27. Normality Testing of the Data in IBM SPSS || Dr. Dhaval Maheta
Summary
TLDRThis video provides a comprehensive overview of normality testing in statistics, focusing on both informal and formal methods. Key topics include the characteristics of a normal distribution, the importance of normality for statistical tests like t-tests and regression, and the methods to assess normality using visual tools such as histograms, box plots, and QQ plots. Additionally, the video explains formal tests like the Shapiro-Wilk and Kolmogorov-Smirnov tests, and how to handle non-normal data through transformations like logarithmic scaling. The lecture also demonstrates the application of these concepts using SPSS software to assess and correct normality in real data.
Takeaways
- đ Normal distribution is a continuous random variable with a bell-shaped curve that extends infinitely in both directions, never touching the horizontal axis.
- đ The characteristics of normal distribution include symmetry, with the mean, median, and mode being equal, and 50% of the data lying on each side of the mean.
- đ The area under the normal curve equals 1, representing the total probability of all possible outcomes for a normally distributed variable.
- đ 68% of data falls within ±1 standard deviation from the mean, 95% within ±2 standard deviations, and 99.7% within ±3 standard deviations.
- đ Data that falls beyond ±3 standard deviations is considered an outlier, which can affect statistical analysis and normality testing.
- đ Parametric tests like t-test, F-test, and regression analysis require data to be normally distributed in order to produce valid results.
- đ Informal methods for assessing normality include histograms, box plots, normal quantile plots, and stem-and-leaf plots, which visually reveal the shape and distribution of the data.
- đ Formal normality tests (like the Shapiro-Wilk and Anderson-Darling tests) are used to statistically assess whether the data follows a normal distribution, with a p-value of <0.05 indicating non-normality.
- đ In SPSS, normality is tested using the 'Explore' function, which generates descriptive statistics, histograms, and normality plots to visually and statistically assess data distribution.
- đ If data is not normally distributed, transformations like logarithmic transformations can be applied to normalize the data, which can then be re-assessed for normality using the same methods.
- đ After transformations, it's essential to recheck normality using both informal (e.g., histograms, box plots) and formal methods (e.g., Shapiro-Wilk test) to determine if the data is now suitable for parametric tests.
Q & A
What are the key characteristics of a normal distribution?
-A normal distribution is a continuous random variable with a bell-shaped curve. It is symmetrical, where the mean, median, and mode are all equal. The distribution extends indefinitely in both directions, approaching but never touching the horizontal axis. Additionally, 68% of the data lies within ±1 standard deviation, 95% within ±2 standard deviations, and 99.7% within ±3 standard deviations.
Why is normality important in statistical analysis?
-Normality is essential because many statistical methods, such as t-tests, F-tests, and regression analysis, assume that the data follows a normal distribution. If the data is not normally distributed, the validity of these parametric tests could be compromised.
What are the common statistical tests that require normality?
-Common tests that require normality include the t-test, ANOVA, and regression analysis. These are parametric tests, which assume that the data is normally distributed in order to produce reliable results.
What are informal methods for testing normality?
-Informal methods for testing normality include visual techniques such as histograms, box plots, stem-and-leaf plots, and normal quantile-quantile (QQ) plots. These methods give an initial idea of whether the data might follow a normal distribution.
What is the role of the histogram in testing normality?
-A histogram provides a visual representation of the data distribution. If the histogram is bell-shaped and symmetrical, it suggests that the data may be normally distributed. However, this method is subjective and may not give definitive results.
How do you interpret the results of a normal quantile-quantile (QQ) plot?
-In a normal QQ plot, if the data is normally distributed, the data points should lie along a straight line. Deviations from the straight line indicate departures from normality. This is a visual diagnostic tool for assessing normality.
What does a box plot reveal about the normality of data?
-A box plot shows the distribution of the data, including the median, quartiles, and potential outliers. In terms of normality, a box plot can reveal skewness or the presence of outliers, which may indicate that the data is not normally distributed.
What is the purpose of the Shapiro-Wilk test for normality?
-The Shapiro-Wilk test is a formal statistical test used to assess whether a sample comes from a normally distributed population. The null hypothesis of the test is that the data is normally distributed. If the p-value is less than 0.05, the null hypothesis is rejected, indicating that the data is not normally distributed.
How do you interpret the p-value in a normality test like Shapiro-Wilk?
-In normality tests like the Shapiro-Wilk test, if the p-value is less than 0.05, the null hypothesis that the data is normally distributed is rejected. A p-value greater than 0.05 indicates that the null hypothesis cannot be rejected, suggesting that the data is approximately normally distributed.
