Améliorer maintenantVoir Plus de Vidéos Connexes
5.0 / 5 (0 votes)