What steps can be taken if the data is not normally distributed?
-If the data is not normally distributed, one option is to transform the data to make it more normal. A common transformation is the logarithmic transformation, which can reduce skewness and help the data approximate a normal distribution. Alternatively, non-parametric tests, which do not assume normality, can be used.
Outlines
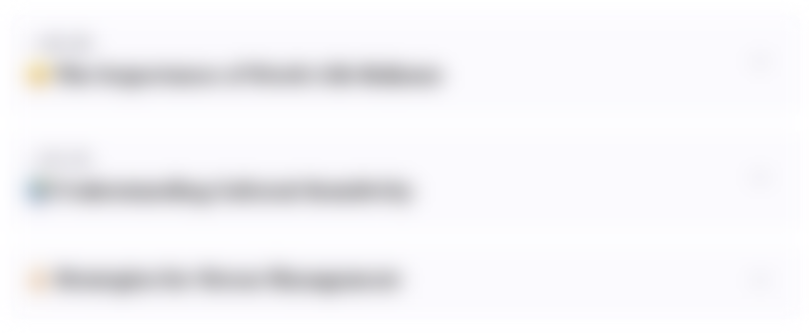
Cette section est réservée aux utilisateurs payants. Améliorez votre compte pour accéder à cette section.
Améliorer maintenantMindmap
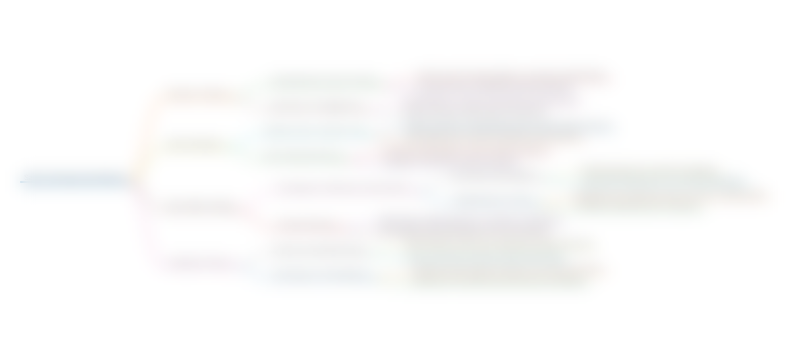
Cette section est réservée aux utilisateurs payants. Améliorez votre compte pour accéder à cette section.
Améliorer maintenantKeywords
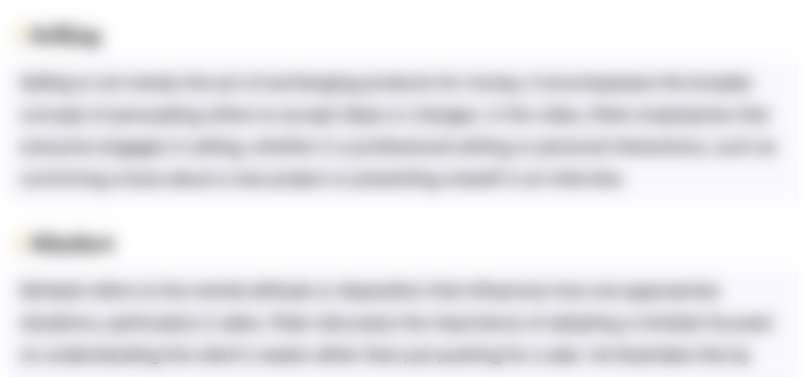
Cette section est réservée aux utilisateurs payants. Améliorez votre compte pour accéder à cette section.
Améliorer maintenantHighlights
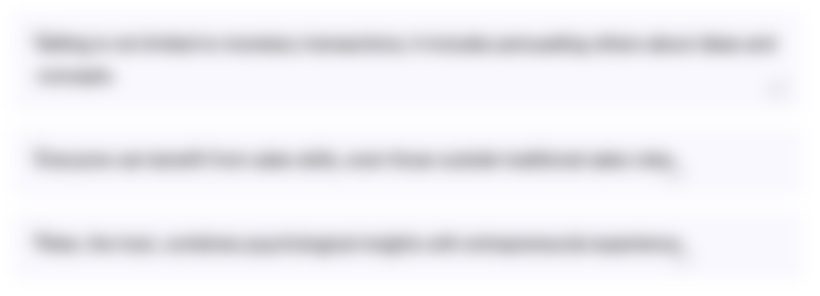
Cette section est réservée aux utilisateurs payants. Améliorez votre compte pour accéder à cette section.
Améliorer maintenantTranscripts
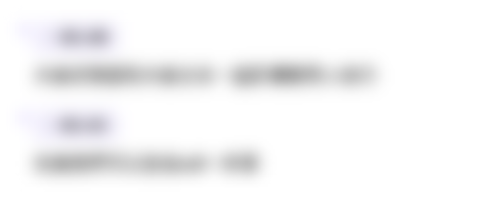
Cette section est réservée aux utilisateurs payants. Améliorez votre compte pour accéder à cette section.
Améliorer maintenantVoir Plus de Vidéos Connexes
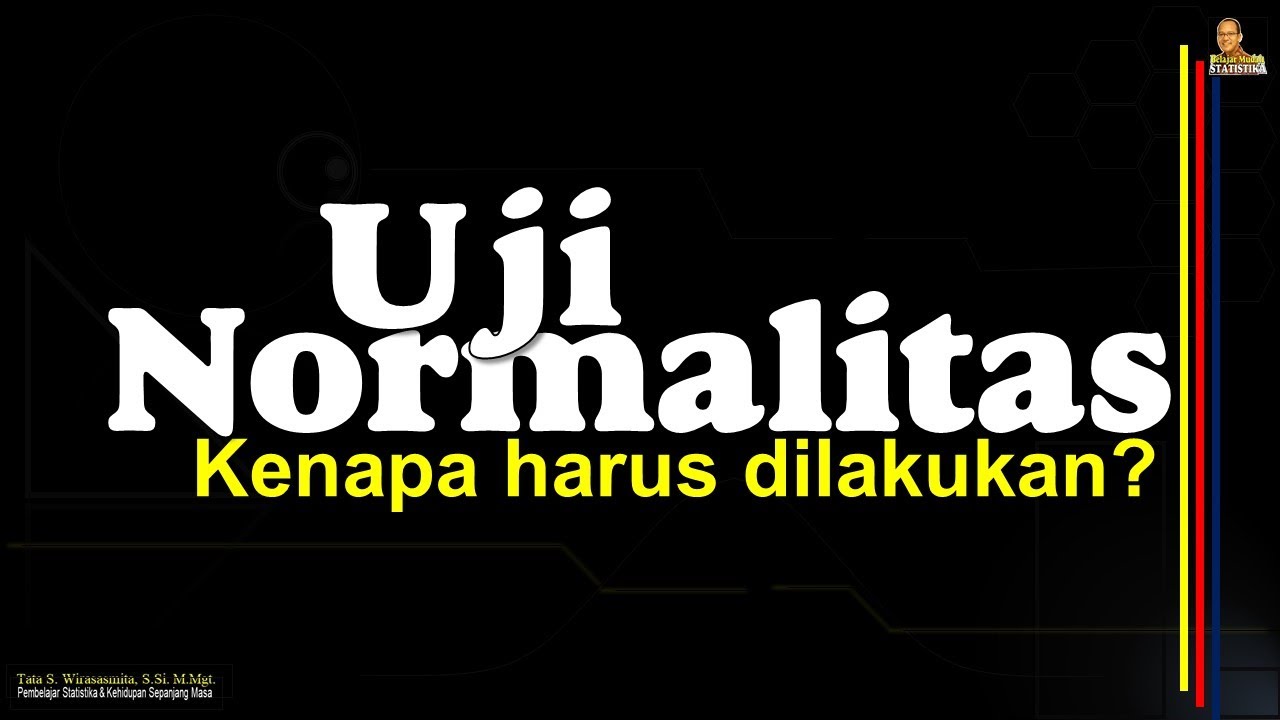
UJI NORMALITAS: Kenapa & Variabel apa yang dapat Diuji Normalitas-nya?
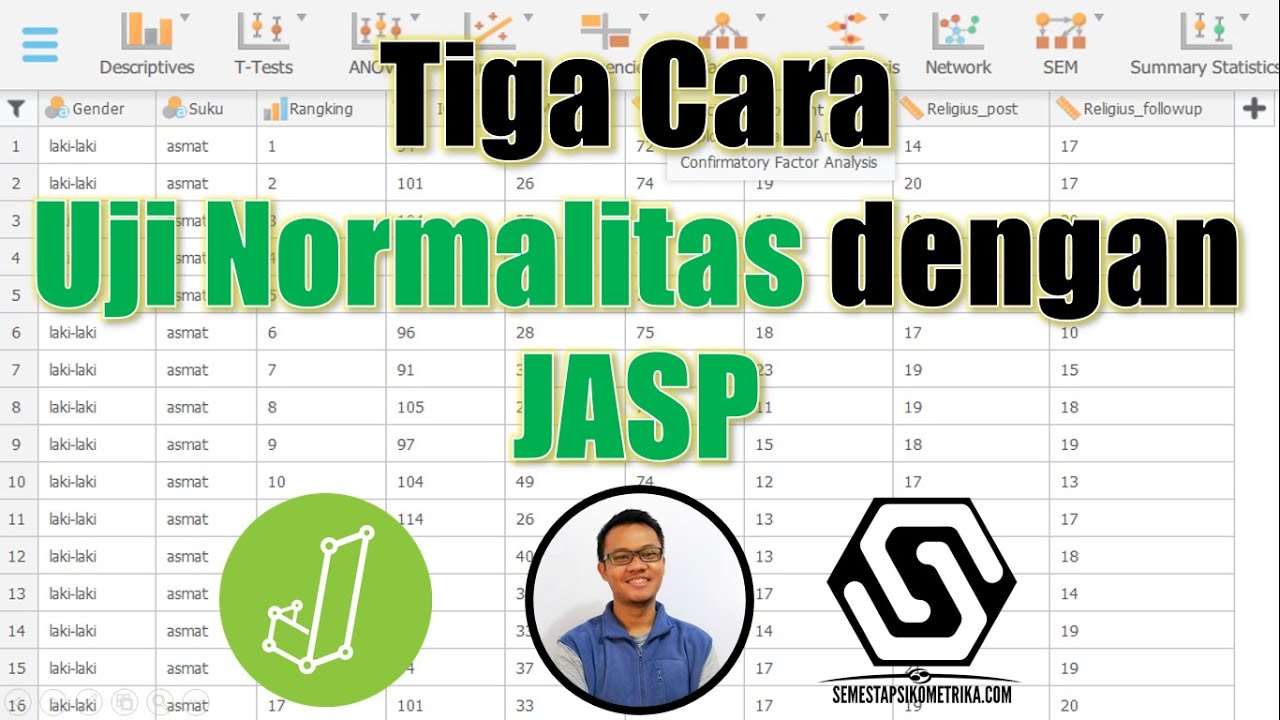
Tutorial Cara Uji Normalitas dengan JASP

Uji Normalitas Lilifors Matkul STATISTIK 2
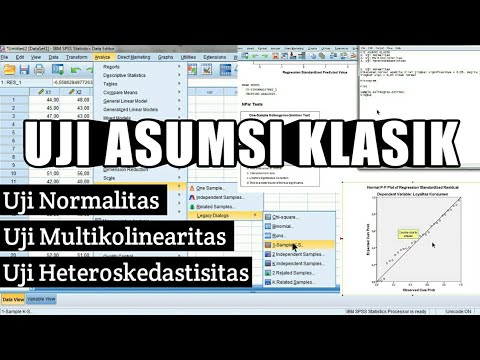
Tutorial SPSS Uji Asumsi Klasik : Uji Normalitas, Uji Multikolinearitas dan Uji Heteroskedastisitas
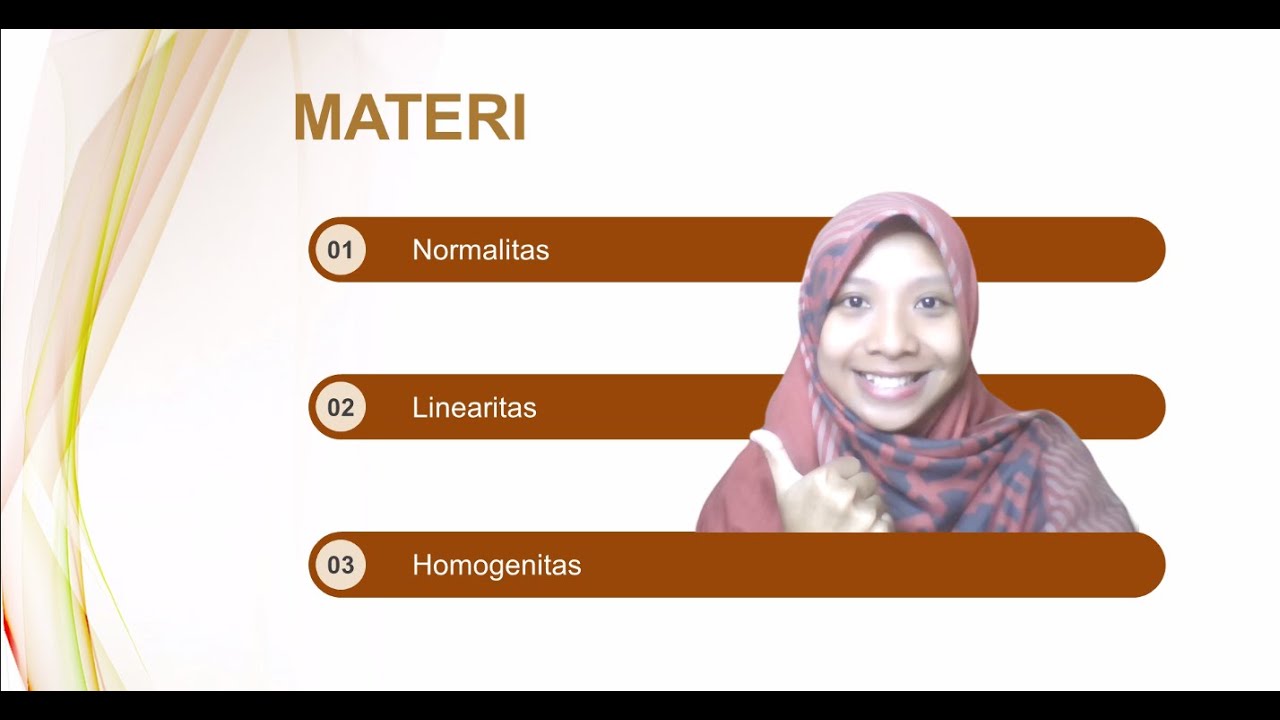
TENTANG UJI ASUMSI (NORMALITAS, LINEARITAS, HOMOGENITAS)
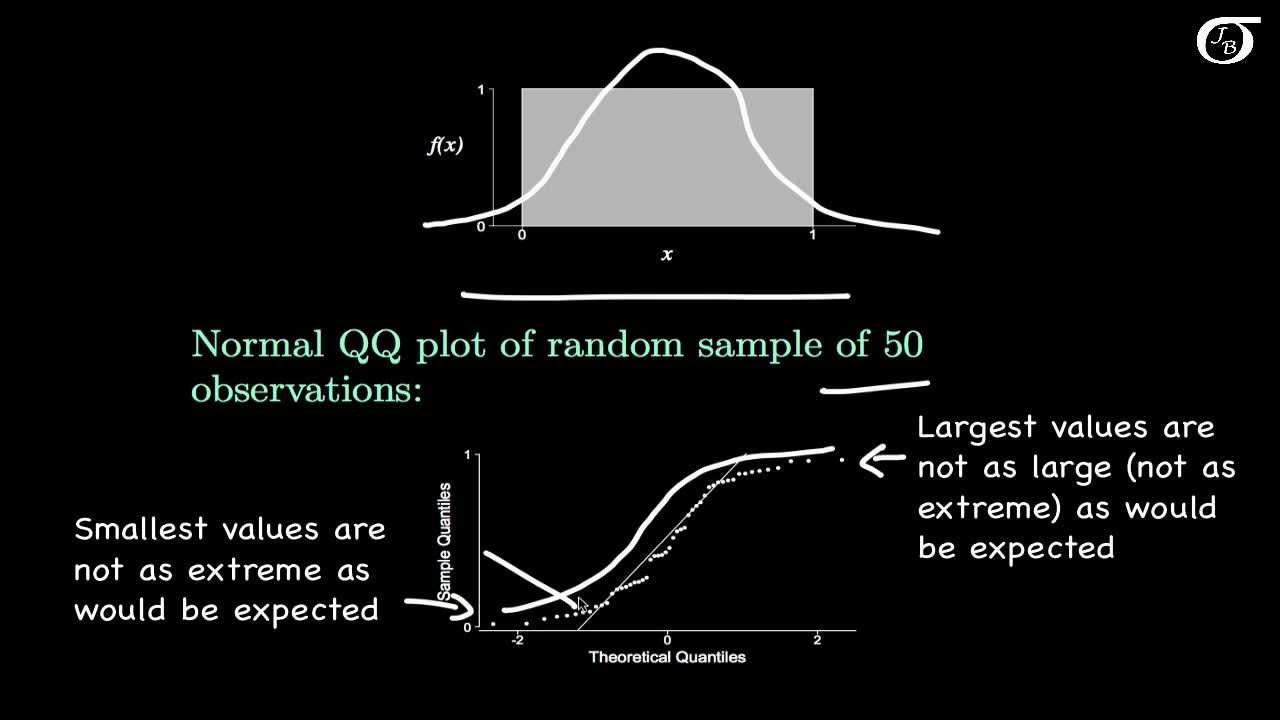
Normal Quantile-Quantile Plots
5.0 / 5 (0 votes)